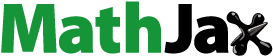
Abstract
The present study investigates the relationship between innovation (INN) and foreign direct investment (FDI) attraction across 66 developing countries from 2013 to 2021. Adopting the Difference Generalized Method of Moments estimation, the study reveals a statistically positive INN-FDI nexus. Panel Granger causality analysis further indicates a bidirectional between the two variables. Additionally, via feature importance analysis, it is evident that market size, labor resources, and financial development play a critical role in strongly influencing FDI inflows, while innovation shows smaller magnitude. Furthermore, trade openness demonstrates a significantly positive impact on FDI with low impact, while inflation has an insignificantly negative effect on FDI. Policy implications are also discussed.
Impact Statement
Developing countries, particularly those seeking to attract foreign direct investment (FDI), consider paying attention to the innovation (INN) factor. Alongside traditional factors such as market size and abundant labor force are strengths of FDI attraction for developing countries, new models should be continually developed. In this context, the researcher hypothesizes that multinational enterprises are interested in new resources related to INN to meet their production requirements. Indeed, INN and its efficacy in attracting FDI in developing countries, which pay less resources to allocate innovation, remains unproven. Drawing upon data from the global innovation index, the experimental findings of this research show the bidirectional FDI-INN nexus and suggest that policies aimed at attracting FDI should prioritize those based on advanced technologies. Additionally, via feature importance analysis, market size and labor force cannot be ignored in the analyzed context.
REVIEWING EDITOR:
1. Introduction
Over the past few decades, foreign direct investment (FDI) has played a significant role in developing countries (DGCs). FDI followed as multinational companies (MNCs) established subsidiaries or outsourced manufacturing operations to host countries to take benefits offered. In addition to providing capital, MNCs are expected to generate spillover effects in host countries by introducing new technologies, transferring skills, enhancing competition, and opening up markets (Khachoo and Sharma, Citation2016). Previous research has consistently shown that FDI has a positive impact on various aspects, including economic growth (A. T. Nguyen et al., Citation2022), international trade (Asiedu & Lien, Citation2004), labor capacity (Rodríguez & Pallas, Citation2008), technological advancement, research and development (Erdal & Göçer, Citation2015), as well as reducing carbon emissions (Ben Jebli et al., Citation2019).
The global flow of FDI into DGCs has exhibited a consistent upward trend. As depicted in , FDI inflows into DGCs remained relatively low prior to 2002, accounting for less than $200 billion and constituting less than 20% of the global share. The figures can be explained by the dominance of key manufacturing industries and the technological advantages held by developed countries, which limited the influx of FDI into DGCs. However, since 2002, the value and global proportion of FDI inflows into DGCs have risen significantly, reaching 50% of the global share in 2014. The year 2020 marked a peak in FDI flows into DGCs, comprising approximately 67% of the global share. The figure underscores the attractiveness of DGCs as prime destinations for FDI worldwide. The growth of FDI in DGCs signifies the expanding business opportunities in these countries, motivating MNCs to seek profitable ventures in the region.
Figure 1. Evolution of FDI inflows in developing countries, 1970–2021.
Source: UNCTAD (Citation2023).
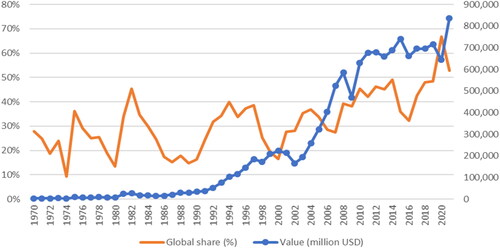
The remarkable increase in FDI can be attributed to their advantages in terms of production efficiency (Coughlin & Segev, Citation1999), infrastructure, such as transportation and electricity (Head & Ries, Citation1996), low labor costs (Cheng & Kwan, Citation2000), or sizeable markets (Narula & Dunning, Citation2000). However, the issues of population aging and high inflation in host countries may cause these advantage factors to diminish (Ezeoha & Cattaneo, Citation2012; Pavlínek, Citation2018). Plus, stringent regulations, especially environmental ones, to select higher quality new generation FDI inflows have been put on the agenda in DGCs (Y. Kim & Rhee, Citation2019). As a result, countries followed the neoclassical Solow (Solow, Citation1956), which highlighted the crucial role of capital and labor (Olorogun et al., Citation2022), ought to adjust paths to attract FDI in the new competitive context. Within this background, innovationFootnote1 (INN) is assumed a pivotal role in governmental policy agendas to foster a technology-driven development model (Looy et al., Citation2003). This is a very recent and encouraging trend that makes room for INN so that it is not only in a few elite economies and clusters but also DGCs (Cornell University, INSEAD, & WIPO, Citation2020). Mueller (Citation2006) further contends that INN, acting as the foundation for research and development, catalyzes regional economic growth, complementing the roles played by capital and labor. This fact challenges Therefore, this study specifically focuses on examining the role of INN in attracting FDI to DGCs. The INN index and FDI inflows into DGCs are globally correlated as MNCs prioritize establishing long-term production and consumption markets in countries where new elements like technology foster competitive environments. In today’s dynamic landscape of global competition, where the inevitable development of new technologies and products occurs to further competitive advantages, traditional determinants such as abundant natural resources or existing factors no longer hold primary importance in driving competitiveness (Erdal & Göçer, Citation2015). Instead, DGCs have recently incorporated innovation policies into their national policy scheme, and MNCs have placed innovation programs at the center of policymaking (Lewis et al., Citation2021). By exploring the relationship between INN and FDI, our findings show that innovation is not only a phenomenon found in advanced economies but also a required condition in DGCs, which seek a new pattern to receive FDI inflows. The findings add to the literature an argument that DGCs consider adapting INN as a new factor of FDI attraction. This article also examines other potential drivers of FDI, which currently play significant roles in attracting FDI. Based on the empirical findings, the study discusses policy-enhancing FDI inflows to this region.
