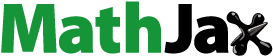
Abstract
This study examines the determinants of time-varying return volatility of Africa’s equity markets using monthly indices of eight top African stock markets. The conditional variance is modelled as a proxy for Africa’s volatility indices using the best fitting model among SGARCH, EGARCH and GJR-GARCH. In a bid to account for the dynamic and persistent nature of volatility, the least squares dummy variable bias correction (LSDVC) model is employed. The study finds that volatility of African stock markets follows a dynamic process and is explained by past volatility, domestic exchange rates, Treasury bill rates, money supply, inflation rates, movements in world crude oil prices, volatility of the US and UK stock markets and COVID-19 shocks. The results are largely robust to sub-sample analysis. Further analysis: pre-, during and post-crisis periods (Global Financial Crisis (GFC) and COVID-19 pandemic) reveal that (1) African stock markets became sensitive to advanced market volatility in the period during the GFC and have remained sensitive in the post GFC period and (2) past domestic market return volatility, Treasury bill rates and exchange rates have been the main drivers of stock return volatility during the COVID-19 pandemic in Africa. Additionally, markets in North Africa are revealed as the only African markets immune to advanced market volatility spillovers. These markets may thus provide international diversification opportunities for portfolio managers.
1. Introduction
The relevance of comprehending and adequately modeling financial risk has—again—become evident during the market turbulences in recent years. Significant surges in equity market volatility during recent major episodes like the COVID-19 pandemic, Russia–Ukraine war and the global financial crisis have reignited intense interest in examining equity market volatility to identify its nature and major drivers (Baker et al., Citation2020; Umar et al., Citation2022). Accurate volatility predictions for asset prices and returns are crucial when projecting risk measures, such as Value-at-Risk (VaR) or expected shortfall, that are commonly used in risk assessment, the design of risk-mitigation strategies, and for regulatory purposes. Discussions on equity market volatility are mainly two-fold. First, contemporary financial theory asserts that stock return volatility is closely related to the movements of macroeconomic variables (Ferson & Harvey, Citation1991; Schwert, Citation1989). This is because the stock market reflects fundamental information about the macroeconomy. Another approach to explain equity return volatility underscores the linkages between volatility in one market (or a group of markets) and international economic environments such as world oil prices, interest rates or stock prices in developed countries. Eun and Shim (Citation1989) reported the transmission of the innovations in the US stock market to the rest of the world. Hamao et al. (Citation1990) found that price volatility spills over across markets. The extant literature therefore suggests that equity return volatility can be domestically or externally sourced. Identifying the key domestic and global factors that drive volatility is crucial for policymakers, macroeconomists, investors, financial analysts and other market participants. Financial analysts and investors, for instance, wish to understand the trends of volatility in financial securities and which events could alter and influence the persistence of volatility overtime (King & Botha, Citation2015). Governments and policymakers wish to predict the business cycle and economic growth (Carlston, Citation2018) and formulate policies that guarantee financial and macroeconomic stability (Abbas et al., Citation2018). These policies will enhance market rules and regulations and predict an economy’s growth path (Guru & Yadav, Citation2019; Poon & Granger, Citation2003; Suhaibu et al., Citation2017). Besides, the sensitivity of an equity market’s volatility to happenings in the international economic environment has relevance for the price discovery process, macroeconomic policies, cross-market diversification benefits, and the vulnerability of global financial markets to shocks from other countries (Bekaert et al., Citation2002; Boamah et al., Citation2020; Büttner & Hayo, Citation2011; Prasad et al., Citation2018; Stulz, Citation1999).
While studies that examine how financial market volatility is influenced by only domestic factors or only global factors exist in the extant literature, we know very little about the collective impact of such factors on the time-varying volatility of indices in financial markets. In particular, studies applying dynamic panel analysis within the least square dummy variable bias corrected (LSDVC) model to identify the key domestic and global factors driving return volatility across international financial markets are non-existent to date. The preponderance of studies analysed the dynamic relationship between stock market indices and domestic macroeconomic fundamentals at the first moment using vector autoregressive (VAR) models (see, for instance, Camilleri et al., Citation2019; Diaz et al., Citation2016; Fama, Citation1981; Gwahula, Citation2018; Ndlovu et al., Citation2018; Sadorsky, Citation1999; Vuyyuri, Citation2005), and the link between stock return volatility and domestic macroeconomic fundamentals at the second moments using volatility models such as the GARCH family of models (see for instance Abbas et al., Citation2018; Bagchi, Citation2017; Cheng & Yip, Citation2017; Forbes & Rigobon, Citation2002; Hamao et al., Citation1990; Koutmos & Booth, Citation1995; Kumari & Mahakud, Citation2015; Li & Majerowska, Citation2008; Peiró, Citation2016; Scheicher, Citation2001; Su et al., Citation2019). Other contributions highlighted the importance of foreign stock market volatility in explaining the volatility of domestic stock markets (see, for instance, Alagidede et al., Citation2011; Alfreedi, Citation2019; Chaudhuri & Koo, Citation2001; Choudhry et al., Citation2016; Mensah & Alagidede, Citation2017; Prasad et al., Citation2018; Umar et al., Citation2022). Although the extant literature provides evidence supporting the relevance of either domestic factors or external factors to equity return volatility, only a smattering of studies have investigated the collective impact of these factors on return volatility. The failure of most related studies to contemporaneously include both factors in models seeking to explain market volatility amounts to omission of relevant variables possibly resulting in incorrectly specified models, biased model estimates and spurious inferences (Wilms et al., Citation2021). Perhaps, the only studies that come close to the current study in simultaneously assessing the impact of domestic and global factors on return volatility are Prasad et al. (Citation2018) for developed and emerging markets in Asia Pacific, Americas and Europe and Chaudhuri and Koo (Citation2001) for emerging markets in Asia. Nonetheless, these studies employed the fixed effects model and VAR model, respectively, not the dynamic panel model used in the current study. The dynamic panel model employed in this current study is estimated using the LSDVC which corrects for bias ignored in closely related extant studies. Also, the significant differences in development, liquidity, efficiency and other microstructural characteristics that exist between Africa’s mostly frontier markets and other emerging and developed markets suggest that Africa’s equity markets present a unique case for re-investigating domestic and global drivers of equity market volatility.
Moreover, several equity markets worldwide have since the advent of the COVID-19 pandemic experienced varying degrees of volatility possibly induced by the pandemic’s attendant uncertainties. The FTSE 100 index and Dow Jones Industrial Average, for instance, experienced their worst quarterly plunges since 1987, falling by 25% and 23%, respectively, as reported by the BBC on 31 March 2020. In like fashion, the S&P 500 also experienced its worst drop since the global financial crisis (GFC) of 2007–2009, falling by some 20%. In Africa, the narrative is no different. Market indices including JSE/FTSE in South Africa, EGX 30 in Egypt, MASI in Morocco, NSE 20 in Kenya, NGSEINDEX in Nigeria, GSE-CI in Ghana all tumbled in wake of the pandemic, plunging by 10%, 24%, 21%, 25%, 12% and 12%, respectively, by May 2020. The COVID-19 pandemic thus seems to present a novel source of volatility to equity markets. Assessing the impact of the pandemic on US markets, Baker et al. (Citation2020) report that volatility increases in US markets attributable to COVID-19 are unprecedented and significantly exceed those recorded for other health-related events such as Ebola in 2015, SARS in 2003 and 1918’s global influenza. Similarly, Uddin et al. (Citation2021) find that the COVID-19 pandemic exacerbated market volatility in 34 developed and emerging markets. Clearly, the scant conversation on Covid-19’s impact on return volatility has so far been focused only on developed and emerging markets much to neglect of frontier markets. This is regardless of the fact that capital flows to frontier markets have been on the ascendancy over the years and even gained momentum after the GFC as declining interest rates in most developed economies prompted investors to turn to frontier markets for superior return opportunities (Atenga & Mougoué, Citation2021; Chen & Zhang, Citation1998; Goriaev, Citation2004). The question of how the COVID-19 pandemic affected return volatility of frontier markets therefore remains relevant as evidence from emerging and developed markets cannot necessarily be generalized to cover frontier markets due to significant institutional differences among these market categories. The relatively high economic uncertainty, political risk, illiquidity, non-transparency and low regulatory quality in frontier markets compared to their developed and emerging market counterparts may have positioned them in a place of higher susceptibility to COVID-19 induced shocks. This study extends the conversation by investigating Covid-19’s impact on Africa’s equity markets, most of which except South Africa and Egypt are classified as frontier markets. Considering that Africa has till date recorded lower COVID-19 cases and deaths relative to other regions, the question of whether lower severity had only marginal or no impact on return volatility remains valid.
This study builds on prior international financial market studies and examines contemporaneously domestic and global factors affecting the time-varying volatility of returns in Africa’s stock markets within a dynamic panel framework. Covid-19’s impact on equity return volatility in Africa’s markets is also examined. Further, the nature and persistence of return volatility spillovers across these equity markets are assessed. The approach enables us to explore not only the time-varying volatility and the link between stock return volatility and the macro-economies of these markets but more importantly, to find out key local (within market), regional and global variables that drive Africa’s stock return volatility. The analysis of return volatility in financial markets is very important for asset pricing, risk management, and fund allocation (Abbas et al., Citation2018). Such analyses are even more desirable in emerging and frontier equity markets, especially in Africa, due to their unique features and the fact that these markets are presently appealing to the global investing community. Within the context of a crisis laden global economy induced by the COVID-19 pandemic and more recently by the Russia–Ukraine war, analyses of this nature are timely.
Understanding the local, regional and global sources of stock market volatility is important to investors, fund managers, financial markets regulators, and policymakers. From the perspective of global investors and fund managers, such understanding shares insights into the nature of volatility patterns and, in particular, events that can influence stock return volatility, thereby facilitating their diversification and investment decisions. From a policy and regulation standpoint, understanding the drivers of the time-varying volatility of stock returns will provide useful insights on the transmission of shocks (such as crises, diseases, natural disasters, and wars), potential capital flights during such abnormal periods, and the appropriate policy responses to globalisation and financial integration.
This paper provides evidence of the time-varying return volatility of Africa’s emerging and frontier markets and their determinants. The study provides evidence that, without considering regional differences and time-varying effects, the return volatility of African stock markets is driven by domestic factors, global factors (including world oil prices and advanced market volatility) and more recently by COVID-19 related shocks. Evidence is provided further that, when time-varying effects are accounted for, the return volatility of Africa’s stock markets is driven only by previous period domestic market volatility in the period before the GFC. Identical domestic macroeconomic factors together with advanced market volatility and world oil price movements drive volatility in Africa’s equity markets in the period during and after the GFC. Evidence is also provided that, accounting for regional differences, the stock indices of southern, west and east Africa display that domestic factors as well as global market volatility and oil price shocks significantly determine their return volatility. Stock indices in North Africa are identified as the only ones immune to advanced market volatility shocks and largely driven by domestic macroeconomic uncertainty and oil price movements. Also, markets in western Africa show some resilience to COVID-19 related shocks as markets in all other regions (east, north and southern) experience an exacerbation of return volatility after the advent of the pandemic.
The remainder of the paper is as follows. Section 2 presents the related literature, whilst Section 3 deals with the methodology and data of the study. The empirical results are presented in Section 4, with Section 5 presenting the conclusion of the study.
2. Related literature
Fama (Citation1970), in his famous efficient market hypothesis, asserts that securities prices are influenced by information—such as firm-level information, earnings announcements, macroeconomic performance, and global news. Also, under the asset pricing theory, Sharpe (Citation1964) and Ross (Citation1976) argue that prices and returns of financial assets are influenced by their risk exposures. Similarly, Markowitz’s (Citation1952) portfolio theory and Fama and French (Citation2004) highlight the link between risk and expected returns of assets. Geske and Roll (Citation1983), Chen et al. (Citation1986), Schwert (Citation1989) and Fama (Citation1981, Citation1990) provide initial evidence on the nexus between economic activity and stock market behaviour. Specifically, Estrella and Mishkin (Citation1996) and Estrella and Hardouvelis (Citation1991) examine the predictability of equity returns based on changes in economic activity. More recently, Bakas and Triantafyllou (Citation2019) reported that greater macroeconomic instability provoked the emergence of higher volatility in the US commodity market.
Stock markets have become an important conduit through which long-term finance is raised for economic growth and sustainable development (Guru & Yadav, Citation2019). Ackert et al. (Citation2019) argue that investors are generally risk-averse and that the volatility of their investments is of prime importance to them. Thus, the tendency of financial market volatility to be influenced by local economic activities and global events has been studied (Bekiros et al., Citation2016). Hondroyiannis and Papapetrou (Citation2001) and very recently, Su et al. (Citation2019) emphasise the need to consider domestic and global factors in explaining the volatility of stock markets. Chaudhuri and Koo (Citation2001) provide evidence that the volatilities in money supply, fiscal policy, exchange rates, and the Japanese market significantly affect the market volatility of four Asian economies of India, South Korea, Malaysia and Thailand. Similarly, Choudhry et al. (Citation2016) also find volatility spillover in the Canadian, Japanese, and UK markets from macroeconomic fundamentals and the US market. More recently, Prasad et al. (Citation2018) report evidence of volatility spillovers from both domestic macroeconomic fundamentals and the US market to markets in the Asia Pacific, the Americas and Europe. Similarly, volatility spillovers have also been reported from the UK market to the GCC stock markets (Alfreedi, Citation2019). Very recently, Camilleri et al. (Citation2019) reported the propagation of price volatility in markets in Portugal, Germany and France, whilst Su et al. (Citation2019) reveal that higher economic policy uncertainty in economies increases the market return volatility in G-7 countries. Su et al. additionally finds financial uncertainty to be irrelevant in explaining changes in return volatility and that news implied volatility and market volatility are inversely related. Emerging studies such as Baker et al. (Citation2020) and Baker et al. (Citation2020) provide evidence suggesting a significant influence of COVID-19 shocks on market volatility in the US. Similar findings have been made by Uddin et al. (Citation2021) for some 34 developed and emerging markets.
Africa’s contributions in this area of research mainly focus on explaining variations in the volatility of stock markets from a macroeconomic activity perspective. Ndlovu et al. (Citation2018), for instance, provide evidence on how changes in movements in macroeconomic variables such as interest rates, money supply, inflation, and exchange rates influence movements in stock prices in South Africa. Similarly, Lawal et al. (Citation2018) provide evidence on the effect of monetary and fiscal policies on the volatility of stock returns in Nigeria, whilst Barakat et al. (Citation2016) report evidence of the significant effect of macroeconomic variables on equity return volatility in Egypt and Tunisia. Few studies have investigated the impact of foreign markets on stock return volatility in Africa. Mensah and Alagidede (Citation2017) provide some evidence on the influence of foreign stock markets on the return volatility of Africa’s emerging markets. Also, Gourène and Mendy (Citation2018) report evidence of crude oil prices influencing movements in the stock returns of six of Africa’s largest markets. Boako and Alagidede (Citation2018) investigate African emerging markets’ level of exposure to volatility spillovers by examining the nature of their dependence on global markets following the global financial crises. Their findings show that African emerging markets became more correlated during the financial crisis and that the strengthening of their relationships was accounted for more by regional than global factors. However, no study on the African markets to date has simultaneously analysed domestic and global factors within a dynamic panel framework. Studies that have assessed the impact of COVID-19 on the volatility of Africa’s stock markets also remain non-existent. Contributing toward the filling of these gaps will significantly improve the literature in this regard.
3. Methodology and data
3.1. Estimating volatility of stock returns
In order to adequately estimate conditional variance as a proxy for the volatility of individual African stock returns, we assess the goodness of fit of the standard GARCH, exponential GARCH and GJR-GARCH models for each series. Akaike's information criterion and log-likelihood estimates dictate best fitting models from which conditional volatilities will be extracted. These methods have been used in previous studies such as Tsay (Citation2010), Switzer et al. (Citation2017), Mensah and Alagidede (Citation2017) and Abbas et al. (Citation2018). For the standard GARCH (p,q) model, the conditional variance is expressed as follows:
Where is the constant. The
denotes proxy short-run shock persistence while
denotes long-run shock persistence.
