Abstract
The life insurance industry plays a crucial role in the economy as it serves as one of the channels through which countries mobilize long-term savings, promote the development of capital markets, foster efficient capital allocation, and substitute and complement government security programs. Therefore, the performance of this sector is imperative. Since the early 1990s, researchers have been paying particular attention to the performance of life insurance firms, with a specific emphasis on identifying the key determinants of their performance. The objective of this study is to synthesize the studies that have explored this topic. Using a systematic literature review approach, the study reviews 129 studies published between 1990 and 2021. The analysis reveals that the literature primarily examines factors such as size, organizational structure, capital composition, diversification, distribution systems, risk management practices, and reinsurance strategies as key firm-specific drivers of life insurer performance. Additionally, the study underscores the importance of competition and macroeconomic conditions as commonly discussed external determinants. While a clear relationship between performance and factors like firm size, organizational structure, and risk management practices is evident, the impact of other factors remains inconclusive. One of the implications of this study is that policymakers should enact laws that promote competition in the insurance industry. The study also reveals several research gaps, including methodological gaps.
PUBLIC INTEREST STATEMENT
The life insurance industry plays a crucial role in the economy as it serves as one of the channels through which countries mobilize long-term savings, promote the development of capital markets, foster efficient capital allocation, and substitute and complement government security programs. Therefore, the performance of this sector is imperative. This study provides an overview of studies that examine the determinants of the performance of life insurance companies from 1990 to 2021. Our examination of the 129 empirical studies indicates that size, organisational form, capital structure, diversification, distribution system, risk and reinsurance are the major firm-level determinants of life insurer performance, while competition and macroeconomic factors are the main external drivers. One of the implications of this survey is that given that larger life insurers are more cost efficient than smaller insurers, there might be a need to promote mergers and acquisitions among smaller and medium-sized insurers. This would encourage healthy competition in the industry.
1. Introduction
The life insurance industry is an integral part of the financial services sector. It serves as a channel through which countries mobilise long-term savings, promote the development of capital markets, foster capital allocation efficiency, complement the intermediation function of banking institutions, and substitute and complement government security programs (Skipper, Citation1997). The importance of the life insurance sector extends beyond economic development; it also carries significant value for individuals and groups, ensuring essential financial security for them and their families. Life insurance comprises two fundamental elements: capital accumulation and financial protection. Capital accumulation policies, represented by savings and investment plans, allow policyholders to incrementally build wealth over an extended period. By making regular premium payments, individuals contribute to these policies, and insurers typically invest these funds to generate returns. At predetermined points, such as policy maturity or retirement, policyholders gain access to the accrued funds, often augmented by interest or investment returns. This category of life insurance empowers individuals to proactively allocate resources toward future financial objectives, such as education expenses, homeownership, or retirement planning.
In parallel, life insurance plays a pivotal role in ensuring financial security for individuals and their dependents. Various policies, including whole life insurance, term life insurance, and endowment policies, offer different forms of protection. Whole life insurance provides lifelong coverage, guaranteeing beneficiaries a predetermined sum upon the policyholder’s demise. Conversely, term life insurance offers coverage for a specified period, paying out benefits if the insured individual passes away during that term. Endowment policies blend protection and savings, delivering a lump-sum payout upon maturity or the policyholder’s death, whichever occurs first. Furthermore, life insurers offer policies that provide financial support in cases of critical illness or disability, shielding policyholders from substantial medical expenses or income loss due to unforeseen health challenges. These policies contribute to peace of mind and financial stability during difficult times. The extended temporal horizon of life insurance policies, compared to non-life insurance, underscores their emphasis on long-term financial security, aligning with the pursuit of enduring financial goals and protection against life’s uncertainties.
Against this backdrop, the continuous progress and financial stability of this industry are of paramount importance in facilitating the rapid expansion of economic activities within any nation and ensuring financial security for individuals and their families during times of need. The life insurance industry is generally subject to stricter regulations than the non-life insurance sector due to its higher leverage (Biener et al., Citation2016a) and susceptibility to systemic risks (Cummins & Weiss, Citation2014; Moreno et al., Citation2020). One primary focus of insurance regulations pertains to the solvency of insurance companies (Hsiao & Whang, Citation2009; Nguyen & Vo, Citation2020). In recent decades, supervisory authorities worldwide have aimed to strengthen their solvency regulatory regimes (Akpan et al., Citation2021; Eling & Schaper, Citation2017). There has been a gradual shift from rules-based (compliance-based) to principle-based (risk-based) regulation in both developed and emerging markets (Cummins & Phillips, Citation2009).
In addition to ongoing changes in global insurance regulatory frameworks, many countries have also deregulated and liberalized their insurance markets to enhance competition (Boonyasai et al., Citation2002; Camino-Mogro et al., Citation2019; Chandrapal, Citation2019). These reforms have been accompanied by a wave of mergers and acquisitions, primarily in Europe and the US (Borges et al., Citation2008; Cummins et al., Citation2015; Davutyan & Klumpes, Citation2008; Klumpes, Citation2022; Zanghieri, Citation2009), as well as an increase in cross-border trade in insurance (Cummins et al., Citation2017). Alongside these changes, life insurers have faced significant pressures to innovate and perform due to widespread economic shifts, increased competition from entities such as banks, mutual funds, and investment advisory firms, low interest rates (Eling & Schaper, Citation2017), aging populations, reductions in public pensions (Swiss Re Institute, Citation2019), and technological advances in sales, underwriting, claims processing, and policy services (Cummins et al., Citation1999b). These developments raise a fundamental question: What factors determine the performance of life insurance firms?
Over the last three decades, numerous studies have delved into this topic. What prominently emerges from this body of research is the diversity of performance dimensions and the myriad factors that influence the performance of life insurers. The literature has considered various performance dimensions, encompassing traditional accounting ratios, efficiency-based measures, market-based measures, insolvency prediction-based measures, and financial strength-based measures (Doumpos et at., 2012). In examining the drivers of life insurer performance, these studies have explored diverse firm-specific factors, such as firm size, organisational form, and reinsurance, as well as external factors, including macroeconomic and institutional conditions (see e.g., Alhassan et al., Citation2021; Cummins & Rubio-Misas, Citation2021; Killins, Citation2020). It is in this context that this study endeavours to synthesise the existing body of literature that explored the drivers of the performance of life insurance companies. This undertaking is vital because it identifies the key factors that affect how well life insurance companies perform while also putting light on the effects of those factors. Additionally, this review demonstrates the current state of research on the subject, including insightful information about the prevailing trends, research trajectories, and the research gaps that still exist in the literature. This review study is important to policyholders, regulators, policymakers, managers, investors and researchers alike.
There are existing review studies (see Amel et al., Citation2004; Cummins & Weiss, Citation2000, Citation2013; Eling & Luhnen, Citation2010b; Kaffash et al., Citation2020) that offer insights into the determinants of insurer performance. However, our study differs from these reviews that mainly focused on the application of frontier efficiencies methodologies in the insurance industry in three crucial ways. First, we consider studies based on five dimensions of insurer performance: efficiency, accounting, market, rating, and insolvency measures. This is vital because merely comparing insurance companies’ performance in terms of technology, scale, profit maximisation, revenue maximisation, and cost minimisation is insufficient. Insurers’ performance should also be evaluated in terms of their claims-paying ability and solvency, given the fiduciary nature of insurance relations (Eling & Jia, Citation2018). Additionally, an insurer’s ability to maximise shareholder value, such as stock returns, is essential (Zhang et al., Citation2019).
Second, unlike previous studies that did not focus on a particular insurance segment, our study concentrates on the life insurance sector. In the literature, life and non-life insurers are predominantly studied separately due to differences in products, operations, outputs (Cummins & Xie, Citation2013; Cummins et al., Citation2010) and regulations (Oetzel and Banerjee, 2008). Consequently, the impact of various firm-specific and external factors may vary from one segment to the other. For instance, the life insurance industry is more sensitive to country-specific institutional factors (e.g. pre-existence of social security and inheritance tax systems), cultural factors, systemic risks (Biener et al., Citation2016a) and interest rate changes (Chen et al., Citation2010; Eling Schaper, Citation2017). In contrast, underwriting cyclesFootnote1 (Chen et al., Citation1999; Eling et al., Citation2020; Hoyt & Trieschmann, Citation1991; Owadally et al., Citation2019; Weiss, Citation2007) and natural catastrophic risks (Biener et al., Citation2016a; Cummins & Xie, Citation2013) are prominent features in the non-life insurance industry. Moreover, inflation tends to increase claims costs for non-life insurers (Cummins and Tennyson, Citation1992; Camino-Mogro and Bermúdez—Barrezueta, 2019; Shaddady, Citation2022), which may not be the case for life insurers because benefits for most mortality, wealth accumulation and longevity protection policies are fixed in nominal terms (Eling & Schaper, Citation2017). Additionally, high growth may be less detrimental for life insurers, likely because life insurance benefit payments typically occur many years later than the premium income; consequently, cash flow underwriting may yield a high growth rate without the immediate effects of claims and failures (Eling & Jia, Citation2018 p. 19).
Finally, we discuss additional factors that influence the performance of life insurers, which past review studies have not discussed, including, risk, reinsurance, business groups and macroeconomic factors. This study also provides updated literature reflecting recent developments and new estimation methods, such as the dynamic network data envelopment analysis (for efficiency estimation) and bootstrap truncated regression (a method for analysing the impact of contextual variables on efficiency).
The rest of this study is structured as follows. Section 1 focuses on our search strategy, data sources and features of the studies we reviewed. Section 2 provides an overview of our findings, and Section 4 summarises the main findings, conclusions, and identifies gaps for further research.
2. Methodology and data description
This section provides a description of our search strategy and characteristics of the studies we reviewed (i.e. data sources, performance measurement, geographical distribution, sample size, time period and methodological approaches).
2.1. Search strategy and data sources
The articles for this study were initially searched in several databases, including Web of Science, EBSCO, Scopus, Science Direct and Google Scholar. For this step, we employed three lists of keywords. The first list consisted of performance-related keywords: performance, profitability, efficiency, productivity, solvency, insolvency and rating. The second list comprised insurance-specific keywords such as life insurance firms, life insurers, life insurance, insurance industry and life insurance companies. The final list covered determinants, and the keywords used included determinants, drivers, market structure, competition, organisational form, distribution system and governance. The initial search yielded 936 studies. After reviewing the abstracts, introductions, and in some cases, the full papers (particularly those examining both life and non-life and/or composite insurance segments), we excluded 856 studies, leaving us with 80 studies that investigated the correlates of life insurer performance. Some of the excluded studies from our initial sample focused on the non-life segment, and some were not written in English.
Subsequently, we identified 49 additional articles from the reference sections of previous reviews and through searches in specific insurance, finance, accounting, economics, and management-related journals. This brought the total number of studies upon which this review is based to 129. Most of these studies (102) exclusively focused on the life insurance sector, while 27 investigated more than one segment but reported the performance of each segment (including the life insurance segment) separately. We restricted our selection to studies published between 1 January 1990 and 31 December 2021. This decision was based on the fact that most studies on the performance of insurance firms, especially those applying frontier efficiency methodologies, began in the early 1990s, coinciding with significant changes in the insurance industry. Of the studies in our database, 88% (113 studies) were published in peer-reviewed journals, with approximately 86% indexed in Scopus. Prominent outlets included the Journal of Risk and Insurance (16 studies), The Geneva Papers on Risk and Insurance-Issues and Practice (10 studies), Journal of Insurance Issues (6 studies), Journal of Banking and Finance (5 studies), Applied Economics Letters (3 studies) and Journal of Productivity Analysis (3 studies). In addition to journal papers, eleven of the studies are working papers, and five are book chapters.
2.2. Performance measurement
The literature on measures of performance of life insurance companies can be categorised into five classes. The first category of studies utilised efficiency measures. These measures provide more meaningful and reliable performance indicators (Cummins & Weiss, Citation2013) than other indicators because they remove the effects of market prices and other external factors that affect observed performance (Bauer et al., Citation1998). Additionally, frontier efficiency methods summarise performance into a single statistic that takes into account differences among firms (Cummins & Weiss, Citation2000, 2013). Approximately, fifty-five percent (71 studies) of the studies in our database (see Table ) are based on efficiency measures, with the most commonly used measure being cost efficiency. A firm’s cost inefficiency has been observed to consume a significant portion of average cost and to be a major source of performance problems compared to other efficiency measures such as scale or product-mix efficiency in the financial services industry (Bauer et al., Citation1998).
Table provides a summary of the methods utilised in frontier efficiency studies, including efficiency estimation techniques, choice of inputs and output types, and yearly average efficiency. Most efficiency studies (51 studies, 68.25%) in our dataset utilised DEA to estimate the efficiency of life insurers, with classic DEA dominating. Cummins and Rubio-Misas (Citation2016, 2021) are the only multi-country studies in our sample that applied the classic DEA in a metafrontier framework. As mentioned before, metafrontier analysis provides an unbiased comparison of insurer efficiency because it takes into account the heterogeneity in competitive, technological, economic, institutional, political, social and cultural conditions across countries or regions (O’Donnell et al., Citation2008).
Table 1. Summary of frontier efficiency studies on life insurance-methodologies, inputs, outputs and average efficiencies: 1990–2021
Only two studies, namely Krupa et al. (Citation2019) and Lu et al. (Citation2014) have applied recent DEA techniques. Krupa et al. (Citation2019) applied the slacks-based measure (SBM) DEA model in the German life insurance industry, while Lu et al. (Citation2014) used the dynamic SBM DEA model to measure the efficiency of life insurers in China. Unlike the classic DEA techniques, non-classic DEA models such as the SBM DEA and network DEA provide accurate measurements of time-specific dynamic efficiencies over an extended period (Kaffash et al., Citation2020). SFA was the second most popular technique and was applied in 21 studies (29.2%).
