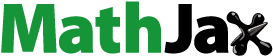
Abstract
This study expands previous research by adding intellectual capital to the capital asset pricing model and deepening the measurement of intellectual capital using more comprehensive proxies. This study is novel in that it is related to evaluation according to market developments using tests on potential factors in the asset pricing model by adding intellectual capital variables. Intangible assets have the explanatory and predictive power of size and value strategies in predicting returns. This study uses a sample of all industries from all companies listed on the Indonesia Stock Exchange during 2012–2022; the sample totals 5767 firm-years. The results show a significant relationship between the six-factor capital asset pricing model, intellectual capital, and excess stock returns. The robustness test supports these findings, suggesting that intellectual capital performs better financially as an intangible asset. This study contributes to the literature regarding the role of intellectual capital in the capital asset pricing model, which can be helpful when making investment decisions in emerging stock markets.
Public Interest Statements
This study adds to the body of knowledge about the role of intellectual capital in the capital asset pricing model (CAPM). It examines how intellectual capital is affected by the six-factor model plus intellectual capital on CAPM and by the use of companies listed on the Indonesia Stock Exchange. This study has proven a significant relationship between the six-factor CAPM plus intellectual capital and excess stock returns. This result is supported by the robustness test results, which confirm the main results. This confirmation indicates that as an intangible asset, intellectual capital has better financial performance. This study brings new opportunities to enrich the literature on the role of intellectual capital in the CAPM, which can be used when making investment decisions in emerging stock markets.
1. Introduction
This current study develops previous studies on the capital asset pricing model (CAPM) conducted by Fama and French (Citation2018) as well as Foye and Valentinčič (Citation2020). They estimate the CAPM using market premium, market capitalization factor (SMB), book-to-market factor ratio (HML), operating profitability, investment, and momentum. This current research expands the previous studies by adding intellectual capital to the capital asset pricing model. The interesting issue of this current study lies in the previous research’s findings on additional intellectual capital variables in the CAPM. The addition of intangible assets has the explanatory and predictive power of size and value strategies to predict returns (Roy & Shijin, Citation2018a). However, this current study is different from the previous studies because it adds intellectual capital to the six-factor capital asset pricing model. The research gap of this study is based on the fact that previous studies on CAPM obtained mixed results. Several studies show that the six-factor model can explain market returns (Cakici et al., Citation2013; Foye & Valentinčič, Citation2020). On the other hand, another study confirms that the six-factor cannot explain market returns (Ayub et al., Citation2020). The results of the earlier studies are contradictory so that this current study is inspired to investigate how the six-factor plus intellectual capital model could affect the CAPM. This study answers the research gap by including additional intellectual capital as one of the factors in CAPM with the intellectual capital to deduce the relationship model. Intellectual capital is based on the resource-based view theory stating that companies with many intangible assets have better financial performance and intellectual capital is related to investment decision-making (Farooq et al., Citation2022; Widiatmoko et al., Citation2020).
Furthermore, this study adds an explanation of intellectual capital measurement by implementing a comprehensive proxy. Referring to the research by Lin et al. (Citation2014), this current study adopted more detailed data on intellectual capital, such as human capital, customer capital, innovation capital, and procedure capital, to estimate the influence of each component of intellectual capital news on a firm’s stock returns and to examine different reactions to each component of intellectual capital. The measurement of intellectual capital in the CAPM employed by this study is the modified value added-intellectual coefficient (M-VAIC) proxy. Calculations with M-VAIC can indicate the ability of intellectual capital to create corporate values (Laing et al., Citation2010; Ulum et al., Citation2017). The novelty of this study is related to market development-based evaluation using tests on potential factors in the asset pricing model that adds intellectual capital variables as intangible assets. These variables have the explanatory and predictive power of size and value strategies to predict returns.
Market-based accounting research (MBAR) continues to evolve and adapt to current issues related to capital market phenomena as a result of the developments from various expansion phenomena in the previous research (Beaver, Citation1982; Lev & Ohlson, Citation1982). Furthermore, investment in the capital market is an interesting phenomenon to study. MBAR is relevant to studies of capital markets, the capital asset pricing model, capital market information, and capital market regulations (Lev & Ohlson, Citation1982). Investors can use the CAPM method to estimate stock portfolios (Ayub et al., Citation2020). Importantly, the CAPM model should be continuously evaluated according to market developments by using tests on potential factors in the asset pricing model (Harvey & Liu, Citation2021). CAPM has identified several potential factors in the asset pricing model carried out by various researchers.
The development of asset pricing has been conducted by Sharpe (Citation1964)who define CAPM. Furthermore, Fama and French (Citation1993) criticize that market beta cannot explain market returns, and they introduce a three-factor model, which constitutes a multi-factor model developed to respond to anomalies found when testing the CAPM. These anomalies include a market premium, market capitalization factor (SMB), and book-to-market ratio factor (HML). Fama and French (Citation2015) add operating profitability and investment factors to the five-factor model. This addition is based on two reasons. First, operating profitability reflects the tendency of stocks with high profitability to be superior to stocks with low profitability. Second, the investment factor reflects the tendency of stocks with low investment to be superior to stocks with high investment.
Study about the CAPM factor is still carried out with anomaly and international testing of the five-factor model to more significantly explain anomalies and improve the description of international stock market returns (Fama & French, Citation2016, Citation2017). Moreover, testing to find the best investor portfolio estimate continues to conduct. Fama and French (Citation2018) add a momentum factor to the five-factor model from the CAPM as an additional factor. Several previous studies have found that the weakness of the five-factor model with the additional momentum factor from the CAPM is its inability to explain excess returns (Azimli, Citation2020; Belimam et al., Citation2018; Fama & French, Citation2015, Citation2017; Khudoykulov & McMillan, Citation2020; Mosoeu & Kodongo, Citation2020; Sembiring, Citation2018). This condition occurs because the main problem with the model cannot capture the low average returns on small stocks whose returns behave like heavily invested firms despite low profitability. As a result, the model’s performance is not sensitive to the way its factors are defined.
The selection of intellectual capital as an explanation for CAPM is based on factors, such as human capital emerging in measuring the CAPM, developed in several previous studies (Barras, Citation2019; Park et al., Citation2021; Roy & Shijin, Citation2018b, Citation2019). Human capital is considered a valuable standard portfolio referred to by investors. In fact, CAPM’s human capital is a major concern for investors (Barras, Citation2019). One of the updates of this study is the addition of the intellectual capital variable. This is the reason why human capital is one component of intellectual capital. Therefore, intellectual capital is a comprehensive variable to describe human capital, which is an important resource for creating corporate values (Sardo & Serrasqueiro, Citation2017). This current study adds other variables by referring to previous research, which aims to measure variation in return predictability; return predictability is a primary concern for investors (Barras, Citation2019; Park et al., Citation2021; Roy, Citation2021; Roy & Shijin, Citation2018b, Citation2019). The motivation of this study is based on the need to expand factors that affect the CAPM so that investors can calculate non-diversifiable risks in a portfolio and compare the risks with the predicted rate of return.
Characteristics of the capital market in Indonesia is a weak form (Worthington & Higgs, Citation2006; Yang & Pangastuti, Citation2016). It is interesting to analyze how the six-factor plus human capital CAPM analysis and excess stock returns in Indonesia provide an overview of the capital market, which reflects emerging markets. This current study contributes to expanding the research conducted by Fama and French (Citation2018) as well as Foye and Valentinčič (Citation2020). This current study adds intellectual capital to the CAPM and deepens the measurement of intellectual capital by using a more comprehensive proxy in the capital market with a weak form. This study successfully shows that the six-factor CAPM plus intellectual capital is more relevant to understand the relationship between risk and return in asset predictability. This study contributes to trigger investors’ attention to intangible asset factors when making investment decisions.
These empirical results can assist financial advisors to determine risks in the market. Investors can comprehensively consider the six-factor in the CAPM and intellectual capital before investing. The M-VAIC proxy for intellectual capital measurement can estimate the influence of intellectual capital components, estimate the influence of each component of intellectual capital news on a firm’s stock returns, and examine different reactions to each component of intellectual capital. The global recession phenomenon predicted by experts has encouraged the Financial Services Authority (OJK) to make 35 policies to develop the economy, including the capital market sector. However, in reality, the regulator has not passed a policy on providing literacy of CAPM for investors. The results of this study can provide recommendations for regulators so that investors can be more aware of the CAPM calculation by using factors that influence investment decision-making. The massive literacy of CAPM about the investment climate in Indonesia is expected to improve investors’ good understanding of investment in the capital market.
The rest of the workflow is as follows. Section 1 presents a literature review of the theory and development of the multi-factor CAPM. Section 2 specifics the developed hypotheses. Section 3 presents the research design of variables, data, and methods. Section 4 reports the empirical results and discussion. Finally, Section 6 summarizes and concludes the study.
2. Literature review
This study refers to the prospect theory proposed by Kahneman et al. (Citation1979), who assert that several risks must be considered when making investments; for example, determining how prospects are perceived, determining how the valuation principle governing the evaluation of advantages and disadvantages, and assessing uncertain outcomes. The capital asset pricing model (CAPM) has been a dominant paradigm in financial markets for pricing risky assets since the early days of prospect theory. According to the prospect theory, four factors influence investor behavior: reference dependence, loss aversion, diminishing sensitivity, and probability weighting (Gregoriou et al., Citation2019). The most important aspect of this theory is how investors perceive risk in their investments. Based on this, investors can use the six-factor plus intellectual capital calculation in the capital markets.
Many previous studies have discussed the development of the capital asset pricing model and defined the CAPM (Sharpe, Citation1964; Litner, 1965; Moosin, 1969). In contrast, Fama and French (Citation1993) criticize that market beta cannot explain market returns, and they introduce a three-factor model, which is a multi-factor model developed to respond to anomalies found when testing the CAPM; these anomalies are a market premium, market capitalization factor (SMB), and book-to-market ratio factor (HML). Fama and French (Citation2015) also add operating profitability and investment factor to the five-factor model. This addition is based on two reasons. First, operating profitability reflects the tendency of stocks with high profitability to be superior to stocks with low profitability. Second, the investment factor reflects the tendency of stocks with low investment to be superior to stocks with high investment. Research on the CAPM factor continues to conduct anomaly and international testing of the five-factor model to more significantly explain anomalies and improve the description of international stock market returns (Fama & French, Citation2016, Citation2017). Testing to find the best investor portfolio estimate continues to be carried out by considering investors’ preferences (Jin & Sui, Citation2022). Fama and French (Citation2018) added a momentum factor to the six-factor model from CAPM.
Several previous studies have tested the five-factor CAPM model in Indonesia and proven that the five-factor CAPM model can explain market returns (Foye & Valentinčič, Citation2020; Wijaya et al., Citation2018). Several previous studies still use the five-factor model in their testing. Meanwhile, other studies used multi-factor spanning tests (Azimli, Citation2020; Fama & French, Citation2018; Foye & Valentinčič, Citation2020; Roy, Citation2021), multiple linear regression (Wijaya et al., Citation2018), Gibbons-Ross-Shanken or GRS test (Harvey & Liu, Citation2021; Mosoeu & Kodongo, Citation2020), and the generalized method of moments (GMM) (Belimam et al., Citation2018; Cakici et al., Citation2013; Mosoeu & Kodongo, Citation2020; Racicot & Rentz, Citation2016; Roy, Citation2021) to test multi-factor on CAPM. Fama and French (Citation2018) analyze various versions of the performance of the six-factor model, and they add momentum to the six-factor model. The factors that include the regression show that the momentum factor adds explanatory power to the six-factor model. The previous studies on CAPM have obtained mixed results. Several studies show that the six-factor model can explain market returns (Cakici et al., Citation2013; Foye & Valentinčič, Citation2020). On the other hand, other studies confirm that the six-factor cannot explain market returns (Ayub et al., Citation2020). Such a condition motivates the researchers to conduct this investigation to help investors calculate the non-diversifiable risks in a portfolio and compare the risks with the predicted rate of return.
This study shows that the six-factor model performs well. Foye and Valentinčič (Citation2020) investigate the Indonesian stock market in the period of December 1996-May 2017. This current study adopted the methodology of Fama and French (Citation2015). However, this current study is different from the previous studies because it includes the momentum factor in the analysis but does not build a set of momentum-ordered portfolios. This current study evaluates and compares 16 models, ranging from the CAPM with the addition of SMB to the six-factor model. In addition, the three-factor and five-factor models produce large interceptions for all portfolio sets, and the five-factor model does not improve the three-factor model. Foye and Valentinčič (Citation2020) argue that Indonesia has properties, such as a privileged financial reporting environment and low quality of earnings, which contribute to research results. Cakici et al. (Citation2013) suggest that the size, value, and influence of momentum on 18 emerging markets are divided into three regions (Asia, Eastern Europe, and Latin America) in monthly stock data and in the period of January 1990-December 2011. Meanwhile, research by Cakici et al. (Citation2013) employed GMM-based test statistics to evaluate and rank the performance of different models. The generalized method of moments (GMM) is employed to serially test abnormal and autocorrelated error terms. The GMM results show that the level of statistical significance of GRS is strong for local factors, and most of the results use the US and global factors. Various previous studies have investigated ways to understand the key factors of CAPM so that investors can identify mistakes when investing and creating an investment portfolio (Chen et al., Citation2019).
Research related to the multi-factor CAPM continues. Roy and Shijin (Citation2018a) suggest that the risk of an asset is measured by the covariance of return on assets with returns on the aggregate market and human capital. Intertemporal and consumption-based CAPM with an extended version of the CAPM framework examines excess returns on Fama and France portfolios sorted by BE/ME-size and momentum across economies. Price factors frequently used in anomalous literature include Fama and French factors, momentum, dividend yields, bond market factors, thrift, aggregate market, and human capital. This study employed panel data from developing and developed countries, panel regression, IV-GMM with random effects, and PCA. This study has found that the aggregate market and human capital are the strongest predictors of return on assets across economies. In addition, the aggregate market, human capital, and savings are strong predictors of return on assets in developing countries while the aggregate market and human capital are the best predictors of return on assets in developed countries. Interestingly, human capital degrades the predictive ability of the Fama and French factors and becomes redundant along with momentum, dividend yield, and bond market factors. Research on the inclusion of human capital helps investors and academics of CAPM (Khan et al., Citation2022) estimate returns more accurately.
Several previous studies have tested the five-factor CAPM model in Indonesia and have revealed that the five-factor CAPM model can explain market returns with mixed results (Foye & Valentinčič, Citation2020; Wijaya et al., Citation2018). This study will test the six-factor CAPM model plus intellectual capital and employ multiple regressions with a robustness test using GMM to overcome the weaknesses of previous research.
3. Hypothesis development
Financial economics calculations are used to estimate the expected return on individual stocks. CAPM provides a straightforward and intuitive relationship between systematic risks and returns. In this case, the importance of CAPM components in explaining excess stock returns is required. The hypotheses of this study are developed by referring to the prospect theory proposed by Kahneman et al. (Citation1979), who argue that risks must be considered when making investments; for example determining how prospects are perceived, determining how the valuation principle governing the evaluation of advantages and disadvantages, and weighting uncertain outcomes. Gregoriou et al. (Citation2019) postulate that the capital asset pricing model (CAPM) has been a dominant paradigm in financial markets for pricing risky assets.
According to CAPM, the natural explanatory variable is the market premium (Fama & French, Citation2018), which can communicate the return. Moreover, the factor of market premiums strongly influences excess stock returns (Fama & French, Citation2017).
H1:
Market premium has a significant effect on excess stock returns.
Market capitalization is one of the components used in portfolio management to determine the size of a portfolio (Fama & French, Citation2017; Khudoykulov & McMillan, Citation2020). The previous study shows that market capitalization has a significant effect on a stock’s expected return (Fama & French, Citation2017).
H2:
Market capitalization has a significant effect on excess stock returns.
Previous research denotes that book-to-market has a significant impact on investment decision-making (Cakici et al., Citation2013; Khudoykulov & McMillan, Citation2020). This shows the importance of book-to-market as a component that can significantly impact excess stock returns.
H3:
Book-to-market has a significant effect on excess stock returns.
Operating profitability is a CAPM component and has been studied. Previous research has shown that operating profitability is an important factor in explaining average return (Fama & French, Citation2015, Citation2017; Foye & Valentinčič, Citation2020).
H4:
Operating profitability has a significant effect on excess stock returns.
Previous research has found that investment plays a role in explaining average return (Fama & French, Citation2017; Foye & Valentinčič, Citation2020). With the addition of investment factors, the CAPM’s value factor becomes redundant to describe average returns in the sample’s examination (Fama & French, Citation2015).
H5:
Investment has a significant effect on excess stock returns.
The momentum effect has an impact on the progress of the equity market (Cakici et al., Citation2013). As a result, momentum is an important CAPM component. Previous research has shown that momentum has a significant impact on explaining portfolio returns (Cakici et al., Citation2013; Fama & French, Citation2015).
H6:
Momentum has a significant effect on excess stock returns.
The results of previous research also show that the six-factor model on the CAPM has a significant effect on excess stock returns (Fama & French, Citation2018; Roy, Citation2021; Roy & Shijin, Citation2018b, Citation2019). Since the 1990s, the development of intellectual capital in several countries has caused the economy driven by innovation; such a condition has provided the momentum for intellectual capital to create competitive advantages (Do et al., Citation2022). It is predicted that the addition of intellectual capital to this research model enables companies with many intangible assets to have better financial performance and create a relationship between intellectual capital and investment decision-making (Farooq et al., Citation2022). This study adds intellectual capital by referring to the resource-based view theory. This theory postulates that companies with many intangible assets have better financial performance and can create a relationship between intellectual capital and investment decision-making (Farooq et al., Citation2022; Widiatmoko et al., Citation2020). This statement is proven by previous research, which has revealed a significant influence between investors’ intellectual capital and investment decision-making.
H7:
Intellectual capital has a significant effect on excess stock returns.
shows the empirical model in this study. There are 7 factors (market premium, market capitalization, book-to-market, operating profitability, investment, momentum, and intellectual capital) as independent variables with excess stock returns as the dependent variable.
4. Research design
4.1. Data
The analysis utilizes a sample of companies listed on the Indonesia Stock Exchange during the period from 2012 to 2022. The total number of companies included in this study amounted to 5767 firm-years. The sampling was conducted by subtracting from the total population of 5862 firm-years (show in Table ).
Table 1. Information sample Companies
The data of this study were collected from the OSIRIS and Bloomberg databases. Panel model regression was used to conduct the analysis. The regression model was estimated using the generalized least squares (GLS) panel method. It replaces the ordinary least squares (OLS), which suffer from two distinct issues: heteroscedasticity and autocorrelation. The STATA statistical tool was utilized to investigate the GLS panel estimation equations to resolve this issue. The robustness test used in this study employed the generalized method of moments (GMM). GMM can rank and evaluate the performance of various models as well as evaluate the economic and statistical performance of asset pricing models (Cakici et al., Citation2013; Roy, Citation2021).
Descriptive statistics, such as mean, median, maximum, minimum, and standard deviation of the research data, were calculated for this study. Descriptive statistic data were calculated to find out the condition of the data from the research variables. The results of the descriptive statistical calculations are in the form of nominal data, which are explained descriptively and based on the processed data.
4.2. Variable definition and measurement
4.2.1. Dependent variables
4.2.1.1. Excess stock return (Rit-Ft)
In this study, excess stock returns (Rit-Ft) refer to Fama and French (Citation2018) as well as Harvey and Liu (Citation2021). Excess stock returns are measured by reducing the monthly return portfolio with one month’s risk-free return. The formula of this calculation is as follows.
4.2.2. Independent variables
4.2.2.1. Market premium (Mkt)
This study employed return on market to measure the market premium (Cakici et al., Citation2013; Foye & Valentinčič, Citation2020).
4.2.2.2. Market capitalization (SMB)
In this study, market capitalization refers to the theory of Fama and French (Citation2018) and the theory of small minus big (SMB) that explains differences in the returns on portfolios of large and small stocks. These theories were employed to measure the size factor. Tests were conducted at SBM to classify companies based on the size of their share capitalization values. When companies were grouped, they were initially calculated based on the formula. Afterward, they were sorted, starting from companies with large capitalization (large market capitalization values) to companies with small capitalization values (Fama & French, Citation2015).
4.2.2.3. Book-to-market (HML)
This study refers to the theory of Fama and French (Citation2018). This theory uses the high minus low book-to-market equity (HML), which explains differences in returns from high and low B/M stock portfolios. This theory was employed to measure value factors. Meanwhile, B/M was calculated by comparing the book value with the company’s market value in the capital market. After that, companies that have high B/M were grouped with companies that have high and low B/M values (Fama & French, Citation2015).
4.2.2.4. Operating profitability (RMW)
This study refers to the theory of Fama and French (Citation2018). This theory uses the robust minus weak (RMW) to measure the profitability factor. The mechanism calculates the difference between returns on diversified stock portfolios with strong profitability and those with weak profitability for the t-period.
4.2.2.5. Investment (CMA)
This study uses CMA (conservative minus aggressive) in measuring the investment factor. First, the difference from asset growth is calculated (Azimli, Citation2020; Fama & French, Citation2015; Foye & Valentinčič, Citation2020).
Afterward, the difference in diversified portfolio returns on stocks of low and high-investment companies, called conservative and aggressive investments, for the t-period was calculated.
4.2.2.6. Momentum (UMD)
This study refers to Fama and French (Citation2018) and Carhart (Citation1997) using UMD (up minus down) in measuring the momentum factor. The calculation is an average of 12 months stock return (Ayub et al., Citation2020).
4.2.2.7. Intellectual capital (IC)
This study it refers to Laing et al. (Citation2010) and Ulum et al. (Citation2017) using MVAIC to measure intellectual capital factors. This calculation is as follows.
Human capital efficiency was calculated using the ratio of value-added and human capital. The calculation of human capital was from total salaries and wages.
Structural capital efficiency was calculated using the ratio of structural capital and value-added. The structural capital calculation was calculated by deducting value-added and human capital.
Relational capital efficiency was calculated by the ratio of relational capital and value-added. The relational capital was calculated with the company’s marketing costs data using the following formula.
Capital employed efficiency was calculated by value-added and capital employed. capital employed was calculated with the book value of total assets.
The full MVAIC formula is as follows.
Based on the conceptual framework and hypotheses in this study, the model specifications are as follows (description of variables see Table ).
Table 2. Description of variables
4.2.3. Control variable
4.2.3.1. Earnings per share (EPS)
This study employed earnings per share, which functions as a control variable. The use of earnings per share as a variable control is justified because the EPS is a component of firm-specific information (Loang & Ahmad, Citation2022).
4.2.3.2. Net profit margin (NPM)
This study employed the net profit margin (NPM) as a control variable. NPM is determined by fundamental factors and used as a control variable (Astuty, Citation2017).
5. Empirical results and discussion
5.1. Empirical results
The mean, standard deviation, minimum, and maximum values for each of the model variables are presented in . This study employed unbalanced panel data using the generalized least square (GLS) in testing. This study employed the random effect as the estimation method. The results of this study in denote that all results are intersecting with excess stock returns. shows that excess stock return (Rit-Ft), market premium, market capitalization (SBM), book-to-market (HML), operating profitability (RMW), and investment (CMA) have positive averages. In contrast, momentum (UMD) and intellectual capital (IC) have negative averages. All of the observation data indicate that they vary greatly. Operating profitability data (RMW) have the highest distribution, as evidenced by the highest standard deviation value.
Table 3. Descriptive statistics
The Pearson correlation results of this study are presented in Table . The results show no endogeneity problem in variables and no multicollinearity problem in the model. These Pearson correlation results are presented in the coefficient numbers as follows.
Table 4. Pearson correlations
Table shows the results of regression testing with three models. Model 1 is the outcome of a six-factor analysis, model 2 is the outcome of a six-factor plus intellectual capital analysis, model 3 is the outcome about a six-factor plus intellectual capital with the control variable analysis. Table shows that the test was conducted from the results of the estimation method of the random effect model. From Table in model 1 shows that market premium (Mkt), market capitalization (SBM), book-to-market (HML), operating profitability (RMW), investment (CMA), and momentum (UMD) have a significant effect on excess stock return (Rit-Ft). Overall, model 1 has a high goodness of fit (F = 862.22; p = 0.000). In addition, the variance of the excess stock return can be explained by a six-factor of 47.26%. These results are consistent with those of previous studies indicating that the six-factor model is a factor that comprehensively explains the capital asset pricing model (Fama & French, Citation2018; Roy, Citation2021).
Table 5. Regression results
Meanwhile, model 2 shows that market premium (Mkt), market capitalization (SBM), book-to-market (HML), operating profitability (RMW), investment (CMA), momentum (UMD), and intellectual capital (IC) have a significant effect on excess stock return (Rit-Ft). Model 2 has high goodness of fit (F = 769.60, p = 0.000). In addition, the variance of the excess stock return can be explained by a six-factor plus intellectual capital of 48.27%.
Model 2 is the core model of this study. This model shows that the market premium has a significant effect on excess stock returns with a p-value of 0.000 (H1 is accepted). These results are supported by Fama and French (Citation2017), who have found that the factor market premium is strongly associated with a significant effect on excess stock returns. The findings of this study show that market capitalization has a significant effect on excess stock returns with a p-value of 0.000 (H2 is accepted). These findings are consistent with those of previous research, which show that market capitalization has a significant effect on the calculation of expected returns of stocks (Fama & French, Citation2017; Khudoykulov & McMillan, Citation2020). This study has proven that book-to-market has a significant effect on excess stock returns with a p-value of 0.000 (H3 is accepted). This result is in line with previous research, which explains that book-to-market has a significant effect on decision-making investment (Cakici et al., Citation2013; Khudoykulov & McMillan, Citation2020). This study has proven that operating profitability has a significant effect on excess stock returns, with a p-value of 0.051 (H4 is accepted). This finding agrees with those of previous research, which shows that operating profitability is a factor that contributes to the explanation of average return (Fama & French, Citation2015, Citation2017; Foye & Valentinčič, Citation2020). This research has discovered a p-value of 0.025, indicating that investment has a significant effect on excess stock returns (H5 is accepted). This finding supports previous research by demonstrating that investment is a factor that contributes to the explanation of average return (Fama & French, Citation2017; Foye & Valentinčič, Citation2020). The result of the study shows that momentum has a significant effect on excess stock returns with a p-value of 0.056 (H6 is accepted). This result is supported by previous research, which proves that momentum has a significant effect on explaining portfolio returns to investors (Cakici et al., Citation2013; Fama & French, Citation2015). This study has revealed that intellectual capital has a statistically significant effect on excess stock returns with a p-value of 0.000 (H7 is accepted). This result is consistent with that of previous research showing that the six-factor model on the CAPM has a significant effect on excess stock returns (Fama & French, Citation2018; Roy, Citation2021; Roy & Shijin, Citation2018b, Citation2019). Since the 1990s, the development of intellectual capital in several countries has caused the economy driven by innovation; such a condition has provided the momentum for intellectual capital to create competitive advantages (Do et al., Citation2022). It is predicted that the addition of intellectual capital to this research model enables companies with many intangible assets to have better financial performance and create a relationship between intellectual capital and investment decision-making (Farooq et al., Citation2022).
Model 3 shows that market premium (Mkt), market capitalization (SBM), book-to-market (HML), operating profitability (RMW), investment (CMA), momentum (UMD), and intellectual capital (IC) with a control variable of EPS has a significant effect on excess stock return (Rit-Ft). However, the control variable of EPS has no significant effect on excess stock return (Rit-Ft). Model 3 has a goodness of fit (F = 705.05, p = 0.000). In addition, the variation in excess stock return can be explained by a six-factor plus intellectual capital and the control variable of 58.13%.
The constant term in the regression in Table is still statistically significant. This indicates that the factors are not fully capable of capturing the movement in returns. It is suggested that to address this issue, further research should be able to predict other factors that influence CAPM from the perspective of local or country-specific factors for better results. However, the overall results of model 1 and model 2 show that with the inclusion of the intellectual capital variable, there is an increase in adjusted R-squared by 0.0101, and with the inclusion of both the intellectual capital and control variables, there is an increase in adjusted R-squared by 0.0987. This suggests that in model 1, model 2, and model 3, all variables are significant, with the adjusted R-squared value increasing further due to the presence of the intellectual capital and control variables.
6. Discussion
This study has discovered that intellectual capital in the capital asset pricing model has a significant effect on excess stock returns of companies listed on the Indonesia Stock Exchange. Intellectual capital has a direct impact and is in line with the empirical theory, which emphasizes that aggregate wealth, both financial and human wealth, drives the pattern of stock returns (Roy, Citation2021). The results of statistical tests show that intellectual capital is considered in the risk and return relationship to predict excess stock returns. This result agrees with the prospect theory that investors can use financial information to make decisions. The results of this study prove that the six-factor plus intellectual capital in capital asset pricing is more relevant to understand the relationship between risk and return and to predict asset returns. These empirical results can assist financial advisors to determine risks in the market. Investors can comprehensively consider the six-factor in the CAPM and intellectual capital before investing.
The statistical results of the robustness test support the main test results of this study. The results indicate that variable of intellectual capital is robust and convincing as an explanatory variable for six factors plus intellectual capital in the capital asset pricing model. This result agrees with the results of research on six factors. The six-factor plus intellectual capital test has revealed that intellectual capital, an intangible asset, is related to investment decision-making based on the resource-based view theory (Farooq et al., Citation2022; Widiatmoko et al., Citation2020). The relationship between human capital, a part of intellectual capital, is in line with the empirical theory, which emphasizes that aggregate wealth, both financial and human wealth, drives the pattern of stock returns (Roy, Citation2021). This also indicates that intellectual capital is a company’s effort to increase its competitive advantage.
The results of this study prove that the six-factor plus intellectual capital in the capital asset pricing model is more relevant to understand the relationship between risk and return and to predict asset returns. These empirical results can assist financial advisors to determine risk in the market. Investors can comprehensively consider the six-factor in the CAPM and intellectual capital before investing. This study contributes to the literature on the role of intellectual capital in the CAPM, which is applicable when making investment decisions in emerging stock markets.
The M-VAIC measurement confirms that intellectual capital can estimate the influence of intellectual capital components, estimate the influence of each component of intellectual capital news on a firm’s stock returns, and examine different reactions to each component of intellectual capital (Lin et al., Citation2014). In this study, the intellectual capital in the CAPM was measured using the modified value-added intellectual coefficient (M-VAIC) proxy. The M-VAIC calculation can indicate the ability of intellectual capital to create corporate value (Laing et al., Citation2010; Ulum et al., Citation2017).
This study contributes to trigger investors’ attention to intangible asset factors when making investment decisions. Investors can use the right model to evaluate the performance of portfolio managers when making investment decisions (Azimli, Citation2020; Wijaya et al., Citation2021; Yuniningsih et al., Citation2020). In this study, an understanding of intellectual capital is emphasized as an important addition to the other six factors in the CAPM pricing model so that investors can get the best model for their investments. It is proven that intellectual capital has a direct impact on investors’ decisions.
This study has also discovered that the use of non-financial information, such as intellectual capital, in the emerging stock market, especially in Indonesia, integrates into the investment decision-making process. This integration occurs because the characteristics of emerging stock markets prioritize the sustainability of the company’s non-financial matters.
6.1. Robustness test
This study employed the generalized method of moments (GMM) for the robustness test. This study also employed a robustness test to see the consistency of the research results. A previous study by Cakici et al. (Citation2013) employed GMM used to evaluate and rank the performance of different models. GMM is also used to evaluate the economic and statistical performance of asset pricing models (Roy, Citation2021).
The robustness test was conducted using a sample of 5767 companies listed on the Indonesia Stock Exchange during the period of 2012–2022. In the GMM test, the T-1 period was employed in the data from the sample period. The data in this study were collected from the OSIRIS and Bloomberg databases. Meanwhile, the panel model regression was employed to conduct the analysis.
The results of the robustness test are presented in Table .
Table 6. Results of GMM Regression
The GMM test results support the main result of this study. The results show that market premium (Mkt), market capitalization (SBM), book-to-market (HML), operating profitability (RMW), investment (CMA), momentum (UMD), and intellectual capital (IC) have a significant effect on excess stock returns (Rit-Ft). The GMM test results on the six factors also support these significant results. This proves that the six factors in the emerging stock market have a significant effect (Roy & Shijin, Citation2019). Moreover, the addition of intellectual capital has a significant influence on the result.
7. Summary and conclusion
The results show that the six-factor plus intellectual capital test in the capital asset pricing model has a significant impact on excess stock returns in Indonesia. This result confirms that the novelty of this study is related to market development-based evaluation using tests on potential factors in the asset pricing model that adds intellectual capital variables, as intangible assets. These variables were selected because they have the explanatory and predictive power of size and value strategies to predict returns. This indicates that as an intangible asset, intellectual capital has better financial performance. This study contributes to the literature on the role of intellectual capital in the capital asset pricing model, which can be used to make investment decisions in emerging stock markets. The results of this study can provide recommendations for regulators so that investors can be more aware of the CAPM calculation by using factors that influence investment decision-making. The massive literacy of CAPM about the investment climate in Indonesia is expected to improve investors’ good understanding of investment in the capital market.
This study aims to expand research conducted by Fama and French (Citation2018) as well as Foye and Valentinčič (Citation2020) by adding the intellectual capital in CAPM. Moreover, this study aims to deepen the measurement of intellectual capital by using more comprehensive proxies. The novelty of this study is related to the market development-based evaluation using tests on potential factors of the asset pricing model that adds intellectual capital variables as the intangible asset. These variables were selected because they have the explanatory and predictive power of size and value strategies to predict returns. The sample of this research was 5767 companies listed on the Indonesia Stock Exchange during the period of 2012–2022. The data were collected from the OSIRIS and Bloomberg statistical testing in the main test using Generalized Least Square (GLS). Meanwhile, the robustness test employed the generalized method of moments (GMM) with the use of the estimation method of the random effect.
This study has some limitations that become an avenue for future research. First, this study is related to the unfair results of research when generalizing to other markets because the characteristics of each market in other countries vary. Further research can re-examine the use of six factors plus intellectual capital in the capital asset pricing model by using measurements suitable to the characteristics of each country. Moreover, further research can use additional regional factors related to specific regions. Second, this study employs proxies that refer to previous research. Future research can create a new proxy that can comprehensively describe financial and non-financial CAPM.
Acknowledgments
We would like to thank Beasiswa Pendidikan Indonesia (BPI) Puslapdik of the Ministry of Education, Culture, Research, and Technology Indonesia and LPDP for granting the scholarship.
Disclosure Statement
No potential conflict of interest was reported by the author(s).
Additional information
Notes on contributors
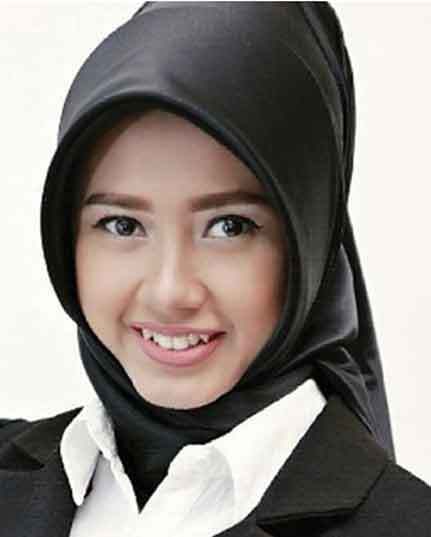
Astrid Maharani
Astrid Maharani is a PhD student at Faculty of Economics and Business, Universitas Airlangga, and a lecturer at at Faculty of Economics and Business, Universitas Muhammadiyah Jember, Indonesia. Her interest research is in the field of Capital Market, Intellectual Capital and Financial Accounting.
I Made Narsa
I Made Narsa is Professor at Faculty of Economics and Business, Universitas Airlangga, Indonesia. His interest research is in the field of Financial and Management Accounting.
References
- Astuty, P. (2017). The influence of fundamental factors and systematic risk to stock prices on companies listed in the Indonesian stock exchange. European Research Studies Journal, 20(4), 230–17. https://doi.org/10.35808/ersj/830
- Ayub, U., Kausar, S., Noreen, U., Zakaria, M., & Jadoon, I. A. (2020). Downside risk-based six-factor capital asset pricing model (CAPM): A new paradigm in asset pricing. Sustainability (Switzerland), 12(17), 1–16. https://doi.org/10.3390/SU12176756
- Azimli, A. (2020). Pricing the common stocks in an emerging capital market: Comparison of the factor models. Borsa Istanbul Review, 20(4), 334–346. https://doi.org/10.1016/j.bir.2020.05.002
- Barras, L. (2019). A large-scale approach for evaluating asset pricing models. Journal of Financial Economics, 134(3), 549–569. https://doi.org/10.1016/j.jfineco.2019.05.007
- Beaver, W. H. (1982). Discussion of market-based empirical research in Accounting: A Review, interpretation, and extension. Journal of Accounting Research, 20, 323. https://doi.org/10.2307/2674686
- Belimam, D., Tan, Y., & Lakhnati, G. (2018). An empirical comparison of asset-pricing models in the shanghai A-Share Exchange market. Asia-Pacific Financial Markets, 25(3), 249–265. https://doi.org/10.1007/s10690-018-9247-4
- Cakici, N., Fabozzi, F. J., & Tan, S. (2013). Size, value, and momentum in emerging market stock returns. Emerging Markets Review, 16, 46–65. https://doi.org/10.1016/j.ememar.2013.03.001
- Carhart, M. M. (1997). On persistence in mutual fund performance. The Journal of Finance, 52(1), 57–82. https://doi.org/10.1111/j.1540-6261.1997.tb03808.x
- Chen, L., Pelger, M., & Zhu, J. (2019). Deep Learning in asset pricing. SSRN Electronic Journal. https://doi.org/10.2139/ssrn.3350138
- Do, M. H., Thanh Tam, V., & Kim-Duc, N. (2022). Investigating intellectual capital: The role of intellectual property rights reform. Cogent Economics & Finance, 10(1). https://doi.org/10.1080/23322039.2022.2106630
- Fama, E. F., & French, K. R. (1993). Common risk factors in the returns on stocks and Bonds. Journal of Hepatology, 18(1), 3–56. https://doi.org/10.1016/0304-405X(93)90023-5
- Fama, E. F., & French, K. R. (2015). A five-factor asset pricing model. Journal of Financial Economics, 116(1), 1–22. https://doi.org/10.1016/j.jfineco.2014.10.010
- Fama, E. F., & French, K. R. (2016). Dissecting anomalies with a five-factor model author (s): Eugene F. Fama and Kenneth R. French Published by: Oxford University Press. Sponsor: The society for financial studies. Dissecting Anomalies with a Five-Factor Model, 29(1), 69–103. https://doi.org/10.1093/rfs/hhv043
- Fama, E. F., & French, K. R. (2017). International tests of a five-factor asset pricing model R. Journal of Financial Economics, 123(3), 441–463. https://doi.org/10.1016/j.jfineco.2016.11.004
- Fama, E. F., & French, K. R. (2018). Choosing factors. Journal of Financial Economics, 128(2), 234–252. https://doi.org/10.1016/j.jfineco.2018.02.012
- Farooq, U., Tabash, M. I., Anagreh, S., & Khudoykulov, K. (2022). How do market capitalization and intellectual capital determine industrial investment? Borsa Istanbul Review, 22(4), 828–837. https://doi.org/10.1016/j.bir.2022.05.002
- Foye, J., & Valentinčič, A. (2020). Testing factor models in Indonesia. Emerging Markets Review, 42(April 2019), 100628. https://doi.org/10.1016/j.ememar.2019.100628
- Gregoriou, A., Healy, J. V., & Le, H. (2019). Prospect theory and stock returns: A seven factor pricing model. Journal of Business Research, 101(June 2018), 315–322. https://doi.org/10.1016/j.jbusres.2019.04.038
- Harvey, C. R., & Liu, Y. (2021). Lucky factors. Journal of Financial Economics, 141(2), 413–435. https://doi.org/10.1016/j.jfineco.2021.04.014
- Jin, L. J., & Sui, P. (2022). Asset pricing with return extrapolation. Journal of Financial Economics, 145(2), 273–295. https://doi.org/10.1016/j.jfineco.2021.10.009
- Kahneman, D., Tversky, A., & Tversky, A. (1979). Prospect theory: An analysis of decision under risk. Source: Econometrica, 47(2), 263–292. https://doi.org/10.2307/1914185
- Khan, N., Zada, H., & Yousaf, I. (2022). Does premium exist in the stock market for labor income growth rate? A six-factor-asset-pricing model: Evidence from Pakistan. Annals of Financial Economics, 17(3), 2250017. https://doi.org/10.1142/S2010495222500178
- Khudoykulov, K., & McMillan, D. (2020). Asset-pricing models: A case of Indian capital market. Cogent Economics & Finance, 8(1), 1832732. https://doi.org/10.1080/23322039.2020.1832732
- Laing, G., Dunn, J., & Hughes‐Lucas, S. (2010). Applying the VAICTM model to Australian hotels. Journal of Intellectual Capital, 11(3), 269–283. https://doi.org/10.1108/14691931011064545
- Lev, B., & Ohlson, J. A. Market-based empirical research in Accounting: A Review, interpretation, and extension source. (1982). Journal of Accounting Research, 20(1982), 249–322. Journal of Accounting Research, Vol. 20, Supplement : Studies on Current Research Methodologies in Accounting : A Critical Evaluation (1982), pp. 249-. https://doi.org/10.2307/2674685
- Lin, Y. M., Lee, C. C., Chao, C. F., & Liu, C. L. (2014). The information content of unexpected stock returns: Evidence from intellectual capital. International Review of Economics and Finance, 37, 208–225. https://doi.org/10.1016/j.iref.2014.11.024
- Loang, O. K., & Ahmad, Z. (2022). Market overreaction, firm-specific information and macroeconomic variables in US and Chinese markets during COVID-19. Journal of Economic Studies, 49(8), 1548–1565. https://doi.org/10.1108/JES-10-2021-0543
- Mosoeu, S., & Kodongo, O. (2020). The Fama-French five-factor model and emerging market equity returns. The Quarterly Review of Economics & Finance. https://doi.org/10.2139/ssrn.3377918
- Park, D., Eom, Y. H., & Hahn, J. (2021). Evaluating the conditional capm using consumption-based state variables: Evidence from the Korean stock market. Korean Journal of Financial Studies, 50(3), 339–367. https://doi.org/10.26845/KJFS.2021.06.50.3.339
- Racicot, F. E., & Rentz, W. F. (2016). Testing Fama–French’s new five-factor asset pricing model: Evidence from robust instruments. Applied Economics Letters, 23(6), 444–448. https://doi.org/10.1080/13504851.2015.1080798
- Roy, R. (2021). A six‐factor asset pricing model: The Japanese evidence. Financial Planning Review, 4(1). https://doi.org/10.1002/cfp2.1109
- Roy, R., & Shijin, S. (2018a). Dissecting anomalies and dynamic human capital: The global evidence. Borsa Istanbul Review, 18(1), 1–32. https://doi.org/10.1016/j.bir.2017.08.005
- Roy, R., & Shijin, S. (2018b). A six-factor asset pricing model. Borsa Istanbul Review, 18(3), 205–217. https://doi.org/10.1016/j.bir.2018.02.001
- Roy, R., & Shijin, S. (2019). Is human capital the sixth factor? Evidence from US data. ACRN Journal of Finance and Risk Perspectives, 8(1), 21–55. https://doi.org/10.35944/jofrp.2019.8.1.002
- Sardo, F., & Serrasqueiro, Z. (2017). A European empirical study of the relationship between firms’ intellectual capital, financial performance and market value. Journal of Intellectual Capital, 18(4), 771–788. https://doi.org/10.1108/JIC-10-2016-0105
- Sembiring, F. M. (2018). Three-factor and five-factor models: Implementation of Fama and French model on market overreaction conditions. GATR Journal of Finance and Banking Review, 3(4), 77–83. https://doi.org/10.35609/jfbr.2018.3.4(6)
- Sharpe, W. F. (1964). Capital asset prices: A theory of market equilibrium under conditions of risk. The Journal of Finance, 19(3), 425–442. https://doi.org/10.1111/j.1540-6261.1964.tb02865.x
- Ulum, I., Kharismawati, N., & Syam, D. (2017). Modified value-added intellectual coefficient (MVAIC) and traditional financial performance of Indonesian biggest companies. International Journal of Learning and Intellectual Capital, 14(3), 207–219. https://doi.org/10.1504/IJLIC.2017.086390
- Widiatmoko, J., Indarti, M. G. K., Pamungkas, I. D., & Ntim, C. G. (2020). Corporate governance on intellectual capital disclosure and market capitalization. Cogent Business & Management, 7(1), 1750332. https://doi.org/10.1080/23311975.2020.1750332
- Wijaya, L. I., Kennardi Irawan, R., & Anom Mahadwartha, P. (2018). Test of Fama and French Five Factor-Model on Indonesian Stock Market. 186(March). https://doi.org/10.2991/insyma-18.2018.12.
- Wijaya, L. I., Narsa, I. M., Irwanto, A., & Setiawan, R. (2021). Are investors’ desires for dividend increases stronger than dividend initiation? International Journal of Trade and Global Markets, 14(6), 559–579. https://doi.org/10.1504/IJTGM.2021.118900
- Worthington, A. C., & Higgs, H. (2006). Weak-form market efficiency in Asian emerging and developed equity markets: Comparative tests of random walk behaviour. Accounting Research Journal, 19(1), 54–63. http://ro.uow.edu.au/cgi/viewcontent.cgi?article=1122&context=commpapers
- Yang, A. S., & Pangastuti, A. (2016). Stock market efficiency and liquidity: The Indonesia stock Exchange merger. Research in International Business and Finance, 36, 28–40. https://doi.org/10.1016/j.ribaf.2015.09.002
- Yuniningsih, Y., Ismiyanti, F., Narsa, I. M., Mawardi, A. I., & Suhada, B. (2020). Risk taking investment as the interaction effect of loss aversion and information (Pilot test). Quality - Access to Success, 21(175), 107–112. https://www.scopus.com/inward/record.uri?eid=2-s2.0-85085177773&partnerID=40&md5=828689392aa46df55b2d31dc063b6d50