The conditional variance of the EGARCH model is expressed as follows:
Where is constant,
is the coefficient of the leverage effect and
is the residuals from mean equation.
and
represent short- and long-run shock persistence, respectively.
The conditional variance of the GJR-GARCH is expressed as follows:
Where represents the coefficient of the asymmetric effect.
proxy constant.
and
are coefficients of ARCH and GARCH terms.
3.2. Estimating dynamic panel model
The study examines the collective impact of domestic macroeconomic factors, global factors such as world oil prices, volatility spillovers from advanced markets and the COVID-19 pandemic on equity market volatility in Africa’s stock markets. Though in this process, we assess how volatility from advanced markets impact the volatility of Africa’s equity markets, models often used to examine financial market linkages like Copulas (e.g. Mensah & Alagidede, Citation2017; Naeem et al., Citation2020), bivariate GARCH models (Umar & Suleman, Citation2017) connectedness approach (Umar et al., Citation2022) among others will not be used as these methods are more appropriate for investigating dependence structure among markets. To understand how domestic macroeconomic factors, advanced market volatility, world oil prices and COVID-19 collectively impact African market volatility, these models may not be effective. Furthermore, the vector autoregressive (VAR) model used in Prasad et al. (Citation2018) to examine equity market volatility determinants poses interpretation problems as the signs of some lagged variables may change across lags. Relationships among economic and financial variables usually involve dynamic adjustment processes. Thus, models of standard time series and cross-sectional data often present limited analyses and potential inability to capture differences in behaviour adequately. We, therefore, consider a class of linear dynamic panel models that allow for endogenous covariates to examine the key drivers of return volatility of African stock indices. Models of standard time series and cross-sectional data may not adequately capture the dynamic processes involved in the data. The baseline dynamic panel model used in this paper (with i = 1, … N, t = 1, … T) is expressed by Eq. (4) as follows:
where is the dependent variable (return volatility) of stock market index i at time t,
is lagged dependent variable with
as the autoregressive parameter. The lagged dependent variable is included to capture the persistence of volatility.
is a set of predictive variables,
denotes the unobserved time-invariant market-specific effects, and
is the idiosyncratic error term. However, by construction, the individual market-specific effects
are thought to be correlated with the lagged dependent and other explanatory variables. In addition, the covariates may also exhibit a non-zero correlation with the contemporaneous or lagged idiosyncratic errors. In effect, the concerns about endogeneity imply that the ordinary least squares, fixed effects and random effects estimators may produce biased or inconsistent results (Wooldridge, Citation2012). Arellano and Bond (Citation1991) initially introduced the differenced generalized method of moments (DGMM) estimator as a viable approach for addressing the issue of endogeneity tied to the lagged dependent variable. Subsequently, Blundell and Bond (Citation1998) enhanced the DGMM estimator by creating a system GMM estimator, which is particularly well suited for scenarios involving a high degree of persistence in the dependent variable. Nonetheless, subsequent research demonstrated the inadequate performance of both these GMM estimators in cases where the panel comprises a limited number of cross-sectional units (Bruno, Citation2005a; Bun & Kiviet, Citation2003). This circumstance applies to our study as we concentrate on merely eight (8) African markets. Consequently, we adopt the Least Squares Dummy Variable Corrected (LSDVC) estimator (Bruno, Citation2005a; Bun & Kiviet, Citation2003; Judson & Owen, Citation1999; Kiviet, Citation1995). This approach commences with a dynamic panel estimation and subsequently applies a sequential correction to mitigate the bias inherent in the fixed effects estimator. While Kiviet (Citation1995) and Bruno (Citation2005a) have demonstrated that the LSDVC estimator displays higher efficiency compared to the original LSDV estimator, as well as commonly used instrumental variables (IV) and GMM estimators, when dealing with a limited number of cross-sectional units, Judson and Owen (Citation1999) have highlighted that the LSDVC estimator is most suitable for balanced panel data (Sasao, Citation2014). Given that these conditions align with our dataset, we employ the LSDVC estimator in this study. Additionally, due to the findings of Bun and Kiviet (Citation2001) revealing that estimated asymptotic standard errors may not accurately approximate in smaller samples, we assess the statistical significance of the LSDVC coefficients using bootstrapped standard errors (50 iterations; see also Bruno (Citation2005b)). Notably, for this study, the bias corrections are initiated using the Anderson and Hsiao estimator (Anderson & Hsiao, Citation1981).
3.3. Data and data sources
The monthly price indices of Africa’s top eight markets based on market classification by Standard and Poor Global (Citation2021), comprising two emerging and six frontier markets, were sourced from DataStream. Data on macroeconomic variables and selected global factors were sourced from the International Financial Statistics (IFS) of the International Monetary Fund. Information on official entry month of COVID-19 into the sampled African countries is gleaned from the official website of the World Health Organization. The data covers the period from July 2003 to December 2021. Given the notion that market volatility exacerbates during crisis periods, the chosen time span allows us to examine significant periods before, during and after the inception of the two most recent global crises (i.e. 2007–2009 GFC and the COVID-19 pandemic). The stock markets studied include the main stock markets in South Africa, Egypt, Nigeria, Kenya, Ghana, Morocco, Botswana, and Mauritius. The selection of the stock markets was based on market size, trading volume, sector representation, and data availability. In fact, these markets represent the largest stock markets in Africa and account for over 95% of the total market capitalisation (PricewaterhouseCoopers, Citation2021). Considering that these markets are thinly traded, the study uses monthly returns in line with Abbas et al. (Citation2018), Agyei‐Ampomah and Fifield (Citation2011), Alagidede (Citation2008) and Mlambo and Biekpe (Citation2007) to address microstructural biases such as stale pricing and nonsynchronous trading. The advanced market indices included in the study include the USA’s Standard and Poor 500 index and the UK’s Financial Times Stock Exchange 100 index. The USA and UK markets are used to proxy sources of advanced markets volatility to Africa in this study as they are among the developed markets, considered the most important financial partners to African countries. While UK investors, for instance, remain the largest holders of foreign assets (worth $65 billion) in Africa as of 2021 (United Nations, 2021), the USA remains Africa’s largest trading partner among the developed markets (World Bank, Citation2020). Besides, Prasad et al. (Citation2018) finds the USA to be the largest transmitter of global volatility, with the UK following closely behind.
We used the volatility of stock returns determined from either EquationEquation (1)(1)
(1) , EquationEquation (2)
(2)
(2) or Equation (3) as the dependent variable in the study. Monthly volatility series are generated from the market return series of each of the individual stock index. The explanatory variables consist of domestic factors (money supply proxied by broad money, M2; inflation proxied by the consumer price index, CPI; exchange rate, proxied by nominal exchange rate, ER and 3-month Treasury bill rate (short-term interest rate), TBR), global factors (world crude oil prices proxied by Brent oil prices, WOP and volatility from advanced markets (including the USA represented by Standard and Poor 500 return volatility (SP500RV) and UK represented by Financial Times Stock Exchange 100 return volatility (FTSE100RV)) and COVID-19 time dummy coded 1 for months when COVID-19 was present in a sampled African country and 0 otherwise. With the first cases of COVID-19 in Nigeria and Egypt recorded in February 2020, the COVID-19 time dummy variable in the case of these countries is coded 0 for all months preceding February 2020 and 1 for February 2020 and all subsequent months where COVID-19 cases have been active. For South Africa, Morocco, Kenya, Ghana, Botswana and Mauritius, all of who recorded first cases of the virus in March 2020, the dummy variable is coded 0 for all months prior to March 2020 and 1 for March 2020 and all subsequent months with active cases. The selection of variables is based on both their theoretical relevance to equity markets and existing studies such as Uddin et al. (Citation2021), Baker et al. (Citation2020), Abbas et al. (Citation2018), Haider et al. (Citation2017), Choudhry et al. (Citation2016) and Chaudhuri and Koo (Citation2001).
4. Empirical results
4.1. Descriptive statistics of the returns
Table (Panel A) presents the descriptive statistics of the returns to the market indices of the sampled markets. Positive mean percentage returns are observed for almost all stock indices. The Kenyan market is the lone exception. The lowest and highest mean returns of the sampled markets are −0.02% (Kenya) and 1.24% (Egypt). While all ASM indices but Kenya are observed to outperform the UK’s FTSE 100 index in mean returns, only South Africa, Egypt and Ghana perform better than the US’s S&P 500 index within the sample period. The standard deviations of the markets range from 3.09% (Botswana—the least volatile market) to 9.31% (Egypt—the most volatile). Standard deviations of the advanced markets of the US and the UK are only higher than Botswana. These standard deviation statistics reinforce the long-held view that ASMs are largely volatile (see, for instance, Alagidede et al., Citation2011; Ndlovu et al., Citation2018). Further, with the exception of Botswana, all stock market returns exhibit negative skewness and have excess kurtosis, signifying a relatively higher probability of extreme negative returns compared to extreme positive returns. The null hypothesis of normality in the return distribution of all markets is strongly rejected by the Jarque–Bera statistic. The ARCH LM test (Engle, Citation1982) on all market returns strongly indicates the presence of ARCH-effects in each series. It therefore suffices to model the return distributions of the selected African market indices and advanced market indices (US’s S&P 500 index and UK’s FTSE 100 index) with GARCH models. The indication is that GARCH models are appropriate to capture return volatility of all the African markets as well as the US market (S&P 500 index) and UK market (FTSE 100 index). The above stylized facts that normally characterize financial time series data are also established in previous related studies such as Mensah and Alagidede (Citation2017), Abbas et al. (Citation2018), Alagidede et al. (Citation2011) among others.
Table 1. Descriptive statistics of the returns and drivers of return volatility
Also, Table (Panel B) presents the descriptive statistics of return volatility and possible key drivers in the sampled markets. Positive mean values are observed for stock return volatility and all of its possible drivers in our sample. In the Table, the corresponding minimum and maximum values, as well as the standard deviations, show the existence of significant deviations in all the variables. Mean return volatility of the sampled ASMs is observed to exceed the average volatilities of the S&P500 return and FTSE100 return. The mean statistics exhibit non-normality for all the variables, excess kurtosis for all but M2 and WOP and positive skewness for all but M2.
4.2. Stock return conditional volatility
Conditional volatility for the dynamic panel analysis is extracted from best fitting GARCH models. Based on the Akaike information criterion (AIC) and log likelihood estimates, the best fitting models are ARMA (1,1)-EGARCH (1,1) for Botswana and Morocco, AR (1)-EGARCH (1,1) for Ghana and Kenya, ARMA (1,3)-GJR-GARCH (1,1) for Nigeria, ARMA (2,3)-GJR-GARCH (1,1) for Mauritius, ARMA (1,3)-EGARCH (1,1) for Egypt, ARMA (3,3)-SGARCH (1,1) for South Africa, ARMA (2,2)-EGARCH (1,1) for the US and ARMA (0,0)-EGARCH (1,1) for the UK. Estimates of the ARMA-GARCH models are presented on Table . Table shows that the estimated conditional variance is influenced by past squared shocks (between −0.346 and 0.461) as well as past conditional variance (around 0.575 to 0.988). The monthly conditional variances obtained from the estimated GARCH models are used as monthly volatility estimates as in the study of Switzer et al. (Citation2017). The monthly volatility estimates from all the considered African markets are pooled to proxy the volatility of African markets. This becomes the dependent variable against which selected domestic, global factors and COVID-19 dummy are regressed to identify the factors influencing African market volatility. shows the time series plots for each market’s return volatility and sheds light on volatility trends over the sample period. For the majority of markets, the 2008–2009 GFC is the period that exhibits the greatest volatility, followed by the COVID-19 pandemic between March and July 2020.
Figure 1. Time series of conditional volatilities.
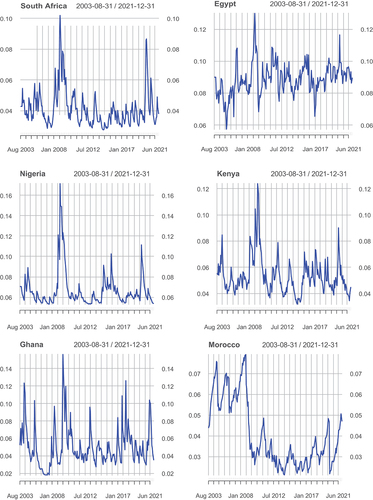
Table 2. Estimates of GARCH (1,1) models
4.3. Factors driving African stock returns volatility
Predictive variables in Eq. (4) are tested to ascertain their stationarity properties before model estimation. This is necessary to avoid the usage of non-stationary variables in estimating the dynamic panel model which could result in unintended spurious conclusions. Table presents the results of panel unit root tests for all variables involved in the panel analysis as well as results for time series unit root tests for all variables with repeated values across all panels. The Levin-Lin-Chu test and Augmented Dickey–Fuller (ADF) test are used for panel and time series unit root tests, respectively, in this paper. The tests are conducted at level and first differences, including only an intercept in the first stage and then both intercept and trend in the second stage. Using the Akaike information criterion to determine the appropriate lag length, we find that exchange rates, Treasury bill rates and (Brent) world oil prices are not stationary at level form. After first differencing, however, all variables become stationary. Predictive variables used in estimating Eq. 4 are therefore in their first difference state.
Table 3. Unit root test results
Table presents the correlations between the predictive variables in Eq. (4). This step is important in detecting potential multicollinearity. Estimations involving highly correlated predictive variables could bring about multicollinearity, which could adversely affect the reliability of the results estimated from Eq. (4). Table indicates that the association between the predictors is very low, largely less than 0.3 with the exception of the correlations between inflation rate (CPI) and Treasury bill rate (TBR) (0.657) and FTSE100 return volatility and S&P500 return volatility (0.796). Thus, the correlations in Table mainly imply that multicollinearity is not present and does not significantly affect the results from estimating Eq. 4.
Table 4. Correlation between predictive variables
We estimate the dynamic panel model of Eq. (4) and present the results in Table . The estimation is done with data for eight of the major stock markets in Africa and 221 monthly observations for each stock market i. Time dummies are included in all estimated models to capture the effect of any event that affects the variables of interest globally and ensures that the estimates are not biased as a consequence of the occurrence of any such events. The first lag of return volatility is found to have a relatively large coefficient which is highly significant in all estimated LSDVC models. This suggests that previous period volatility in African markets persists into the future.
Table 5. LSDVC estimates: drivers of African stock markets return volatility
The results in Table display statistically significant coefficients for all our predictive variables except the coefficient of domestic inflation rates. The findings indicate that both domestic factors (i.e., past volatility, money supply, exchange rates and Treasury bill rates) and global factors (i.e., advanced market volatility (US’s S&P 500 volatility and UK’s FTSE 100 volatility) and world crude oil prices) drive the return volatility of Africa’s stock markets. The exogenous shock from the novel coronavirus pandemic is also observed to have influenced the volatility of stock market returns in Africa. The findings further indicate that return volatility is persistent within the African equity markets as one period of past return volatility is seen to exert a significant positive influence on current return volatility. The finding that rising domestic economic uncertainty increases return volatility in African stock markets is consistent with studies such as Camilleri et al. (Citation2019) for European countries and Bakas and Triantafyllou (Citation2019) for the US market. Also, our findings are similar to those in Choudhry et al. (Citation2016), who found that world crude oil prices and the US market volatility are major sources of global shock.
Table shows a negative relationship between Treasury bill rates and stock market volatility in Africa. This may be explained by the opportunity cost effect. As Treasury bill rates rise, the opportunity cost of investing in stocks increases. Investors may find the yield from risk-free Treasury bills more appealing relative to the potential returns from stocks. This can result in reduced demand for stocks, leading to less trading activity and, consequently, lower volatility in the stock market (Pagan & Schwert, Citation1990; Schwert, Citation1981). The positive relationship observed between nominal exchange rates and stock market volatility suggests that increasing depreciations of African currencies fuel volatility in their markets. This may be because central banks often respond to currency depreciation by raising interest rates to curb inflation and stabilize the currency. Higher interest rates can increase borrowing costs for companies and consumers, potentially affecting economic growth and corporate profitability which could lead to investor flight. Additionally, higher rates in one country can attract foreign capital seeking better returns, potentially causing fluctuations in capital flows and impacting stock prices (Bagchi, Citation2017). The positive relationship observed between stock market volatility and money supply could be explained by the fact that increases in the money supply can sometimes lead to exaggerated market reactions, as investors may perceive the expansion as an overreaction to economic challenges. This can cause markets to swing between periods of irrational exuberance and sudden pessimism, resulting in heightened volatility (Bagchi, Citation2017; Engle & Ng, Citation1993). The finding of significant volatility spillover effects from the advanced markets of the US and the UK demonstrates the increasing interconnectedness of global financial markets. Heightened volatility in major economies often prompts international investors to reassess their risk exposure and seek safer assets, potentially leading to capital outflows from emerging markets like Africa. This can result in decreased demand for African stocks, triggering price declines, reduced liquidity and heightened uncertainty in local markets. Additionally, economic ties and trade relationships between global economies and African nations can transmit negative impacts, such as reduced demand for African exports, further influencing stock market volatility in the region.
The study also examines the time-varying effects of domestic and global factors on the return volatility of African stock markets. This is in keeping with the view that the influence of macroeconomic variables on stock market (return) volatility may strengthen during crisis periods (Abbas et al., Citation2018; Schwert, Citation1989). In consequence, we re-estimated Eq. (4) using sub-sample data to investigate the nature of the dependence of return volatility on domestic and global factors due to the 2007–2009 global financial crises and the COVID-19 pandemic. Table presents the results of the sub-sample analysis for the periods before, during, and after the GFC as well as during the COVID-19 pandemic. Table indicates that market return volatility in Africa before the GFC was primarily driven by previous period volatility. Consistent with the assertion of Schwert (Citation1989) and Abbas et al. (Citation2018) that macroeconomic factors have a stronger impact on volatility during crisis periods, significant impacts of domestic macroeconomic factors (i.e., money supply, Treasury bill rates and exchange rates) are observed on market return volatility in Africa during the GFC. Additionally, strong volatility spillover effects are observed from the advanced markets of the US and the UK to Africa’s equity markets during the period of the GFC. In the period after the GFC, domestic factors including previous period volatility, inflation rates, Treasury bill rates and exchange rates as well as global factors including world oil prices and US market volatility are observed to drive stock return volatility in African markets. The finding of significant volatility spillover effects from the US market (S&P 500) and UK market (FTSE 100) to African markets in the period during and after the GFC particularly suggests that African markets are not generally immune to shock spillovers from advanced markets. This finding contradicts the assertion that shock transmissions from global markets to developing markets may stagger in the midst of crisis and strengthen in the post-crisis period (Dungey & Gajurel, Citation2015). The evidence of contagious effects from advanced markets to African markets suggests that the various policies formulated by African governments over the years to foster market integration have been effective to some extent as the findings provide evidence of enhanced interconnectedness with global markets. During the COVID-19 pandemic, previous period volatility, Treasury bill rates and exchange rate movements comprise the factors identified as drivers of volatility in Africa’s equity markets. Though Africa’s markets seem not to have responded to global market volatility spillovers during the acute periods of the pandemic, immunity to global shocks is not inferred. Overall, the findings show that the return volatility of Africa’s equity markets follows a dynamic process and is largely driven by previous-period volatility, macroeconomic uncertainty and advanced market volatility.
Table 6. Drivers of African stock markets return volatility before, during and after crisis (GFC and Covid-19)
Furthermore, the study investigates drivers of return volatility across different regions in Africa. Understanding whether return volatility in the different regions responds in a similar fashion, particularly to the ongoing COVID-19 pandemic and to global factors such as advanced market volatility spillovers and world oil price changes, is of importance especially to investors seeking international portfolio diversification opportunities in Africa. We therefore re-estimate Eq. (4) to examine the drivers of return volatility in the Southern Africa region (South Africa and Botswana), Northern Africa region (Morocco and Egypt), Eastern Africa region (Kenya and Mauritius) and Western Africa region (Ghana and Nigeria). The resultant sub-sample analysis aids in checking the robustness of the results exhibited on Table . Table reports the results of drivers of return volatility within different regions in Africa.
Table 7. Drivers of stock return volatility within African regions
The results on Table identify previous period volatility, money supply, exchange rate and Treasury bill rates as the domestic factors that significantly drive stock market return volatility in all the regional blocs of Africa. While previous period volatility and Treasury bill rates relate positively and negatively, respectively, with current volatility across markets in all the regions, the impact of money supply and exchange rate is rather asymmetrical. A surge in money supply is particularly observed to trigger increases in return volatility in North Africa and West Africa but provoke decreases in return volatility in Southern Africa and East Africa. Increases in nominal exchange rates in North and East African countries (representing nominal depreciations of their currencies against the US dollar) are seen to drive return volatility upwards while similar increases in Southern and West African countries are observed to depress return volatility. The results further provide evidence indicating that increasing inflation rates fuel stock return volatility in markets located in North and West Africa. The positive nexus observed between return volatility and innovations in domestic macroeconomic factors is expected as increased macroeconomic uncertainty amplifies both systematic and idiosyncratic risk, leading to increased volatility in the stock markets as investors respond to rebalance their portfolios. The findings are consistent with previous studies including Su et al. (Citation2019), Prasad et al. (Citation2018) and Barakat et al. (Citation2016). Increasing money supply to address recurring episodes of negative and near zero inflation in East Africa during the study period, particularly in Mauritius, may explain the inverse relationship observed between money supply and volatility in the region. In such contexts, increasing money supply enhances eroding investor confidence reducing the tendency of portfolio rebalancing and market volatility. In line with Dornbush and Fischer’s (1980) goods market approach, our finding of a negative relationship between the nominal exchange rate and volatility in Southern and West Africa seems to suggest that listed firms in these regions take advantage of their depreciated currencies to boost exports and revenue as their goods become relatively cheaper on the international market. Return volatility therefore perhaps slows down in response to the possible declines in investor tendency to sell off as firm performance improves.
The results further identify movements in world crude oil prices as a global factor that significantly drives stock return volatility across all the regions of Africa with the exception of East Africa. Increases in oil prices are seen to cause depressions in return volatility for markets in North Africa and West Africa but trigger a surge in volatility for markets in Southern Africa. Among the African markets considered, Nigeria is the largest producer of oil followed by Egypt and Ghana. It is therefore not surprising that volatility in these markets decline in response to increasing oil prices. South Africa meanwhile is the largest importer of crude oil in Africa. Increasing world crude oil prices is therefore expected to introduce significant uncertainty in the region and fuel market volatility. Abbas et al. (Citation2018) make similar observations for huge oil-producing markets and huge oil-importing markets within the G-7 fraternity.
Volatility spillovers originating from the US market and the UK market are also identified as significant drivers of volatility in some regions of Africa. We find that increases in US market volatility fuels stock return volatility in Southern, East and West Africa. The significant sensitivity of these markets to shocks from the US market may be due to the continuing strengthening of their economic links to the US, especially in the area of international trade (see Sugimoto et al., Citation2014). Although an increase in UK market volatility is observed to exert an upward pressure on volatility in Southern and East African markets, the magnitude of the impact is larger in Southern Africa. This may largely be due to the presence of South Africa in the Southern African region. South Africa is a member of BRICS, and its stock index tends to move closely with developed markets. South Africa is also the UK’s largest trading partner in Africa. Moreover, there is a dual listing agreement between South Africa’s stock exchange and that of the UK, so most of South Africa’s large companies have exposure to the UK. It, therefore, comes as no surprise that UK market volatility exerts a significant influence on the volatility of markets in Southern Africa. This finding is consistent with the finding of Mensah and Alagidede (Citation2017), who found similar links between South Africa and the UK market. Alfreedi (Citation2019) observes similar links between the UK market and GCC markets. Markets in North Africa are identified as the only markets not responsive to advanced market volatility spillovers.
The exogenous shock from the COVID-19 pandemic is also identified to fuel return volatility in Southern, North, and East African equity markets. Specifically, we find that return volatilities in these markets exacerbated in the months following the advent of COVID-19 pandemic. COVID-19 periods are seen to record volatilities which exceed pre-Covid-19 levels averagely by 8.7%, 5.8% and 1.56%, respectively. The findings are expected as the COVID-19 pandemic acts as a source of systematic risk which increases economic uncertainty. Since its arrival, news concerning its case count, deaths, second, third and ongoing waves may have resulted in significant fear-driven sell-offs in these three regions, causing a surge in their return volatility. The finding is consistent with Baker et al. (Citation2020), Baker et al. (Citation2020) and Uddin et al. (Citation2021).
Overall, the results on Table demonstrate that even with the exclusion of other regions from the sample, our finding of domestic and global factors as well as COVID-19 to have a significant impact on return volatility in Africa as presented on Table remains largely robust.
5. Conclusion
The paper examined the determinants of stock return volatility in African markets. Initial analyses reveal that market volatility in Africa is driven by domestic factors, global factors as well as COVID-19 induced uncertainty. Time variant analyses reveal that equity return volatility in Africa’s markets was driven primarily by previous period volatility before the 2007–2009 GFC. During and post the GFC, identical domestic macroeconomic factors as well as global factors comprising advanced market volatility spillovers and world oil price movements are identified to fuel return volatility in Africa. The seeming spontaneous reaction of Africa’s equity markets to increased advanced market volatility contests the notion that shock transmissions from advanced markets to developing markets stagger in the midst of crisis and strengthen in the post-crisis period as advanced in one strand of the literature. Regional considerations further reveal that although domestic factors drive market volatility in all the regions of Africa, the impact of global factors differs from region to region. The pronounced significance of domestic factors across markets in all the regions upholds the arguments of the arbitrage pricing theory. Markets in the North African region are identified as the only markets that are immune to advanced market volatility spillovers as significant spillover effects are observed from the US and UK markets to markets in East, West and Southern Africa only. The finding effectually suggests that markets in the North African region provide an effective hedge against global risk and are as such avenues for international portfolio diversification. The COVID-19 pandemic with its attendant economic uncertainties is identified to have exacerbated return volatility in markets in all regions except West Africa. The finding perhaps reflects confidence investors have in the measures adopted by various governments in Africa to combat the COVID-19 pandemic. The empirical results of the GARCH family models also revealed the predictable nature of African market returns at both the first and second moments. The finding that previous returns (volatility) influence current returns (volatility) for all African markets points to the inefficient nature of African markets. The finding thus provides evidence against the weak form efficiency of African markets reported in the earlier study of Appiah-Kusi and Mensah (Citation2003).
In general, Africa’s equity markets return volatility is driven by domestic factors, global factors and more recently by COVID-19 related shocks. However, the extent of the effect varies, taking into consideration the regional dissimilarity and time-varying effects. The paper’s findings have some important implications for policy-makers and market participants. First, given that the effects from global market shocks may be pronouncedly felt in Africa during and even after crisis periods as evidenced by our findings on the GFC, African governments should concentrate their efforts towards weaning the continents’ stock markets from the negative repercussions of global market crashes by proactively developing frameworks and programs to mitigate the shocks at the very nascent stage. They could conduct rigorous risk assessments to identify vulnerabilities that make them susceptible to external shocks. Policies to mitigate these vulnerabilities, such as strengthening financial institutions and reducing exposure to specific sectors, can help manage the impact of spillover effects Second, the absence of significant volatility spillover effects from advanced markets to the equity markets in North Africa point to possible gains for international investors holding stocks in North African equity markets. There may, however, be limits to international portfolio diversification benefits if the stock indices of markets in other regions of Africa are held. Moreover, policymakers seeking to draw greater portfolio investment into the African continent may find the results useful. Policymakers in Southern, West and East Africa could, in their integration agenda, identify and target optimal levels of market integration that will mitigate their exposure to global shock spillovers, allow them to reap the benefits of scale economies and make them viable options for international portfolio diversification. Third, the exacerbation of equity return volatility by the COVID-19 pandemic as evidenced in this paper points to its potential as well as that of future pandemics to plunge most African economies into financial crisis. Although the acute periods of the pandemic seem to have faded, it is still imperative for policymakers to identify effective economic policies capable of reducing equity market volatility in the event of similar events in the future so as to avert potential financial crises empirically found to be associated with significant sudden increases in market volatility. Overall, we recommend that stakeholders, governments, companies, investors, financial markets and fund managers have an in-depth understanding of the varying volatilities of the equity markets. This will help stakeholders make informed investment decisions. Likewise, understanding market drivers will save stakeholders from normal and abnormal market periods.
Although the study makes useful findings on the behaviour of equity return volatility during the COVID-19 pandemic in Africa, it does not identify measures that will be effective enough to mitigate the impact of the pandemic and similar events on market volatility. Future studies are entreated to investigate and identify factors that prove to be particularly effective in reducing equity market volatility in Africa’s developing markets during the pandemic so as to inform the adoption and implementation of appropriate economic policy.
Availability of data and material
The data used for this study are available upon request from the authors.
Disclosure statement
No potential conflict of interest was reported by the author(s).
Additional information
Funding
References
- Abbas, G., McMillan, D. G., & Wang, S. (2018). Conditional volatility nexus between stock markets and macroeconomic variables: Empirical evidence of G-7 countries. Journal of Economic Studies, 45(1), 7–22. https://doi.org/10.1108/JES-03-2017-0062
- Ackert, L. F., Kluger, B. D., & Qi, L. (2019). Implied volatility and investor beliefs in experimental asset markets. Journal of Financial Markets, 43, 121–136. https://doi.org/10.1016/j.finmar.2019.02.001
- Agyei‐Ampomah, S., & Fifield, S. G. M. (2011). Stock market integration in Africa. Managerial Finance, 37(3), 242–256. https://doi.org/10.1108/03074351111113306
- Alagidede, P. (2008). African stock market integration: Implications for portfolio diversification and international risk sharing. In Proceedings of the African Economic Conferences, 30 November, Tunis-Tunisia (pp. 25–54). Economica.
- Alagidede, P., Panagiotidis, T., & Zhang, X. (2011). Causal relationship between stock prices and exchange rates. The Journal of International Trade and Economic Development, 20(1), 67–86. https://doi.org/10.1080/09638199.2011.538186
- Alfreedi, A. A. (2019). Shocks and volatility spillover between stock markets of developed countries and GCC stock markets. Journal of Taibah University for Science, 13(1), 112–120. https://doi.org/10.1080/16583655.2018.1544348
- Anderson, T. W., & Hsiao, C. (1981). Estimation of dynamic models with error components. Journal of American Statistics Assoc, 76(375), 598–606. https://doi.org/10.1080/01621459.1981.10477691
- Appiah-Kusi, J., & Mensah, K. (2003). Return predictability in Africa stock markets. Review of Financial Economics, 12(3), 247–270. https://doi.org/10.1016/S1058-3300(02)00073-3
- Arellano, M., & Bond, S. (1991). Some tests of specification for panel data: Monte Carlo evidence and an application to employment equations. The Review of Economic Studies, 58(2), 277–297. https://doi.org/10.2307/2297968
- Atenga, E. M. E., & Mougoué, M. (2021). Return and volatility spillovers to African equity markets and their determinants. Empirical Economics, 61(2), 883–918. https://doi.org/10.1007/s00181-020-01881-9
- Bagchi, B. (2017). Volatility spillovers between crude oil price and stock markets: Evidence from BRIC countries. International Journal of Emerging Markets, 12(2), 352–365. https://doi.org/10.1108/IJoEM-04-2015-0077
- Bakas, D., & Triantafyllou, A. (2019). Volatility forecasting in commodity markets using macro uncertainty. Energy Economics, 81, 79–94. https://doi.org/10.1016/j.eneco.2019.03.016
- Baker, S. R., Bloom, N., Davis, S. J., Kost, K., Sammon, M., & Viratyosi, T., (2020). The unprecedented stock market impact of COVID-19. Working Paper 26945, National Bureau of Economic Research.
- Baker, S. R., Bloom, N., Davis, S. J., & Terry, S. J., (2020). COVID-Induced economic uncertainty. NBER Working Paper No. 26983, National Bureau of Economic Research,
- Barakat, M. R., Elgazzar, S. H., & Hanafy, K. M. (2016). Impact of macroeconomic variables on stock markets: Evidence from emerging markets. International Journal of Economics and Finance, 8(1), 195–207. https://doi.org/10.5539/ijef.v8n1p195
- Bekaert, G., Harvey, C. R., & Lumsdaine, R. L. (2002). Dating the integration of world equity markets. Journal of Financial Economics, 65(2), 203–247. https://doi.org/10.1016/S0304-405X(02)00139-3
- Bekiros, S., Gupta, R., & Kyei, C. (2016). On economic uncertainty, stock market predictability and nonlinear spillover effects. The North American Journal of Economics & Finance, 36, 184–191. https://doi.org/10.1016/j.najef.2016.01.003
- Blundell, R., & Bond, S. (1998). Initial conditions and moment restrictions in dynamic panel data models. Journal of Econometrics, 87(1), 115–143. https://doi.org/10.1016/S0304-4076(98)00009-8
- Boako, G., & Alagidede, P. (2018). African stock markets in the midst of the global financial crisis: Recoupling or decoupling? Research in International Business and Finance, 46, 166–180. https://doi.org/10.1016/j.ribaf.2018.02.001
- Boamah, N. A., Akotey, J. O., & Aawaar, G. (2020). Economic engagement and within emerging markets integration. Research in International Business and Finance, 52, 1–11. https://doi.org/10.1016/j.ribaf.2019.101106
- Bruno, G. S. F. (2005a). Approximating the bias of the LSDV estimator for dynamic unbalanced panel data models. Economics Letters, 87(3), 361–366. https://doi.org/10.1016/j.econlet.2005.01.005
- Bruno, G. S. F. (2005b). Estimation and inference in dynamic unbalanced panel data models with a small number of individuals. Stata Journal, 5(4), 473–500. https://doi.org/10.1177/1536867X0500500401
- Bun, M. J. G., & Kiviet, J. F., (2001). The accuracy of inference in small samples of dynamic panel data models, Tinbergen Institute Discussion Paper TI 2001- 006/4,
- Bun, M. J. G., & Kiviet, J. F. (2003). On the diminishing returns of higher order terms in asymptotic expansions of bias. Economics Letters, 79(2), 145–152. https://doi.org/10.1016/S0165-1765(02)00299-9
- Büttner, D., & Hayo, B. (2011). Determinants of European stock market integration. Economic Systems, 35(4), 574–585. https://doi.org/10.1016/j.ecosys.2010.10.004
- Camilleri, S. J., Scicluna, N., & Bai, Y. (2019). Do stock markets lead or lag macroeconomic variables? Evidence from select European countries. The North American Journal of Economics & Finance, 48, 170–186. https://doi.org/10.1016/j.najef.2019.01.019
- Carlston, B. (2018). Can stock market liquidity and volatility predict business cycles? Studies in Economics and Finance, 35(1), 81–96. https://doi.org/10.1108/SEF-05-2016-0131
- Chaudhuri, K., & Koo, K. (2001). Volatility of stock returns: Importance of economic fundamentals. Economic and Political Weekly, 36(40), 3852–3856.
- Cheng, A. W. W., & Yip, I. W. H. (2017). China’s macroeconomic fundamentals on stock market volatility: Evidence from Shanghai and Hong Kong. Review of Pacific Basin Financial Markets and Policies, 20(2), 1750014. https://doi.org/10.1142/S021909151750014X
- Chen, N. F., Roll, R., & Ross, S. A. (1986). Economic forces and the stock market. The Journal of Business, 59(3), 383–403. https://doi.org/10.1086/296344
- Chen, N. F., & Zhang, F. (1998). Risk and return of value stocks. The Journal of Business, 71(4), 501–535. https://doi.org/10.1086/209755
- Choudhry, T., Papadimitriou, F. I., & Shabi, S. (2016). Stock market volatility and business cycle: Evidence from linear and nonlinear causality tests. Journal of Banking and Finance, 66, 89–101. https://doi.org/10.1016/j.jbankfin.2016.02.005
- Diaz, E. M., Molero, J. C., & de Gracia, F. P. (2016). Oil price volatility and stock returns in the G7 economies. Energy Economics, 54, 417–430. https://doi.org/10.1016/j.eneco.2016.01.002
- Dungey, M., & Gajurel, D. (2015). Contagion and banking crisis -international evidence for 2007- 2009. Journal of Banking and Finance, 60, 271–283. https://doi.org/10.1016/j.jbankfin.2015.08.007
- Engle, R. F. (1982). Autoregressive conditional heteroskedasticity with estimates of the variance of UK inflation. Econometrica, 50(4), 987–1007. https://doi.org/10.2307/1912773
- Engle, R. F., & Ng, V. K. (1993). Measuring and testing the impact of news on volatility. The Journal of Finance, 48(5), 1749–1778. https://doi.org/10.1111/j.1540-6261.1993.tb05127.x
- Estrella, A., & Hardouvelis, G. A. (1991). The term structure as a predictor of real economic activity. The Journal of Finance, 46(2), 555–576. https://doi.org/10.1111/j.1540-6261.1991.tb02674.x
- Estrella, A., & Mishkin, F. S. (1996). The yield curve as a predictor of US recessions. Current Issues in Economics and Finance, 2(7), https://doi.org/10.2139/ssrn.1001228
- Eun, C. S., & Shim, S. (1989). International transmission of stock market movements. The Journal of Financial and Quantitative Analysis, 24(2), 241–256. https://doi.org/10.2307/2330774
- Fama, E. F. (1970). Efficient capital markets: A Review of theory and empirical work. The Journal of Finance, 25(2), 383–417. https://doi.org/10.2307/2325486
- Fama, E. F. (1981). Stock returns, real activity, inflation, and money. The American Economic Review, 71(4), 545–565.
- Fama, E. F. (1990). Stock returns, expected returns, and real activity. The Journal of Finance, 45(4), 1089–1108. https://doi.org/10.1111/j.1540-6261.1990.tb02428.x
- Fama, E. F., & French, K. R. (2004). The capital asset pricing model: Theory and evidence. Journal of Economic Perspectives, 18(3), 25–46. https://doi.org/10.1257/0895330042162430
- Ferson, W. E., & Harvey, C. R. (1991). The variation of economic risk premiums. Journal of Political Economy, 99(2), 385–415. https://doi.org/10.1086/261755
- Forbes, K. J., & Rigobon, R. (2002). No contagion, only interdependence: Measuring stock market comovements. The Journal of Finance, 57(5), 2223–2261. https://doi.org/10.1111/0022-1082.00494
- Geske, R., & Roll, R. (1983). The fiscal and monetary linkage between stock returns and inflation. The Journal of Finance, 38(1), 1–33. https://doi.org/10.1111/j.1540-6261.1983.tb03623.x
- Goriaev, A. (2004). Risk factors in the Russian stock market. Emerging Market Review, 2(1), 67–89.
- Gourène, G. A. Z., & Mendy, P. (2018). Oil prices and African stock markets co-movement: A time and frequency analysis. Journal of African Trade, 5(1–2), 55–67. https://doi.org/10.1016/j.joat.2018.03.002
- Guru, B. K., & Yadav, I. S. (2019). Financial development and economic growth: Panel evidence from BRICS. Journal of Economics, Finance and Administrative Science, 24(47), 113–126. https://doi.org/10.1108/JEFAS-12-2017-0125
- Gwahula, R. (2018). Examining key macroeconomic factors influencing the stock market performance: Evidence from Tanzania. International Journal of Academic Research in Accounting, Finance and Management Sciences, 8(2), 228–234. https://doi.org/10.6007/IJARAFMS/v8-i2/4315
- Haider, S. K. A., Hashmi, S. H., & Ahmed, I. (2017). Systematic risk factors and stock return volatility. APSTRACT: Applied Studies in Agribusiness and Commerce, 11(1–2), 61–70. https://doi.org/10.19041/APSTRACT/2017/1-2/8
- Hamao, Y., Masulis, R. W., & Ng, V. (1990). Correlations in price changes and volatility across international stock markets. The Review of Financial Studies, 3(2), 281–307. https://doi.org/10.1093/rfs/3.2.281
- Hondroyiannis, G., & Papapetrou, E. (2001). Macroeconomic influences on the stock market. Journal of Economics & Finance, 25(1), 33–49. https://doi.org/10.1007/BF02759685
- Judson, R. A., & Owen, A. L. (1999). Estimating dynamic panel data models: A guide for macroeconomists. Economics Letters, 65(1), 9–15. https://doi.org/10.1016/S0165-1765(99)00130-5
- King, D., & Botha, F. (2015). Modelling stock return volatility dynamics in selected African markets. Economic Modelling, 45, 50–73. https://doi.org/10.1016/j.econmod.2014.11.008
- Kiviet, J. F. (1995). On bias, inconsistency and efficiency of various estimators in dynamic panel data models. Journal of Econometrics, 68(1), 53–78. https://doi.org/10.1016/0304-4076(94)01643-E
- Koutmos, G., & Booth, G. G. (1995). Asymmetric volatility transmission in international stock markets. Journal of International Money and Finance, 14(6), 747–762. https://doi.org/10.1016/0261-5606(95)00031-3
- Kumari, J., & Mahakud, J. (2015). Relationship between conditional volatility of domestic macroeconomic factors and conditional stock market volatility: Some further evidence from India. Asia-Pacific Financial Markets, 22(1), 87–111. https://doi.org/10.1007/s10690-014-9194-7
- Lawal, A. I., Somoye, R. O., Babajide, A. A., & Nwanji, T. I. (2018). The effect of fiscal and monetary policies interaction on stock market performance: Evidence from Nigeria. Future Business Journal, 4(1), 16–33. https://doi.org/10.1016/j.fbj.2017.11.004
- Li, H., & Majerowska, E. (2008). Testing stock market linkages for Poland and Hungary: A multivariate GARCH approach. Research in International Business and Finance, 22(3), 247–266. https://doi.org/10.1016/j.ribaf.2007.06.001
- Markowitz, H. (1952). Portfolio selection. The Journal of Finance, 7(1), 77–91. https://doi.org/10.1111/j.1540-6261.1952.tb01525.x
- Mensah, J. O., & Alagidede, P. (2017). How are Africa’s emerging stock markets related to advanced markets? Evidence from copulas. Economic Modelling, 60, 1–10. https://doi.org/10.1016/j.econmod.2016.08.022
- Mlambo, C., & Biekpe, N. (2007). The efficient market hypothesis: Evidence from ten African stock markets. Investment Analysts Journal, 36(66), 5–17. https://doi.org/10.1080/10293523.2007.11082489
- Naeem, M., Umar, Z., Ahmed, S., & Ferrouhi, E. M. (2020). Dynamic dependence between ETFs and crude oil prices by using EGARCH-Copula approach. Physica A: Statistical Mechanics and Its Applications, 557, 124885. https://doi.org/10.1016/j.physa.2020.124885
- Ndlovu, B., Faisa, F., Resatoglu, N. G., & Türsoy, T. (2018). The impact macroeconomic variables on stock returns: A case of the Johannesburg stock exchange. Romanian Statistical Review, 2, 87.
- Pagan, A. R., & Schwert, G. W. (1990). Alternative models for conditional stock volatility. Journal of Econometrics, 45(1–2), 267–290. https://doi.org/10.1016/0304-4076(90)90101-X
- Peiró, A. (2016). Stock prices and macroeconomic factors: Some European evidence. International Review of Economics & Finance, 41, 287–294. https://doi.org/10.1016/j.iref.2015.08.004
- Poon, S. H., & Granger, C. W. (2003). Forecasting volatility in financial markets: A review. Journal of Economic Literature, 41(2), 478–539. https://doi.org/10.1257/.41.2.478
- Prasad, N., Grant, A., & Kim, S. J. (2018). Time varying volatility indices and their determinants: Evidence from developed and emerging stock markets. International Review of Financial Analysis, 60, 115–126. https://doi.org/10.1016/j.irfa.2018.09.006
- PricewaterhouseCoopers, (2021). Africa capital markets Watch. Accessed 15 March 2022 https://www.pwc.co.za/en/publications/africa-capital-markets-watch.html
- Ross, S. A. (1976). The arbitrage pricing theory of capital assets pricing. Journal of Economic Theory, 13(3), 341–360. https://doi.org/10.1016/0022-0531(76)90046-6
- Sadorsky, P. (1999). Oil price shocks and stock market activity. Energy Economics, 21(5), 449–469. https://doi.org/10.1016/S0140-9883(99)00020-1
- Sasao, T. (2014). Does industrial waste taxation contribute to reduction of landfilled waste? Dynamic panel analysis considering industrial waste category in Japan. Waste Management, 34(11), 2239–2250. https://doi.org/10.1016/j.wasman.2014.07.014
- Scheicher, M. (2001). The comovements of stock markets in Hungary, Poland and the Czech Republic. International Journal of Finance & Economics, 6(1), 27–39. https://doi.org/10.1002/ijfe.141
- Schwert, G. W. (1981). The adjustment of stock prices to information about inflation. The Journal of Finance, 36(1), 15–29. https://doi.org/10.1111/j.1540-6261.1981.tb03531.x
- Schwert, G. W. (1989). Why does stock market volatility change over time? The Journal of Finance, 44(5), 1115–1153. https://doi.org/10.1111/j.1540-6261.1989.tb02647.x
- Sharpe, W. F. (1964). Capital asset prices: A theory of market equilibrium under conditions of risk. The Journal of Finance, 19(3), 425–442. https://doi.org/10.1111/j.1540-6261.1964.tb02865.x
- Standard & Poor Global. (2021). Annual country classification Review. S&P Dow Jones Indices.
- Stulz, R. M. (1999). Globalisation, corporate Finance, and the cost of capital. Journal of Applied Corporate Finance, 12(3), 8–25. https://doi.org/10.1111/j.1745-6622.1999.tb00027.x
- Su, Z., Fang, T., & Yin, L. (2019). Understanding stock market volatility: What is the role of US uncertainty? The North American Journal of Economics & Finance, 48, 582–590. https://doi.org/10.1016/j.najef.2018.07.014
- Sugimoto, K., Matsuki, T., & Yoshida, Y. (2014). The global financial crisis: An analysis of the spillover effects on African stock markets. Emerging Markets Review, 21, 201–233. https://doi.org/10.1016/j.ememar.2014.09.004
- Suhaibu, I., Harvey, S. K., & Amidu, M. (2017). The impact of monetary policy on stock market performance: Evidence from twelve (12) African countries. Research in International Business and Finance, 42, 1372–1382. https://doi.org/10.1016/j.ribaf.2017.07.075
- Switzer, L. N., Tahaoglu, C., & Zhao, Y. (2017). Volatility measures as predictors of extreme returns. Review of Financial Economics, 35(1), 1–10. https://doi.org/10.1016/j.rfe.2017.04.001
- Tsay, R. S. (2010). Analysis of financial time series. Wiley. https://doi.org/10.1002/9780470644560
- Uddin, M., Chowdhury, A., Anderson, K., & Chaudhuri, K. (2021). The effect of COVID-19 pandemic on global stock market volatility: Can economic strength help to manage the uncertainty? Journal of Business Research, 128, 31–44. https://doi.org/10.1016/j.jbusres.2021.01.061
- Umar, Z., Polat, O., Choi, S. Y., & Teplova, T. (2022). The impact of the Russia-Ukraine conflict on the connectedness of financial markets. Finance Research Letters, 48, 102976. https://doi.org/10.1016/j.frl.2022.102976
- Umar, Z., & Suleman, T. (2017). Asymmetric return and volatility transmission in conventional and Islamic equities. Risks, 5(2), 22. https://doi.org/10.3390/risks5020022
- Vuyyuri, S., (2005). Relationship between real and financial variables in India: A cointegration analysis. Working Paper, National Science Research Network.
- Wilms, R., Mäthner, E., Winnen, L., & Lanwehr, R. (2021). Omitted variable bias: A threat to estimating causal relationships. Methods in Psychology, 5, 100075. https://doi.org/10.1016/j.metip.2021.100075
- Wooldridge, J. (2012). Introductory econometrics: A modern approach. Cengage Learning.
- World Bank, (2020). Sub-saharan Africa trade, Retrieved from https://wits.worldbank.org/CountryProfile/en/SSF