2. Review of related literature
FDI has been observed to positively impact the performance of local companies (LCCs). The positive effect is primarily because multinational corporations (MNCs) have access to advanced technologies and specialized know-how, which surpasses that available to local companies (Cantwell & Iammarino, Citation2000). FDI generates spillover effects within and across industries via competitiveness and labor mobility, helping LCCs increase their productivity (Khachoo & Sharma, Citation2016). Regarding the intra-industry channel, FDI influences LCCs through learning by doing (Günther, Citation2002) or transferring human capital from MNCs. As a result, LCCs perform better due to experience and knowledge from trained human resources (Cheung & Ping, Citation2004).
MNCs support the effectiveness of LCCs primarily through the transfer of new products, knowledge, and technology. This phenomenon is known as the inter-industry channel (Iammarino & McCann, Citation2013). When MNCs enter local markets with high levels of productivity and advanced technology, it pressures LCCs to pursue innovation by increasing their investment in research and development (R&D) (Keller, Citation2010). R&D, in turn, enables LCCs to enhance their competitive capabilities by adopting new technologies and improving management techniques, all facilitated by FDI. Cheung and Ping (Citation2004) identify three strategies through which MNCs support the potential for innovation among LCCs. Firstly, MNCs introduce advanced products and technologies, allowing LCCs to learn from these technologies. Secondly, since these products have already been tested in developed markets, LCCs are incentivized to engage in innovation activities. Lastly, MNCs and LCCs can share expertise and transfer technology, leading to direct vertical integration between the two entities.
Empirical research has supported the positive effects of FDI on INN. Ascani et al. (Citation2020) state that superior knowledge possessed by MNCs can be diffused to LCCs, thereby increasing LCCs’ capacity for innovation, particularly in science-based industries. Erdal and Göçer (Citation2015) find that FDI stimulates R&D activities and patent applications in ten developing countries in Asia. Crescenzi et al. (Citation2015) examine the impact of MNCs on LCCs’ innovative performance in the UK. The findings reveal that LCCs operating in regions with higher levels of MNCs investment demonstrated greater innovative performance than others. However, the level of competitive positioning between MNCs and LCCs, particularly in terms of vertical connectedness, determines the speed of innovation. Particularly, LCCs primarily operating in the local market have stronger connections with MNCs, allowing them to benefit from spillover effects. Conversely, LCCs with access to the global market are less driven to rely on vertical FDI for their innovation efforts.
Furthermore, factors conducive to innovation are crucial for attracting FDI flows. It indicates a nation’s transition to advanced manufacturing processes. As a result, MNCs could optimize inputs and outputs to reduce production costs. Additionally, FDI and innovation exhibit a bidirectional relationship, supporting positive spillover effects on a nation’s innovation ability. Governments recognize the role of FDI in knowledge transfer, international technology diffusion, and fostering innovation development (Li et al., Citation2021). Governments also strengthen intellectual property protection to attract FDI (Hsu & Tiao, Citation2015; Khoury & Peng, Citation2011).
The existing studies have identified research gaps in the relationship between FDI and INN. In other words, it is still unclear if these increases in innovation have increased the appeal of FDI. Furthermore, previous research has primarily focused on developed economies with high levels of innovation (Crescenzi et al., Citation2015; Li et al., Citation2021), while DGCs often face challenges such as insufficient technological infrastructure and limited investment in R&D (Luthra & Mangla, Citation2018; S. Wang et al., Citation2021). Therefore, there is a need to address this gap and explore the FDI-INN nexus in DGCs. Secondly, prior studies often used patent applications, R&D expenditure, and scientific publications as proxies for measuring INN (Ascani et al., Citation2020; Erdal & Göçer, Citation2015; Vernon et al., Citation2021), while this article utilizes the INN index from the Global Invention Index, which incorporates seven pillars to assess inventive innovation (Soumitra Dutta, Citation2011). INN encompasses social, business model, and technological innovation, and it may also be more broadly defined and horizontal in character (Cornell University, INSEAD, & WIPO, Citation2020). Using a broader measure of INN, this study offers new insights into the FDI-INN nexus in DGCs and provides pragmatic managerial implications.
3. Model and data
3.1. Proposed model
Based on previous literature models (Economou et al., Citation2017; Saini & Singhania, Citation2018; Xaypanya et al., Citation2015), we propose an empirical model to examine the FDI-INN nexus as the following equation:
(1)
(1)
where FDI represents FDI inflows, INN is the innovation variable, and other control variables include trade openness (TRA), market size (MAR), labor force (LAB), inflation rate (INF), and financial development (FID). In EquationEquation (1)
(1)
(1) , i represents countries (i = 1, 2…, N), and t represents test periods (t = 1, 2, …, T). The model also includes error term
to account for unobserved factors.
The following steps will be taken to investigate the relationship between those variables in EquationEquation (1)(1)
(1) : (i) testing the stationarity of variables; (ii) adopting Generalized Method of Moments (GMM) for coefficients; and (iii) examining the contribution of explanatory variables by Feature Importance Analysis (FIA).
In step (i), since the panel data includes time series characteristics, it is crucial to test for stationarity. Stationarity is a prerequisite to avoid spurious regression, where statistical significance between variables can be falsely observed due to non-stationary phenomena (Gujarati & Porter, Citation2009; Y. Wang et al., Citation2017). In this study, we applied three approaches to test for unit root, i.e., Im-Pesara-Shin proposed by Im et al. (Citation2003), ADF-Fisher, and PP-Fisher proposed by Maddala and Wu (Citation1999).
In step (ii), the GMM estimator is employed to address the potential endogeneity issues that may arise within the macro-variables in EquationEquation (1)(1)
(1) (Antonakis et al., Citation2010; Hill et al., Citation2021). The GMM estimator, proposed by Hansen (Citation1982), is considered more suitable than other instrumental variable methods like 2SLS, particularly when dealing with heteroskedasticity (Baum et al., Citation2003). Furthermore, considering the short panel characteristics of 66 countries over nine years (panels with large N and small T), the Ordinary Least Squares (OLS) estimator, such as the Fixed Effects Model (FEM), cannot yield stable covariance estimates (Allison & Waterman, Citation2002). Hence, we exclude OLS and 2SLS methods and opt for the GMM estimator due to its suit for the short panel (Góes, Citation2016). The GMM method comprises two types, i.e., Difference GMM (D-GMM) and System GMM (S-GMM). In this study, we employ D-GMM, which Arellano and Bond (Citation1991) introduced. The estimation form for D-GMM is as follows:
(2)
(2)
where
represents the coefficient of the lagged dependent variable, k, and
denotes the error term varying across countries (i) and time periods (t).
It is important to note that when the regressive process occurs continuously, may still be correlated with
leading to a potential downward bias in the D-GMM estimation, especially when T is small in the sample. The S-GMM estimator addresses this concern, which involves estimating two simultaneous equations (one at the level with first-lagged instruments and another in difference with k-lagged instruments) (Blundell & Bond, Citation1998). Let
and
represent the coefficients of the lagged variable
obtained through pooled OLS estimation, D-GMM, and fixed effects, respectively. If
>
>
choosing D-GMM is appropriate. Conversely, if
<
indicating a tendency towards downward bias, S-GMM estimation is preferred (Bond, Citation2002). Additionally, we conducted robustness tests to ensure the effectiveness of the GMM estimator. Two commonly used tests are the J Sargan-Hansen and the Arellano-Bond tests for over-identification restrictions in linear models with instruments (Anatolyev & Gospodinov, Citation2011). The J Sargan–Hansen test (Hansen, Citation1982; Sargan, Citation1958) assesses the appropriateness of instruments by testing the null hypothesis that the instruments are strictly exogenous. The Arellano and Bond (Citation1991) test examines the presence of first- and second-order autocorrelation of residuals, with the null hypothesis H0 stating that there is no such autocorrelation.
Furthermore, we adopted the Granger causality test (Granger, Citation1969) to investigate the directions of causality between variables. The approach assumes that all of the coefficients in the panel are the same and that there are no cross-sectional variations. The Granger causality test can show the overall direction of causality among variables. The null hypothesis, which states that there is no pairwise Granger causality among the variables, would be rejected at certain significance levels based on an analysis of the F-statistics (D.-W. Kim et al., Citation2018; Onifade et al., Citation2020).
In step (iii), we conducted FIA by using a game-theoretic approach – SHapley Additive exPlanations (SHAP) – to assess the contribution of explanatory variables to the dependent variable and identify the dominant factor in the relationship (Colak Oz et al., Citation2023). This analysis enables us to provide hierarchical drivers of FDI in the interests of DGCs.
3.2. Innovation index variable
The INN index is a composite index derived from seven pillars, each divided into three sub-pillars, that assess different aspects of innovativeness in a country. The seven pillars include institutions, human capital and research, infrastructure, market sophistication, business sophistication, knowledge and technology outputs, and creative outputs. Each pillar has three sub-pillars, and each subpillar has two to five individual indicators. The indicators’ scores aggregate the sub-pillar scores, and the sub-pillar scores comprise the pillar scores. The average scores of the first five and the last two pillars complement the INN (Cornell University, INSEAD, & WIPO, Citation2020). A higher value of the INN indicates a higher level of FDI (Ascani et al., Citation2020).
In our sample, the data includes 66 DGCs (classified as low-income, lower-middle-income, and upper-middle-income countries, according to the World Bank) between 2013 and 2021. DGCs have paid attention to enhancing their performance of seven pillars for consecutive years. In particular, several developing nations are outperforming expectations given their stage of economic growth (WIPO, Citation2021). For the upper-middle-income group, Bulgaria has output sub-indices comparable to the high-income group, such as Norway and Italy. At the same time, India’s innovation performance is above the average for the upper middle-income group in five of the seven innovation pillars. India is a successful case in developing sophisticated services that are technologically dynamic and traded internationally. Low-income sub-Saharan African economies such as Malawi, Madagascar, Ethiopia, and Guinea are efficient cases of transforming their limited innovation inputs and resources into innovation outputs. Readers could refer to the sheet “Appendix 1” (supplementary material) in the data file for detailed scores of seven pillars in our sample.
3.3. Data sources and expected signs
The data for the INN variable were extracted from the Global Innovation Index. FDI, TRA, MAR, INF, and FID variables were extracted from the World Development Indicator. Variables measurement and expected signs between the independent variables and the dependent variable (FDI) are presented in .
Table 1. Variables measurement and expected signs.
The tests were conducted using Eviews 10 software, while the FIA was performed using Python programming language code. In total, there were 594 observations available for the analysis. In order to access and replicate the results, the data has been deposited in the Mendeley data repository at the following link: https://data.mendeley.com/datasets/s7brrc9b63/3
4. Findings and discussion
4.1. Descriptive statistics
The variables’ description is presented in . From 2013 to 2021, the average FDI/GDP ratio was 3.64%. The number indicates that FDI substantially influences the region’s economy relative to GDP size. Moreover, the standard deviation of FDI stood at 4.09%, surpassing the average value. It suggests a considerable disparity in the countries’ ability to attract FDI.
Table 2. Variables description.
Regarding the INN index, the mean value amounts to 29.67. China had the first position between 2013 and 2021 with the highest score at 54.8. These numbers demonstrate China’s supremacy in terms of FDI volume and the INN category among DGCs. Notably, the standard deviation averagely is 6.46 and is lower than the minimum score, meaning the scores between countries are small. In other words, the 66 developing countries in the sample have the same condition in term of INN. Other variables like trade openness, market size, labor force, and financial development indicate significantly high average levels for DGCs, further reinforcing their capacity to attract FDI.
4.2. Correlation
The correlation test results for the analyzed variables are presented in . Most variables exhibit a significant correlation with each other at a 1% level of statistical significance. However, it is crucial to highlight that one pair of independent variables shows a high correlation coefficient of r(MAR, LAB) = 0.837, suggesting potential multicollinearity issues in the model (Gujarati & Porter, Citation2009). To investigate this problem, we conducted the Wald test to verify the necessity of including both variables in the proposed model. The test results indicate an F-value (MAR, LAB) = 18.16 at a significance level of 1%, which allows us to reject the null hypothesis H0 (a = b = 0). Therefore, the selected variables meet the inclusion criteria for the proposed model.
Table 3. Correlation matrix.
4.3. Panel unit root tests
presents the stationarity analysis for the variables included in the model, demonstrating that they are predominantly stationary at the level. Both the ADF-Fisher and PP-Fisher methods confirm this finding. Stationarity at the level implies that the variables follow a random walk, with their estimated values (mean, variance) remaining stable over time. Thus, the variables included in the model fulfill the necessary conditions for subsequent testing.
Table 4. Panel unit root tests.
4.4. Regression estimation results
The estimated coefficients of FDI(-1) using different estimators are presented in the first three rows of . The POLS, D-GMM, and FEM estimators yield coefficients of 0.75, 0.36, and 0.23, respectively. Notably, the estimated coefficient obtained via D-GMM surpasses the lower bound. It suggests that the D-GMM method is suitable for the sample under examination.
Table 5. Regression estimation results.
The findings in reveal a significant positive impact of INN on FDI at a 1% significance level. It aligns with previous research conducted by Li et al. (Citation2021) on innovative activities in OECD countries. These results provide evidence of a positive correlation between innovation and FDI attraction in DGCs. One possible explanation for this relationship is that increased innovation enhances the attractiveness of DGCs to MNCs, leading to higher inflows of FDI into these countries. This finding aligns with the practical deployment of innovative initiatives in DGCs globally to attract FDI, e.g., Panama and Tunisia (Lewis et al., Citation2021). Moreover, the Granger causality analysis demonstrates a reciprocal influence between INN and FDI at a significant level of 5%. This finding emphasizes that innovation has become a crucial production driver and is vital in attracting FDI alongside traditional resources in DGCs, such as abundant and affordable labor. In turn, FDI inflows stimulate innovative activities in DGCs. Specifically, FDI may have a favorable impact on the competitiveness of the inward economy’s production system since it activates the R&D in the host countries (Sekuloska, Citation2015). As a result, LCCs can benefit from new production methods introduced by MNCs. In other words, MNCs’ superior expertise may be shared with local companies. This is evidence not only in the case of developed countries (Ascani et al., Citation2020) but also in DGCs. This observation confirms the spillover effects of FDI on local production factors and corresponds to the levels of product quality and available resources in DGCs (Cantwell & Iammarino, Citation2000; Khachoo & Sharma, Citation2016). However, we note that the intensity of this trend likely depends on the host economies’ capability for absorption and their availability of assets that complement innovation (Fu, Citation2008). Therefore, the bidirectional causation indicates that policymakers consider creating innovative conditions to attract foreign investment.
Additionally, the findings depicted in highlight the continued significance of labor resources and market size as crucial factors in attracting FDI. These results are consistent with previous studies (Cieślik & Tran, Citation2019; Narula & Dunning, Citation2000; Shan, Lin, Li, & Zeng, Citation2018). It suggests that MNCs persist in redirecting their production capital flows towards DGCs to expand their market presence and leverage local labor resources. In a similar vein, the financial development significantly increases FDI inflows. This empirical evidence confirms the argument that countries with developed financial markets will be able to finance the operations of MNCs at reasonable fixed costs. In other words, the availability of external financing makes it easier for MNCs to cover the fixed costs of implementing FDI, leading to a high intention to invest in host countries (Donaubauer et al., Citation2020). The finding affirms the empirical evidence found within DGCs for the vital role of the financial sector (Olorogun et al., Citation2022). This finding also complements the results in C. P. Nguyen and Lee (Citation2021), which only shows the nexus between FID and FDI in developed host countries.
The impact of trade openness on FDI, although present, appears relatively small. The reason could be that while some MNCs prefer open markets with fewer trade barriers to reduce transaction costs, others may seek domestic markets with imposed barriers to limit competition from rivals and maximize profits within the domestic market (Asiedu, Citation2002). In other words, MNCs might be more interested in producing for the domestic consumer market rather than foreign markets within DGCs. As a result, while trade openness does influence FDI, the estimated coefficient reflects a minor effect with weak statistical significance.
As anticipated, inflation in DGCs exhibits an inverse relationship with FDI. An increase in inflation signals market instability, which diminishes the attractiveness of FDI. This is because the profit generated by MNCs may not adequately compensate for the losses caused by inflation. However, the estimated coefficient for inflation is not statistically significant in the sample, indicating that inflation is not a crucial factor in explaining FDI attraction.
Robust testing, such as the J Sargan-Hansen and Arellano-Bond (AR) tests, confirm the reliability of the D-GMM method. The probability results of P(J-statistic) = 0.1589 suggest that the null hypothesis H0, which states that instrumental variables are strictly exogenous, cannot be rejected. The probabilities of AR(1) and AR(2) are 0.0035 and 0.4616, respectively, indicating the presence of first-order autocorrelation but the absence of second-order autocorrelation. Consequently, the D-GMM estimator can be considered reliable.
This study also conducted the FIA to determine the magnitude of the impact of explanatory variables on FDI. The FIA results, as illustrated in , align with the regression findings in regarding the direction and magnitude of the impact. Specifically, market size demonstrates the most crucial role in explaining FDI attraction in DGCs, followed by labor resources (LAB), trade openness (TRA), and innovation (INN). Inflation (INF) has a counteracting effect of low magnitude in explaining FDI attraction in DGCs. These results reinforce the suggestion that innovation is a potential driver of FDI, while traditional strengths of DGCs, such as abundant labor resources and a sizeable market, continue to play critical roles in attracting FDI.
5. Conclusion and implications
This study examines the determinants of FDI, specifically focusing on the role of innovation, using data from 66 developing countries between 2013 and 2021. We emphasize DGCs, which are currently emerging as primary destinations for global FDI flows, thereby addressing research gaps in the existing literature. Using D-GMM estimation and panel Granger causality, we provide robust evidence of a positive bidirectional relationship between INN and FDI in our sample. Plus, FIA further corroborates that market size and the quantity of the labor force play crucial roles in driving FDI.
Our results highlight the significance of innovation in attracting FDI to DGCs and vice versa. Therefore, policymakers consider promoting innovation by encouraging an innovative conditions, as it directly contributes to FDI attraction. Innovation will foster the growth of science and technology-related factors, becoming vital with labor resources. When this symbiotic relationship is established, countries that embrace innovation will possess the necessary conditions to attract MNCs and directly benefit from the inflow of FDI.
Aside from the bidirectional nexus INN-FDI, we also find that market size and abundant labor resources in DGCs continue to influence attracting FDI. The results indicate that DGCs still have a room of prioritizing the exploitation of consumer markets and labor resources, which mainly aimed by MNCs. Policy makers, therefore, focus on maintaining the size of martket as well as the capacity of labor resources by fostering their development and ensuring their ability to absorb advanced production factors from MNCs.
Supplemental Material
Download MS Excel (108.8 KB)Disclosure statement
The author reports there are no competing interests to declare.
Data availability statement
The data have been deposited in the Mendeley data repository at the following link: https://data.mendeley.com/datasets/s7brrc9b63/3
Additional information
Notes on contributors
Nguyen Anh Tu
Nguyen Anh Tu is a lecturer at the Faculty of Banking, Ho Chi Minh City University of Banking. He received a Ph.D. in Business Administration from the National Taiwan University of Science and Technology. His main research interests include economic development, international trade, macroeconomics, and supply chain management integration. His favorite methods relate to programming and econometrics. His work has been published in Applied Economics, International Journal of Shipping and Transport Logistics, and Expert Systems with Applications.
Notes
1 INN is the implementation of a new or significantly improved product (service), a new process, a new marketing method, or a new organizational method in an organization/workplace, or new foreign relations (S Dutta et al., Citation2017).
References
- Allison, P. D., & Waterman, R. P. (2002). Fixed-effects negative binomial regression models. Sociological Methodology, 32(1), 247–265. https://doi.org/10.1111/1467-9531.00117
- Anatolyev, S., & Gospodinov, N. (2011). Specification testing in models with many instruments. Econometric Theory, 27(2), 427–441. https://doi.org/10.1017/S0266466610000307
- Antonakis, J., Bendahan, S., Jacquart, P., & Lalive, R. (2010). On making causal claims: A review and recommendations. The Leadership Quarterly, 21(6), 1086–1120. https://doi.org/10.1016/j.leaqua.2010.10.010
- Arellano, M., & Bond, S. (1991). Some tests of specification for panel data: Monte Carlo evidence and an application to employment equations. The Review of Economic Studies, 58(2), 277–297. https://doi.org/10.2307/2297968
- Ascani, A., Balland, P.-A., & Morrison, A. (2020). Heterogeneous foreign direct investment and local innovation in Italian Provinces. Structural Change and Economic Dynamics, 53, 388–401. https://doi.org/10.1016/j.strueco.2019.06.004
- Asiedu, E. (2002). On the determinants of foreign direct investment to developing countries: Is Africa different? World Development, 30(1), 107–119. https://doi.org/10.1016/S0305-750X(01)00100-0
- Asiedu, E., & Lien, D. (2004). Capital controls and foreign direct investment. World Development, 32(3), 479–490. https://doi.org/10.1016/j.worlddev.2003.06.016
- Baum, C. F., Schaffer, M. E., & Stillman, S. (2003). Instrumental variables and GMM: Estimation and testing. The Stata Journal: Promoting Communications on Statistics and Stata, 3(1), 1–31. https://doi.org/10.1177/1536867X0300300101
- Ben Jebli, M., Ben Youssef, S., & Apergis, N. (2019). The dynamic linkage between renewable energy, tourism, CO2 emissions, economic growth, foreign direct investment, and trade. Latin American Economic Review, 28(1), 1–19. https://doi.org/10.1186/s40503-019-0063-7
- Blundell, R., & Bond, S. (1998). Initial conditions and moment restrictions in dynamic panel data models. Journal of Econometrics, 87(1), 115–143. https://doi.org/10.1016/S0304-4076(98)00009-8
- Bond, S. R. (2002). Dynamic panel data models: A guide to micro data methods and practice. Portuguese Economic Journal, 1(2), 141–162. https://doi.org/10.1007/s10258-002-0009-9
- Buckley, P. J., Buckley, P. J., Clegg, L. J., Cross, A., Liu, X., Voss, H., & Zheng, P. (2010). The determinants of Chinese outward foreign direct investment. Springer.
- Cantwell, J., & Iammarino, S. (2000). Multinational corporations and the location of technological innovation in the UK regions. Regional Studies, 34(4), 317–332. https://doi.org/10.1080/00343400050078105
- Cheng, L., & Kwan, Y. K. (2000). The location of foreign direct investment in Chinese regions: further analysis of labor quality. In The role of Foreign Direct Investment in East Asian economic development (pp. 213–238). University of Chicago Press.
- Cheung, K-y., & Ping, L. (2004). Spillover effects of FDI on innovation in China: Evidence from the provincial data. China Economic Review, 15(1), 25–44. https://doi.org/10.1016/S1043-951X(03)00027-0
- Cieślik, A., & Tran, G. H. (2019). Determinants of outward FDI from emerging economies. Equilibrium. Quarterly Journal of Economics and Economic Policy, 14(2), 209–231. https://doi.org/10.24136/eq.2019.010
- Colak Oz, H., Güven, Ç., & Nápoles, G. (2023). School dropout prediction and feature importance exploration in Malawi using household panel data: machine learning approach. Journal of Computational Social Science, 6(1), 245–287. https://doi.org/10.1007/s42001-022-00195-3
- Cornell University, INSEAD, & WIPO. (2020). The Global Innovation Index 2020: Who will finance innovation. Cornell University.
- Coughlin, C. C., & Segev, E. (1999). Foreign direct investment in China: A spatial econometric study. Research Department, Federal Reserve Bank of St. Louis.
- Crescenzi, R., Gagliardi, L., & Iammarino, S. (2015). Foreign multinationals and domestic innovation: Intra-industry effects and firm heterogeneity. Research Policy, 44(3), 596–609. https://doi.org/10.1016/j.respol.2014.12.009
- Donaubauer, J., Neumayer, E., & Nunnenkamp, P. (2020). Financial market development in host and source countries and their effects on bilateral foreign direct investment. The World Economy, 43(3), 534–556. https://doi.org/10.1111/twec.12884
- Dutta, S. (2011). Global Innovation Index 2011: Accelerating growth and development. WIPO.
- Dutta, S., Lanvin, B., & Wunsch-Vincent, S. (2017). The Global Innovation Index(GII) conceptual framework. The Global Innovation Index 2011: Innovation feeding the world. WIPO.
- Economou, F., Hassapis, C., Philippas, N., & Tsionas, M. (2017). Foreign direct investment determinants in OECD and developing countries. Review of Development Economics, 21(3), 527–542. https://doi.org/10.1111/rode.12269
- Erdal, L., & Göçer, İ. (2015). The effects of foreign direct investment on R&D and innovations: Panel data analysis for developing Asian countries. Procedia - Social and Behavioral Sciences, 195, 749–758. https://doi.org/10.1016/j.sbspro.2015.06.469
- Ezeoha, A. E., & Cattaneo, N. (2012). FDI flows to Sub-Saharan Africa: The impact of finance, institutions, and natural resource endowment. Comparative Economic Studies, 54(3), 597–632. https://doi.org/10.1057/ces.2012.18
- Fu, X. (2008). Foreign direct investment, absorptive capacity and regional innovation capabilities: evidence from China. Oxford Development Studies, 36(1), 89–110. https://doi.org/10.1080/13600810701848193
- Góes, C. (2016). Institutions and growth: A GMM/IV panel VAR approach. Economics Letters, 138, 85–91. https://doi.org/10.1016/j.econlet.2015.11.024
- Granger, C. W. (1969). Investigating causal relations by econometric models and cross-spectral methods. Econometrica, 37(3), 424–438. https://doi.org/10.2307/1912791
- Gujarati, D. N., & Porter, D. (2009). Basic Econometric. McGraw-Hill.
- Günther, J. (2002). FDI as a multiplier of modern technology in Hungarian industry. Intereconomics, 37(5), 263–269. https://doi.org/10.1007/BF02928885
- Hansen, L. P. (1982). Large sample properties of generalized method of moments estimators. Econometrica, 50(4), 1029–1054. https://doi.org/10.2307/1912775
- Head, K., & Ries, J. (1996). Inter-city competition for foreign investment: Static and dynamic effects of China’s incentive areas. Journal of Urban Economics, 40(1), 38–60. https://doi.org/10.1006/juec.1996.0022
- Hill, A. D., Johnson, S. G., Greco, L. M., O’Boyle, E. H., & Walter, S. L. (2021). Endogeneity: A review and agenda for the methodology-practice divide affecting micro and macro research. Journal of Management, 47(1), 105–143. https://doi.org/10.1177/0149206320960533
- Hsu, J., & Tiao, Y.-E. (2015). Patent rights protection and foreign direct investment in Asian countries. Economic Modelling, 44, 1–6. https://doi.org/10.1016/j.econmod.2014.08.018
- Iammarino, S., & McCann, P. (2013). Multinationals and economic geography: Location, technology and innovation. Edward Elgar Publishing.
- Im, K. S., Pesaran, M. H., & Shin, Y. (2003). Testing for unit roots in heterogeneous panels. Journal of Econometrics, 115(1), 53–74. https://doi.org/10.1016/S0304-4076(03)00092-7
- Keller, W. (2010). International trade, foreign direct investment, and technology spillovers. In Handbook of the Economics of Innovation (Vol. 2, pp. 793–829): Elsevier.
- Khachoo, Q., & Sharma, R. (2016). FDI and innovation: An investigation into intra-and inter-industry effects. Global Economic Review, 45(4), 311–330. https://doi.org/10.1080/1226508X.2016.1218294
- Khoury, T. A., & Peng, M. W. (2011). Does institutional reform of intellectual property rights lead to more inbound FDI? Evidence from Latin America and the Caribbean. Journal of World Business, 46(3), 337–345. https://doi.org/10.1016/j.jwb.2010.07.015
- Kim, D.-W., Yu, J.-S., & Hassan, M. K. (2018). Financial inclusion and economic growth in OIC countries. Research in International Business and Finance, 43, 1–14. https://doi.org/10.1016/j.ribaf.2017.07.178
- Kim, Y., & Rhee, D.-E. (2019). Do stringent environmental regulations attract foreign direct investment in developing countries? Evidence on the “Race to the Top” from cross-country panel data. Emerging Markets Finance and Trade, 55(12), 2796–2808. https://doi.org/10.1080/1540496X.2018.1531240
- Lewis, J., Schneegans, S., & Straza, T. (2021). UNESCO Science Report: The race against time for smarter development (Vol. 2021). UNESCO Publishing.
- Li, B., Chang, C. P., & Zheng, M. (2021). Assessment of innovation and foreign direct investment: An investigation of OECD countries. Pacific Economic Review, 26(3), 392–403. https://doi.org/10.1111/1468-0106.12346
- Looy, B. V., Debackere, K., & Andries, P. (2003). Policies to stimulate regional innovation capabilities via university–industry collaboration: An analysis and an assessment. R&D Management, 33(2), 209–229. https://doi.org/10.1111/1467-9310.00293
- Luthra, S., & Mangla, S. K. (2018). Evaluating challenges to Industry 4.0 initiatives for supply chain sustainability in emerging economies. Process Safety and Environmental Protection, 117, 168–179. https://doi.org/10.1016/j.psep.2018.04.018
- Maddala, G. S., & Wu, S. (1999). A comparative study of unit root tests with panel data and a new simple test. Oxford Bulletin of Economics and Statistics, 61(S1), 631–652. https://doi.org/10.1111/1468-0084.0610s1631
- Minh, V. T. H., & Trinh, P. T. T. (2023). The impact of FDI on economic growth in developing countries: the role of FDI inflow and trade openness. The Economics and Finance Letters, 10(3), 216–229.
- Mueller, P. (2006). Exploring the knowledge filter: How entrepreneurship and university–industry relationships drive economic growth. Research Policy, 35(10), 1499–1508. https://doi.org/10.1016/j.respol.2006.09.023
- Narula, R., & Dunning, J. H. (2000). Industrial development, globalization and multinational enterprises: New realities for developing countries. Oxford Development Studies, 28(2), 141–167. https://doi.org/10.1080/713688313
- Nguyen, A. T., Anwar, S., Alexander, W. R. J., & Lu, S.-H. (2022). Openness to trade, foreign direct investment, and economic growth in Vietnam. Applied Economics, 54(29), 3373–3391. https://doi.org/10.1080/00036846.2021.2009112
- Nguyen, C. P., & Lee, G. S. (2021). Uncertainty, financial development, and FDI inflows: Global evidence. Economic Modelling, 99, 105473. https://doi.org/10.1016/j.econmod.2021.02.014
- Olorogun, L. A., Salami, M. A., & Bekun, F. V. (2022). Revisiting the Nexus between FDI, financial development and economic growth: Empirical evidence from Nigeria. