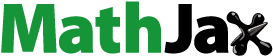
Abstract
This study explores the impact of revenue diversification and intellectual capital on the performance of sub-Saharan African banks. The study applied a panel regression technique to a data set containing information on 80 banks from 21 countries from 2000 to 2020. Secondary data was collected from the unconsolidated financial statements of banks in the Bank scope database, WDI database, and Refinitiv database. The study utilized the two-step system GMM and the dynamic panel threshold regression estimation methods to evaluate the hypotheses. The study revealed that income diversification has a negative and significant impact on the profitability of banks in Sub-Saharan Africa. The study also indicated that value-added intellectual coefficient (VAIC), human capital efficiency (HCE), and structural capital efficiency (SCE) have a positive and significant effect on the performance of banks; however, capital employed efficiency (CEE) has a negative and significant impact on banks’ profitability. The study also revealed that VAIC and HCE positively and significantly moderate the relationship between income diversification and the profitability of banks. SCE has a positive and insignificant moderating effect on the relationship between income diversification and bank performance. The study also revealed that CEE negatively and significantly mediates the relationship between income diversification and banks’ profitability. The threshold results also provide evidence that VAIC and HCE positively affect profitability; however, VAIC and HCE affect profitability above the threshold value. We also found evidence of a threshold effect of SCE below the threshold and a negative effect above the threshold value. The study also revealed that CEE negatively affects banks’ profitability at all levels. The detrimental impact of income diversification on the performance of sub-Saharan banks should be a concern for bank managers. The findings are crucial to comprehending the banking industry developments in Sub-Saharan Africa (SSA) from 2000 to 2020. The results provide important insights into the significance of the development of intellectual capital for the diversification of banking activities in SSA.
1. Introduction
In the past few decades, the financial sector of both developing and developed nations have undergone significant changes. Deregulation, rising competition, and globalization have enhanced banks’ focus on traditional interest-based operations and diversified business lines (Ikeora et al., Citation2016; Li, Citation2019). The financial reforms that have characterized African nations have forced banks to adopt a strategy for obtaining funds from non-traditional banking activities (Abuzayed et al., Citation2018; Adesina, Citation2021; Boadi, Citation2018). Nyantakyi-Frimpong (Citation2017) concludes that Africa’s banking sector became as competitive as those in high-income Organisation for Economic Co-operation and Development (OECD) nations and the Caribbean and Latin America region because of these financial reforms.
Significant advances in the banking industries of both developing and developed nations have caused banks to shift their focus to income diversification and intellectual capital for competitive advantage and enhanced financial performance. Diversification is seen as part of the extreme gap in bank behaviour in the post-crisis era (Hidayat et al., Citation2012; Le et al., Citation2022; Nguyen, Citation2019; Wang & Lin, Citation2021; Zou & Xinyi Cai, Citation2021). The bank will diversify its asset portfolio by incorporating new revenue streams or assets, such as investment provision, brokerage services, stock trading, and underwriting services (Adesina, Citation2021; Buch & Drages, Citation2018; Meslier et al., Citation2014). A well-diversified bank is expected to yield higher financial returns on its portfolio of investments (Olarewaju et al., Citation2018). This is because incomes from different sources, whether uncorrelated or imperfectly correlated, result in steady and stable streams of overall bank profits (Nisar et al., Citation2018). In banking literature, it is known that income diversification generally reduces the risks of loan failure (Duho et al., Citation2021; Hamid & Ibrahim, Citation2020; Sharma & Anand, Citation2018). This strategy leads to greater diversification of income sources, which might help banks reduce risks and stabilize profits. However, banking institutions may reach a point of disintermediation by expanding non-interest product activities. These activities are associated with much higher risks than other income sources and could destabilize individual banks and the entire banking system (Brahmana et al., Citation2018a; Tariq et al., Citation2021).
Researchers have also discovered that intangible resources, such as intellectual capital, are just as crucial to modern economies as financial and physical capital (Majumder et al., Citation2023; Nguyen et al., Citation2023; North & Kumta, Citation2018). Intellectual capital is essential in banks’ financial performance and in creating value (Le et al., Citation2020; Vo & Tran, Citation2021; Yaseen & Al-Amarneh, Citation2021). Studies revealed that banks with high intellectual capital tend to focus on the traditional banking business and provide it with high quality, agility, and speed (Abuzayed et al., Citation2018). According to proponents of resource-based and knowledge-based views, income diversification (IC) is a firm-specific resource that is priceless, uncommon, unique, and non-substitutable. As a result, IC has a greater positive impact on an organization’s financial performance and competitive advantage than real resources (Khan et al., Citation2019; Valaei et al., Citation2022). As a result, managers and stakeholders are now demonstrating a more substantial interest in monitoring, appraising, and disclosing their stock of intangible assets as crucial performance indicators and sources of long-term competitive advantage (Nguyen et al., Citation2023). Since IC is a multifaceted resource of expertise, information, and practical skills, it is, therefore, necessary to examine how banks’ intellectual capital efforts drive their diversification strategy (Căpraru et al., Citation2020; Carnes et al., Citation2017; Feng et al., Citation2017). To the best of our knowledge, this is the first study to shed new insight by investigating the moderating role of intellectual capital on the relationship between income diversification and bank performance in SSA using the VAIC technique and its components developed by Pulic (Citation2000). The study hypothesized that;
Ho1:
Income diversification does not significantly affect banks’ profitability in SSA.
Ho2:
Intellectual capital does not significantly affect banks’ profitability in SSA.
Ho3:
Intellectual capital does not significantly mediate the relationship between income diversification and banks’ profitability in SSA.
Ho4:
Intellectual capital has no non-linear effect on the relationship between income diversification and banks’ profitability in SSA.
The present empirical research fills the gaps in contemporary literature in various ways. First, unlike previous studies (Amidu & Wolfe, Citation2013; Gafrej & Boujelbéne, Citation2021; Suseno et al., Citation2019; Ur Rehman et al., Citation2022), this paper comprehensively examines whether IC moderates the relationship between income diversification and banks’ profitability in SSA. We pay particular attention to the SSA because it has less developed financial systems and is perceived as risky due to uncertainties with government policy, a low quality of infrastructure, and political instability (Asiedu, Citation2002). Banks in emerging markets like SSA are less mature and have different regulatory systems than advanced economies. Analysing their income diversification response to banks’ profitability can help make policy recommendations. Moreover, the results from the developed economy may not apply to developing economies in SSA. Second, another meaningful deliberation that the literature has failed to consider is the breakdown of IC into their respective components, such as human capital efficiency (HCE), structural capital efficiency (SCE), and physical capital employed efficiency (CEE), as proposed by Pulic (Pulic, Citation1998, Citation2000, Citation2004, Citation2008). This study disaggregates IC into its components to avoid aggregation bias (Pulic, 2008), since their impact and interaction with income diversification on banks’ profitability in SSA may vary. In so doing, appropriate policy directions can be proposed and promoted to enhance banks’ profitability in SSA. Finally, in contrast to previous studies, this paper also used the Two-Step System GMM and the Seo et al. (Citation2019) dynamic panel threshold regression, which have not been used in a single study to investigate these relationships. The study employs the Seo et al. (Citation2019) dynamic panel threshold regression technique to estimate whether IC has an a non-linear impact on the relationship between income diversification and profitability of banks in SSA, which may differ at certain levels of intellectual capital. This is because the institutional environment, financial systems, and banks’ intellectual capital may differ across economies in SSA. The impact of IC on the relationship between income diversification and banks’ profitability may not be constant from bank to bank over time due to differences in the value creation of banks.
The remaining sections are structured as follows: Section 2 provides a review of the theoretical and empirical literature on income diversification, IC, and banks’ profitability; Section 3 describes the study design, empirical model specification, and data source; and Section 4 provides an analysis and discussion of the results. The conclusions and policy recommendations were provided in the investigation’s final section.
2. Literature review
2.1. Theoretical underpinnings
Four significant theories, namely the Portfolio theory, resource-based view, conglomerate theory and strategic focus hypothesis, are the foundation for the relationship between revenue diversification, intellectual capital, and banks’ profitability.
2.1.1. Portfolio theory
According to Markowitz’s (Citation1952) portfolio theory, investors must diversify to optimize the expected return on investments. If the investor decides to invest in two commodities at the same risk of return, the value of his investments will remain unchanged (Ferreira et al., Citation2018). The Portfolio theory indicates that a well-diversified portfolio will positively impact banks’ profitability and efficiency. By referring to portfolio theory, Elsas et al. (Citation2010) argue that banks will benefit from economies of scale, gradually decreasing banks’ risk and increasing profitability. The portfolio theory was used in previous empirical income diversification studies (Elsas et al., Citation2010; Githaiga, Citation2020a; Le & McMillan, Citation2021; Uddin et al., Citation2021). The modern portfolio theory is hinged on this study because by diversifying income sources, banks in SSA can optimize the expected return of the portfolio for a certain level of risk or subsequently reduce the risk for a specific rate of expected return by carefully selecting the dimensions of various assets. This theory indicates that where diversification is well implemented as a performance improvement strategy, it may enable banks to attain a competitive advantage by improving the financial performance of banks.
2.1.2. Resource-based view
Penrose (Citation1960) first introduced the resource-based view in her book “The Theory of the Growth of the Firm. The theory examines how firms create sustainable competitive advantage through managing and deploying internal resources. According to Barney (Citation1991), firm resources are all assets, capabilities, processes, attributes, information, knowledge, etc., controlled by a firm. In an era of knowledge-based economies, banks must utilise their knowledge resources optimally for competitive advantage and superior performance (Albert & Dieter, Citation2003; Bontis, Citation2001; Guthrie, Citation2001; Serenko & Bontis, Citation2004). Banks should leverage their intellectual capital resources and capabilities towards activities where they gain a competitive advantage relative to competitors, irrespective of size and geographical location (Kolachi & Shah, Citation2013). The resource-based view theory was used by previous researchers (Amidu & Wolfe, 2013; Boadi, Citation2018; Githaiga, 2020a; Akkas & Asutay, Citation2022b; Ur Rehman et al., Citation2022). The RBV is significant to this study because the unique and valuable human capital, structural capital, and capital employed resources can significantly impact a bank’s competitive advantage and overall performance in the region. By leveraging these resources effectively, banks can differentiate themselves from competitors and achieve sustained success in a challenging market environment.
2.1.3. Conglomeration hypothesis
Proponents of the conglomeration hypothesis argue that owning and operating a broad range of businesses can add value by exploiting economies of scope by sharing inputs in joint production (Teece, Citation1980), which conforms with Markowitz’s portfolio theory. Conglomeration may diversify risk, reducing the expected costs of financial distress or bankruptcy, lowering greater financial leverage, and permitting firms to earn higher revenues from risk-sensitive customers willing to pay more or accept reduced services in return for lower default risk (Lewellen, Citation1971).
2.1.4. Strategic focus
In contrast, proponents of the strategic focus hypothesis contend that enterprises can maximize value by concentrating on their core businesses and expertise. According to the strategic-focus theory, income diversity correlates with high-profit volatility, agency expenses, high monitoring costs, and difficulty monitoring. This means that income diversification may also retard the financial performance of banks instead of promoting it. According to this hypothesis, conglomeration may reflect agency problems in which managers may add businesses to protect the value of their human capital (Amihud & Lev, Citation1981) or to increase their private benefits (Jensen, Citation1986). The strategic focus and the conglomeration hypothesis were used by Boadi (Citation2018b), who studied income diversification and bank performance from an African market perspective.
The strategic focus hypothesis contradicts income diversification, suggesting a non-linear relationship between income diversification and banks’ performance (Boadi, Citation2018). These opposing views have led to empirical research into the effects of income diversification on bank profitability. Still, empirical evidence shows that the influence of income diversification on banks’ profitability can be either direct or through an interactive variable. To this end, studies on the direct impact of income diversification on banks’ profitability are still inconclusive. While some studies show that income diversification enhances banks’ performance (Amidu & Wolfe, Citation2013; Gafrej & Boujelbéne, Citation2021; Githaiga, Citation2020a; Hunjra et al., Citation2020; Luu et al., Citation2020; Nisar et al., Citation2018; Olarewaju et al., Citation2018; Sharma & Anand, Citation2018, Citation2020), others show contrary results (Abuzayed et al., Citation2018a; Carl & Duho, Citation2020; Cuong et al., 2020), which is creating conflicting findings. Other scholars emphasised intervening variables such as experience, bank size, and ownership structure (Espinosa-Méndez et al., Citation2021; Luu et al., Citation2019; Quyen et al., Citation2021), financial crisis (Quyen et al., Citation2021), competition (Boamah et al., Citation2022), capital ratio (Brighi & Venturelli, Citation2014), corporate governance (Fajriyanti et al., Citation2021; Liang et al., Citation2020), capital structure (Jouida & Hellara, Citation2018), and market concentration (Shim, 2019), among others, whereas intellectual capital (IC) has not been considered in Sub-Saharan Africa (SSA).
2.2. Related studies
2.2.1. Income diversification and banks’ profitability
The income structure of banks has significantly changed from interest income sources to include non-traditional income activities. In response, a pool of studies has emerged examining why banks have diversified their income sources and the impact of such diversification on their performance (Meng et al., Citation2018). Although the effects of income diversification on banks’ performance are well addressed in the literature, there is no consensus. Some evidence (Ahamed, Citation2017; Nisar et al., Citation2018) shows the presence of economies of scale, while others (Addai et al., Citation2022; Lee et al., Citation2020; Stiroh & Rumble, Citation2006) portray diseconomies of income diversification.