Another approach that has received attention in the literature is the distribution-free approach (DFA). Approximately 11.11% (7 studies) of the studies utilised this technique in a two-stage framework (see Gardner & Grace, Citation1993; Hao & Chou, Citation2005; Hussels & Ward, Citation2007; Meador et al., Citation1997; Ryan & Schellhorn, Citation2000). Nevertheless, approaches such as the thick frontier approach (TFA), free disposal hull (FDH) and Varian’s weak axiom of profit were less popular, jointly accounting for about 4.76% (3 studies).
The second stream (41 studies, 31.8%) is based on accounting ratios such as ROA, ROE, loss ratio, expense ratio, investment yield, and combined ratio (see, for example, Cummins et al., Citation2017; Ho, Citation2011, Maichel-Guggemoos and Wagner, 2019; Olaosebikan, Citation2013). ROA is the most frequently used measure in this class. The third strand of the literature utilised market-based measures, including stock return, Tobin’s Q, Treynor’s measure, and Jensen’s Alpha (for instance, Brewer et al., Citation2007; Zhang et al., Citation2019). Studies based on market measures account for only 1.6% (2) of the studies in our dataset.
Another group is represented by insolvency-prediction studies. This group examines the factors are associated with insolvency (represented mainly by a dummy variable, 1 for insurers that became insolvent and zero otherwise) of life insurers (for example, Ambrose & Carroll, Citation1994; Browne et al., Citation1999; Carson & Hoyt, Citation2000, Citation1995). Only 10 studies in our database represent this stream. The final strand uses ratings of firms, and only one study (0.8%) represents this strand (see Pottier, Citation1997).
Despite the adoption of different performance measures in insurance studies, the literature indicates that these indicators should be highly correlated (see, for example Bauer et al., Citation1998; Cummins et al., Citation1999b).
2.3. Geographical distribution of studies
One-hundred and nine studies in our database are country case studies covering 22 countries (see Figure ). The US has the highest concentration with 37 studies (28.7%), followed by the UK (15 studies, 11.6%), Taiwan (15 studies, 11.6%), Germany (7 studies, 5.4%) and China (7 studies, 5.4%). In our database, there are 20 cross-country studies (15.5%) and more than half of them focusing exclusively on the EU. The most extensive cross-country study covered 91 countries from both developed and developing markets (see Eling and Jia, Citation2019), while the least extensive study covered four Asian countries (see Chen & Wong, Citation2004).
It is clear that most studies focused on countries with the most advanced insurance markets compared to less advanced economies such as those in Africa and Latin America, which are represented only by Ghana (3 studies), Nigeria (1 study), and South Africa (2 studies) and Ecuador (2 studies), respectively.
2.4. Sample size
As shown in Table , studies differ considerably in terms of sample sizes (average number of insurers per year). Approximately 29.4% have a sample size of at least 150 insurers. About 19.4% examined the performance of fewer than 21 life insurers, while 23.8% considered between 21 and 40 insurers, and 14.3% between 41 and 60 insurers. For country-specific studies, sample sizes range from 8 (Zhang et al., Citation2019) to 1,593 (Browne et al., Citation2001), while for cross-country studies, they range from 15 (Biener & Eling, Citation2011) to 1,815 (Eling and Jia, Citation2019).
Table 2. Classification of studies on insurer performance
However, it is vital to note that the variation in sample sizes makes it difficult to compare results across studies. The problem lies mainly with studies based on small sample sizes because small sample sizes may lead to biased estimates of regression coefficients and standard errors (Scherbaum & Ferreter, Citation2009; Maas and Hox, 2005). Based on Monte Carlo simulation, Zhang and Bartels (1998) confirmed that for standard DEA applications, average TE in the electricity distribution industry varies with sample sizes. Staat (2001) concludes that sample size bias has much wider implications than suggested by Zhang and Bartels (1998).
2.5. Time period covered
There is a wide variation in the average time periods considered in the literature, with 57.6% of the studies having a sample range of not more than 9 years (see Table ). Among these studies, approximately half have an average sample period of between 4 and 6 years, while about 18.9% have an average period of between 7 and 9 years. Studies that considered an average time period of between 1 and 3 years constitute 11.4%, while those with an average time period of between 10 and 12 years account for 18.2%. Close to 24.2% of the studies have an average sample period of at least 13 years. What is evident is that only a few studies have data covering relatively long periods. For example, Chuang and Tang (Citation2015), McNamara (Citation1991), Brewer et al. (Citation2007), Jeng and Lai (Citation2008) and Hao and Chou (Citation2005) have data covering between 24 and 35 years. The time period has an implication on the estimation of efficiency scores. For multiple-year data, efficiency scores can be estimated based on separate or pooled (global) frontiers. Estimation of efficiency scores based on separate frontiers helps in productivity analysis. However, for the second stage regression, it may not be appropriate to use efficiency scores based on separate frontiers because they are not comparable; instead, efficiency scores of the pooled or common frontier should be used.
2.6. Methodological Approaches
To determine efficiency drivers, the estimated efficiency scores are generally regressed against several factors (or one or more factors and some control variables) that are known to influence the performance of DMUs, in this case, life insurance firms. Different regression models can be used for this purpose, including OLS, Tobit and bootstrap truncated regression models, which have been central to the debate in the literature (see Hoff, Citation2007; McDonald, Citation2009; Simar & Wilson, Citation2011). In our dataset, Tobit and OLS dominate as second stage models (see, for example, Bian & Wang, Citation2017, Cummins and Rubio-Misas, Citation2016; Boonyasai et al., Citation2002; Carr et al., Citation1999; Chadwick & Cappelli, Citation1999). It is worth mentioning that some DEA studies used statistical tests (for example, Trigo-Gamarra & Growitsch, Citation2010), cross-frontier analysis (see Chen & McNamara, Citation2014; Jeng & Lai, Citation2008) and/or a combination of these techniques (see Cummins & Zi, Citation1998; Erhemjamts & Leverty, Citation2010; Fukuyama, Citation1997) to analyse the factors associated with the efficiency of life insurers.
To examine the drivers of efficiency using SFA, the literature recommends a one-stage approach instead of a two-stage approach (Danquah et al., Citation2018). This is because a second stage approach contradicts the assumption of identically distributed inefficiency effects in the stochastic frontier (Battese & Coelli, Citation1995). Out of 19 studies that utilised SFA in our dataset, only six adopted a one-stage approach in their empirical analysis (see Eling & Luhnen, Citation2010a; Ennsfellner et al., Citation2004; Fenn et al., Citation2008; Yuengert, Citation1993; Zanghieri, Citation2009). Others used a two-stage regression approach (see Bian & Wang, Citation2017; Chuang & Tang, Citation2015; Hao & Chou, Citation2005; Hardwick et al., Citation2011; Hu & Yu, Citation2015; Klumpes, Citation2004) while authors such as Cummins and Zi (Citation1998), Huang et al. (Citation2019) and Hardwick (Citation1997) applied statistical tests and/or other methods.
Regarding accounting-based studies, different techniques have also been adopted, but OLS, fixed effect, and random effect panel techniques have been used more frequently (see, for instance, Akotey et al., Citation2013; Browne et al., Citation2001; Camino-Mogro et al., Citation2019; Collins et al., Citation1997; Connelly & Limpaphayom, Citation2004; Fields et al., Citation2012; Shiu, Citation2009, 2006). Techniques such as the generalised method of moments (GMM), two-stage least squares, and Heckman two-stage regression have been utilised in fewer studies.
3. Determinants of life insurer performance
This section discusses the empirical findings of various studies. The decision to include a particular study is based on its relevance both to the issues being discussed and to emerging markets and developing countries. Different studies in our dataset employed a wide variety of factors as determinants of life insurer performance. These factors can be broadly grouped into two main categories: firm-level (internal) and external factors. We distinguish between country-specific and cross-country determinants of performance. The firm-level factors that have been widely examined in the literature are insurer size, organisational form, capital structure, diversification, distribution system, risk and reinsurance. Market structure and macroeconomic factors are the most commonly analysed external factors in both country-specific and cross-country studies. Appendix 1 provides a summary of the effect of different firm-level and external factors on different measures of performance while Table in Appendix 2 shows a summary of studies that examined drivers of life insurer performance.
3.1. Firm-level determinants of life insurer performance
3.1.1. Firm size and life insurer performance
Amongst the determinants of the performance of life insurers commonly investigated in the literature, company size has received the greatest scholarly attention. Size is generally used as a proxy for economies of scale (Fecher et al., Citation1991; Yuengert, Citation1993). As an insurer’s output increases, its costs of determining a premium rate, classify a policy, pay a claim and invest a premium increase at a lower rate than its output (Katrishen & Scordis, Citation1998; Skogh, Citation1982). However, further expansion may create inefficiencies because of increased complexity, managerial conflicts, agency costs and costs of coordinating operations (Cummins & Weiss, Citation2013; Katrishen & Scordis, Citation1998). It therefore follows that the relationship between size and performance may be non-linear.
Most frontier efficiency studies showed that larger life insurers are more technically efficient (see for example Borges et al., Citation2008; Cummins, Citation1999; Eling & Schaper, Citation2017; Fecher et al., Citation1993, 1991), cost efficient (see Biener et al., Citation2016b; Carr et al., Citation1999, Cummins and Rubio-Misas, Citation2016; Cummins & Zi, Citation1998), Cummins (Citation1999) and revenue efficient (Cummins and Rubio-Misas, Citation2016; Cummins, Citation1999; Cummins et al., Citation2010; Hitt, Citation1999; Pottier, Citation2011) than smaller and medium-sized insurers.
The evidence on the effect of size on other efficiency measures such as scale, pure technical, profit and allocative efficiency, is mostly inconclusive, possibly reflecting differences in jurisdictions, estimation methods, sample sizes and time periods (see e.g. Huang et al., Citation2019; Leverty et al., Citation2004). One study in this category is noteworthy: Boonyasai et al. (Citation2002), who examined the effect of liberalisation and regulation on life insurer efficiency in four Asian countries: South Korea, the Philippines, Taiwan and Thailand, using DEA in the first stage and Tobit in the second stage. The authors found that size (measured as a natural logarithm of premiums) is positively related to pure technical and scale efficiency in South Korea, Taiwan and Thailand; in the Philippines they found size to have a negative effect on pure technical efficiency and an insignificant impact on scale efficiency. The results from this study should however be treated caution because they are based on premiums both as an output and size proxy. This is problematic because premiums do not present output but rather price times quantity of output (Yuengert, Citation1993).
A few studies that explored the nature of the relationship between life insurer size and efficiency confirm a non-linear relation (mainly U-shaped) (see Diacon et al., Citation2002; Hardwick et al., Citation2003, 2011; Ryan & Schellhorn, Citation2000; Zanghieri, Citation2009). The studies that utilised other performance measures largely revealed that larger life insurers are more profitable (see for example Akotey et al., Citation2013; Alhassan et al., Citation2015, Biener et al., Citation2016b; Browne et al., Citation2001), have lower expenses (Connelly & Limpaphayom, Citation2004; Fields et al., Citation2012, Harris and Katz, 1991; Pottier, Citation2011), are more stable (see BarNiv & Hershbarger, Citation1990; Chen & Wong, Citation2004; Eling & Jia, Citation2018) and tend to receive a higher financial strength rating (see Pottier (Citation1997) than smaller insurers.
In summary, most studies generally agree that larger life insurers are more efficient (cost, technical and revenue), profitable (mainly return on assets), stable, and have lower expenses than smaller firms. This is irrespective of different input and output variables (for efficiency-based studies), sample sizes, sample periods, countries of focus, right-hand variables and proxies thereof, and methodologies used. Nevertheless, the evidence on the effect of size on pure technical, scale, allocative and profit efficiency remains mixed.
3.1.2. Organisational form and life insurer performance
The insurance industry is characterised by diverse classes of ownership (Lamm-Tennant and Starks, 1993), including stock, mutual, reciprocal, and Lloyds’ associations, whose performance vary with context because of different incentives for contracting parties (Lai & Limpaphayom, Citation2003). Another organisational aspect that has been of interest to researchers is group affiliation. Like other firms, insurance firms may be affiliated to business groups. In the US, EU, and other insurance markets, insurance groups, where several insurers are under a common ownership, dominate as an organisational model. Although these affiliated insurers operate independently in terms of marketing, other functional areas such as information systems, investments, and policyholder services are usually managed at the group level (Cummins et al., Citation1999a). As a result, group member firms may share fixed costs, provide an environment for cross selling of products, and benefit from internal capital markets. In this regard, affiliated members may outperform their unaffiliated counterparts.
In markets such as Japan and South Korea, insurers may be affiliated with corporate groups that include manufacturing firms, trading firms, and a commercial bank, usually called the “main bank” (Jeng & Lai, Citation2005). In Japan, they are called Keiretsu (Lai & Limpaphayom, Citation2003), while in South Korea, they are known as chaebol (see Regan & Hur, Citation2007). Like other ownership forms, such organisational structures affect member firms’ financial characteristics and behaviour and hence their performance (Yanase & Limpaphayom, Citation2017). The literature shows that firms affiliated with such business groups are more profitable (Nakatani, Citation1984), have a lower risk of bankruptcy (Hoshi et al., Citation1990), are less prone to information asymmetry problems (Pinkowitz & Williamson, Citation2001), and pay lower effective taxes (Gramlich et al., Citation2004) than independent firms.
However, most studies we reviewed compared the performance of stock and mutual insurance companies. These studies tested two main theories, the expense preference hypothesis and the managerial discretion hypothesis (Cummins and Turchetti, 1996). The expense preference hypothesis suggests that stock firms outperform mutual companies because they have methods for controlling and disciplining managers, for example, through the alienability of residual claims, proxy fights, and the market for takeovers (Fama & Jensen, Citation1983a, 1983b; Mester, 1989). According to the managerial discretion theory, stock firms do better in commercial lines, which require more managerial discretion, whereas mutual firms perform well in personal lines, which require less managerial discretion (underwriting and pricing individual risk). Thus, both organizational types may be equally effective in their respective areas (Cummins et al., Citation1999a).
In total, 56 country-specific studies in our dataset explored the impact of organisational form on life insurance firms’ performance. Concerning efficiency differences between stock and mutual life insurers, the evidence is mixed for most efficiency measures. An exception is cost efficiency, where the results seem to indicate that the two organisational forms are equally efficient (see for example Cummins & Zi, Citation1998; Cummins et al., Citation2010; Gardner & Grace, Citation1993, Greene and Segal, Citation2004; Pottier, Citation2011).
However, most multi-country studies showed that mutual insurers are more efficient (see Davutyan & Klumpes, Citation2008; Diacon et al., Citation2002; Eling & Luhnen, Citation2010a; Eling & Schaper, Citation2017) and stable (see Eling & Jia, Citation2018) than stock insurers. Among studies that explored the performance of insurance companies in a multi-country setting, Biener and Eling (Citation2011) require further analysis. Biener and Eling (Citation2011) investigated the efficiency of microinsurance insurance companies operating in Africa (Benin, Burkina Faso, Congo, Mali, Senegal and Togo), Asia (Bangladesh, Cambodia, India, Indonesia and Vietnam) and Latin America (Bolivia, Guatemala, Mexico and Peru). Applying DEA in the first stage and bootstrap truncated regression in the second stage, their findings showed that profit-oriented microinsurance firms are less technically efficient than non-profit-oriented firms. These results are expected because in general, microinsurance operations have been pioneered by non-profit organisations with a social mission (Biener & Eling, Citation2011). Since these firms have been in the industry for a more extended period, they have a competitive advantage compared to new firms. It is essential to note that the microinsurers considered in this study operate in different socio-economic, political and institutional environments which the authors ignored, thus the results may be biased.
One strand of the literature focuses on the effects of demutualisation (a process of converting from being a mutual to be a stock insurer) in the US insurance industry (Erhemjamts & Leverty, Citation2010; Jeng et al., Citation2007; Xie et al., Citation2011). Based on cross-sectional regression, Jeng et al. (Citation2007) found an improvement in cost efficiency of 10 mutual insurers (relative to mutual control insurers) that demutualised between 1984 and 1995 but only under the financial intermediary approach; results from the value-added approach showed no improvement in cost efficiency,Footnote2 suggesting that the approach to measuring outputs may influence the findings. Erhemjamts and Leverty (Citation2010) analysed 40 mutual insurers that converted between 1995 and 2004 and found that the technical efficiency of demutualised insurers improved after conversion. Their study also revealed that stock insurers’ efficiency dominates that of the mutual structure during the sample period.
Xie et al. (Citation2011) investigated the effect of demutualisation on 37 insurers that converted during the period 1993–2003. They found that demutualisation enhances the cost efficiency and profitability of insurers that convert through initial public offerings (IPOs). However, the effect of demutualisation on technical efficiency was not significant. The authors also reported an insignificant effect on technical and cost efficiency of insurers that convert but remain private. It is clear from the three studies discussed above that their sample sizes and periods differ, but it is more pronounced between Jeng et al. (Citation2007) and the other two studies. This could perhaps explain why results from the former study’s value-added approach indicated an insignificant effect of demutualisation on efficiency.
A cross reading of the literature that considered the effects of different organisational forms on accounting-, market- and insolvency prediction-based measures shows inconclusive results (see Carson & Hoyt, Citation1995, 2000; Chen & McNamara, Citation2014). Similarly, the findings from the limited number of studies that examined the performance effects of other classes of ownership structures such as domestic and foreign (see Bian & Wang, Citation2017, Chen et al., Citation2009a; Huang et al., Citation2019; Kasman & Turgutlu, Citation2009; Qiu & Chen, Citation2006; Tien & Yang, Citation2014) and listed and unlisted (see Borges et al., Citation2008; Hu & Yu, Citation2015) are mixed and most of them concentrate on emerging countries.
Regarding the performance of life insurers affiliated with business groups (usually measured using a dummy variable), the literature is scant. In Taiwan, two studies (Hu & Yu, Citation2015; Huang et al., Citation2019) reported no difference in cost efficiency between affiliated (to financial holdings company) and non-affiliated insurers. Huang et al. (Citation2019) further pointed out that affiliated life insurers are more technically efficient than non-affiliated insurers but only in the marketing activity stage; they are equally technically efficient in the investment activity stage. When the authors applied the traditional SFA, the results showed no significant difference in the technical efficiency of the two organisational forms. These findings highlight the importance of adopting methods that do not treat life insurer operations as a black box.
Using dynamic DEA and bootstrap truncated regression to explore the efficiency difference between affiliated and unaffiliated life insurers, Lu et al. (Citation2014) found that group affiliation is not a significant determinant of the operating efficiency of Chinese life insurers. Yanase et al. (Citation2008), the only study that focused on Japan’s life insurance industry, explored different organisational forms of risk-taking behaviour, including Keiretsu life insurance firms. Their findings revealed that Keiretsu life insurers have lower risk (measured using the loss ratio’s standard deviation) than independent insurers. Yanase and Limpaphayom (Citation2017) confirmed these results in the Japanese non-life insurance industry using reinsurance as a risk-taking measure. Also in the non-life insurance industry, Lai and Limpaphayom (Citation2003) reported that although Keiretsu insurers have higher loss ratios, they are more profitable than independent insurers, while Jeng and Lai (Citation2005) showed that Keiretsu non-life insurers are more cost-efficient than non-specialised independent firms.
An overall assessment of the empirical evidence shows inconclusive results on the effect of organisational form on most efficiency measures except for cost efficiency, which indicates no significant differences between mutual and stock life insurers. The largely insignificant effect of organisational form on cost efficiency negates a wave of demutualisation that was witnessed in the insurance industry in the 1990s and early 2000s. However, the two studies (Erhemjamts & Leverty, Citation2010; Xie et al., Citation2011) that explored the effect of demutualisation on life insurer performance showed beneficial effects for demutualised insurers. Regarding the other performance measures, the studies also showed mixed effects of different forms of ownership. The results on the performance of affiliated and unaffiliated life insurers were inconclusive, except for the Japanese insurance industry where the results suggested that Keiretsu-affiliated insurers outperform independent firms. However, the evidence is mostly from the non-life insurance industry.
3.1.3. Diversification and life insurer performance
The diversification-performance nexus has been extensively studied in the finance literature, both theoretically and empirically. Theory indicates that diversification has both costs and benefits. On the one hand, diversification may exacerbate managerial agency problems if managers overinvest and inefficiently misallocate capital (by cross-subsidising poorly performing subsidiaries or product lines). On the other hand, diversification may enhance firm performance due to tax advantages, larger internal capital markets, risk reduction, and scope economies (see Erdorf et al., Citation2013). In the finance literature, the effect of diversification on firm performance has shown mixed findings (Kuppuswamy & Villalonga, Citation2016; Martin & Sayrak, Citation2003). However, recent research suggests that the diversification-performance relationship varies from firm to firm and that corporate diversification alone does not lead to a discount or premium but rather, its effect is heterogeneous across certain industry settings, economic conditions and governance structures (Erdorf et al., Citation2013; Kuppuswamy & Villalonga, Citation2016).
Insurers can diversify both across (life and non-life) and within (products) industries and geographically (locally, regionally or internationally). The diversification-performance debate in the life insurance industry is centred on two primary hypotheses: conglomeration and strategic focus hypotheses. The conglomeration hypothesis suggests that life insurers that offer multiple insurance and investment product lines benefit from cost scope (by sharing inputs) and revenue scope economies (resulting from the added convenience of financial supermarkets) (Berger et al., Citation2000; Meador et al., Citation1997). The hypothesis thus predicts a positive relationship between diversification and insurer performance. On the contrary, strategic focus hypothesis posits that firms maximise value by specialising in their core business and core competencies (Berger et al., Citation2000). Hence this hypothesis predicts a negative (positive) relation between diversification (concentration) and performance.
The studies that explored the effect of diversification on life insurer performance appear to vary with performance measure and methodologies and sometimes both, within and across studies and countries. In Taiwan, diversified (across products) life insurers do not perform better than specialised insurers in terms of cost, technical, and allocative efficiency (see Hao, Citation2007; Hao & Chou, Citation2005; Wang et al., Citation2007). Based on SFA and Tobit, Bian and Wang (Citation2017) found that Chinese life insurers that diversify across products are more profit-efficient than insurers that choose to specialise in one product line. Their study, however, showed no significant difference between diversified and specialised life insurers in terms of cost efficiency. Using DEA and bootstrap truncated regression, Biener et al. (Citation2016b) reported that over the period 1997–2013, internationally diversified (measured by the ratio of foreign premiums to total premiums) Swiss life insurers outperform domestically focused insurers in terms of technical, cost, and revenue efficiency. On product diversification, they only found supporting evidence for technical efficiency, although the evidence is weak.
In the US, studies that utilised the DEA methodology irrespective of the second stage estimation technique provided more evidence that product diversification is associated with lower cost (Cummins, Citation1999; Cummins et al., Citation2010), technical (Cummins, Citation1999; Cummins et al., Citation2010), revenue (Cummins et al., Citation2010; Pottier, Citation2011), and profit (Cummins et al., Citation2010) efficiency. An exception is Emm (Citation2014) who reported similar findings on cost efficiency using a different efficiency measurement technique, Varian’s Weak Axiom of Profit Maximization. Contrary to other studies, Pottier (Citation2011) reported that diversified and concentrated life insurers are equally cost- and profit-efficient. The two studies that adopted DFA to measure the US’s life insurer efficiency found that diversified insurers are more cost-efficient than their undiversified counterparts (Meador et al., Citation1997; Ryan & Schellhorn, Citation2000). Concerning whether geographically diversified outperform geographically focused insurers, Cummins et al. (Citation1999a) and Pottier (Citation2011) found conflicting evidence. Cummins et al. (Citation1999a) found that firms that operate in more than one geographic area (state) are more cost-, technical-, and revenue-efficient and experience higher total factor productivity than those that operate in one state. In contrast, Pottier (Citation2011) reported that diversification does not influence an insurer’s cost, revenue, and profit efficiency. It should be pointed out that the former study covered the period 1990–1993, while the latter covered only 2005. This could explain the differences in the findings as the relationship between two variables may not be static.
Studies that utilised non-efficiency measures of performance showed mixed results. For example, based on GMM, Olaosebikan (Citation2013) found that diversified insurers in Nigeria are more profitable than focused insurers. In Ecuador, Camino-Mogro and Bermúdez-Barrezueta (2019) reported opposite results using a panel corrected standard errors model.
In conclusion, the empirical evidence showed that the effect of diversification on life insurers’ performance is not unidirectional. The relationship may vary both within and across studies depending on the measure of performance, methodology, sample size, and time period. Therefore, the results confirmed the relevance of both the conglomeration and strategic focus hypotheses in line with the evidence from other industries, which could reflect the different institutional and economic conditions under which life insurers operate. A key point to note from the studies analysed above is that life insurance studies are yet to shift from the average effect of diversification to moderating factors in line with the current trajectory of the diversification-performance research (see de la Fuente & Velasco, Citation2015). In the corporate finance literature, for example, Kuppuswamy et al. (Citation2012) found that the value of diversified firms relative to their focused counterparts is higher in countries with less efficient labour and capital markets, while Kuppuswamy and Villalonga (Citation2016) confirmed that the value of diversified firms increased significantly during the 2007–2009 financial crisis.
3.1.4. Distribution system and life insurer performance
The choice of the distribution channel is an important strategic decision in the insurance industry (Carr et al., Citation1999). Life insurance is sold through direct writer and independent agency systems (Dionne et al., Citation2007; Ward, Citation2002). The direct writer process utilises employee sales representatives, exclusive agents, and mass marketing, whereas the independent agency system uses brokers and non-employee agents who sell for several companies. Two principal theories, market-imperfections, and product-quality theories, help explain the coexistence of these two distribution systems. According to the market imperfections hypothesis, independent agency insurers survive despite incurring higher costs than direct writers because of market imperfections such price regulation (Weiss, Citation1990), search costs, and sluggish information transmission in insurance markets (Berger et al., Citation1989). The product-quality hypothesis indicates that higher expenses of independent-agency writers reflect greater service quality or intensity, such as claims settlement services, a wider product range, and lower search costs.
Fifteen country studies in our dataset analysed the effect of the distribution system on insurers’ efficiency. Most of these studies employed a dummy variable to test the differences in performance between different distribution channels. The empirical evidence broadly showed that insurers that utilise the direct distribution system are more cost-efficient (Klumpes, Citation2004; Klumpes & Schuermann, Citation2011; Meador et al., Citation1997), profit-efficient (Klumpes, Citation2004; Klumpes & Schuermann, Citation2011), revenue-efficient (Carr et al., Citation1999; Hitt, Citation1999), technically-efficient (Chen & Chang, Citation2010; Cummins, Citation1999) and scale efficient (Chen & Chang, Citation2010) than insurers that utilise the independent agency system. In contrast, Cummins (Citation1999) reported the independent agency system to be more cost-, revenue-, and allocative-efficient than the direct writer system.
Another group of studies support the view that an insurer’s distribution system does not affect its efficiency (Chadwick & Cappelli, Citation1999; Gardner & Grace, Citation1993; Ryan & Schellhorn, Citation2000; Ward, Citation2002). Three studies (Cummins, Citation1999; Lu et al., Citation2014; Trigo-Gamarra & Growitsch, Citation2010) in our sample explored the efficiency differences between single-channel and multiple-channel insurers. Cummins (Citation1999) and Lu et al. (Citation2014) reported that multichannel insurers are efficient than single channel insurers in the US and China, respectively, while Trigo-Gamarra and Growitsch (Citation2010) found that German specialised single-channel insurers and multi-channel insurers are equally cost-and profit-efficient. Hu et al. (Citation2009) suggested that Chinese insurers that use the bancassurance and brokers channel (measured using a dummy variable) are more efficient (technical, pure technical and scale).
To summarise, the empirical studies considered different distribution arrangements which make it difficult to compare them. The studies that utilised similar distribution systems are far from reaching a consensus on whether an insurer that uses a particular system performs better. This might be because they employed different efficiency measures, methods, and focused on different jurisdictions. Also, it explains the co-existence of the different distribution systems.
3.1.5. Risk and life insurer performance
Numerous risks are inherent in the operations of life insurance firms. In finance, conventional wisdom provides that there is a trade-off between risk and return; hence, the two are positively correlated. Nevertheless, excessive risk-taking may harm performance due to increased insolvency costs (Eling et al., Citation2018; Cummins & Danzon, Citation1997; Wakker et al., Citation1997). Dionne et al. (Citation2007) argue that insurers with a higher risk of insolvency (undercapitalised) are penalised by lower insurance prices and loss of customers. Therefore, such firms may underperform firms with a lower insolvency risk.
Twenty-five studies in our database explored the effect of risk on the performance of life insurers. Two major types of risks, underwriting (measured mainly as a ratio of incurred losses to earned premiums) and investment risk (measured as a proportion of risky assets, e.g. common stock, real estate and junk bonds), have received greater attention from scholars. Almost all insolvency-prediction studies showed that insurers with a higher concentration of investments in risky assets, for example, common stock, real estate, and junk bonds (investment risk), are more likely to fail than insurers with a lower concentration (see, for instance, BarNiv & Hershbarger, Citation1990; Carson & Hoyt, Citation1995, Citation2000). Chen et al. (Citation2009b) and Killins (Citation2020) also found that investment risk hurts Chinese and Canadian insurance firms’ profitability, respectively. Similarly, Cummins et al. (Citation2010) confirmed that insurers with a higher proportion of total invested assets in stocks, mortgage loans, and real estate are less technical-, cost-, scale-, and profit-efficient. Using the ordered logit and naïve models, Pottier (Citation1997) found that life insurance firms with a higher concentration of investments in risk assets (i.e., affiliated investments, non-investment grade bonds, common stock, mortgage, and real estate) receive a lower credit rating than insurers with a lower concentration.
Most studies that considered the impact of underwriting risk on life insurer performance provided evidence of a positive relationship (see, for example, Asare et al., Citation2017; Connelly & Limpaphayom, Citation2004; Fecher et al., Citation1993; Shiu, Citation2006; Suganthi & Rajaram, Citation2016; Zainudin et al., Citation2018). Although Altuntas et al. (Citation2017) found a positive relationship between risk (measured by cash flow volatility, i.e. the variance of five years’ operating cash flows scaled by total assets) and life insurer profitability, they reported a negative association between risk and firm value (Tobin’s Q). Using SFA and 2-stage least squares, Hu and Yu (Citation2015) indicated that insurers that write more health and accident insurance business (product risk) are less cost-efficient. However, asset risk (ratio of risk-weighted assets to total assets) improves a life insurer’s efficiency.
In summary, there is agreement in the empirical literature that investment risk negatively influences performance, and that underwriting risk has a positive effect. However, results on the effect of underwriting risk (measured as losses incurred over earned premiums) ought to be treated with caution because of the long-term nature of life insurance contracts. Claims experiences captured in studies may relate to risks underwritten many years outside the study period and this may give biased results.
3.1.6. Reinsurance and life insurer performance
Reinsurance is one of the most important risk management tools available to insurers. However, its effect on performance is uncertain. A greater spread of risk through reinsurance might mean lower premiums and/or increased profitability for insurers, but it could also mean higher expenses (due to higher expenditure loading) that undermine their performance (Berry-Stölzle et al., Citation2011). Additionally, reinsurance may enhance direct insurers’ solvency (as they cede their risk) on the one hand while increasing insurers’ insolvency risk (if reinsurers fail to honor their obligations) on the other (Chen et al., Citation2001). As a result, reinsurance can have a positive or negative impact on performance.
Despite the different efficiency measures and methodologies that frontier efficiency studies employed, the evidence from developed insurance markets confirms that life insurers that purchase more reinsurance cover are inefficient (see Fecher et al., Citation1991, Citation1993; Cummins et al., Citation2010; Diacon et al., Citation2002; Cummins and Rubio-Misas, Citation2016). However, the two studies that explored the reinsurance-efficiency linkage in a multi-country context found that reinsurance improves a direct insurer’s cost efficiency (see Biener et al., Citation2016b; Cummins and Rubio-Misas, Citation2016). One of the earliest inter-country studies that examined insurers from 15 EU countries reported that reinsurance does not matter when it comes to a life insurer’s technical and scale efficiency (see Diacon et al., Citation2002). Two separate studies that focused on Ghanaian (Akotey et al., Citation2013) and Nigerian (Olaosebikan, Citation2013) life insurance industries concluded that reinsurance enhances an insurer’s profitability. In the context of India, Suganthi and Rajaram (Citation2016) and Ho (Citation2011) found that reinsurance is not an important determinant of life insurer’s profitability. Shiu (Citation2010) reported comparable results in Taiwan in terms of solvency.
Notably, all studies in our dataset utilised a premium-based reinsurance measure (ratio of ceded premiums to the sum of gross written premiums and reinsurance assumed) which reflects the amount of reinsurance an insurer desires. However, this measure ignores reinsurance recoverables, which represent the benefit of purchasing reinsurance (Venter et al., Citation2007). Also, the studies ignore the interdependence between reinsurance and life insurer performance, as well as the fact that reinsurance is endogenously influenced by choice of capital structure (MacMinn, Citation1987; Plantin, Citation2006) and risk (Dionne & Triki, Citation2013), which are some of the most commonly used right-hand variables.
To summarise, the results on the effect of reinsurance on life insurer performance vary across markets and performance measures. In developed markets, reinsurance is principally negatively associated with efficiency, while in emerging markets, profitability and solvency are independent of life insurers’ reinsurance decisions. In the case of developing countries, reinsurance purchase improves an insurer’s profitability. These results reflect the complicated trade-off between the costs and benefits of reinsurance that may vary nationally, regionally and internationally (Lei, Citation2019).
3.1.7. Capital structure and life insurer performance
The impact of capital structure on performance is unclear. According to one school of thought, leverage (capitalisation) has a positive (negative) influence on performance because it aligns managers’ and owners’ interests by alleviating free cash flow problems (Jensen, Citation1986). The other school of thought contends that more leverage increases an insurer’s risk of insolvency, which in turn, diminishes product demand (Dionne et al., Citation2007). This viewpoint predicts that leverage (capitalisation) is adversely (positively) related to performance.
In general, the literature shows mixed evidence on the effect of capital structure on life insurer performance. However, a closer examination of the studies provides some important insights. First, only inter-country, the US, and Germany studies showed that capitalisation is negatively related to life insurers’ cost efficiency (see Cummins and Rubio-Misas, Citation2016; Cummins et al., Citation2010; Eling & Luhnen, Citation2010a; Eling & Schaper, Citation2017; Hussels & Ward, Citation2007; Pottier, Citation2011; Ryan & Schellhorn, Citation2000). Second, studies that examined the life insurance markets in China (Bian & Wang, Citation2017) and the UK (see Hussels & Ward, Citation2007) reported that capitalisation is not a significant determinant of a life insurer’s cost efficiency. Third, the studies on the US and Taiwanese insurance markets indicated that highly leveraged life insurers have a higher probability of becoming insolvent (see Carson & Hoyt, Citation1995, Citation2000; Hsiao & Whang, Citation2009; Pottier, Citation1997). Finally, the evidence from emerging and developing countries showed mainly a negative (Alhassan et al., Citation2015; Chen et al., Citation2009b) or an insignificant (Akotey et al., Citation2013; Olaosebikan, Citation2013) effect of leverage on profitability. Overall, results from the literature showed that the relationship between capital structure and performance varies across countries and performance measures and is generally inconclusive for most measures.
3.2. External factors and life insurer performance
This section focuses on external determinants of life insurer performance. Three major drivers—deregulation, competition and macroeconomic conditions—have been frequently discussed in the literature.
3.2.1. Deregulation and life insurer performance
Most governments have prioritised insurance industry deregulation since the early 1990s. Deregulation of insurance markets removes price and foreign entry barriers, to boost competition (Cummins & Rubio-Misas, Citation2006). Competition is predicted to reduce insurance premiums by lowering insurers’ monopolistic power. Competition may also make insurance businesses more efficient. In competitive marketplaces, enterprises that fail to innovate and enhance efficiency may leave the market, either voluntarily or involuntarily, or through consolidations (Cummins & Rubio-Misas, Citation2006).
A few studies examined the effect of deregulation on life insurers’ performance (see Table in the appendix). For example, Jeng and Lai (Citation2008) investigated the impact of deregulation and liberalisation on Taiwanese life insurance companies’ efficiency for the period 1981–2004. They applied DEA to estimate efficiency scores and the Malmquist Index technique to measure the efficiency and productivity changes over the observation period. The evidence from both DEA and Malmquist shows that in the long run, deregulation and liberalisation have no significant adverse impact on technical, cost and revenue efficiency of domestic life insurers. Their results also indicated that although new entrants find it relatively easy to become technically efficient in the first few years of entering, they find it difficult to be cost- and revenue-efficient. This may be expected in the life insurance industry because it is costly for policyholders to switch from one insurer to the other given the long-term nature of life insurance contracts, unlike in property and casualty insurance where contracts are of a very short-term nature, usually up to one year. Besides, foreign insurers may need more time and experience to learn about the local market dynamics than domestic insurers that may be enjoying the trust, reputation, favourable tax environment and informational advantages (Cummins & Rubio-Misas, Citation2006; Fenn et al., Citation2008).
Using an SFA, Trigo-Gamarra (2008) found that following the German insurance market’s deregulation and liberalisation, life insurers’ cost and profit efficiency remained stable on average. However, the author reported that German life insurers’ total factor productivity and scale efficiency improved substantially over the observation period (1995–2002). In a related study, Hussels and Ward (Citation2007) explored the link between efficiency and regulatory change in the UK and German markets using DFA and DEA. The authors found no clear evidence linking deregulation to improved efficiency and productivity in both countries. Although the UK life insurance market evidence showed that competition drove more insurers towards the frontier, the global frontier of the stringently regulated German industry dominated the UK global frontier both before and after deregulation. For the German market, Hussels and Ward (Citation2007) considered life insurance firms that survived the entire period; thus, their results may be susceptible to survivorship bias; nevertheless, they are mainly in line with Trigo-Gamarra (2008) who utilised an unbalanced sample of 91 life insurers and a different frontier efficiency estimation technique, SFA.
In the UK and German insurance industries, Rees and Kessner (Citation1999) found a positive effect of lighter regulation and increased competition on life insurers’ technical efficiency. However, what is notable is that Rees and Kessner’s (1999) sample period, 1992–1994 May 2001not be adequate to capture the full impact of deregulation on life insurer technical efficiency. As Trigo-Gamarra (2008 p. 33) argues, “the aims of the liberalisation process to increase market efficiency significantly were not reached until 2002” in the German life insurance market.
Lee and Lin (Citation2016) reported an adverse effect of liberalisation on net written premiums and expense ratios for 1,324 life insurers in 30 OECD countries over 2004–2011. Ryan and Schellhorn (Citation2000) showed an insignificant influence of adopting a risk-based supervision framework on life insurer efficiency in the US for the 1990–1995 sample period. Boonyasai et al. (Citation2002) found an improvement in productivity following the liberalisation and deregulation of the South Korean and Philippines life insurance markets: their results showed an insignificant effect of liberalisation on Taiwanese and Thai life insurers’ productivity growth.
Overall, the empirical evidence on the impact of deregulation on life insurer performance is scant, inconclusive, and context-specific. The findings’ inconclusiveness could stem from different combinations of output measures, output and input prices, sample sizes, and methodologies employed in the literature.
3.2.2. Competition and life insurer performance
The structure—conduct–performance hypothesis (SCP), relative market power hypothesis (RMP), and efficiency structure hypothesis (ESH) underpin industrial organisation literature on competition—performance nexus. SCP argues that in concentrated market firms can collude to increase prices and thus, make huge profits. This proposition suggests that concentration improves performance. RMP contends that firms with bigger market share and differentiated products make supernormal profits by charging higher prices. Thus, market share increases performance, according to this theory. ESH proponents claim that more efficient enterprises with better management or production technologies gain market share due to lower costs (Demsetz, Citation1973; Liebenstein, Citation1966). These businesses can make more money while charging less, depending on competition (Weiss & Choi, Citation2008). Thus, ESH predicts that competition improves performance.
Three studies that explored the effects of market share on cost efficiency in the US life insurance industry based on DFA concur that this measure of competition does not matter (see Gardner & Grace, Citation1993; Meador et al., Citation1997; Ryan & Schellhorn, Citation2000). This is in contrast to studies in China (see Hu et al., Citation2009) and Taiwan (see Chuang & Tang, Citation2015; Hao & Chou, Citation2005) that reported mixed findings across similar or different methodologies and performance measures. Fenn et al. (Citation2008) investigated the effect of market structure in the EU market and concluded that insurers with a larger market share are more inefficient than insurers with a smaller market share. In a later and related study, Zanghieri (Citation2009) found a positive effect of market share on cost and profit efficiency in the EU insurance industry. What could account for differences in results between the preceding two studies despite using a similar efficiency estimation method (SFA) is that Zanghieri captured life insurers’ performance over a more extended period (1997–2006) than the 1995–2001 period that Fenn et al. (Citation2008) considered. Chadwick and Cappelli (Citation1999) and Hu et al. (Citation2009) also confirmed that in the US and China, respectively, market power (market share) is accompanied by improved efficiency.
Cummins and Rubio-Misas (Citation2016) and Eling and Schaper (Citation2017) found that concentration is negatively associated with cost efficiency in the EU insurance market. Cummins and Rubio-Misas (Citation2016) also found similar results with respect to revenue efficiency. Cummins et al. (Citation2017) also explored the effect of competition (using the Boone indicator) on the solvency and profitability of life insurers in the EU, and concluded that competition improves insurers’ solvency and profitability. They also reported a positive association between concentration (cumulative market share of the five largest life insurers in each country) and soundness. Their findings confirmed the drawbacks of relying on structural measures of competition because a highly concentrated market can be highly competitive. Browne et al. (Citation1999) found that increased competition (measured by the number of insurers) is associated with higher insolvency in the US. Alhassan et al. (Citation2015), Brokešová et al. (Citation2016) and Killins (Citation2020) found that concentration is negatively related to life insurers’ profitability in Ghana, the Slovak Republic and Canada, respectively.
Notably, results from the literature on the effect of competition on life insurer performance vary both within, and across studies, reflecting the diversity in proxies for both the dependent and independent variables, countries of focus, econometric methods and sample sizes used, among others. However, in general, evidence suggests that competition improves the performance of the life insurance industry.
3.2.3. Macroeconomic conditions and life insurer performance
The success or failure of an insurer’s underwriting and investment strategies is largely influenced by the macroeconomic conditions (Browne et al., Citation2001). A growing economy with low unemployment rates, high per capita income and stable inflation and interest rates is expected to be accompanied by an increase in demand for life insurance (Browne & Kim, Citation1993; Ma & Pope, Citation2003) and minimal disintermediation (policy surrenders and loans) problems (Browne et al., Citation1999, Citation2001). This fosters a more robust life insurer performance (Ma & Pope, Citation2003). However, an economy with poor macroeconomic fundamentals is associated with a decline in insurance demand and an increase in policy surrenders and lapses, which may adversely affect life insurance firms’ performance. Generally, most studies investigate the effect of two popular macroeconomic indicators, GDP growth and inflation on life insurer performance. Studies that adopted accounting measures dominate.
A few studies, which are all inter-country studies, confirm that macroeconomic conditions significantly influence life insurer efficiency in line with theoretical predictions although the findings vary across performance measures. One such study is Eling and Schaper (Citation2017) who examined the effects of the business environment on European life insurance companies’ efficiency and productivity for the period 2002–2013. Based on DEA and bootstrap truncated regression, they showed that growth in a country’s economy positively impacts life insurers’ cost efficiency. They also confirmed that inflation and unemployment lead to a deterioration in life insurers’ technical and cost efficiency, respectively.
Contrary to a priori expectations, the authors found a positive relationship between inflation and cost efficiency. They proffered two reasons for the result. First, they discussed it in light of anticipated and unanticipated inflation. If inflation is anticipated by management, they likely respond to it, for example, by implementing cost-cutting measures and price surcharges. Second, they argued that higher operating costs may be over-compensated by higher asset returns such as real estate investments, reducing the pressure to improve cost efficiency. Using DEA and three second-stage estimation techniques (bootstrap truncated regression, Tobit and least squares dummy variable), Biener et al. (Citation2016a) also reported a negative relationship between GDP growth and cost efficiency, suggesting that insurers are less concerned with efficiency under expansive demand conditions. The authors focused on life insurers operating in 15 countries from Europe, Asia and Oceania, including South Africa over the period 2003–2013.
Davutyan and Klumpes (Citation2008) revealed that economic growth is not a significant determinant of life insurer efficiency-technical, pure technical and scale-in seven EU countries based on DEA and fixed effects model. Cummins and Rubio-Misas (Citation2016) concluded that macroeconomic factors, specifically GDP growth and inflation, have no significant effect on European life insurers’ cost and revenue efficiency. Eling and Schaper (Citation2017) confirmed that economic growth and unemployment have no significant influence on European life insurers’ technical efficiency.
Like frontier efficiency-based studies, only a few studies that employed other performance measures reported that macroeconomic factors are important drivers of life insurer performance. Among the earliest studies are Browne et al. (Citation1999, Citation2001). Browne et al. (Citation1999) used Poisson regression analysis to determine the economic and market predictors of insolvency in the US life-health insurance industry. They found that unemployment and disposable income are positively related to life insurer insolvency. Although the effect of disposable income on insolvency appears counter-intuitive, it is in fact in line with the alternative hypothesis that posits that “increased sales result in greater leverage and a drain on surplus” (Browne et al., Citation1999 p. 648). Browne et al. (Citation2001) concluded that a person’s disposable income is positively related to profitability, while unanticipated inflation is negatively associated with profitability.
Akotey et al. (Citation2013) confirmed a negative effect of inflation on life insurer profitability in Ghana. Similarly, Shiu (Citation2005) reported a negative association between inflation and solvency in the UK life insurance industry for the period 1986–1999. In general, most non-frontier efficiency studies reported that GDP growth (see Akotey et al., Citation2013; Alhassan et al., Citation2015; Biener et al., Citation2016a; Cummins et al., Citation2017) and inflation (see Cummins et al., Citation2017; Olaosebikan, Citation2013, Camino-Mogro and Bermúdez-Barrezueta, 2019; Browne et al., Citation2001) have an insignificant effect on life insurer profitability. Two studies that investigated the impact of economic (GDP) growth on life insurers’ performance in Ghana (Alhassan et al., Citation2015) and Nigeria (Olaosebikan, Citation2013) are noteworthy because of their sample periods that may not adequately capture the effects of GDP growth.
To conclude, most studies provide evidence that macroeconomic factors have no significant influence on life insurer performance. These studies are mainly based on profitability measures. All the frontier efficiency studies explored the effects of macroeconomic conditions in a multi-country context, while studies that considered other performance measures are mainly country-specific studies.
4. Summary and conclusions
This study provides an overview of studies that discuss the determinants of the performance of life insurance companies. We reviewed 128 studies published from 1990 through December 2021. The study provides a description of our search strategy and data sources as well as an analysis of the characteristics of the papers, including measures, methodological approaches and geographic distribution. The majority of the studies we reviewed concentrated on a few jurisdictions (i.e. the US, the UK, Taiwan, Germany and China) and are based on frontier efficiency methodologies (71 studies, 55.7%) dominated by the classic DEA model in the first stage and Tobit and OLS in the second stage. Studies that utilised accounting ratios (40 studies, 31.3%) represent the second most popular stream of the literature we examined. This stream has mainly employed pooled OLS, fixed effects and random effects panel data econometric techniques.
Our examination of the empirical evidence indicates that size, organisational form, capital structure, diversification, distribution system, risk and reinsurance are the major firm-level determinants of life insurer performance, while competition and macroeconomic factors are the main external drivers. The literature suggests that insurer size is positively related to performance. However, it also shows no significant differences between the performance of mutual and stock life insurers. Regarding the effect of risk on performance, investment risk has a negative effect, while underwriting risk has a positive influence. The effect of reinsurance on performance varies with regions: its effect is positive in developing countries, negative in developed countries and insignificant in emerging countries. Highly competitive markets appear to improve life insurer performance. The literature also shows that highly capitalised (leveraged) insurers are less efficient (have a higher risk of insolvency), especially in the US. Overall results indicate that macroeconomic factors appear not to be important drivers of life insurer performance. Other factors show mixed results: they reported a positive or negative or insignificant effect. The ambiguity in results could arise from heterogeneity in proxies for performance measures, determinants, methodologies employed, sample sizes and periods.
The study has important practical implications. Given that the effect of some factors, particularly reinsurance, tends to vary from region to region, the results cannot be generalised, hence more specific studies are needed, particularly in developing countries. Policymakers should enact laws that promote competition in the industry. Given that larger life insurers are more cost efficient than smaller insurers, there might be a need to promote mergers and acquisitions among smaller and medium-sized insurers. This would encourage healthy competition in the industry. The issue of solvency should be of concern to regulators and supervisors, therefore there should be widespread adoption of risk-based supervisory frameworks across markets, especially in developing markets. As the evidence suggests, investment risk has detrimental effects on solvency and efficiency of life insurers. This implies that the share of risky assets in the portfolio should be restricted in the interest of policyholders. Also, the role of reinsurance in solvency frameworks need to be re-examined given that results from the literature show a positive effect on profitability (in developing countries) and a negative effect on efficiency (in developed countries).
This study reveals several research gaps. First, we identify gaps related to methodologies. Most frontier efficiency-based studies that applied the DEA model in the first stage, are based on classic DEA (except Krupa et al. (Citation2019) and Lu et al. (Citation2014), who applied the slacks-based measure in Germany and China, respectively), which ignores the internal structure of the insurance management process (Tone et al., Citation2019). Future studies could apply newly developed two-stage DEA models that consider the interrelationship among sub-structures within the system, such as network DEA, dynamic network DEA, and dynamic slacks-based measure with network structure. Lu et al. (Citation2014) confirm that DNSBM efficiency scores are significantly different from those of traditional DEA. Huang et al. (Citation2019) confirm similar results using network SFA. It would be more revealing to examine the extent to which the performance determinants vary with each stage.
Second, the effect of diversification on life insurer performance requires further empirical investigation. Researchers could investigate the conditions under which diversification can add value to life insurers. Studies could also examine the non-linear relation between diversification and life insurer performance using methods such as quantile regression.
Third, we observed that studies exploring the effect of reinsurance on performance often used a premium-based measure of reinsurance that does not consider reinsurance recoverables. Therefore, future studies, subject to data availability, could consider using premiums ceded scaled by reinsurance recoverables or reinsurance recoverables scaled by ceded premiums as a measure of net reinsurance cost, in line with Lei (Citation2019).
Fourth, the literature fails to consider the interdependence between reinsurance and performance. Scholars paid less attention to the fact that reinsurance is also endogenously influenced by the choice of capital structure and risk. Therefore, any study that could account for the potential endogeneity of these variables would make a significant contribution to knowledge.
Fifth, studies that explored the effect of competition on life insurer performance relied mainly on structural measures of competition such as market share, number of companies and concentration ratios. This leaves a huge gap on the direct impact of competition on life insurer performance. This calls for further studies that utilise non-structural measures of competition such as the Boone indicator, Lerner index and Panzar-Rosse H-statistic.
Sixth, the effect of corporate governance on life insurer performance has received little attention in the literature. What would be of valuable contribution is the effect of corporate governance on cash holdings.
Finally, we notice that very few studies have examined life insurance firms’ performance in developing countries, especially in Africa and Latin America. An understanding of the determinants of life insurer performance would give important insights given the adoption of new technologies, risk-based supervision frameworks, corporate governance guidelines, risk management systems and the drive by governments and policy-makers in these markets to promote the development of the insurance sector.
Disclosure statement
No potential conflict of interest was reported by the authors.
Additional information
Notes on contributors
Tafadzwanashe Zinyoro
Tafadzwanashe Zinyoro is a PhD Candidate (Development Finance) at Stellenbosch University Business School. His thesis is titled ‘Insurer Performance and its Determinants: Evidence from Selected African Countries.’ His research interests encompass the efficiency and productivity of insurers and other financial service providers, the regulation of insurance markets, inclusive insurance, as well as climate and disaster risk financing and insurance.
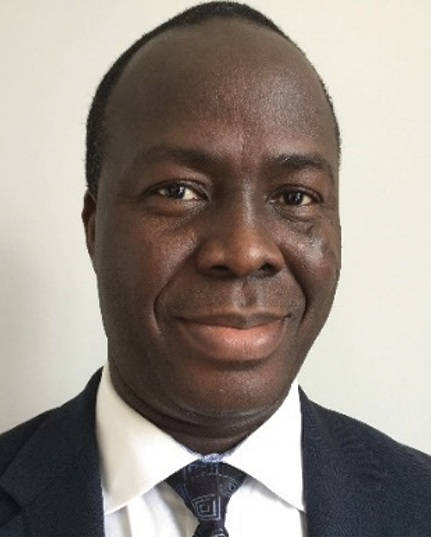
Meshach Jesse Aziakpono
Meshach Aziakpono is a Professor of Economics in the Department of Economics and Economic History at Rhodes University, South Africa. Before joining Rhodes University, he was a Professor of Development Finance and the Head of Development Finance programmes at Stellenbosch Business School. He holds PhD degree in Economics from the University of the Free State, Bloemfontein in South Africa. His PhD thesis titled: “The Depth of Financial Integration and its Effects on Financial Development and Economic Performance of the Southern African Customs Union Countries” won the Founders’ Medal for the best PhD dissertation in Economics in South Africa. He has published over 40 papers in international peer-reviewed journals and chapters of books.
Notes
1. Underwriting cycles simply refer to periods when the supply of insurance coverage is abundant as prices and profitability fall (soft market) and when the supply of insurance shrinks as prices and profits increase (hard market).
2. See Cummins and Weiss (Citation2013) for a discussion of the financial intermediary and value-added approaches.
References
- Akotey, J. O., Sackey, F. G., Amoah, L., & Manso, R. F. (2013). The financial performance of life insurance companies in Ghana. The Journal of Risk Finance, 14(3), 286–50. https://doi.org/10.1108/JRF-11-2012-0081
- Akpan, S. S., Mahat, F., Bany-Ariffin, N., & Nassir, A. (2021). Performance effect of risk-based capital policy on insurers: Longitudinal microdata evidence. Journal of African Business, 22(1), 85–105. https://doi.org/10.1080/15228916.2019.1695181
- Alhassan, A. L., Addisson, G. K., & Asamoah, M. E. (2015). Market structure, efficiency and profitability of insurance companies in Ghana. International Journal of Emerging Markets, 10(4), 648–669. https://doi.org/10.1108/IJoEM-06-2014-0173
- Alhassan, A. L., Zyambo, K., & Afua Boakye, M. A. (2021). ‘Welcome on board’: Resource dependency and agency theoretic evidence from the South African life insurance market. Corporate Governance the International Journal of Business in Society, 21(4), 626–644. https://doi.org/10.1108/CG-12-2019-0375
- Altuntas, M., Liebenberg, A. P., Watson, E. D., & Yildiz, S. (2017). Hedging, cash flows, and firm value: Evidence of an indirect effect. Journal of Insurance Issues, 40(1), 1–22.
- Ambrose, J. M., & Carroll, A. M. (1994). Using best’s ratings in life insurer insolvency prediction. The Journal of Risk and Insurance, 61(2), 317–327. https://doi.org/10.2307/253713
- Amel, D., Barnes, C., Panetta, F., & Salleo, C. (2004). Consolidation and efficiency in the financial sector: A review of the international evidence. Journal of Banking and Finance, 28(10), 2493–2519. https://doi.org/10.1016/j.jbankfin.2003.10.013
- Asare, N., Alhassan, A. L., Asamoah, M. E., & Ntow–Gyamfi, M. (2017). Intellectual capital and profitability in an emerging insurance market. Journal of Economic and Administrative Sciences, 33(1), 2–19. https://doi.org/10.1108/JEAS-06-2016-0016
- BarNiv, R., & Hershbarger, R. A. (1990). Classifying financial distress in the life insurance industry. The Journal of Risk and Insurance, 57(1), 110–136. https://doi.org/10.2307/252927
- Battese, G. E., & Coelli, T. J. (1995). A model for technical inefficiency effects in a stochastic frontier production function for panel data. Empirical Economics, 20(2), 325–332. https://doi.org/10.1007/BF01205442
- Bauer, P. W., Berger, A. N., Ferrier, G. D., & Humphrey, D. B. (1998). Consistency conditions for regulatory analysis of financial institutions: A comparison of frontier efficiency methods. Journal of Economics and Business, 50(2), 85–114. https://doi.org/10.1016/S0148-6195(97)00072-6
- Berger, A. N., Cummins, J. D., Weiss, M. A., & Zi, H. (2000). Conglomeration versus strategic focus: Evidence from the insurance industry. Journal of Financial Intermediation, 9(4), 323–362. https://doi.org/10.1006/jfin.2000.0295
- Berger, L. A., Kleindorfer, P. R., & Kunreuther, H. (1989). A dynamic model of the transmission of price information in auto insurance markets. The Journal of Risk and Insurance, 56(1), 17–33. https://doi.org/10.2307/253012
- Berry-Stölzle, T. R., Weiss, M. A., & Wende, S. (2011). Market structure, efficiency, and performance in the European property-liability insurance industry. In ARIA 2011 Meeting. Retrieved from http://citeseerx.ist.psu.edu/viewdoc/download?doi=10.1.1.386.7220andrep=rep1andtype=pdf.
- Bian, W., & Wang, X. (2017). The openness of China’s insurance industry and the efficiency of domestic vs. foreign life insurers. Asia–Pacific Journal of Accounting and Economics, 26(6), 731–746. https://doi.org/10.1080/16081625.2017.1404919
- Biener, C., & Eling, M. (2011). The performance of microinsurance programs: A data envelopment analysis. The Journal of Risk and Insurance, 78(1), 83–115. https://doi.org/10.1111/j.1539-6975.2010.01404.x
- Biener, C., Eling, M., & Jia, R. (2016a). The roles of industry idiosyncrasy, cost efficiency, and risk in internationalization: Evidence from the insurance industry. University of St. Gallen, School of Finance Research Paper, (2016/2). Retrieved from SSRN https://papers.ssrn.com/sol3/papers.cfm?abstract_id=2722426
- Biener, C., Eling, M., & Wirfs, J. H. (2016b). The determinants of efficiency and productivity in the Swiss insurance industry. European Journal of Operational Research, 248(2), 703–714. https://doi.org/10.1016/j.ejor.2015.07.055
- Boonyasai, T., Grace, M. F., & Skipper, H. D., Jr. (2002). The effect of liberalization and deregulation on life insurer efficiency (Working paper no. 02–2). Centre for Risk Management and Insurance Research, Georgia State University, Retrieved from https://www.researchgate.net/profile/Harold_Skipper/publication/241760718_The_Effect_of_Liberalization_and_Deregulation_on_Life_Insurer_Efficiency/links/0deec5304a54db6c70000000.pdf
- Borges, M. R., Nektarios, M., & Barros, C. P. (2008). Analysing the efficiency of the Greek life insurance industry. European Research Studies, 11(3), 1–18.
- Brewer, E., III, Carson, J. M., Elyasiani, E., Mansur, I., & Scott, W. L. (2007). Interest rate risk and equity values of life insurance companies: A GARCH–M model. The Journal of Risk and Insurance, 74(2), 401–423. https://doi.org/10.1111/j.1539-6975.2007.00218.x
- Brokešová, Z., Ondruška, T., Pastoráková, E., & Péliová, J. (2016). Industry concentration and performance: Case of life insurance industry. In T. Dudycz, G. Osbert-Pociecha, & B. Brycz (Eds.), The essence and measurement of organizational efficiency (pp. 29–37). Springer International Publishing. https://doi.org/10.1007/978-3-319-21139-8_3
- Browne, M. J., Carson, J. M., & Hoyt, R. E. (1999). Economic and market predictors of insolvencies in the life–health insurance industry. The Journal of Risk and Insurance, 66(4), 643–659. https://doi.org/10.2307/253868
- Browne, M. J., Carson, J. M., & Hoyt, R. E. (2001). Dynamic financial models of life insurers. North American Actuarial Journal, 5(2), 11–26. https://doi.org/10.1080/10920277.2001.10595981
- Browne, M. J., & Kim, K. (1993). An international analysis of life insurance demand. The Journal of Risk and Insurance, 60(4), 616–634. https://doi.org/10.2307/253382
- Camino-Mogro, S., Armijos-Bravo, G., & Cornejo-Marcos, G. (2019). Competition in the insurance industry in Ecuador: An econometric analysis in life and non-life markets. The Quarterly Review of Economics & Finance, 71, 291–302. https://doi.org/10.1016/j.qref.2018.10.001
- Carr, R. M., Cummins, J. D., & Regan, L. (1999). Efficiency and competitiveness in the US life insurance industry: Corporate, product, and distribution strategies. In J. D. Cummins (Ed.), Changes in the Life Insurance Industry: Efficiency, technology and risk management (pp. 117–157). Springer. https://doi.org/10.1007/978-1-4615-5045-7_4
- Carson, J. M., & Hoyt, R. E. (1995). Life insurer financial distress: Classification models and empirical evidence. The Journal of Risk and Insurance, 62(4), 764–775. https://doi.org/10.2307/253595
- Carson, J. M., & Hoyt, R. E. (2000). Evaluating the risk of life insurer insolvency: Implications from the US for the European Union. Journal of Multinational Financial Management, 10(3–4), 297–314. https://doi.org/10.1016/S1042-444X(00)00023-2
- Chadwick, C., & Cappelli, P. (1999). Alternatives to generic strategy typologies in strategic human resource management. In P. M. Wright, L. D. Dyer, J. W. Boudreau, & G. T. Milkovich (Eds.), Research in personnel and human resources management (Vol. Supplement 4, pp. 1–29). JAI Press, Inc.
- Chandrapal, J. D. (2019). Impact of liberalisation on Indian life insurance industry: A truly multivariate approach. IIMB Management Review, 31(3), 283–297. https://doi.org/10.1016/j.iimb.2019.03.003
- Chen, M. S., & Chang, P. L. (2010). Distribution channel strategy and efficiency performance of the life insurance industry in Taiwan. Journal of Financial Services Marketing, 15(1), 62–75. https://doi.org/10.1057/fsm.2010.6
- Chen, J. S., Chen, M. C., Liao, W. J., & Chen, T. H. (2009b). Influence of capital structure and operational risk on profitability of life insurance industry in Taiwan. Journal of Modelling in Management, 4(1), 7–18. https://doi.org/10.1108/17465660910943720
- Chen, L., Eckles, D. L., & Pottier, S. W. (2013). Ownership form and efficiency: The coexistence of stock and mutual life insurers. Journal of Insurance Issues, 36(2), 121–148.
- Chen, Y., Hamwi, I. S., & Hudson, T. (2001). The effect of ceded reinsurance on solvency of primary insurers. International Advances in Economic Research, 7(1), 65–82. https://doi.org/10.1007/BF02296592
- Chen, L. R., & McNamara, M. J. (2014). An examination of the relative efficiency of fraternal insurers. Journal of Insurance Issues, 37(1), 1–31.
- Chen, B., Powers, M. R., & Qiu, J. (2009a). Life‐insurance efficiency in China: A comparison of foreign and domestic firms. China & World Economy, 17(6), 43–63. https://doi.org/10.1111/j.1749-124X.2009.01173.x
- Chen, R., & Wong, K. A. (2004). The determinants of financial health of Asian insurance companies. The Journal of Risk and Insurance, 71(3), 469–499. https://doi.org/10.1111/j.0022-4367.2004.00099.x
- Chen, R., Wong, K. A., & Lee, H. C. (1999). Underwriting cycles in Asia. The Journal of Risk and Insurance, 66(1), 29–47. https://doi.org/10.2307/253876
- Chuang, C. C., & Tang, Y. C. (2015). Asymmetric dependence between efficiency and market power in the Taiwanese life insurance industry. Panoeconomicus, 62(4), 511–525. https://doi.org/10.2298/PAN1504511C
- Collins, J. H., Geisler, G. G., & Shackelford, D. A. (1997). The effects of taxes, regulation, earnings, and organizational form on life insurers’ investment portfolio realizations. Journal of Accounting and Economics, 24(3), 337–361. https://doi.org/10.1016/S0165-4101(98)00011-1
- Connelly, J. T., & Limpaphayom, P. (2004). Board characteristics and firm performance: Evidence from the life insurance industry in Thailand. Chulalongkorn Journal of Economics, 16(2), 101–124.
- Cummins, J. D. (1999). Efficiency in the US life insurance industry: Are insurers minimizing costs and maximizing revenues? In J. D. Cummins (Ed.), Changes in the Life Insurance Industry: Efficiency, technology and risk management (pp. 75–115). Springer. https://doi.org/10.1007/978-1-4615-5045-7_3
- Cummins, J. D., & Danzon, P. M. (1997). Price, financial quality, and capital flows in insurance markets. Journal of Financial Intermediation, 6(1), 3–38. https://doi.org/10.1006/jfin.1996.0205
- Cummins, J. D., Klumpes, P., & Weiss, M. A. (2015). Mergers and acquisitions in the global insurance industry: Valuation effects. The Geneva Papers on Risk and Insurance–Issues and Practice, 40(3), 444–473. https://doi.org/10.1057/gpp.2015.18
- Cummins, J. D., & Phillips, R. D. (2009). Capital adequacy and insurance risk–based capital systems. Journal of Insurance Regulation, 28(1), 25–72.
- Cummins, J. D., & Rubio–Misas, M. (2016). Integration and efficiency convergence in European life insurance markets. Working Paper.
- Cummins, J. D., Rubio–Misas, M., & Vencappa, D. (2017). Competition, efficiency and soundness in European life insurance markets. Journal of Financial Stability, 28, 66–78. https://doi.org/10.1016/j.jfs.2016.11.007
- Cummins, J. D., & Rubio-Misas, M. (2006). Deregulation, consolidation, and efficiency: Evidence from the Spanish insurance industry. Journal of Money, Credit, and Banking, 38(2), 323–355. https://doi.org/10.1353/mcb.2006.0029
- Cummins, J. D., & Rubio-Misas, M. (2021). Country factor behavior for integration improvement of European life insurance markets. Economic Analysis & Policy, 72, 186–202. https://doi.org/10.1016/j.eap.2021.08.004
- Cummins, J. D., Tennyson, S., & Weiss, M. A. (1999a). Consolidation and efficiency in the US life insurance industry. Journal of Banking and Finance, 23(2–4), 325–357. https://doi.org/10.1016/S0378-4266(98)00089-2
- Cummins, J. D., & Weiss, M. A. (2000). Analyzing firm performance in the insurance industry using frontier efficiency and productivity methods. In G. Dionne (Ed.), Handbook of insurance (pp. 767–829). Springer Netherlands. https://doi.org/10.1007/978-94-010-0642-2_24
- Cummins, J. D., & Weiss, M. A. (2013). Analyzing firm performance in the insurance industry using frontier efficiency and productivity methods. In G. Dionne (Ed.), Handbook of insurance (pp. 795–861). Springer New York. https://doi.org/10.1007/978-1-4614-0155-1_28
- Cummins, J. D., & Weiss, M. A. (2014). Systemic risk and the US insurance sector. The Journal of Risk and Insurance, 81(3), 489–528. https://doi.org/10.1111/jori.12039
- Cummins, J. D., Weiss, M. A., Xie, X., & Zi, H. (2010). Economies of scope in financial services: A DEA efficiency analysis of the US insurance industry. Journal of Banking and Finance, 34(7), 1525–1539. https://doi.org/10.1016/j.jbankfin.2010.02.025
- Cummins, J. D., Weiss, M. A., & Zi, H. (1999b). Organizational form and efficiency: The coexistence of stock and mutual property-liability insurers. Management Science, 45(9), 1254–1269. https://doi.org/10.1287/mnsc.45.9.1254
- Cummins, J. D., & Xie, X. (2013). Efficiency, productivity, and scale economies in the US property-liability insurance industry. Journal of Productivity Analysis, 39(2), 141–164. https://doi.org/10.1007/s11123-012-0302-2
- Cummins, J. D., & Zi, H. (1998). Comparison of frontier efficiency methods: An application to the U.S. Life insurance industry. Journal of Productivity Analysis, 10(2), 131–152. https://doi.org/10.1023/A:1026402922367
- Danquah, M., Otoo, D. M., & Baah‐Nuakoh, A. (2018). Cost efficiency of insurance firms in Ghana. Managerial and Decision Economics, 39(2), 213–225. https://doi.org/10.1002/mde.2897
- Davutyan, N., & Klumpes, P. J. (2008). Consolidation and efficiency in the major European insurance markets: A non–discretionary inputs approach. Conference paper, Imperial College Business School, held 4-5 July.
- de la Fuente, G., & Velasco, P. (2015). The effect of the financial crisis on the value of corporate diversification in Spanish firms. Spanish Journal of Finance and Accounting/Revista Espanola de Financiacion Y Contabilidad, 44(1), 1–23. https://doi.org/10.1080/02102412.2014.982385
- Demsetz, H. (1973). Industry structure, market rivalry, and public policy. Journal of Law & Economics, 16(1), 1–9. https://doi.org/10.1086/466752
- Diacon, S. R., Starkey, K., & O’Brien, C. (2002). Size and efficiency in European long–term insurance companies: An International comparison. Geneva Papers on Risk and Insurance – Issues and Practice, 27(3), 444–466. https://doi.org/10.1111/1468-0440.00184
- Dionne, G., Gagné, R., & Nouira, A. (2007). Determinants of insurers’ performance in risk pooling, risk management, and financial intermediation activities (Cahier de recherche/Working Paper 07–15) HEC Montreal. Retrieved from http://citeseerx.ist.psu.edu/viewdoc/download?doi=10.1.1.954.5804andrep=rep1andtype=pdf
- Dionne, G., & Triki, T. (2013). On risk management determinants: What really matters? European Journal of Finance, 19(2), 145–164. https://doi.org/10.1080/1351847X.2012.664156
- Eling, M., Hoyt, R. E., & Schaper, P. (2020). The impact of capacity on price and productivity change in insurance markets. Journal of Insurance Issues, 43(1), 22–58.
- Eling, M., & Jia, R. (2018). Business failure, efficiency, and volatility: Evidence from the European insurance industry. International Review of Financial Analysis, 59, 58–76. https://doi.org/10.1016/j.irfa.2018.07.007
- Eling, M., & Luhnen, M. (2010a). Efficiency in the international insurance industry: A cross–country comparison. Journal of Banking and Finance, 34(7), 1497–1509. https://doi.org/10.1016/j.jbankfin.2009.08.026
- Eling, M., & Luhnen, M. (2010b). Frontier efficiency methodologies to measure performance in the insurance industry: Overview, systematization, and recent developments. The Geneva Papers on Risk and Insurance – Issues and Practice, 35(2), 217–265. https://doi.org/10.1057/gpp.2010.1
- Eling, M., & Schaper, P. (2017). Under pressure: How the business environment affects productivity and efficiency of European life insurance companies. European Journal of Operational Research, 258(3), 1082–1094. https://doi.org/10.1016/j.ejor.2016.08.070
- Emm, E. E. (2014). Intra–industry diversification and firm efficiency: A study of the US insurance industry. International Review of Accounting, Banking and Finance, 6(1), 1–43.
- Ennsfellner, K. C., Lewis, D., & Anderson, R. I. (2004). Production efficiency in the Austrian insurance industry: A Bayesian examination. The Journal of Risk and Insurance, 71(1), 135–159. https://doi.org/10.1111/j.0022-4367.2004.00082.x
- Erdorf, S., Hartmann-Wendels, T., Heinrichs, N., & Matz, M. (2013). Corporate diversification and firm value: A survey of recent literature. Financial Markets and Portfolio Management, 27(2), 187–215. https://doi.org/10.1007/s11408-013-0209-6
- Erhemjamts, O., & Leverty, J. T. (2010). The demise of the mutual organizational form: An investigation of the life insurance industry. Journal of Money, Credit, and Banking, 42(6), 1011–1036. https://doi.org/10.1111/j.1538-4616.2010.00318.x
- Fama, E. F., & Jensen, M. C. (1983a). Agency problems and residual claims. Journal of Law & Economics, 26(2), 327–349. https://doi.org/10.1086/467038
- Fecher, F., Kessler, D., Perelman, S., & Pestieau, P. (1993). Productive performance of the French insurance industry. Journal of Productivity Analysis, 4(1), 77–93. https://doi.org/10.1007/BF01073467
- Fecher, F., Perelman, S., & Pestieau, P. (1991). Scale economies and performance in the French insurance industry. The Geneva Papers on Risk and Insurance–Issues and Practice, 16(3), 315–326. https://doi.org/10.1057/gpp.1991.23
- Fenn, P., Vencappa, D., Diacon, S., Klumpes, P., & O’Brien, C. (2008). Market structure and the efficiency of European insurance companies: A stochastic frontier analysis. Journal of Banking and Finance, 32(1), 86–100. https://doi.org/10.1016/j.jbankfin.2007.09.005
- Fields, L. P., Gupta, M., & Prakash, P. (2012). Risk taking and performance of public insurers: An international comparison. The Journal of Risk and Insurance, 79(4), 931–962. https://doi.org/10.1111/j.1539-6975.2012.01479.x
- Fukuyama, H. (1997). Investigating productive efficiency and productivity changes of Japanese life insurance companies. Pacific–Basin Finance Journal, 5(4), 481–509. https://doi.org/10.1016/S0927-538X(97)00016-4
- Gardner, L. A., & Grace, M. F. (1993). X–efficiency in the US life insurance industry. Journal of Banking and Finance, 17(2–3), 497–510. https://doi.org/10.1016/0378-4266(93)90048-I
- Gramlich, J. D., Limpaphayom, P., & Rhee, S. G. (2004). Taxes, Keiretsu affiliation, and income shifting. Journal of Accounting and Economics, 37(2), 203–228. https://doi.org/10.1016/j.jacceco.2003.10.001
- Greene, W. H., & Segal, D. (2004). Profitability and efficiency in the US life insurance industry. Journal of Productivity Analysis, 21(3), 229–247. https://doi.org/10.1023/B:PROD.0000022092.70204.fa
- Hao, J. C. (2007). Efficiency test on Taiwan’s life insurance industry-using X-efficiency approach. Information and Management Sciences, 18(1), 37–48.
- Hao, J. C., & Chou, L. Y. (2005). The estimation of efficiency for life insurance industry: The case in Taiwan. Journal of Asian Economics, 16(5), 847–860. https://doi.org/10.1016/j.asieco.2005.08.004
- Hardwick, P. (1997). Measuring cost inefficiency in the UK life insurance industry. Applied Financial Economics, 7(1), 37–44. https://doi.org/10.1080/096031097333835
- Hardwick, P., Adams, M., & Zou, H. (2003). Corporate governance and cost efficiency in the United Kingdom life insurance industry (EBMS working paper EBMS/2003/1). European Business Management School,
- Hardwick, P., Adams, M., & Zou, H. (2011). Board characteristics and profit efficiency in the United Kingdom life insurance industry. Journal of Business Finance & Accounting, 38(7‐8), 987–1015. https://doi.org/10.1111/j.1468-5957.2011.02255.x
- Hitt, L. M. (1999). The impact of information technology management practices on the performance of life insurance companies. In J. D. Cummins & A. M. Santomero (Eds.), Changes in the Life Insurance Industry: Efficiency, technology and risk management (pp. 211–243). Springer US. https://doi.org/10.1007/978-1-4615-5045-7_7
- Ho, C. L. (2011). Board composition, risk management and corporate performance in the Taiwanese life insurance industry. IUP Journal of Risk and Insurance, 8(4), 7–27.
- Hoff, A. (2007). Second stage DEA: Comparison of approaches for modelling the DEA score. European Journal of Operational Research, 181(1), 425–435. https://doi.org/10.1016/j.ejor.2006.05.019
- Ho-Li, Y. (2007). The effect of financial independence on the performances of life companies: An empirical study. International Journal of Management, 24(3), 522–540.
- Hoshi, T., Kashyap, A., & Scharfstein, D. (1990). The role of banks in reducing the costs of financial distress in Japan. Journal of Financial Economics, 27(1), 67–88. https://doi.org/10.1016/0304-405X(90)90021-Q
- Hoyt, R. E., & Trieschmann, J. S. (1991). Risk/Return relationships for life–health, property–liability, and diversified insurers. The Journal of Risk and Insurance, 58(2), 322–330. https://doi.org/10.2307/253240
- Hsiao, S. H., & Whang, T. J. (2009). A study of financial insolvency prediction model for life insurers. Expert Systems with Applications, 36(3), 6100–6107. https://doi.org/10.1016/j.eswa.2008.07.024
- Huang, L. Y., Hsiao, T. Y., & Lai, G. C. (2007). Does corporate governance and ownership structure influence performance? Evidence from Taiwan life insurance companies. Journal of Insurance Issues, 30(2), 123–151.
- Huang, T. H., Lin, C. I., & Wu, R. C. (2019). Assessing the marketing and investment efficiency of Taiwan’s life insurance firms under network structures. The Quarterly Review of Economics & Finance, 71, 132–147. https://doi.org/10.1016/j.qref.2018.07.002
- Hussels, S., & Ward, D. R. (2007). The impact of deregulation on the German and UK life insurance markets: An analysis of efficiency and productivity between 1991–2002. (Working paper). Cranfield University School of Management. Retrieved from https://dspace.lib.cranfield.ac.uk/handle/1826/3947
- Hu, J. L., & Yu, H. E. (2015). Risk, capital, and operating efficiency: Evidence from Taiwan’s life insurance market. Emerging Markets Finance & Trade, 51(sup1), S121–S132. https://doi.org/10.1080/1540496X.2014.998907
- Hu, X., Zhang, C., Hu, J. L., & Zhu, N. (2009). Analysing efficiency in the Chinese life insurance industry. Management Research News, 32(10), 905–920. https://doi.org/10.1108/01409170910994123
- Jeng, V., & Lai, G. C. (2005). Ownership structure, agency costs, specialization, and efficiency: Analysis of Keiretsu and independent insurers in the Japanese nonlife insurance industry. The Journal of Risk and Insurance, 72(1), 105–158. https://doi.org/10.1111/j.0022-4367.2005.00118.x
- Jeng, V., & Lai, G. C. (2008). The impact of deregulation on efficiency: An analysis of life insurance industry in Taiwan from 1981 to 2004. Risk Management and Insurance Review, 11(2), 349–375. https://doi.org/10.1111/j.1540-6296.2008.00144.x
- Jeng, V., Lai, G. C., & McNamara, M. J. (2007). Efficiency and demutualization: Evidence from the US life insurance industry in the 1980s and 1990s. The Journal of Risk and Insurance, 74(3), 683–711. https://doi.org/10.1111/j.1539-6975.2007.00230.x
- Jensen, M. C. (1986). Agency costs of free cash flow, corporate finance, and takeovers. The American Economic Review, 76(2), 323–329.
- Kaffash, S., Azizi, R., Huang, Y., & Zhu, J. (2020). A survey of data envelopment analysis applications in the insurance industry 1993–2018. European Journal of Operational Research, 284(3), 801–813. https://doi.org/10.1016/j.ejor.2019.07.034
- Kasman, A., & Turgutlu, E. (2009). Total factor productivity in the Turkish insurance industry. International Journal of the Economics of Business, 16(2), 239–247. https://doi.org/10.1080/13571510902917558
- Katrishen, F. A., & Scordis, N. A. (1998). Economies of scale in services: A study of multinational insurers. Journal of International Business Studies, 29(2), 305–323. https://doi.org/10.1057/palgrave.jibs.8490038
- Killins, R. N. (2020). Firm-specific, industry-specific and macroeconomic factors of life insurers’ profitability: Evidence from Canada. The North American Journal of Economics & Finance, 51, 1–16. https://doi.org/10.1016/j.najef.2019.101068
- Klumpes, P. J. M. (2004). Performance benchmarking in financial services: Evidence from the UK life insurance industry. The Journal of Business, 77(2), 257–273. https://doi.org/10.1086/381281
- Klumpes, P. J. M. (2022). Consolidation and efficiency in the major European insurance markets. Working Paper, Aaborg University.
- Klumpes, P. J., & Schuermann, S. (2011). Corporate, product and distribution strategies in the European life insurance industry. The Geneva Papers on Risk and Insurance–Issues and Practice, 36(1), 50–75. https://doi.org/10.1057/gpp.2010.38
- Krupa, T., Farbarzevics, K., & Salame, B. (2019). Investment efficiency of life insurance companies in Germany: Application of a two–stage SBM. Contemporary Economy, 10(1), 79–91. https://doi.org/10.26881/wg.2019.1.08
- Kuppuswamy, V., Serafeim, G., & Villalonga, B. (2012). The effect of institutional factors on the value of corporate diversification. Working Paper, Harvard Business School.
- Kuppuswamy, V., & Villalonga, B. (2016). Does diversification create value in the presence of external financing constraints? Evidence from the 2007–2009 financial crisis. Management Science, 62(4), 905–923. https://doi.org/10.1287/mnsc.2015.2165
- Lai, G. C., & Limpaphayom, P. (2003). Organizational structure and performance: Evidence from the nonlife insurance industry in Japan. The Journal of Risk and Insurance, 70(4), 735–757. https://doi.org/10.1046/j.0022-4367.2003.00073.x
- Lei, Y. (2019). Reinsurance and insurers’ risk-return profile. Journal of Insurance Issues, 42(1), 37–65.
- Leverty, T., Lin, Y., & Zhou, H. (2004). Firm performance in the Chinese insurance industry. Working paper, Georgia State University.
- Liebenstein, H. (1966). Allocative efficiency v. X–inefficiency. The American Economic Review, 56(3), 392–415.
- Lu, W. M., Wang, W. K., & Kweh, Q. L. (2014). Intellectual capital and performance in the Chinese life insurance industry. Omega, 42(1), 65–74. https://doi.org/10.1016/j.omega.2013.03.002
- MacMinn, R. D. (1987). Insurance and corporate risk management. The Journal of Risk and Insurance, 54(4), 658–677. https://doi.org/10.2307/253115
- Maichel–Guggemoos, L., & Wagner, J. (2019). Balancing growth, profitability and safety in the German insurance market. Zeitschrift für die Gesamte Versicherungswissenschaft, 108(5), 421–442. https://doi.org/10.1007/s12297-019-00439-8
- Ma, Y. L., & Pope, N. (2003). Determinants of international insurers’ participation in foreign non‐life markets. The Journal of Risk and Insurance, 70(2), 235–248. https://doi.org/10.1111/1539-6975.00058
- Martin, J. D., & Sayrak, A. (2003). Corporate diversification and shareholder value: A survey of recent literature. Journal of Corporate Finance, 9(1), 37–57. https://doi.org/10.1016/S0929-1199(01)00053-0
- McDonald, J. (2009). Using least squares and tobit in second stage DEA efficiency analyses. European Journal of Operational Research, 197(2), 792–798. https://doi.org/10.1016/j.ejor.2008.07.039
- Meador, J. W., Ryan, H. E., Jr., & Schellhorn, C. D. (1997). Product focus versus diversification: Estimates of x–efficiency for the US life insurance industry (Working Paper). The Wharton School, University of Pennsylvania. Retrieved from https://www.researchgate.net/publication/2590758_Product_Focus_Versus_Diversification_Estimates_of_X-Efficiency_for_the_US_Life_Insurance_Industry
- Moreno, I., Parrado‐Martínez, P., & Trujillo‐Ponce, A. (2020). Economic crisis and determinants of solvency in the insurance sector: New evidence from Spain. Accounting & Finance, 60(3), 2965–2994. https://doi.org/10.1111/acfi.12422
- Nakatani, I. (1984). The economic role of financial corporate grouping. In M. Aoki (Ed.), The economic analysis of the Japanese firm (pp. 227–258). Elsevier Science Ltd, North-Holland.
- Nguyen, D. K., & Vo, D. T. (2020). Enterprise risk management and solvency: The case of the listed EU insurers. Journal of Business Research, 113, 360–369. https://doi.org/10.1016/j.jbusres.2019.09.034
- O’Donnell, C. J., Rao, D. P., & Battese, G. E. (2008). Metafrontier frameworks for the study of firm-level efficiencies and technology ratios. Empirical Economics, 34(2), 231–255. https://doi.org/10.1007/s00181-007-0119-4
- Olaosebikan, O. (2013). The determinants of the profitability of micro–life insurers in Nigeria. The Geneva Papers on Risk and Insurance–Issues and Practice, 38(1), 140–159. https://doi.org/10.1057/gpp.2012.18
- Owadally, I., Zhou, F., Otunba, R., Lin, J., & Wright, D. (2019). Time series data mining with an application to the measurement of underwriting cycles. North American Actuarial Journal, 23(3), 469–484. https://doi.org/10.1080/10920277.2019.1570468
- Pinkowitz, L., & Williamson, R. (2001). Bank power and cash holdings: Evidence from Japan. The Review of Financial Studies, 14(4), 1059–1082. https://doi.org/10.1093/rfs/14.4.1059
- Plantin, G. (2006). Does reinsurance need reinsurers. Journal of Risk Insurance, 73(1), 153–168. https://doi.org/10.1111/j.1539-6975.2006.00169.x
- Pottier, S. W. (1997). Life insurer risk characteristics and the rating process. Journal of Insurance Issues, 20(2), 111–130.
- Pottier, S. W. (2011). Life insurer efficiency and state regulation: Evidence of optimal firm behavior. Journal of Regulatory Economics, 39(2), 169–193. https://doi.org/10.1007/s11149-010-9139-7
- Qiu, S., & Chen, B. (2006). Efficiencies of life insurers in China—an application of data envelopment analysis. Working Paper.
- Rees, R., & Kessner, E. (1999). Regulation and efficiency in European insurance markets. Economic Policy, 14(29), 364–397. https://doi.org/10.1111/1468-0327.00053
- Regan, L., & Hur, Y. (2007). On the corporate demand for insurance: The case of Korean nonfinancial firms. The Journal of Risk and Insurance, 74(4), 829–850. https://doi.org/10.1111/j.1539-6975.2007.00236.x
- Ryan, H. E., Jr., & Schellhorn, C. D. (2000). Life insurer cost efficiency before and after implementation of the NAIC–risk based capital standards. Journal of Insurance Regulation, 18(3), 362–382.
- Scherbaum, C. A., & Ferreter, J. M. (2009). Estimating statistical power and required sample sizes for organizational research using multilevel modeling. Organizational Research Methods, 12(2), 347–367. https://doi.org/10.1177/1094428107308906
- Shaddady, A. (2022). Business environment, political risk, governance, shariah compliance and efficiency in insurance companies in the MENA region. The Geneva Papers on Risk and Insurance-Issues and Practice, 47(4), 861–904. https://doi.org/10.1057/s41288-021-00232-8
- Shiu, Y. M. (2005). The determinants of solvency in the United Kingdom life insurance market. Applied Economics Letters, 12(6), 339–344. https://doi.org/10.1080/13504850500092640
- Shiu, Y. M. (2006). Corporate liquidity: Evidence from the United Kingdom life insurance industry. Applied Economics Letters, 13(15), 993–998. https://doi.org/10.1080/13504850500425790
- Shiu, Y. M. (2009). Economic factors, firm characteristics and performance: A panel data analysis for United Kingdom life offices. Applied Economics Letters, 16(10), 1033–1037. https://doi.org/10.1080/13504850701335269
- Shiu, Y. M. (2010). Derivative hedging and insurer solvency: Evidence from Taiwan. The Geneva Papers on Risk and Insurance–Issues and Practice, 35(3), 469–483. https://doi.org/10.1057/gpp.2010.13
- Simar, L., & Wilson, P. W. (2011). Two-stage DEA: Caveat emptor. Journal of Productivity Analysis, 36(2), 205–218. https://doi.org/10.1007/s11123-011-0230-6
- Skipper, H. D. (1997). Foreign insurers in emerging markets: Issues and concerns. International Insurance Foundation. Working paper. Retrieved from http://citeseerx.ist.psu.edu/viewdoc/download?doi=10.1.1.539.4747andrep=rep1andtype=pdf
- Skogh, G. (1982). Public insurance and accident prevention. International Review of Law and Economics, 2(1), 67–80. https://doi.org/10.1016/0144-8188(82)90014-X
- Suganthi, P., & Rajaram, S. (2016). Determinants of financial performance of Indian life insurance sector: Panel evidence. The IUP Journal of Applied Finance, 22(4), 76–89.
- Swiss Re Institute. (2019) Explore and visualise sigma data on natural catastrophes and the world insurance markets. Retrieved from www.sigma-explorer.com
- Tien, J. J., & Yang, S. S. (2014). The determinants of life insurer’s growth for a developing insurance market: Domestic vs foreign insurance firms. The Geneva Papers on Risk and Insurance–Issues and Practice, 39(1), 1–24. https://doi.org/10.1057/gpp.2013.6
- Tone, K., Kweh, Q. L., Lu, W. M., & Ting, I. W. K. (2019). Modeling investments in the dynamic network performance of insurance companies. Omega, 88, 237–247. https://doi.org/10.1016/j.omega.2018.09.005
- Trigo Gamarra, L. (2008). The effects of liberalization and deregulation on the performance of financial institutions: The case of the German life insurance market. Working Paper No. 93, University of Rostock, Institute of Economics.
- Trigo-Gamarra, L., & Growitsch, C. (2010). Comparing single–and multichannel distribution strategies in the German life insurance market: An analysis of cost and profit. Schmalenbach Business Review, 62(4), 401–417. https://doi.org/10.1007/BF03396813
- Venter, G. G., Gluck, S. M., & Brehm, P. J. (2007). Measuring value in reinsurance. In P. J. Brehm, G. R. Perry, G. G. Venter, & E. W. Susan (Eds.), Enterprise risk analysis for property and liability insurance companies (pp. 179–199). Guy Carpenter and Company.
- Wakker, P., Thaler, R., & Tversky, A. (1997). Probabilistic insurance. Journal of Risk and Uncertainty, 15(1), 7–28. https://doi.org/10.1023/A:1007799303256
- Ward, D. (2002). The costs of distribution in the UK life insurance market. Applied Economics, 34(15), 1959–1968. https://doi.org/10.1080/00036840210129428
- Weiss, L. A. (1990). Bankruptcy resolution: Direct costs and violation of priority of claims. Journal of Financial Economics, 27(2), 285–314. https://doi.org/10.1016/0304-405X(90)90058-8
- Weiss, M. A. (2007). Underwriting cycles: A synthesis and further directions. Journal of Insurance Issues, 30(1), 31–45.
- Weiss, M. A., & Choi, B. P. (2008). State regulation and the structure, conduct, efficiency and performance of US auto insurers. Journal of Banking and Finance, 32(1), 134–156. https://doi.org/10.1016/j.jbankfin.2007.09.010
- Wu, D., Yang, Z., Vela, S., & Liang, L. (2007). Simultaneous analysis of production and investment performance of Canadian life and health insurance companies using data envelopment analysis. Computers & Operations Research, 34(1), 180–198. https://doi.org/10.1016/j.cor.2005.05.005
- Xie, X., Lu, W., Reising, J., & Stohs, M. H. (2011). Demutualisation, control and efficiency in the US life insurance industry. The Geneva Papers on Risk and Insurance–Issues and Practice, 36(2), 197–225. https://doi.org/10.1057/gpp.2011.6
- Yanase, N., Asai, Y., & Lai, G. (2008). Organisational structure and risk taking: Evidence from the life insurance industry in Japan. Conference Paper, APRIA 12th Annual Conference.
- Yanase, N., & Limpaphayom, P. (2017). Organization structure and corporate demand for reinsurance: The case of the Japanese Keiretsu. The Journal of Risk and Insurance, 84(2), 599–629. https://doi.org/10.1111/jori.12092
- Yuengert, A. M. (1993). The measurement of efficiency in life insurance: Estimates of a mixed normal–gamma error model. Journal of Banking and Finance, 17(2/3), 483–496. https://doi.org/10.1016/0378-4266(93)90047-H
- Zainudin, R., Mahdzan, N. S. A., & Leong, E. S. (2018). Firm–specific internal determinants of profitability performance: An exploratory study of selected life insurance firms in Asia. Journal of Asia Business Studies, 12(4), 533–550. https://doi.org/10.1108/JABS-09-2016-0129
- Zanghieri, P. (2009). Efficiency of European insurance companies: Do local factors matter? (Working Paper). Working Paper.
- Zhang, L., Nielson, N. L., & Haley, J. (2019). Risk and return determinants of US insurers. The International Journal of Business and Finance Research, 13(1), 63–72.
Appendix 1.
Effect of firm-level and external factors on life insurer performance
Table A1. Summary of the findings on the direct influence of firm-level and external factors on insurer performance
Appendix 2.
Characteristics of studies that examined drivers of life insurer performance.
Table A2. Summary of studies that examined drivers of life insurer performance