While some studies report that banks benefit from income diversification (Brahmana et al., Citation2018b; Ahamed, Citation2017; Vidyarthi, Citation2020), others lament that income diversification ruins bank profitability (Francis et al., Citation2018; Lee et al., Citation2020; Stiroh & Rumble, Citation2006). Chiorazzo et al. (Citation2008) found that non-interest income significantly improves risk-adjusted returns. Their results also showed an inverted U-shape association between risk-adjusted profit and bank size. Beyond its optimal size, an increase in bank size only led to a fall in risk-adjusted profit. The direct relation between risk-adjusted returns and non-interest income activities was stronger for larger banks, which afforded bigger banks stability in income. Performance improvements were also found with an increase in non-interest income activities generally. Similarly, Brahmana et al. (Citation2018a) found that diversification improves the performance of Malaysian banks. The other evidence on emerging markets also reveals that income diversification improves banks’ performance (Ishak & Napier, Citation2006; Meslier et al., Citation2014; Nguyen et al., Citation2015; Sanya & Wolfe, Citation2011). Some of these reports show that diversification leads to an increase in bank profitability (Ahamed, Citation2017), cost efficiency (Doan et al., Citation2018), and financial stability (Köhler, Citation2015; Yu et al., Citation2014). Huynh et al. (Citation2021) found that income diversification does not reduce shareholder value but somewhat improves bank profitability. Similarly, Ahamed (Citation2017) finds that a shift towards non-traditional banking activities increased the profitability of Indian banks during the 1998–2014 period. Nisar et al. (Citation2018) discovered that diversification of revenue into noninterest streams generally positively impacts the profitability and stability of commercial banks domiciled in countries in South Asia. Addai et al. (Citation2022) study examines foreign banks’ heterogeneity in income diversification and performance nexus. The study finds that increased income diversification improves banks’ performance, and the global and emerging banks perform better than the regional African and domestic banks. Regarding how different foreign banks benefit from income diversification, the study finds that global banks benefit more than their counterparts operating in the region. The emerging country banks outperform the African and domestic banks, while the local banks in the region benefit from income diversification more than the regional African banks.
Conversely, some studies from developed regions have shown that income diversification worsens the performance of financial institutions. Stiroh (Citation2004) asserts that increased revenue from fees leads to a deteriorating trade-off between risk and return results. Moreover, Deyoung and Roland (Citation2001) provide empirical proof that banks witness a drop in performance when they diversify their income streams into non-traditional activities instead of the usual banking operations. Drawing on their study of banks in Italy, Acharya et al. (Citation2006) conclude that banks, through asset diversification, cannot achieve superior performance and risk reduction. Esho et al. (Citation2005) conclude from the prevailing evidence that revenue increases stemming from transaction fees tend to increase risk and shrink returns. In contrast, activities that lead to a rise in revenue from residential lending reduce both risk and return. Francis et al. (Citation2018) reveal that diversification (focus) at the asset, industry, and borrower levels decreases returns. However, once banks’ screening and monitoring abilities are controlled, the effect of diversification/focus either gets weaker or disappears. Stiroh and Rumble (Citation2006) find that diversification activities worsen financial performance.
2.2.2. Intellectual capital and profitability of banks
The first empirical study of intellectual capital was conducted by Pulic (Citation1998), which examined IC’s effect on firm performance. Previous research on the association between IC (as assessed by the value-added intellectual coefficient, VAIC) and bank performance has yielded conflicting results. Ozkan et al. (Citation2017) conducted a survey examining IC’s influence on bank performance in Turkish. The VAIC was used to calculate IC, which was then disaggregated into human capital efficiency, structural capital efficiency, and capital-employed efficiency. The study discovered that HCE and CEE had a large and beneficial influence on bank profitability, but SCE had no effect. Ousama et al. (Citation2020) examined the impact of IC on the bank performance of Islamic banks. The study used a sample of 37 banks and annual financial data from 2011 to 2013. The results indicate that both HCE and CEE had a beneficial and statistically significant impact on performance. However, SCE had no appreciable effect on bank performance. The purpose of the Ur Rehman et al. (Citation2022) study was to shed light on the extent of intellectual capital efficiency (ICE) and the relationship between its three components (human capital efficiency, structural capital efficiency, and relational capital efficiency) and Islamic banking performance (in terms of return on assets, return on equity, and Tobin’s q) in Muslim countries. The researchers develop hypotheses on this relationship, drawing on resource-based theory. The study employs the two-step generalized method of moments (2SYS-GMM) estimator to analyze the data collected from 129 Islamic banks in 29 Muslim countries from 2008 to 2017. The study provides evidence that Islamic banks’ (IBs) performance is driven primarily by investment in ICE. The results indicate that SCE and relational capital efficiency (RCE) are the essential drivers of value in achieving high performance at Islamic banks. The results reveal that HCE negatively affects the performance of IBs. Ghosh and Maji (Citation2015) empirically investigate the validity of the basic propositions of the VAIC and extend VAIC models in the Indian knowledge-based sector. Using panel data relating to 62 firms from two Indian knowledge-based sectors, namely, the electronics and banking sectors, for a period of 10 years (from 2001–2002 to 2010–2011), the study indicates that the VAIC model cannot be rejected as a technique technique for measuring intellectual capital. The result shows that VAIC significantly and positively influences corporate performance as measured by return on assets (ROA) and market-to-book (M/B) ratio. All the components of VAIC except structural capital (SC) and SCE significantly and positively influence corporate performance. Inkinen (Citation2015) reviewed empirical research on intellectual capital and firm performance. The findings demonstrate that IC affects firm performance mainly through interactions, combinations, and mediations. Maji and Hussain's (Citation2021) study on technical efficiency, intellectual capital efficiency, and bank performance in emerging markets in India also observed a positive influence of IC on bank performance, which indicates the relevance of intellectual resources in enhancing banks’ value.
Tiwari and Vidyarthi’s (Citation2018) paper also explored and explained the linkage between banks’ intellectual capital efficiency and performance using 39 public and private banks listed on the Bombay Stock Exchange from 1999 to 2015. Results of the study provide evidence of a positive association between IC and the performance of banks; however, only HCE and SCE have shown significant positive linkage with banks’ performance. The results also indicate that the IC efficiency of private-sector banks is better than public-sector banks in India. In another related study, Tiwari et al. (Citation2023) empirically investigated the influence of intellectual capital on changes in the total factor productivity of 36 BSE-listed banks in India from 2005 to 2019. According to the findings, productivity growth is primarily driven by efficiency rather than technological changes. Furthermore, regression results show that the IC index and its two sub-components, HCE and CEE, strongly impact bank productivity. In another related study in Vietnam, Vo and Tran (Citation2021) examined the effect of intellectual capital on bank performance. Their paper decomposes intellectual capital into CEE, HCE and SCE. The paper uses an unbalanced panel dataset on 14 listed banks in Vietnam for 2009–2018, for which required data are available, with the generalized method of moments. The findings indicate that intellectual capital contributes significantly and positively to bank performance in Vietnam. In addition, bank performance is driven primarily by capital-employed efficiency. Although human capital efficiency contributes positively to bank performance, the effect seems marginal. Using VAIC, Yaseen and Al-Amarneh’s (Citation2021) study aims to investigate the impact of IC on the performance of Jordanian banks listed in the Amman Stock Exchange (ASE) during 2005–2018. Two empirical models were designed to test the effect of VAIC and its three components, including CEE, HCE, and SCE, on banking performance. The study results show a significant and positive relationship between VAIC and banks’ profitability as measured by return on assets (ROA). Meanwhile, when VAIC is split into components, SCE, CEE, and HCE significantly and positively impact banks’ performance. Yet, CEE has more influence on performance compared to HCE and SCE.
Soewarno and Tjahjadi (Citation2020) investigated the impact of IC on the financial performance of publicly traded Indonesian banks. The study results demonstrate that HCE had a negative, though slight, influence on ROA, whereas SCE and CEE positively influenced ROA. The study showed that physical capital is more important to Indonesian banking organizations than intangible assets like human capital. In Ghana, Onumah and Duho (Citation2020) investigated the impact of intellectual capital on bank efficiency in emerging markets. Using the VAIC model of Pulic (Citation2000) and its additive components, HCE, SCE, and CEE, to measure IC and data envelopment analysis to estimate efficiency scores, the study used Ghanaian data from 32 banks from 2000 to 2015 to examine the nexus. The study found that IC instigates efficiency in banks, borne largely from HCE, indicating the prevalence of the human capital theory. The results of the impact of SCE and CEE are insignificant except for the significant positive effect of CEE on profit efficiency. Alhassan and Asare (Citation2016) investigated the impact of intellectual capital on bank productivity in the rising market of Africa. A sample of 18 Ghanaians and panel data from 2003 to 2011 were utilized. HCE and CEE had a good and significant influence on bank productivity. Furthermore, Maji and Hussain (Citation2021), employing Indian banks, found that the results show that IC positively impacts bank performance. This was supported by Natsir and Bangun (Citation2021; 2022, Citation2022), who also revealed that IC positively influences the performance and success of a firm. Given the conflicting results from banks worldwide, further research into the relationship between intellectual capital and bank profitability between SSA is required using GMM dynamic and the dynamic panel threshold regression, which has not been used in a single study to provide insight.
3. Methodology
3.1. Data and information references
Secondary data on the profitability of banks (ROA), intellectual capital (human capital, structural capital, and capital employed) indicators, and income diversification were taken from the unconsolidated financial statements of banks in the most recent bank scope database, the Refinitiv database of banks in various countries in SSA, and Ghana Association of Bankers covering the period 2000–2020. We controlled for bank-specific variables obtained from bank focus and Refinitiv workplace. Country-specific data on macroeconomic variables were also obtained from the World Development Indicators (WDI) database (2022). We require each bank to have at least three consecutive yearly observations to avoid short-panel bias. This selection criterion reduces the sample to 80 banks and 1680 observations in 21 SSA countries. These selections enabled us to reduce the possibility of introducing aggregation bias into the results.
3.2. Variables selection, description, and measurement
The study included one dependent variable (ROA), one independent variable (income diversification), one moderating variable (intellectual capital and its components), and six control variables. Table presents the measurements as well as the sources of data for all variables used in the study. The variables were selected using two basic criteria in terms of their popularity in income diversification and financial performance literature (Amidu & Wolfe, Citation2013; Carl & Duho, Citation2020; Cuong et al., Citation2020; Gafrej & Boujelbéne, Citation2021; Githaiga, Citation2020a; Hunjra et al., Citation2020; Luu et al., Citation2020; Nisar et al., Citation2018), intellectual capital and financial performance literature (Ardiansari et al., Citation2021; Le et al., Citation2020; Tariq et al., Citation2021; Vo & Tran, Citation2021; Yaseen & Al-Amarneh, 2021), and the availability of financial data provided by bank scope database, refinitiv database, and WDI.
Table 1. Summary of variables description and measurement
3.2.1. Dependent variable
3.2.1.1. Financial performance
Financial performance was measured by return on assets (ROA) as used in previous studies (Addai et al., Citation2022; Berger et al., Citation2010; Boamah et al., Citation2022; Duho et al., Citation2019; Githaiga, Citation2020b). It demonstrates the profits generated and how effectively the bank’s assets are managed to generate revenues (Dietrich & Wanzenried, Citation2011). It is the most common measure of bank profitability in literature (Pasiouras & Kosmidou, Citation2007). A higher return on assets shows better utilization of banks’ assets to generate profits, while a lower ROA indicates inefficient use of assets.
3.2.2. Independent variables
3.2.2.1. Income diversification
The study employed the diversification indices Laeven and Levine (Citation2007) proposed to measure income diversification. We used the Herfindahl Index (HHI) measures of income diversification as used in previous studies ((Duho et al., Citation2019; Githaiga, Citation2020a, Citation2022; Mercieca et al., Citation2007; Uddin et al., Citation2021). Therefore, the study measures income diversification as follows:
The following diversification scale was adopted in this study to judge the values of the Herfindahl Index (HHI), as shown in Table below: As the HHI rises, the bank becomes more concentrated and less diversified, HHI varies between 0 and 1.0 (Mercieca et al., Citation2007; Stiroh & Rumble, Citation2006).
Table 2. Diversification scale
3.2.3. Moderating variable
3.2.3.1. Intellectual capital
The study relied on the VAIC method proposed by Pulic (Pulic, Citation1998, Citation2000, Citation2004, Citation2008) to compute the IC performance of banks in Sub-Saharan Africa as used in literature (Duho & Onumah, Citation2021; Hejazi et al., Citation2016; Hoang et al., Citation2020; Mondal & Ghosh, Citation2012; Onumah & Duho, Citation2015; Smriti & Das, Citation2018; Weqar et al., Citation2021). This score measures the bank’s efficiency in deriving value from its stakeholders. It is a measure of the knowledge base of the banks and can drive value, competitive advantage, and transient advantage. The VAICTM method measures a company’s value-creation performance and postulates that IC is the sum of HCE, SCE and CEE. The three components can be developed from the value added by the banks.
VAICTM is the intellectual capital coefficient, HCE is human capital efficiency, SCE is structural capital efficiency, and CEE is capital employed efficiency. First, we must determine how competent a bank is in Value Added (VA), calculated as the difference between output and input: OUT—IN = VA. Output (OUT), revenue, represents the overall income from all the products and services sold on the market. Input (IN) contains all the expenses of everything that came into the company (Pulic, Citation2000, Citation2008). The VA is therefore obtained by subtracting operating expenses from total revenue (Pulic, Citation2000). The formulae for the computation of VA have been used in previous empirical studies (Tran & Vo, Citation2018; Tran et al., Citation2020; Ulum et al., Citation2014) and supported by recent theoretical papers (Marzo, Citation2022). A high coefficient indicates higher value creation using the firm’s resources, including IC. However, according to Pulic (Citation2008), value-added can be calculated from a company’s account as VA = P + C + D + A. Where P = operating profit, C = employee costs, D = depreciation, and A = amortization.
3.2.3.2. Human capital efficiency
Human capital efficiency (HCE) measures the efficiency of human capital resources (Pulic, Citation1998, Citation2004). Human capital can be defined as the set of capabilities, skills or abilities, expertise, and experiences or knowledge of bank employees who leave a bank when the employees leave. HCE is calculated by dividing the VA by the total employee costs or payroll expenditure (staff salaries, pensions, insurance, and related expenses). HCE: , But HC = Total salaries and wages (personnel expenses). Where VA is the value-added described in 1, and HC is the wages and salaries constituting total remuneration. VA is defined as the value added to the banks.
3.2.3.3. Structural capital efficiency
Structural capital efficiency (SCE) indicates structural capital (innovation, process, and customer). Structural capital consists of organizational processes, procedures, policies, systems and control, organizational infrastructure, production techniques, supply chains, intellectual properties, information systems and databases, or technological infrastructure at employees’ disposal (Pulic, Citation1998, Citation2004). Unlike human capital, structural capital is owned by the organization and does not leave when employees do. SCE is calculated by dividing the total expenses on structural capital by the firm’s VA. The equation specified below for SCE: SCE = , But SC = VA—HC
3.2.3.4. Capital employed efficiency
Capital employed efficiency (CEE) shows the value created for every monetary unit invested in financial or physical capital. Physical capital efficiency is the physical capital total funds shareholders invest (Pulic, Citation1998, Citation2004). CEE is obtained by dividing its VA by the book value of the net assets. The equation for CEE is computed as follows. CEE = , But CE = Book value of the net asset (Total Assets—Depreciation). Essentially, the value formation efficiency is the sum of all value-making efficiencies.
3.2.4. Control variables
The study incorporated six control variables in line with extant literature into the estimation model. The study thus controls for bank-specific characteristics and macroeconomic environment characteristics that affect banks’ profitability. The bank-specific variables included in the study are bank size (Duho & Onumah, Citation2019), liquidity (Huynh et al., Citation2021), and bank capitalization (Githaiga, Citation2020a), whereas the macroeconomic variables include inflation, GDP growth and interest rate (Alhassan & Asare, Citation2016; Duho & Onumah, Citation2021). The study included a set of bank-level characteristics that previous literature suggests related to bank performance (Buyuran, Citation2020; Guerry & Wallmeier, Citation2017; Liang et al., Citation2020).
3.2.4.1. Gross domestic product (GDP) growth
The study used GDP growth (GDPG), proxied by the annual GDP growth rates used in previous studies to influence bank profitability (Addai et al., Citation2022; Ammar & Boughrara, Citation2019; Boamah et al., Citation2022; Ljubownikow & Ang, Citation2020). GDP growth controls general economic development, macroeconomic stability, and institutional frameworks that are likely to affect bank performance in a country. A weak macroeconomic environment will deter foreign investments, reverse capital flows, and discourage financial innovation. GDP growth is measured as the annual rate of GDP growth.
3.2.4.2. Inflation
Staikouras (Citation2003) and Ibrahim and Alagidede (Citation2017) indicated that inflation (INF) does not have a one-way effect on bank profitability; it could directly or indirectly influence banks’ profitability. The impact of INF on bank returns could be either predicted or unpredicted. If predicted, the interest rates are adjusted appropriately, increasing revenues faster than costs and positively affecting profitability. Nevertheless, the banks may be relaxed in changing their interest rates if INF is unpredicted, resulting in bank operational costs increasing faster than revenues and negatively affecting banks’ profitability. This has been used to measure macroeconomic indicators in literature (Addai et al., Citation2022; Uddin et al., Citation2021).
3.2.4.3. Interest rate
According to the literature, the real interest rate (RIR) affects banks’ profitability (Boadi, Citation2018b). Avkiran (Citation2009) notes that interest rates negatively affect commercial banks’ profitability.
3.2.4.4. Bank size
The natural logarithm of banks’ total assets is used as a proxy for bank size as used in literature (Addai et al., Citation2022; Antwi et al., Citation2023; Duho & Onumah, Citation2019; Githaiga, Citation2022; Uddin et al., Citation2021). Larger banks can expand operations with several customers and a geographical presence, and a more significant customer base may imply more capital available for further investments and growth. Larger banks may have better diversification opportunities and, as a result, less income volatility from entering new markets (Demsetz et al., Citation2011).
3.2.4.5. Liquidity
Furthermore, the study considered bank liquidity (LIQ) and measured it by the ratio of liquid assets to deposits and short-term funding in line with the literature (Adesina, Citation2021; Ammar & Boughrara, Citation2019; Chiaramonte & Casu, Citation2017; Huynh et al., Citation2021). Since too much liquidity comes at the cost of less bank intermediation (Bertay et al., Citation2015), the study expects LIQ to have a negative relationship with bank profitability.
3.2.4.6. Bank capitalization
The capitalization ratio (CAP) is the percentage of a bank’s capital to its risk-weighted assets. This can be computed as the equity ratio to the bank’s total assets, as used in literature (Githaiga, Citation2020a; Guerry & Wallmeier, Citation2017). An increase in bank capital reduces the expected costs of financial distress and bankruptcy, resulting in a lower cost of capital (Frigerio & Vandone, Citation2018).
3.3. Estimation technique justifications and model specification
3.3.1. Justification of the system generalized method of moments
The study employs the two-step generalised method of moments (SGMM) estimator in estimating the hypothesised relationships. The SGMM overcomes the problems associated with using the difference GMM, which is biased because it uses lagged levels of explanatory variables as instruments. Further, the SGMM adds a level equation to the difference equation to deal with the issue of weak tools (Roodman, , Citation2009a). The two-step SGMM estimator, due to its loftier advantage as an estimation technique, has been increasingly used. Wintoki et al. (Citation2012) agree that the GMM estimator can effectively deal with the problem of unobserved heterogeneity. The System GMM is robust to missing data, and adding other time-invariant, institutional, and regulatory variables is possible. The study employs the Sargan/Hansen test of overidentification restrictions and the Arellano and Bond test for second-order serial correlation in the error term. Further, the two-step SGMM approach treats PERF as a dynamic process where the previous PERF influences the current PERF. However, the second order must not be serially correlated because that could be a sign of a misspecification of the model.
3.3.2. Model specification
The current study adapts the regression model used by earlier studies (Alhassan & Asare, Citation2016; Antwi et al., Citation2023; Chen et al., Citation2018; Duho & Onumah, Citation2019; Uddin et al., Citation2021).
Where PROFi,j,t represents the profitability of banks i in country j over the period t and is proxied by the ROA proxy for profitability, PROFijt-1 represents the one-period lagged profitability of banks, and VAICijt represents the value-added intellectual capital coefficient, HCEijt represents human capital efficiency, SCEijt represents structural capital efficiency, and CEEijt represents capital employed efficiency. BANKi,j,t represents a vector of three (3) bank-specific variables in bank i, in-country j at time t, which include bank growth, capitalization ratio, and size of the bank. MACROi stands for a vector of three (3) macroeconomic factors (inflation, GDP and interest rate) in a country i at time t. is the regression coefficient of IDIV,
is the regression coefficient of VAIC, is the regression coefficient of HCE,
is the regression coefficient of SCE, and is the regression coefficient for CEE. Ø is the regression coefficient of the control variable (bank-specific variables), ð is the regression coefficient of the control variable (macroeconomic variables), and Ԑ is the error term. The t stands for the period 2000 through 2020, i stands for the SSA countries used in the study, and j stands for SSA banks used in the study
3.3.3. Moderation effect model specification
To investigate the strength of the relationship between income diversification and profitability of banks in the presence of intellectual capital, the moderation model was estimated according to literature (Ofoeda et al., 2022).
The study used IC and its components, VAIC, HCE, SCE, and CEE, to moderate the relationship between income diversification and profitability of banks in SSA in separate models.
3.3.4. Estimating the total effect of IDIV on PROF
Further, in models (5), (6), (7), and (8), the interaction term between IDIV and the components of IC (VAIC, HCE, SCE, and CEE) However, to obtain the total effect of the moderating role of IC on the relationship between IDIV and PROF of banks in SSA, the study takes the partial differential of profitability concerning IC (VAIC, HCE, SCE, and CEE) following Wooldridge (Citation2013) and empirical studies by Baltagi et al., Citation2009; Mohammed et al., and Ofoeda et al., Citation2022), which is given below:
3.3.5. Justification of the dynamic panel threshold regression
In this study, a dynamic panel threshold regression was also employed to determine the nonlinear or threshold effect of IC on the relationship between IDIV and the PROF of banks in SSA. The study argues that the relationship between VAIC and profitability may differ across the different levels of VAIC among banks in SSA. The study hypothesizes that IC may moderate a threshold relationship between diversification and bank profitability. This approach extends the cross-sectional threshold model of Caner and Hansen (Citation2004) and the dynamic panel threshold estimation approach (Kremer et al., Citation2013). Seo et al. (Citation2019) address endogeneity with the regressors and threshold variable, accounting for the lagged explained variables. Caner and Hansen’s (Citation2004) model deals with endogenous regressors for cross-sectional data. Kremer and Kremer et al. (Citation2013) addressed endogeneity for dynamic panel data regressors. The study investigated the effect of income diversification on the financial performance of banks at different levels or intervals of intellectual capital. It determined whether intellectual capital moderates a threshold relationship between income diversity and profitability in SSA.
Consequently, the impact of IDIV on banks’ profitability largely depends on the sign and magnitude of the coefficients of the other variables and the extent of effectiveness of the VAIC of a bank. Also, the dynamic panel threshold regression model can be described as follows.
Where subscripts I, j and t refer to bank, country, and time, respectively. PROFi,j,t represents the financial performance of banks in SSA, while IDIVjit denotes income diversification. ICjit (VAIC, HCE, SCE, CEE) denotes the intellectual capital of banks in SSA, whereas λ,j represents the country-specific fixed effects, and µjit is a zero mean, finite variance, i.i.d. disturbance. The study denotes the control variables hypothesized to affect profitability by a vector Xjit. ICjit is the regime-switching or threshold variable used to split the data into two sample groups, while γ is the threshold value. Furthermore, β1 and θ1 are the coefficients of IDIV and VAIC below the threshold value γ, whereas β2 and θ2 are the coefficients of IDIV and VAIC above the threshold value γ. Seo et al. (Citation2019) and Seo and Shin (Citation2016) proposed the first difference transformation that relaxes the exogeneity assumption of regressors and the threshold variable. The bootstrap method allows for constructing a confidence interval and assessing the statistical significance of the identified thresholds. The study uses 2000 bootstrap replications, a 15% trimming percentage, and 100 grid numbers to test the existence of thresholds.
3.4. Estimation diagnostics
We tested for endogeneity using the Durbin-Wu-Hausman (DWH) test, as Davidson et al. (Citation1995) suggested. The endogeneity test is performed to verify whether the variables used are endogenous. The results indicate a small p-value below the threshold of 5%, suggesting that the regression suffers from endogeneity (Table ). Therefore, OLS regression is inconsistent, and GMM was more suitable for the analysis (Roodman, Citation2009a). The authors also tested for heteroscedasticity, as indicated in Table A2. The findings showed that heteroscedasticity suggests that the GMM method is preferable to instrumental variables (Baum et al., Citation2003). Table also indicates the presence of autocorrelation in the data because the p-value is less than 0.05. According to Rootman (2009b), GMM estimation gives robust outcomes even in the presence of heteroscedasticity, autocorrelation, and endogeneity. The system GMM estimator also addresses the unit root property problem and provides more accurate findings (Saif-Alyousfi, Citation2020). The threshold regression with instrumental variables modelling approach proposed by Caner and Hansen (Citation2004) and the dynamic panel threshold approach proposed by Kremer et al., Citation2013; Seo et al., Citation2019) are often employed to deal with the problem of endogeneity in panel threshold estimations. However, due to the presence of heteroscedasticity, autocorrelation, and endogeneity in our data, we proceed with the System GMM and Seo et al. (Citation2019) threshold regression estimation approach.
4. Results and discussions
4.1. Descriptive analysis
Table reports the summary statistics and dataset structure. ROA, which measured the ratio of net profits to total assets, has a mean value of 2.448, ranges between a maximum of 34.109 and a minimum of −12.113, has a standard deviation of 2.758, and is positively skewed at 4.497. This suggests that banks in SSA markets generated a 2.448 percent return on assets between 2000 and 2020. Intriguingly, the result did not show any wide deviation for the variables used in the study.
Table 3. Descriptive statistics
One of the key components of the analysis is IDIV. An average IDIV measure had a mean of 0.635 and a standard deviation of 1.09. This indicates that the banking industry in SSA can attain only 0.635 percent of its potential non-interest revenue. The study revealed that the average mean of IDIV in this current study is higher than what was reported by Addai et al. (Citation2022) within the period 2011–2018 and Boadi (Citation2018) within the period 2011–2013, with their average mean of 0.503 and 0.4, respectively. An average mean of 0.635 indicated that banks in SSA have diversified into various non-income-generating sources. It can be realized that the overall average VAIC of the banks is 6.863 and ranges from 0.000 to 79.365, which is higher than what was reported by Alhassan and Asare (Citation2016) and Duho and Onumah (Citation2019), who reported (4.31) in Africa. This implies that the average VAIC has increased (from 4.31 in 2016 to 6.863 in 2020) during the study period. A higher value for VAIC depicts a greater efficiency of IC in creating values for a firm (Pulic, Citation1998, Citation2000, Citation2008). The average VAIC of the banking sector in SSA in this study is less than the values reported by Gho (Citation2005) for Malaysian banks (7.11). Akkas and Asutay (Citation2022a) for Islamic and conventional banks in the Gulf Cooperation Council (GCC) countries (8.987), but greater than Vo and Tran's (Citation2021) banking sector in Vietnam (3.053). Abeysekera (Citation2007) attributes these dissimilarities in value and practise to economic, social, and political factors. Consequently, the value-generation efficiency of SSA banks relative to banks on other continents is relatively low on average. Even though the VAIC of banks has improved, as reported in the descriptive statistics, the study suggests that banks’ VAIC investments are still highly inefficient in SSA compared to the VAIC of banks in developed economies. This may explain SSA’s relatively low degree of economic growth compared to other continents. The other components of intellectual capital (IC) of banks in SSA, HCE, SCE, and CEE had a mean of 4.744, 0.900, and 1.223, with a standard deviation of 5.834, 2.261, and 5.660, respectively. HCE has the highest mean. Human capital is mostly seen as the key resource that ultimately drives all the other constituents of IC (Alhassan & Asare, Citation2016). This finding is consistent with the results of most studies undertaken in the banking sector in specific countries and continents Ozkan et al. (Citation2017) in Turkey; Sardo and Serrasqueiro (Citation2017) in Europe (Asare et al., Citation2017); and Alhassan and Asare (Citation2016) in Ghana, which also finds that HCE dominates the IC of firms
4.2. Pairwise correlation analysis
Table further reports the correlation matrix, which analyses the correlation among the variables used in the study. Statistically, multicollinearity is present when correlation coefficients are above 0.9 (Saunders et al., Citation2009), 0.8 (Garson, Citation2014; Gujarati, Citation2012), and 0.7 (Kennedy, Citation2008; Sekaran & Bougie, Citation2011). In keeping with Garson (Citation2014) and Gujarati (Citation2012) that multicollinearity exists when the correlation coefficients between any two (2) variables are greater than 0.8. The results presented show that all the variables in the regressions do not result in any multicollinearity, which could bias the models’ coefficients.
Table 4. Pairwise correlations
4.3. The effect of income diversification on bank performance
The study presents the GMM estimation results in Table , Column (1), which were used to test hypothesis H1, whether there is no significant relationship between income diversification and profitability of banks in SSA. The Sargan test indicates the absence of over-identifying restrictions. The Arellano-Bond (AB) test AR (2) shows no evidence of second-order autocorrelation. The Hansen value for ROA was 0.272. The magnitude and significance levels of the reported lagged dependent variable indicated that SSA banks’ profitability persists over time. The estimated GMM models in Table suggest that the ROA (profitability) lag is positive and statistically significant at 5%. This means that the banks’ previous level of profitability is the main determinant of their current profitability in SSA. The result is consistent with earlier studies like Boadi (Citation2018), Addai et al. (Citation2022), and Githaiga (Citation2020b).
Table 5. Estimation of two-step system GMM regression results
The study shows that income diversification negatively impacts banks’ profitability in SSA at 1% significant level. This result implies that the more banks in SSA diversify, the less profitable they become. This also means that a one-unit increase in income diversification will lead to a 0.516 decrease in banks’ performance, implying that diversified banks in SSA are less profitable. The study did not agree with the Portfolio Theory (Markowitz, Citation1991), which says that investors need to diversify to optimize the expected return of investments. According to portfolio theory, diversified banks benefit from economies of scope that improve performance and reduce risk; however, the study findings are inconsistent with this theoretical assumption. The study did not agree with the market power theory, the financial intermediation theory, or the delegated monitoring theory. The result is also inconsistent with the conglomeration hypothesis, which argues that owning and operating a broad range of businesses can add value by exploiting economies of scope by sharing inputs in joint production (Teece, Citation1980). Besides, this result invalidates the empirical works of (Amidu & Wolfe, Citation2013; Gafrej & Boujelbéne, Citation2021; Githaiga, Citation2020a; Hunjra et al., Citation2020; Luu et al., Citation2020; Nisar et al., Citation2018; Olarewaju et al., Citation2018; Sharma & Anand, Citation2018; Sharma & Anand, Citation2018; Sharma & Anand, Citation2020; These findings are also contrary to (Syahyunan Muda et al., Citation2017), who revealed that income diversification has a positive but non-significant effect on bank stability. In a corresponding study, Olarewaju et al. (Citation2018) revealed that the diversification of operational activities in SSA commercial banks directly affects their financial performances. However, the results support the proponents of the strategic focus hypothesis, which argues that firms can maximize value by focusing on their core businesses and competencies. The strategic-focus hypothesis opines that high earnings volatility, agency costs, high monitoring costs, and difficulty in monitoring are associated with diversification (Elyasiani & Wang, Citation2012; Laeven & Levine, Citation2007; Stiroh, Citation2004). This is because as banks in SSA diversified, it negatively affected their performance. The result also finds support in the works of Boadi (Citation2018), Stiroh and Rumble (Citation2006), Stiroh (Citation2004), Elyasiani and Wang (Citation2012).
The negative relationship between income diversification and profitability (ROA) in the SSA banking sector is surprising, considering the widespread belief that diversification reduces risk and financial instability. However, the finding aligns with Chen et al. (Citation2018) for conventional banks. Deyoung and Roland (Citation2001), Stiroh and Rumble (Citation2006), and Demirgüç-Kunt and Huizinga (Citation2010) also observe a negative relationship between bank diversification and financial performance.
In general, the results for income diversification are consistent with the view that agency problems arising from financial conglomerates increase in severity when the level of diversification is higher, such that the gains from the accompanied economies of scope are not sufficient to defray the costs brought about by the diseconomies and increased risks associated with intensified agency problems (Deyoung & Roland, Citation2001; Laeven & Levine, Citation2007). Thus, this study’s findings suggest that bank managers and employees in the SSA banking sector may lack the requisite specialized skills and experience to carry out non-lending activities, which lowers labour productivity. Again, internal information asymmetries increase with the degree of diversification. According to the theory of bounded rationality, internal information asymmetry limits workers’ ability to synthesize heterogeneous information from diverse business lines, ultimately lowering efficiency. Also, increased business activities lead to information overload, disallowing employees from taking advantage of learning opportunities that may arise from income diversification. Bank managers in SSA may allocate too much of their managerial capital to integrate non-lending activities with lending activities, leading to an inefficient allocation of human capital. This result is consistent with the assertion that income diversification makes bank managers operate outside their areas of expertise (Klein & Saidenberg, Citation2005), invest too much in lines of business with poor investment opportunities (Stulz, Citation1990), and therefore reduce their profits (Berger et al., Citation2010).
Regarding the control variables, the study revealed that banks’ capitalization ratio has a significant positive relationship with banks’ profitability in SSA at a 5% significance level. Again, the interest rate negatively impacts ROA at a 5% significant level. The GDP growth rate also shows a significant positive impact on performance in Column 1. This suggests that a bank’s profitability can influence the gross domestic product (GDP) by affecting financial performance.
4.4. The effect of intellectual capital on banks’ performance
The study also estimated the effect of IC on banks’ profitability in SSA in Table , column 2. Column 3, Column 4, and Column 5. The Hansen test was 0.281, 0.225, 0.329, and 0.357 for columns (2), (3), (4), and (5), respectively. This paper uses the VAIC method to measure intellectual capital performance (Pulic, Citation1998). The components of VAIC (Column 2) are HCE (Column 3), SCE (Column 4), and CEE (Column 5). The Arellano-Bond (AB) test AR (2) shows no evidence of second-order autocorrelation. The study also revealed that VAIC has a positive (0.034) and significant relationship with banks’ profitability in SAA in column (2) at a 1% significance level. This implies that the VAIC of banks in SSA positively contributes to their performance. The study findings are in line with Alhassan and Asare (Citation2016), Duho and Onumah (Citation2019), Acuña-Opazo and González (Citation2021); Al-Musali and Ku Ismail (Citation2016); Asare et al. (Citation2017); Meles et al. (Citation2016); Sgrò et al. (Citation2020); Shahzad et al. (Citation2021); Vidyarthi (Citation2019), who also found that VAIC positively affects performance. The result also supported the Resource-Based View Theory, which posits that superior performance stems from firm resources that create a competitive advantage for firms to enhance their performance. The findings also support the theoretical propositions of the RBV that the banks’ competitive advantage and performance are determined by their stock of intellectual capital and leveraging on income diversification. This confirms Adesina (Citation2019) findings that banks’ intellectual capital enhances banks’ performance.
More specifically, among VAIC components, HCE (Column 3) and SCE (Column 4) are the only components with a significant positive relationship with banks’ profitability in SSA. At a 1% level of significance and a 99% confidence level, HCE (0.138) and SCE (0.425) have a meaningful positive relationship with SSA banks’ performance. The findings reflect the resource-based view theory that knowledge resources are strategically crucial to a firm since they are the sole drivers of sustained superior performance and competitiveness. This indicates that issues of human capital like the number of employees, value-added per employee, years of service, the median age of employees, revenue generation per employee, employee competency index, productivity per employee, pre-tax profit per employee, turnover rate, assets per employee, employees by race, gender, and religion, disability, and many others, can be exploited to improve the profitability of banks. Therefore, this supports the resource-based view theory. However, the findings do not agree with Alipour (Citation2012), whose results revealed that SCE negatively influences banks’ performance. Still, the study agrees with Adesina (Citation2019), who showed that HCE positively impacts banks’ performance. However, Smriti and Das (Citation2018), Firer and Mitchell Williams (Citation2003), and Kor and Mahoney (Citation2005) found that human capital had a negative effect on organisational performance. However, in column 5, CEE negatively affects banks’ profitability in SSA. There is thus a profound suggestion that the efficiency of capital employed by banks, when improved, makes the bank less stable. Findings agree with Adesina (Citation2019), who revealed that CEE negatively affects banks’ performance. Therefore, it can be concluded that SSA banks’ capital base (resources) contributes to their poor performance, which is consistent with the resource-based view theory. Still, Kaplan and Norton's (Citation2004) empirical study also revealed that the capital base of most banks in SSA is not significant and robust and could account for these negative relationships between CEE and banks’ profitability in SSA.
Analysis of results for the control variables generally suggests that BSIZE has a positive and significant association with the profitability of banks at 1% significant level in columns column (2), column (3), column (4), and column (5) in Table above. As already intimated, there is evidence to support that banks’ capitalization ratio positively affects banks’ profitability in SSA at 1% significant level across column (2), column (3), column (4), and column (5) in Table . This means that the banks’ capitalization ratio positively influences the growth of banks in SSA. The results align with Meng et al. (Citation2018), who explained their findings from the perspective of the economy of scope.
In conclusion, the results on value-added intellectual capital align with the resource-based view that firms require resources to aid in implementing strategies, ultimately determining their efficiency and effectiveness and influencing their performance. This is in line with the literature that suggests that banks that engage in activities to create innovative products and services in the forms of effective human capital development, improvement of the banks’ structures, and adequate capital may lead to improvement in the performance of those banks (Sgrò et al., Citation2020; Muhammad et al., Citation2021).
4.5. The interactions of income diversification, intellectual capital, and bank performance
One key aim of this study was to examine how intellectual capital interacts with income diversification in determining banks’ profitability in SSA. Table , column 6, column 7, column 8, and Column 9 present the results of Model (5), (6), (7) and (8), whether IC moderated the relationship between income diversification and profitability of banks in SSA. The results from the two-step GMM of the interaction between income diversification and VAIC on the financial performance of banks in SSA are presented in Table . Although the interaction effect of overall VAIC and the financial performance of banks in Sub-Saharan Africa was positive and statistically significant, one of the results from the interaction effect of income diversification and the disaggregated component of VAIC shows a negative influence on the financial performance of banks in SSA. Table further indicates the interaction between the income diversification of VAIC and the disaggregated components of VAIC. From Table above, the interaction effect between (VAIC*IDIV) shows a positive and significant impact. The partial result shows the effect of IDIV on PROF when VAIC is zero. Income diversification remains positive but statistically insignificant after the introduction of the interaction, but the interaction effect is positive and significant. This suggests that intellectual capital plays an important role, as far as income diversification is concerned, in influencing banks’ profitability in SSA positively.
Based on the results, the significance of the interaction terms was tested by following Wooldridge (Citation2016) and first testing the partial effect of IDIV in the presence of VAIC, followed by the disaggregated components of VAIC. The total impact of IDIV in the presence of VAIC, HCE, SCE, and CEE gives the estimated coefficients of (dy/dx = 0.257), (dy/dx = 0.515), (dy/dx = 0.073), and (dy/dx = −0.103), respectively. Empirically, this process has been used in the Ofoeda et al. (Citation2022) study.
The total effect of VAIC (*jit) is positive (0.257), statistically significant at 1%, and has a greater effect than the effect without considering VAIC. The implication is that the gain from bank income diversification will be significantly higher for SSA banks when bank managers and employees in SSA have the requisite specialized skills and experience to carry out banks’ diversified non-lending activities, which improves performance according to the RBV. The predictable, significant positive coefficient of 0.257 can only occur if banks in SSA have more sophisticated technology, managerial skills, and the expertise required to be more competitive in non-traditional banking activities (Meslier et al., Citation2014; Sanya & Wolfe, Citation2011). This current finding can be explained by the fact that banks need sophisticated human resources and skills to make efficient business decisions in their various areas of diversification (Ahamed et al., Citation2017; Nguyen, Citation2018).
The total effect ( +
*
jit) of IDIV and HCE on banks’ profitability in SSA gives a coefficient of 0.515. This implies that the total effect of the interaction of IDIV and HCE on banks’ profitability is positive, with a coefficient of 0.515, which is statistically significant. It is imperative to note that the interaction of human capital and income diversification is greater than the partial effect when human capital efficiency is ignored. This suggests that the interaction term has a higher effect on the performance of banks in SSA. As shown in the descriptive statistics in Table , the HCE of banks is much higher across the sampled banks than SCE and CEE, even though the value is not encouraging. In line with the Resource-Based View Theory, for competitiveness and long-term survival, firms should concentrate on organizational learning to nurture combinative capabilities that facilitate exploiting intellectual capital resources and synergies (Wu et al., Citation2007). This argument emerges superior financial performance results from the best match of banks’ resources, robust strategies, and market opportunities.
The study also revealed that the coefficient of the total effect ( +
*
jit) is positive (0.073) but statistically insignificant. However, it is crucial to recognize that the interaction between SCE and income diversification is less than the total effect shown when SCE was neglected. This suggests that the interaction term has a relatively small effect on the financial performance of banks in SSA. The descriptive statistics show a minimal mean value for SCE for the 80 sampled banks in the selected 21 SSA countries. This, therefore, means that banks in SSA must improve upon their non-human stock of knowledge, which includes culture, systems, procedures, information technology, trademarks, patents, and plans, which databases can represent, software, hardware, and organizational structures (Al-Zoubi, Citation2013). According to Ahangar (Citation2011), SCE is a supportive structure for HCE because a firm with a robust SCE will have support systems and a culture for learning and development.
The total effect of income diversification on banks’ profitability is conditioned on CEE ( + *jit) surprisingly indicated a negative impact with a coefficient of −0.103. The output suggests that the coefficient is statistically significant. Considering the means used by banks, the result for the total effect shows a negative impact of −0.103 and is statistically significant. It is worth noting again that the interaction term is less than the total effect, suggesting that the CEE of banks in SSA reduces the financial performance of banks for the 80 banks sampled from the 21 SSA countries when interacting with income diversification. Thus, the interaction has a decreasing effect on the financial performance of banks. There is widespread speculation that the capital employed by banks in SSA is low, which is also evident from the descriptive statistics. Not surprisingly, Kaplan and Norton (Citation2004) also found that the capital structure of most African banks is not big and robust, which could account for the poor positive associations between CEE and banks’ performance in SSA found in this study.
4.6. Panel threshold regression analysis
This study aimed to determine the nonlinear effect of IC on the relationship between income diversification and the performance of banks in SSA. The study employs Seo et al. (Citation2019) dynamic panel threshold estimation to test whether the hypothesised relationships are monotonic. Thus, the study hypothesised that IC has no threshold effect (i.e., a nonlinear relationship) between income diversification and banks’ profitability in SSA. Accordingly, the study tests the null hypothesis of linearity, where θ1 = θ2, against the alternative hypothesis of a threshold model, that is, θ1 ≠ θ2. If the null hypothesis holds, a threshold does not exist; otherwise, it implies that the threshold effect exists. The study used 2000 bootstrap replications, a 15% trimming percentage, and 100 grid numbers to test whether intellectual capital has no threshold effect (i.e., a nonlinear relationship) between income diversification and banks’ profitability in Sub-Saharan Africa. The study tested the threshold effect for VAIC and all its components. In Table , the study presents the results of the existence of the threshold test. The threshold test results presented in Table suggest that value-added intellectual capital (VAIC) and all the other components of VAIC have a nonlinear relationship between income diversification and the profitability of banks in Sub-Saharan Africa at a significant level of 1%. Therefore, the study rejects the null hypothesis of no threshold and concludes that IC has a threshold effect on the relationship between income diversification and banks’ profitability in SSA.
Table 6. Dynamic panel threshold test of intellectual capital on the relationship between income diversification and banks’ performance
The study’s findings suggest that VAIC and all the other components of VAIC on the relationship between income diversification and profitability of banks in SSA are determined by the extent of IC of a particular bank. Hence, the study divided the sample into two groups: regime one is above the threshold value, and regime two is below the threshold value. Given that the threshold effects exist in the hypothesized relationships, the study proceeds with the dynamic panel threshold regression proposed by Seo et al. (Citation2019). The Seo et al. (Citation2019) threshold regression presents the overall or linear regression, the low-regime, and the high-regime results. The study shows the results of the dynamic panel threshold regression for VAIC and all the other components of VAIC as propounded by Pulic (Pulic, Citation1998, Citation2000), HCE, SCE, and CEE samples in Table . VAIC is in column (1), column (2), and column (3) for the low regime, high regime, and overall regime, respectively. However, the measures of HCE are in columns (4), column (5), and column (6) for the low regime, high regime, and overall regression, respectively, while SCE is in columns (7), column (8), and column (9) for the low regime, high regime, and overall regime, respectively, and finally, CEE is in column (10), column (11) and column (12) for the low regime, high regime, and overall regime, respectively. Table , column 1, presents the results of Model 13, which was used to test whether IC has no linear effect on the relationship between income diversification and profitability of banks in SSA. The authors used the VAIC that a bank has as the regime-switching trigger for column 1 in Table . The study also used the components of VAIC as the regime-switching trigger. In columns (4), column (5), and column (6), HCE was used as the regime-switching trigger; in column (7), columns (8), and column (9), SCE was used as the regime-switching trigger; and in column (10), column (11) and column (12) the authors used CEE as the regime-switching trigger.
Table 7. Dynamic panel threshold regression results of intellectual capital on the relationship between income diversification and bank performance
The study found threshold values of 4.201 for VAIC. In column 1, the study found a significant positive coefficient of 0.790 above the threshold value and a significant negative coefficient of −0.737 below the threshold in column 2. Findings imply that VAIC of banks only contributes to the profitability of banks at the high regime of VAIC. The results align with Barney’s (Citation2012) study, which indicated that an organization’s resources influence its effectiveness. According to the resource-based theory, firms require resources to implement strategies that ultimately determine their efficiency and effectiveness level (Barney, Citation2012). The findings also imply that the impact of VAIC on the performance of banks in SSA is stronger in the high regime of VAIC above a threshold value of 4.201. The positive effects of VAIC on banks’ profitability in SSA are only set above a certain threshold of bank VAIC. It can also be explained that VAIC has a non-linear significant positive impact on the relationship between income diversification and profitability of banks in SSA above a threshold value of 4.201. The positive and significant effect of VAIC on bank performance at the high regime of VAIC can be explained by the superior financial performance of SSA banks, which is a product of the best match of firm resources, strategies, and market opportunities. Specifically, SSA banks should continually invest in VAIC and innovation to renew and reconfigure congruent competencies with the external environment. The resource-based perspective hypothesis, therefore, portends that VAIC is crucial in building competitive advantages and, consequently, might result in variations in performance among enterprises, nations, and regions. Based on the resource-based view theory, it can also be argued that diversified banks with high-level IC will tend to focus on their non-traditional banking businesses and provide them with high quality, agility, and speed to improve their financial performance. Studies have also revealed that firms can leverage their knowledge resources through diversification to enhance performance (Chung et al., Citation2019; Fisch & Schmeisser, Citation2020).
As part of the components of VAIC, the study also used HCE as the regime-switching trigger for columns (4), column (5), and column (6). The study also found threshold values of 4.431 for HCE for banks in SSA. The study found an insignificant negative coefficient of −0.013 below the threshold value of 4.431 for HCE. The study found that HCE positively affects banks’ profitability for the overall sample in column 6 with a coefficient value of 0.131 at a 1% significance level. However, the impact is only positive and statistically significant for banks above the threshold value of 4.431, with a coefficient of 0.436 in column 5, greater than the coefficient of the overall sample of 0.131. It can be argued that the positive impact of banks’ human capital on profitability above the threshold in column 5 disappears when the human capital efficiency of banks weakens below the threshold value of 4.431. This implies that banks with weak HCE in SSA may have harmful outcomes for income diversification and profitability. It can also be argued that SSA banks with experienced and knowledgeable employees can provide innovative products and services to increase profitability. Studies revealed that high financial performance emanates from matching the firm’s capabilities to market opportunities (Carnes et al., Citation2017; Feng et al., Citation2017). Addressing weak human capital efficiency in SSA banking sector requires concerted efforts from various stakeholders, including banks, regulatory authorities, educational institutions, and industry associations. Investments in training and development programs, talent acquisition strategies, and collaborations with academic institutions can help enhance the skills and capabilities of banking professionals in SSA. It can be concluded that SSA countries should invest in human capital development in the banking sector because investment in human capital is expected to promote the financial performance of banks in SSA.
As presented in Table , the results of the study show threshold values of 0.348 for SCE, which was also used as the regime-switching trigger for columns (7), column (8), and column (9). The study results found that SCE positively impacts banks’ performance for the overall sample, which is significant at 10%. However, the study reports an insignificant negative coefficient (0.012) above the threshold and a significant positive coefficient (0.268) below the threshold for SCE. The findings show that although SCE positively impacts banks’ profitability, the impact is only felt below the threshold value with a coefficient of (0.268). The results indicated that the positive effects of SCE on banks’ profitability wane and become negative above a threshold value of (0.348). This implies that banks in SSA have not been able to invest much in SCE. It can be argued that structural capital investment in banks, such as core banking systems, digital channels and platforms, risk management systems, data analytics and business intelligence, cybersecurity infrastructure and compliance and regulatory systems in SSA, is inadequate, resulting in a negative coefficient above the threshold value of SCE. However, it may also be argued that structural capital investment has become a costly and resource-intensive endeavour for banks in SSA. This implies that the costs associated with structural capital investment result in higher transaction costs for banks in SSA, rendering them highly uncompetitive.
Consequently, excessive structural capital investment may somewhat discourage the financial performance of banks in SSA due to the cost associated with investing in banks’ structural capital, such as robust IT infrastructure and good internal processes and procedures. Findings also suggest that weak investment in structural capital in the banking sector in SSA might negatively affect banks’ profitability at the high regime. According to Swart (Citation2006), structural capital is the backbone of an organization. This implies that a diversified bank with high and robust structural capital in SSA will have supportive structures and a culture for learning and development, creating value for the bank. Previous empirical studies concluded that a diversified bank coupled with poor databases, poor software and hardware, and weak organizational structures would have minimal success (Al-Zoubi, Citation2013; Bontis, Citation1996).
Finally, the study used CEE, which is also a component of VAIC, as the regime-switching trigger for column (10), column (11), and column (12) in Table . The study provides evidence of a negative impact of capital employed on banks’ profitability across all capital employed levels for the overall sample. The study shows a significant negative coefficient (−0.083) below the threshold and a significant negative coefficient (−0.058) above the threshold value. Although this study shows a negative impact of capital employed on the profitability of banks in SSA at all levels of capital employed column (10), column (11), and column (12), the negative effect is more pronounced at a higher level (−0.083) of capital employed than at the lower regime (below the threshold) value of −0.058 of capital employed. This indicated that for SSA banks to improve their financial performance through income diversification, they needed to improve their capital base.
5. Conclusion and policy recommendations
Globally, the banking business is metamorphosing from a traditional intermediation business due to competition, liberalization, technological development, globalization, and customer-centricity. Banks are exploring new ways to provide a comprehensive service to their customers with speed, agility, integrity, and a lower cost. The question of what diversification strategy to employ or how a bank’s revenue model needs to be are essential issues discussed by analysts, bankers, and decision-support staff such as management accountants. This current study analyzed the effect of income diversification and intellectual capital on banks’ profitability in SSA. The study results show that increased income diversification adversely impacted banks’ profitability in SSA. Specifically, a shift from interest-yielding income to non-interest income activities results in bank losses regarding banks’ profits. Therefore, the study concludes that focused banks in SSA are more profitable than diversified banks. The study results highlighted that focusing on non-interest income sources provides an income diversification loss to banks, particularly SSA banks. This implies that SSA banks may not have sophisticated technology and other resources required to compete in non-traditional banking activities. The importance of knowledge-based capital is gradually increasing, particularly in today’s era of knowledge-based economies. The study also revealed that IC improves bank performance in SSA. This is driven by human capital and the structural capital base of SSA banks. Even though there has been an improvement in VAIC and its components in SSA, the study concludes that the efficiency of banks’ IC investments is still relatively low in SSA.
The study also found that VAIC positively and significantly moderated the relationship between income diversification and banks’ profitability in SSA. Hence, leveraging intellectual capital through non-traditional banking activities increases income diversification’s impact on the profitability of SSA banks. The findings clearly show the role of income diversification in improving the performance of banks through intellectual capital. The results of this study suggest that the effects of income diversification on banks’ profitability may differ for banks due to the different levels of intellectual capital of banks in SSA. The study revealed that VAIC, HCE, and SCE significantly and positively impact the relationship between income diversification and bank performance in SSA below a certain threshold value. However, an adverse effect of VAIC, HCE, and SCE on banks’ performance in SSA set above a certain threshold of VAIC, HCE, and SCE that banks have attained. It can be argued that diversified banks with high levels of intellectual capital will tend to focus on their non-traditional banking businesses and provide them with high quality, agility, and speed to improve their financial performance. This study also provides empirical evidence explained by the resource-based view and signalling theories to justify the claim that banks with better IC have greater financial performance.
The findings of the study have some policy implications. First, bank managers in SSA should leverage intellectual capital to contribute most significantly toward the value creation efficiency of the banking system in SSA. This is because, in line with the Resource-Based View Theory, for competitiveness and long-term survival, bank managers should concentrate on organizational learning to nurture the combined capabilities of their human resources to ensure effective and prudent facilitation and use of intellectual capital resources for enhanced bank performance. Secondly, the study recommends that when bank managers in SSA operate outside the scope of their traditional banking activities, they should have sophisticated human resources and managerial skills to make the banking system in SSA competitive. Bank managers must, therefore, ensure the prudent and innovative use of resources to enhance the financial performance of SSA banks’ diversified portfolios. Bank managers should consequently focus on a strategy that focuses on increasing their interest income to avoid losses from non-traditional banking activities in SSA banking system. Thirdly, the study provides evidence that the impact of IC on the profitability of banks in SSA is non-linear and, therefore, threshold-specific. Consequently, policymakers in the banking sector in SSA should be aware of potential threshold effects to reap the maximum benefits from intellectual capital. Therefore, policymakers and regulators in the banking sector in SSA should develop a policy that increases the intellectual capital of banks to improve the profit of diversified non-traditional banking activities in SSA.
Lastly, limitations to this study may offer opportunities for further research. The study used only ROA as a measure of banks’ profitability. While this keeps the analysis simple, future studies may use various performance indicators in examining the impact of the various components of IC on bank performance across the globe. Future studies can extend to other countries and sectors of the economies, which might shed more light on income diversification, asset diversification, geographical diversification, and intellectual capital’s effect on financial performance. Subsequently, there is a need to undertake a cross-industry study comparing the regions in Africa or the countries or a cross-continent study comparing banks in Africa to banks on other continents.
Disclosure statement
No potential conflict of interest was reported by the author(s).
References
- Abeysekera, I. (2007). Intellectual capital reporting between a developing and developed nations Journal of Intellectual Capital, 8(2), 329–35. https://doi.org/10.1108/14691930710742871
- Abuzayed, B., Al-Fayoumi, N., & Molyneux, P. (2018). Diversification and bank stability in the GCC Journal of International Financial Markets, Institutions, and Money, 57, 17–43. https://doi.org/10.1016/j.intfin.2018.04.005
- Abuzayed, B., Al-Fayoumi, N., & Molyneux, P. (2018a). Journal of International Financial Markets, Institutions, Money Diversification, and Bank Stability in the GCC Journal of International Financial Markets, Institutions, and Money, 57, 17–43. https://doi.org/10.1016/j.intfin.2018.04.005
- Acharya, V. V., Hasan, I., & Saunders, A. (2006). Should banks be diversified? Evidence from individual bank loan portfolios The Journal of Business, 79(3), 1355–1412. https://doi.org/10.1086/500679
- Acuña-Opazo, C., & González, O. C. (2021). The impacts of intellectual capital on financial performance and the value-added of the production evidence from Chile Journal of Economics, Finance, and Administrative Science, 26(51), 127–142. https://doi.org/10.1108/JEFAS-08-2019-0178
- Addai, B., Tang, W., & Agyeman, A. S. (2022). Examining the impact of income diversification on bank performance: Are foreign banks heterogeneous? Journal of Applied Economics, 25(1), 1–21. https://doi.org/10.1080/15140326.2021.2022828
- Adesina, K. S. (2019). Bank technical, allocative, and cost efficiencies in Africa: The influence of intellectual capital The North American Journal of Economics & Finance, 48 (February), 419–433. https://doi.org/10.1016/j.najef.2019.03.009
- Adesina, K. S. (2021). How diversification affects bank performance: The role of human capital Economic Modelling, 94, 303–319. https://doi.org/10.1016/j.econmod.2020.10.016
- Ahamed, M. (2017) Asset quality, non-interest income, and bank profitability: evidence from Indian banks Economic Modelling, 63, 1–14. https://doi.org/10.1016/j.econmod.2017.01.016
- Ahangar, R. G. (2011). The relationship between intellectual capital and financial performance: An empirical investigation in an Iranian company African Journal of Business Management. https://doi.org/10.5897/AJBM10.712
- Akkas, E., & Asutay, M. (2022a). The impact of intellectual capital formation and knowledge economy on banking performance: A case study of GCC’s conventional and Islamic banks Journal of Financial Reporting and Accounting https://doi.org/10.1108/JFRA-08-2021-0251
- Akkas, E., & Asutay, M. (2022b). Intellectual capital disclosure and financial performance nexus in Islamic and conventional banks in the GCC countries International Journal of Islamic and Middle Eastern Finance and Management, 15(5), 943–966. https://doi.org/10.1108/IMEFM-01-2021-0015
- Albert, Z. Z., & Dieter, F. (2003). The intellectual capital web: A systematic linking of intellectual capital and knowledge management Journal of Intellectual Capital, 4 (1), 34–48.
- Alhassan, A. L., & Asare, N. (2016). Intellectual capital and bank productivity in emerging markets: Evidence from Ghana Management Decision, 54(3), 589–609. https://doi.org/10.1108/MD-01-2015-0025
- Alipour, M. (2012). The effect of intellectual capital on firm performance: An investigation of Iranian insurance companies Measuring Business Excellence, 16(1), 53–66. https://doi.org/10.1108/13683041211204671
- Al-Musali, M. A., & Ku Ismail, K. N. I. (2016) Cross-country comparison of intellectual capital performance and its impact on the financial performance of commercial banks in GCC countries International Journal of Islamic & Middle Eastern Finance & Management, 9(4), 512–531. https://doi.org/10.1108/IMEFM-03-2015-0029
- Al-Zoubi, M. R. (2013). The impact of intellectual capital on SWOT analysis among Jordanian banking industry “empirical study”. International Journal of Business and Social Science, 4(2), 123–137.
- Amidu, M., & Wolfe, S. (2013). Does bank competition and diversification lead to greater stability? Evidence from emerging markets Journal of Advanced Research, 3(3), 152–166 https://doi.org/10.1016/j.rdf.2013.08.002
- Amihud, Y., & Lev, B. (1981). Risk reduction as a managerial motive for conglomerate mergers The Bell Journal of Economics, 12(2), 605. https://doi.org/10.2307/3003575
- Ammar, N., & Boughrara, A. (2019). The impact of revenue diversification on bank profitability and risk: Evidence from the MENA banking industry Macroeconomics and Finance in Emerging Market Economies, 12(1), 36–70 https://doi.org/10.1080/17520843.2018.1535513
- Antwi, S., Tetteh, A. B., Armah, P., & Dankwah, E. O. (2023). Anti-money laundering measures and financial sector development: empirical evidence from Africa Cogent Economics & Finance, 11(1) https://doi.org/10.1080/23322039.2023.2209957
- Ardiansari, A., Ridloah, S., Pangestuti, I. R. D., & Indriyani, P. (2021). The influence of intellectual capital on the company’s financial performance and market value Universal Journal of Accounting and Finance https://doi.org/10.13189/UJAF.2021.090211
- Asare, N., Alhassan, A. L., Asamoah, M. E., & Ntow-Gyamfi, M. (2017). Intellectual capital and profitability in an emerging insurance market Journal of Economic and Administrative Sciences, 33(1), 2–19. https://doi.org/10.1108/jeas-06-2016-0016
- Asiedu, E. (2002). On the determinants of foreign direct investment in developing countries: Is Africa different? World Development, 30(1), 107–119. https://doi.org/10.1016/S0305-750X(01)00100-0
- Avkiran, N. K. (2009). Removing the impact of the environment with units-invariant efficient frontier analysis: An illustrative case study with intertemporal panel data Omega, 37(3), 535–544. https://doi.org/10.1016/j.omega.2007.10.002
- Baltagi, B. H., Demetriades, P. O., & Law, S. H. (2009). Financial development and openness: evidence from panel data Journal of Development Economics, 89(2), 285–296. https://doi.org/10.1016/j.jdeveco.2008.06.006
- Barney, J. (1991). Firm resources and sustained competitive advantage Journal of Management, 17(1), 99–120. https://doi.org/10.1177/014920639101700108
- Barney, J. B. (2012). Purchasing, supply chain management, and sustained competitive advantage: the relevance of resource-based theory The Journal of Supply Chain Management, 48(2), 3–6. https://doi.org/10.1111/j.1745-493X.2012.03265.x
- Baum, C. F., Schaffer, M. E., & Stillman, S. (2003). Instrumental variables and GMM: estimation and testing The Stata Journal: Promoting Communications on Statistics and Stata, 3(1), 1–31. https://doi.org/10.1177/1536867x0300300101
- Berger, A. N., Hasan, I., & Zhou, M. (2010). The effects of focus versus diversification on bank performance: Evidence from Chinese banks Journal of Banking and Finance, 34(7), 1417–1435. https://doi.org/10.1016/j.jbankfin.2010.01.010
- Bertay, A. C., Demirgüç-Kunt, A., & Huizinga, H. (2015). Bank ownership and credit over the business cycle: Is lending by state banks less procyclical? Journal of Banking and Finance, 50, 326–339. https://doi.org/10.1016/j.jbankfin.2014.03.012
- Boadi, I. (2018). Income diversification and banks’ profitability from an African market perspective: A relief for SMEs? African Entrepreneurship. https://doi.org/10.1007/978-3-319-73700-3_8
- Boamah, N. A., Boakye-Dankwa, A., & Opoku, E. (2022). Risk-taking behaviour, competition, diversification, and performance of frontier and emerging economy banks Asian Journal of Economics and Banking, 6(1), 50–68. https://doi.org/10.1108/ajeb-04-2021-0047
- Bontis, N. (1996). Management Decision Intellectual Capital: An exploratory study that develops measures and models Management Decision Journal of Intellectual Capital Planning Review, 36 (2). https://doi.org/10.1108/00251749810204142
- Bontis, N. (2001). Assessing knowledge assets: A review of the models used to measure intellectual capital International Journal of Management Reviews, 3(1), 41–60. https://doi.org/10.1111/1468-2370.00053
- Brahmana, R., Kontesa, M., & Gilbert, R. E. (2018a). Income diversification and bank performance: evidence from Malaysian banks. Economics Bulletin, 38 (2) 799–809.
- Brahmana, R., Kontesa, M., & Gilbert, R. E. (2018b). Volume 38, Issue 2, income diversification and bank performance: Evidence from Malaysian Banks Economics Bulletin, 38(2), 799–809.
- Brighi, P., & Venturelli, V. (2014). How do income diversification, firm size, and capital ratio affect performance? Evidence for bank holding companies Applied Financial Economics, 24(21), 1375–1392. https://doi.org/10.1080/09603107.2014.925064
- Buch, C., & Drages, B. G. (2018) Structural changes in banking after the crisis. In CGFS Papers (Issue 60), https://www.bis.org/publ/cgfs60.pdf
- Buyuran, B. (2020). Revenue diversification and bank performance: evidence from Turkey South-Eastern Europe Journal of Economics, 18(1), 7–18.
- Caner, M., & Hansen, B. E. (2004). Instrumental variable estimation of a threshold model Econometric Theory, 20(5) https://doi.org/10.1017/S0266466604205011
- Căpraru, B., Ihnatov, I., & Pintilie, N. L. (2020). Competition and diversification in the European banking sector Research in International Business and Finance, 51, 51. https://doi.org/10.1016/j.ribaf.2018.09.014
- Carl, K., & Duho, T. (2020). Bank diversification and performance in an emerging market August 2019. https://doi.org/10.1108/IJMF-04-2019-0137
- Carnes, C. M., Chirico, F., Hitt, M. A., Huh, D. W., & Pisano, V. (2017). Resource orchestration for innovation: structuring and bundling resources in growth- and maturity-stage firms Long Range Planning, 50(4), 472–486. https://doi.org/10.1016/j.lrp.2016.07.003
- Chen, N., Liang, H. Y., & Yu, M. T. (2018) Asset diversification and bank performance: evidence from three Asian countries with a dual banking system Pacific Basin Finance Journal, 52, 40–53. May 2019. https://doi.org/10.1016/j.pacfin.2018.02.007
- Chiaramonte, L., & Casu, B. (2017). Capital and liquidity ratios and financial distress Evidence from the European banking industry The British Accounting Review, 49(2), 138–161. https://doi.org/10.1016/j.bar.2016.04.001
- Chiorazzo, V., Milani, C., & Salvini, F. (2008). Income diversification and bank performance: evidence from Italian banks Journal of Financial Services Research, 33(3), 181–203. https://doi.org/10.1007/s10693-008-0029-4
- Chung, D., Kim, M. J., & Kang, J. (2019). Influence of alliance portfolio diversity on innovation performance: The role of internal capabilities in value creation Review of Managerial Science, 13(5), 1093–1120. https://doi.org/10.1007/s11846-018-0281-4
- Cuong, H. V., Ngoc Luu, H., Quynh Thi Nguyen, L., & Chu, V. T. (2020). Income structure, diversification strategy, and owners’ benefit in cooperative financial institutions International Journal of Managerial Finance, 16(4), 481–500. https://doi.org/10.1108/IJMF-11-2018-0346
- Davidson, J., Davidson, R., & MacKinnon, J. G. (1995). Estimation and inference in econometrics Economica, 62 (245), 133. https://doi.org/10.2307/2554780
- Demirgüç-Kunt, A., & Huizinga, H. (2010). Bank activity and funding strategies: The impact on risk and returns Journal of Financial Economics, 98(3), 626–650 https://doi.org/10.1016/j.jfineco.2010.06.004
- Demsetz, R. S., Saidenberg, M. R., & Strahan, P. E. (2011) Agency problems and risk-taking at banks. SSRN Electronic Journal. https://doi.org/10.2139/ssrn.943507
- Deyoung, R., & Roland, K. P. (2001). Product mix and earnings volatility at commercial banks: evidence from a degree of total leverage model Journal of Financial Intermediation, 10(1), 54–84. https://doi.org/10.1006/jfin.2000.0305
- Dietrich, A., & Wanzenried, G. (2011). Determinants of bank profitability before and during the crisis: Evidence from Switzerland Journal of International Financial Markets, Institutions, and Money, 21(3), 307–327 https://doi.org/10.1016/j.intfin.2010.11.002
- Doan, A. T., Lin, K. L., & Doong, S. C. (2018) What drives bank efficiency? The interaction of bank income diversification and ownership International Review of Economics and Finance, 55, 203–219. https://doi.org/10.1016/j.iref.2017.07.019
- Duho, K. C. T., Duho, D. M., & Forson, J. A. (2021). Impact of income diversification strategies on credit risk and market risk among microfinance institutions Journal of Economic and Administrative Sciences, 39(2), 523–546. ahead-of-p(ahead-of-print). https://doi.org/10.1108/jeas-09-2020-0166
- Duho, K. C. T., & Onumah, J. M. (2019). Bank diversification strategy and intellectual capital in Ghana: An empirical analysis Asian Journal of Accounting Research, 4(2), 246–259. https://doi.org/10.1108/AJAR-04-2019-0026
- Duho, K. C. T., & Onumah, J. M. (2021). Determinants of intellectual capital performance in banks: empirical insights from an emerging market Afro-Asian Journal of Finance and Accounting, 11(4), 583 https://doi.org/10.1504/AAJFA.2021.117740
- Duho, K. C. T., Onumah, J. M., & Owodo, R. A. (2019). Bank diversification and performance in an emerging market International Journal of Managerial Finance, 16(1), 120–138. https://doi.org/10.1108/IJMF-04-2019-0137
- Elsas, R., Hackethal, A., & Holzhäuser, M. (2010). The anatomy of bank diversification Journal of Banking and Finance, 34(6), 1274–1287 https://doi.org/10.1016/j.jbankfin.2009.11.024
- Elyasiani, E., & Wang, Y. (2012). Bank holding company diversification and production efficiency. Applied Financial Economics, 22(17), 1409–1428. https://doi.org/10.1080/09603107.2012.657351
- Esho, N., Kofman, P., & Sharpe, I. G. (2005). Diversification, fee income, and credit union risk Journal of Financial Services Research, 27(3), 259–281. https://doi.org/10.1007/s10693-005-1804-0
- Espinosa-Méndez, C., Araya-Castillo, L., Jara Bertín, M., & Gorigoitía, J. (2021). International diversification, ownership structure, and performance in an emerging market: evidence from Chile Economic Research-Ekonomska Istrazivanja, 34(1), 1202–123. https://doi.org/10.1080/1331677X.2020.1820359
- Fajriyanti, N., Sukoharsono, E. G., & Abid, N. (2021). Examining the effect of diversification, corporate governance, and intellectual capital on sustainability performance International Journal of Research in Business and Social Science (2147–4478), 10(2), 12–20. https://doi.org/10.20525/ijrbs.v10i2.1053
- Feng, H., Morgan, N. A., & Rego, L. L. (2017). Firm capabilities and growth: The moderating role of market conditions Journal of the Academy of Marketing Science, 45(1), 76–92. https://doi.org/10.1007/s11747-016-0472-y
- Ferreira, J. H. L., Zanini, F. A. M., & Alves, T. W. (2018) Bank revenue diversification: its impact on risk and return in Brazilian banks https://doi.org/10.1590/1808-057x201805810
- Firer, S., & Mitchell Williams, S. (2003). Intellectual capital and traditional measures of corporate performance Journal of Intellectual Capital, 4(3), 348–360 https://doi.org/10.1108/14691930310487806
- Fisch, J. H., & Schmeisser, B. (2020). Phasing the operation mode of foreign subsidiaries: reaping the benefits of multi-nationality through internal capital markets Journal of International Business Studies, 51(8), 1223–1255. https://doi.org/10.1057/s41267-020-00321-1
- Francis, B. B., Hasan, I., Küllü, A. M., & Zhou, M. (2018). Should banks diversify or focus? Know thyself: The role of abilities Economic Systems, 42(1), 106–118. https://doi.org/10.1016/j.ecosys.2017.12.001
- Frigerio, M., & Vandone, D. (2018) Bank ownership and firm-level performance: An empirical assessment of state-owned development banks https://doi.org/10.1007/978-3-319-90294-4_9
- Gafrej, O., & Boujelbéne, M. (2021). The impact of performance, liquidity, and credit risks on banking diversification under financial stress International Journal of Islamic and Middle Eastern Finance and Management, 15(1), 66–82. https://doi.org/10.1108/IMEFM-09-2020-0488
- Garson, G. D. (2014). Fundamentals of hierarchical linear and multilevel modelling Hierarchical Linear Modelling: A Guide and Applications https://doi.org/10.4135/9781483384450.n1
- Gho, P. C. (2005). Intellectual capital performance of commercial banks in Malaysia. Journal of Intellectual Capital. https://doi.org/10.1108/14691930510611120
- Ghosh, S. K., & Maji, S. G. (2015). Empirical validity of the value-added intellectual coefficient model in the Indian knowledge-based sector Global Business Review, 16(6), 947–962. https://doi.org/10.1177/0972150915597597
- Githaiga, P. N. (2020a). Human capital, income diversification, and bank performance—an empirical study of East African banks Asian Journal of Accounting Research, 6(1), 95–108. https://doi.org/10.1108/ajar-06-2020-0041
- Githaiga, P. N. (2020b). Intellectual capital, income diversification, and financial performance of commercial banks in Kenya Journal ofEconomics and Business, 23(9), 151–156.
- Githaiga, P. N. (2022). Revenue diversification and financial sustainability of microfinance institutions Asian Journal of Accounting Research, 7(1), 31–43. https://doi.org/10.1108/ajar-11-2020-0122
- Guerry, N., & Wallmeier, M. (2017). Valuation of diversified banks: new evidence Journal of Banking and Finance, 80, 203–214. https://doi.org/10.1016/j.jbankfin.2017.04.004
- Gujarati, J. (2012). A comprehensive induction system A key to the retention of highly qualified teachers. The Educational Forum, 76(2), 218–223. https://doi.org/10.1080/00131725.2011.652293
- Guthrie, J. P. (2001). High-involvement work practises, turnover, and productivity: Evidence from New Zealand Academy of Management Journal, 44(1), 180–190. https://doi.org/10.2307/3069345
- Hair, J. F., & Joseph, F. (2007). Research methods for Business. Education + Training, 49(4), 336–337. https://doi.org/10.1108/et.2007.49.4.336.2
- Hair, J. F., & Joseph, F. (2007). Successful strategies for teaching multivariate statistics Proceedings of the 7th International Conference, 49(4), 336–337. https://doi.org/10.1108/et.2007.49.4.336.2
- Hamid, F. S., & Ibrahim, M. H. (2020). Competition, diversification, and performance in dual banking: A panel VAR analysis competition, diversification, and performance in dual Economic Research-Ekonomska Istraživanja, 1–27. https://doi.org/10.1080/1331677X.2020.1782242
- Hejazi, R., Ghanbari, M., & Alipour, M. (2016). Intellectual, human, and structural capital effects on firm performance as measured by Tobin’s Q. Knowledge and Process Management https://doi.org/10.1002/kpm.1529
- Hidayat, W. Y., Kakinaka, M., & Miyamoto, H. (2012). Bank risk and non-interest income activities in the Indonesian banking industry Journal of Asian Economics, 23(4), 335–343. https://doi.org/10.1016/j.asieco.2012.03.008
- Hoang, H. T., Nguyen, H. T. H., Vu, N. H., Le, A. H., & Quach, H. H. (2020). Intellectual capital and firm performance in Vietnam, 2012–2016 International Journal of Learning and Intellectual Capital, 17(1), 27. https://doi.org/10.1504/IJLIC.2020.105325
- Hunjra, A. I., Hanif, M., Mehmood, R., & Nguyen, L. V. (2020). Diversification, corporate governance, regulation, and bank risk-taking Journal of Financial Reporting and Accounting, 19(1), 92–108 https://doi.org/10.1108/JFRA-03-2020-0071
- Huynh, J., Dang, V. D., & McMillan, D. (2021). Loan portfolio diversification and bank returns: Do business models and market power matter? Cogent Economics & Finance, 9(1) https://doi.org/10.1080/23322039.2021.1891709
- Ibrahim, M., & Alagidede, P. (2017). Financial development, growth volatility, and information asymmetry in sub-Saharan Africa: Does Law Matter? South African Journal of Economics, 85(4), 570–588 https://doi.org/10.1111/saje.12176
- Ikeora, J. J., Igbodika, M. A., & Andabai, P. (2016). Banking sector reforms and the performance of the Nigerian economy: an A vector error correction investigation (VECM) European Journal of Research and Reflection in Management Sciences. 4 (2).
- Inkinen, H. (2015). Review of empirical research on intellectual capital and firm performance Journal of Intellectual Capital, 16(3), 518–565. https://doi.org/10.1108/JIC-01-2015-0002
- Ishak, Z., & Napier, C. (2006). Expropriation of minority interests and corporate diversification in Malaysia Asian Academy of Management Journal of Accounting and Finance, 2, 85–113.
- Jensen, C. M. (1986). Agency costs of free cash flow, corporate Finance, and takeovers agency costs of free cash flow, corporate Finance, and takeovers. The American Economic Review, 76 (2) 323–329.
- Jouida, S., & Hellara, S. (2018). Diversification, capital structure, and performance: A simultaneous equation approach Managerial and Decision Economics, 39(2), 117–130. https://doi.org/10.1002/mde.2874
- Kaplan, R. S., & Norton, D. P. (2004). Measuring the strategic readiness of intangible assets Harvard Business Review, 82 (2) 52–63.
- Kennedy, P. (2008). A guide to Econometrics (6th ed.). Wiley-Blackwell.
- Khan, S. Z., Yang, Q., & Waheed, A. (2019). Investment in intangible resources and capabilities spurs sustainable competitive advantage and firm performance. Corporate Social Responsibility and Environmental Management, 26(2), 285–295. https://doi.org/10.1002/csr.1678
- Klein, P. G., & Saidenberg, M. R. (2005) Diversification, organisation, and efficiency: evidence from bank holding companies SSRN Electronic Journal. https://doi.org/10.2139/ssrn.98653
- Köhler, M. (2015). Which banks are more risky? The impact of business models on bank stability Journal of Financial Stability, 16, 195–212. https://doi.org/10.1016/j.jfs.2014.02.005
- Kolachi, N. A., & Shah, H. A. (2013) BRICS countries and their strategic HRD agenda in 2020 International Journal of Management and Information Systems (IJMIS), 17(2), 105 https://doi.org/10.19030/ijmis.v17i2.7714
- Kor, Y. Y., & Mahoney, J. T. (2005). How dynamics, management, and governance of resource deployments influence firm-level performance Strategic Management Journal, 26(5), 489–496. https://doi.org/10.1002/smj.459
- Kremer, S., Bick, A., & Nautz, D. (2013). Inflation and growth: new evidence from a dynamic panel threshold analysis Empirical Economics, 44(2), 861–878. https://doi.org/10.1007/s00181-012-0553-9
- Laeven, L., & Levine, R. (2007). Is there a diversification discount in financial conglomerates? Journal of Financial Economics, 85(2), 331–367. https://doi.org/10.1016/j.jfineco.2005.06.001
- Lee, Y., Kim, W. C., & Kim, J. H. (2020). Achieving portfolio diversification for individuals with low financial sustainability Sustainability, 12(17), 7073. https://doi.org/10.3390/su12177073
- Le, T. D., Ho, T. H., Nguyen, D. T., & Ngo, T. (2022). A cross-country analysis on diversification, Sukuk investment, and the performance of Islamic banking systems under the COVID-19 pandemic Heliyon, 8(3), e09106. https://doi.org/10.1016/j.heliyon.2022.e09106
- Le, T. D. Q., & McMillan, D. (2021). Geographic expansion, income diversification, and bank stability: evidence from Vietnam Cogent Business & Management, 8(1) https://doi.org/10.1080/23311975.2021.1885149
- Le, T. D. Q., Nguyen, D. T., & McMillan, D. (2020). Intellectual capital and bank profitability: new evidence from Vietnam Cogent Business & Management, 7(1), 1859666. https://doi.org/10.1080/23311975.2020.1859666
- Lewellen, W. G. (1971). A pure financial rationale for the conglomerate merger. The Journal of Finance, 26(2), 521–537. https://doi.org/10.1111/j.1540-6261.1971.tb00912.x
- Li, S. (2019). Banking sector reform, competition, and bank stability: An empirical analysis of transition countries Emerging Markets Finance and Trade, 55(13), 3069–3093. https://doi.org/10.1080/1540496X.2018.1540349
- Liang, H. Y., Kuo, L. W., Chan, K. C., & Chen, S. H. (2020). Bank diversification, performance, and corporate governance: Evidence from China Asia-Pacific Journal of Accounting and Economics, 27(4), 389–405. https://doi.org/10.1080/16081625.2018.1452618
- Ljubownikow, G., & Ang, S. H. (2020). Competition, diversification, and performance Journal of Business Research, 112, 81–94. https://doi.org/10.1016/j.jbusres.2020.03.002
- Luu, H. N., Nguyen, L. Q. T., Vu, Q. H., & Tuan, L. Q. (2019). Income diversification and financial performance of commercial banks in Vietnam Review of Behavioural Finance, 12(3), 185–199. https://doi.org/10.1108/rbf-05-2019-0066
- Luu, H. N., Nguyen, L. Q. T., Vu, Q. H., & Tuan, L. Q. (2020). Income diversification and financial performance of commercial banks in Vietnam: Do experience and ownership structure matter? Review of Behavioural Finance, 12(3), 185–199. https://doi.org/10.1108/RBF-05-2019-0066
- Maji, S. G., & Hussain, F. (2021). Technical efficiency, intellectual capital efficiency, and bank performance in emerging markets: the case of India Journal of Advances in Management Research, 18(5), 708–737 https://doi.org/10.1108/JAMR-09-2020-0218
- Majumder, M. T. H., Ruma, I. J., & Akter, A. (2023). Does intellectual capital affect bank performance? Evidence from Bangladesh LBS Journal of Management & Research, ahead-of-print https://doi.org/10.1108/LBSJMR-05-2022-0016
- Markowitz, H. M. (1952). Portfolio selection. Journal of Finance, 7(1), 77–91. https://doi.org/10.1111/j.1540-6261.1952.tb01525.x
- Markowitz, H. M. (1991). Foundations of portfolio theory Harry Markowitz: Selected Works, 46(2), 481–490. https://doi.org/10.2307/2328831
- Marzo, G. (2022). A theoretical analysis of the value-added intellectual coefficient (VAIC) Journal of Management & Governance, 26(2), 551–577. https://doi.org/10.1007/s10997-021-09565-x
- Meles, A., Porzio, C., Sampagnaro, G., & Verdoliva, V. (2016). The impact of intellectual capital efficiency on commercial bank performance: Evidence from the US Journal of Multinational Financial Management, 36, 64–74. https://doi.org/10.1016/j.mulfin.2016.04.003
- Meng, X., Cavoli, T., & Deng, X. (2018) Determinants of income diversification: Evidence from Chinese banks Applied Economics, 50(17), 1934–1951. https://doi.org/10.1080/00036846.2017.1383594
- Mercieca, S., Schaeck, K., & Wolfe, S. (2007). Small European banks: benefits from diversification? Journal of Banking and Finance, 31(7), 1975–1998. https://doi.org/10.1016/j.jbankfin.2007.01.004
- Meslier, C., Tacneng, R., & Tarazi, A. (2014). Is bank income diversification beneficial? Evidence from an emerging economy Journal of International Financial Markets, Institutions, and Money, 31, 97–126 https://doi.org/10.1016/j.intfin.2014.03.007
- Mohammed, J. I., Karimu, A., Fiador, V. O., & Abor, J. Y. (2020). Oil revenues and economic growth in oil-producing countries: The role of domestic financial markets Resources Policy, 69, 101832. https://doi.org/10.1016/j.resourpol.2020.101832
- Mondal, A., & Ghosh, S. K. (2012). Intellectual capital and financial performance of Indian banks Journal of Intellectual Capital, 13(4), 515–530 https://doi.org/10.1108/14691931211276115
- Muhammad, R., Mangawing, M. A., & Salsabilla, S. (2021). The influence of intellectual capital and corporate governance on financial performance of Islamic banks. Jurnal Ekonomi & Keuangan Islam. https://doi.org/10.20885/jeki.vol7.iss1.art6
- Natsir, K., & Bangun, N. (2021). The role of intellectual capital in increasing company value with profitability as an intervening variable Proceedings of the Ninth International Conference on Entrepreneurship and Business Management (ICEBM 2020) https://doi.org/10.2991/aebmr.k.210507.016
- Nguyen, D. Q. (2018). The impact of intellectual capital and knowledge flows on incremental and radical innovation: Empirical findings from a transition economy of Vietnam. Asia-Pacific Journal of Business Administration. https://doi.org/10.1108/APJBA-03-2018-0044
- Nguyen, N. (2019). Revenue diversification, risk, and bank performance of Vietnamese commercial banks Journal of Risk and Financial Management, 12(3), 138 https://doi.org/10.3390/jrfm12030138
- Nguyen, D. T., Le, T. D. Q., & Tran, S. H. (2023). The moderating role of income diversification on the relationship between intellectual capital and bank performance is evidenced in Viet Nam. Cogent Business & Management, 10(1) https://doi.org/10.1080/23311975.2023.2182621
- Nguyen, T. C., Vinh Vo, D., & Nguyen, V. C. (2015) Risk and income diversification in the Vietnamese banking system Journal of Applied Finance and Banking, 5 (1), 93.
- Nisar, S., Peng, K., Wang, S., & Ashraf, B. (2018). The impact of revenue diversification on bank profitability and stability: empirical evidence from South Asian countries International Journal of Financial Studies, 6(2), 40 https://doi.org/10.3390/ijfs6020040
- North, K., & Kumta, G. (2018). Towards a digitally enabled knowledge society https://doi.org/10.1007/978-3-319-59978-6_1
- Nyantakyi-Frimpong, H. (2017). Agricultural diversification and dietary diversity: A feminist political ecology of the everyday experiences of landless and smallholder households in northern Ghana Geoforum, 86, 63–75. https://doi.org/10.1016/j.geoforum.2017.09.003
- Ofoeda, I., Agbloyor, E., & Abor, J. Y. (2022). Financial sector development, anti-money laundering regulations, and economic growth International Journal of Emerging Markets https://doi.org/10.1108/IJOEM-12-2021-1823
- Olarewaju, O. M., Migiro, S. O., & Sibanda, M. (2018) Operational diversification and financial performance of sub-Saharan African commercial banks: a static and dynamic approach Financial institutions and services operational diversification and financial performance of sub-Saharan African commercial banks Financial Institutions and Services, 13(5), 84–106.
- Onumah, J. M., & Duho, K. C. T. (2015). Intellectual capital: its impact on the financial performance and financial stability of Ghanaian banks Athens Journal of Business and Economics. https://doi.org/10.30958/ajbe.5-3-4
- Onumah, J. M., & Duho, K. C. T. (2020). Impact of intellectual capital on bank efficiency in emerging markets: Evidence from Ghana International Journal of Banking, Accounting and Finance, 11(4), 435. https://doi.org/10.1504/IJBAAF.2020.110303
- Ousama, A. A., Hammami, H., & Abdulkarim, M. (2020). The association between intellectual capital and financial performance in the Islamic banking industry: An analysis of the GCC banks International Journal of Islamic and Middle Eastern Finance and Management, 13(1), 75–93. https://doi.org/10.1108/IMEFM-05-2016-0073
- Ozkan, N., Cakan, S., & Kayacan, M. (2017). Intellectual capital and financial performance: A study of the Turkish banking sector Borsa Istanbul Review, 17(3), 190–198. https://doi.org/10.1016/j.bir.2016.03.001
- Pasiouras, F., & Kosmidou, K. (2007). Factors influencing the profitability of domestic and foreign commercial banks in the European Union Research in International Business and Finance, 21(2), 222–237. https://doi.org/10.1016/j.ribaf.2006.03.007
- Penrose, E. T. (1960). The growth of the firm—A case study: The Hercules Powder Company Business History Review, 34(1), 1–23. https://doi.org/10.2307/3111776
- Pulic, A. (1998). Measuring the performance of intellectual potential in the knowledge economy The 2nd “World Congress on the Management of Intellectual Capital”.
- Pulic, A. (2000). VAICTM isan accounting tool for IC management. International Journal of Technology Management, 20(5/6/7/8), 702. https://doi.org/10.1504/ijtm.2000.002891
- Pulic, A. (2004). Intellectual capital – does it create or destroy value? Measuring Business Excellence, 8(1), 62–68. https://doi.org/10.1108/13683040410524757
- Pulic, A. (2008). The principles of intellectual capital efficiency—a - a brief description Croatian Intellectual Capital Centre.
- Quyen, P. G., Ha, N. T. T., Darsono, S. N. A. C., & Minh, T. D. T. (2021). Income diversification and financial performance: The mediating effect of banks’ size, ownership structure, and the financial crisis in Vietnam Journal of Accounting and Investment, 22(2) https://doi.org/10.18196/jai.v22i2.10775
- Roodman, D. (2009a). How to do xtabond2: An Introduction to Difference and System GMM in Stata The Stata Journal: Promoting Communications on Statistics and Stata, 9(1), 86–136. https://doi.org/10.1177/1536867x0900900106
- Roodman, D. (2009a). A note on the theme of too many instruments Oxford Bulletin of Economics and Statistics, 71(1), 135–158. https://doi.org/10.1111/j.1468-0084.2008.00542.x
- Saif-Alyousfi, A. Y. H. (2020). Determinants of bank shareholder value: Evidence from GCC countries International Journal of Managerial Finance, 16(2), 224–252. https://doi.org/10.1108/IJMF-05-2019-0170
- Sanya, S., & Wolfe, S. (2011). Can banks in emerging economies benefit from revenue diversification? Journal of Financial Services Research, 40(1-2), 79–101. https://doi.org/10.1007/s10693-010-0098-z
- Sardo, F., & Serrasqueiro, Z. (2017). A European empirical study of the relationship between firms’ intellectual capital, financial performance, and market value. Journal of Intellectual Capital, 18(4), 771–788 https://doi.org/10.1108/JIC-10-2016-0105
- Saunders, M., Lewis, P., & Thornhill, A. (2009). Research methods for business students (Fitfth ed.) Pearson Education.
- Sekaran, U., & Bougie, R. (2011). Business research methods: A skill-building approach Wiley. http://as.wiley.com/WileyCDA/WileyTitle/productCd-111994225X.html#
- Seo, M. H., Kim, S., & Kim, Y. J. (2019). Estimation of a dynamic panel threshold model using Stata Stata Journal, 19(3), 685–697. https://doi.org/10.1177/1536867X19874243
- Seo, M. H., & Shin, Y. (2016). Dynamic panels with threshold effects and endogeneity Journal of Econometrics, 195(2), 169–186. https://doi.org/10.1016/j.jeconom.2016.03.005
- Serenko, A., & Bontis, N. (2004). Meta-review of knowledge management and intellectual capital literature: Citation impact and research productivity rankings Knowledge & Process Management, 11(3), 185–198. https://doi.org/10.1002/kpm.203
- Sgrò, F., Ciambotti, G., Bontis, N., & Ayiku, A. (2020). Intellectual capital in east and West African social enterprises. Knowledge & Process Management, 27(4), 332–344. https://doi.org/10.1002/kpm.1638
- Shahzad, F., Baig, M. H., Rehman, I. U., Saeed, A., & Asim, G. A. (2021). Does intellectual capital efficiency explain corporate social responsibility engagement-firm performance relationship? Evidence from environmental, social and governance performance of US listed firms. Borsa Istanbul Review, 22(2), 295–305. https://doi.org/10.1016/j.bir.2021.05.003
- Sharma, S., & Anand, A. (2018). Income diversification and bank performance: Evidence from BRICS nations. International Journal of Productivity and Performance Management, 67(9), 1625–1639. https://doi.org/10.1108/IJPPM-01-2018-0013
- Sharma, S., & Anand, A. (2020). Geographical diversification and bank performance: Evidence from Indian banks. International Journal of Productivity and Performance Management, 69(3), 583–596. https://doi.org/10.1108/IJPPM-01-2019-0049
- Shim, J. (2019). Loan portfolio diversification, market structure and bank stability. Journal of Banking and Finance, 104, 103–115. https://doi.org/10.1016/j.jbankfin.2019.04.006
- Smriti, N., & Das, N. (2018). The impact of intellectual capital on firm performance: A study of Indian firms listed in COSPI. Journal of Intellectual Capital, 19(5), 935–964. https://doi.org/10.1108/JIC-11-2017-0156
- Soewarno, N., & Tjahjadi, B. (2020). Measures that matter: An empirical investigation of banking firms’ intellectual capital and financial performance in Indonesia. Journal of Intellectual Capital, 21(6), 1085–1106. https://doi.org/10.1108/JIC-09-2019-0225
- Staikouras, S. K. (2003). The interest rate risk exposure of financial intermediaries: A Review of the theory and empirical evidence. In Financial markets. Institutions and Instruments. https://doi.org/10.1111/1468-0416.t01-1-00002
- Stiroh, K. J. (2004). Diversification in banking: Is noninterest income the answer? Journal of Money, Credit, and Banking, 36(5), 853–882. https://doi.org/10.1353/mcb.2004.0076
- Stiroh, K. J., & Rumble, A. (2006). The dark side of diversification: The case of US financial holding companies. Journal of Banking and Finance, 30(8), 2131–2161. https://doi.org/10.1016/j.jbankfin.2005.04.030
- Stulz, R. M. (1990). Managerial discretion and optimal financing policies. Journal of Financial Economics, 26(1), 3–27. https://doi.org/10.1016/0304-405X(90)90011-N
- Suseno, N. S., Hermina, T., Ramdhani, A., & Utari, L. (2019). The impact of intellectual capital on financial performance. International Journal of Recent Technology and Engineering, 8(1), 359–365. https://doi.org/10.30871/jama.v1i1.1239
- Swart, J. (2006). Intellectual capital: Disentangling an enigmatic concept. Journal of Intellectual Capital, 7(2), 136–159. https://doi.org/10.1108/14691930610661827
- Syahyunan Muda, I., Siregar, H. S., Sadalia, I., & Chandra, G. (2017). The effect of Lerner Index and income diversification on the general bank stability in Indonesia. Banks and Bank Systems, 12(4), 56–64. https://doi.org/10.21511/bbs.12(4).2017.05
- Tariq, W., Usman, M., Tariq, A., Rashid, R., Yin, J., Memon, M. A., & Ashfaq, M. (2021). Bank maturity, income diversification, and bank stability. Journal of Business Economics and Management, 22(6), 1492–1511. https://doi.org/10.3846/jbem.2021.15583
- Teece, D. J. (1980). Economies of scope and the scope of the enterprise. Journal of Economic Behavior and Organization, 1(3), 223–247. https://doi.org/10.1016/0167-2681(80)90002-5
- Tiwari, R., & Vidyarthi, H. (2018). Intellectual capital and corporate performance: A case of Indian banks. Journal of Accounting in Emerging Economies, 8(1), 84–105. https://doi.org/10.1108/JAEE-07-2016-0067
- Tiwari, R., Vidyarthi, H., & Kumar, A. (2023). Nexus between intellectual capital and bank productivity in India. Journal of Risk and Financial Management, 16(1), 54. https://doi.org/10.3390/jrfm16010054
- Tran, N. P., Van, L. T. H., & Vo, D. H. (2020). The nexus between corporate governance and intellectual capital in Vietnam. Journal of Asia Business Studies, 14(5), 637–650. https://doi.org/10.1108/JABS-01-2020-0007
- Tran, D. B., & Vo, D. H. (2018). Should bankers be concerned with intellectual capital? A study of the Thai banking sector. Journal of Intellectual Capital, 19(5), 897–914. https://doi.org/10.1108/JIC-12-2017-0185
- Uddin, M. J., Majumder, M. T. H., Akter, A., & Zaman, R. (2021). Do the diversification of income and assets spur bank profitability in Bangladesh? A dynamic panel data analysis. Vilakshan - XIMB Journal of Management, 19(2), 177–194. https://doi.org/10.1108/xjm-01-2021-0023
- Ulum, I., Ghozali, I., & Purwanto, A. (2014). Intellectual capital performance of Indonesian banking sector: A modified VAIC (M-VAIC) perspective. Asian Journal of Finance & Accounting, 6(2), 103. https://doi.org/10.5296/ajfa.v6i2.5246
- Ur Rehman, A., Aslam, E., & Iqbal, A. (2022). Intellectual capital efficiency and bank performance: Evidence from Islamic banks. Borsa Istanbul Review, 22(1), 113–121. https://doi.org/10.1016/j.bir.2021.02.004
- Uslu, H. (2022). The role of intellectual capital in financial development: Evidence from the banking sector of Turkey. Competitiveness Review, 32(2), 230–249. https://doi.org/10.1108/CR-06-2020-0084
- Valaei, N., Rezaei, S., Bressolles, G., & Dent, M. M. (2022). Indispensable components of creativity, innovation, and FMCG companies’ competitive performance: A resource-based view (RBV) of the firm. Asia-Pacific Journal of Business Administration https://doi.org/10.1108/APJBA-11-2020-0420
- Vidyarthi, H. (2019). Dynamics of intellectual capitals and bank efficiency in India. The Service Industries Journal, 39(1), 1–24. https://doi.org/10.1080/02642069.2018.1435641
- Vidyarthi, H. (2020). Dynamics of income diversification and bank performance in India. Journal of Financial Economic Policy, 12(3), 383–407. https://doi.org/10.1108/JFEP-05-2019-0084
- Vo, D. H., & Tran, N. P. (2021). Intellectual capital and bank performance in Vietnam. Managerial Finance, 47(8), 1094–1106. https://doi.org/10.1108/MF-03-2020-0143
- Wang, C., & Lin, Y. (2021). Income diversification and bank risk in Asia Pacific. The North American Journal of Economics & Finance, 57, 101448. https://doi.org/10.1016/j.najef.2021.101448
- Weqar, F., Sofi, Z. A., & Haque, S. M. I. (2021). Nexus between intellectual capital and business performance: Evidence from India. Asian Journal of Accounting Research, 6(2), 180–195. https://doi.org/10.1108/ajar-07-2020-0064
- Wintoki, M. B., Linck, J. S., & Netter, J. M. (2012). Endogeneity and the dynamics of internal corporate governance. Journal of Financial Economics, 105(3), 581–606. https://doi.org/10.1016/j.jfineco.2012.03.005
- Wooldridge, J. M. (2013). Introductory econometrics a modern approach (5th ed.). Cengage Learning.
- Wooldridge, J. M. (2016). Introductory econometrics 6th edition. Economica, 42(165).
- Wu, S. H., Lin, L. Y., & Hsu, M. Y. (2007). Intellectual capital, dynamic capabilities and innovative performance of organisations. International Journal of Technology Management, 39(3/4), 279. https://doi.org/10.1504/IJTM.2007.013496
- Yaseen, H., & Al-Amarneh, A. (2021). Intellectual capital and financial performance: Case of the emerging market banks. Journal of Governance & Regulation, 10(1), 35–41. https://doi.org/10.22495/jgrv10i1art4
- Yu, J. R., Lee, W. Y., & Chiou, W. J. P. (2014). Diversified portfolios with different entropy measures. Applied Mathematics and Computation, 241, 47–63. https://doi.org/10.1016/j.amc.2014.04.006
- Zou, K., & Xinyi Cai, J. H. (2021). An empirical study on the influence of diversification on the operating cost of commercial banks. Converter, 2021(5), 757–770. https://doi.org/10.17762/converter.342
Appendix
Table A1. Endogeneity test
Table A2. Breusch-Pagan/Cook-Weisberg test for heteroscedasticity
Table A3. Wooldridge test for autocorrelation in panel data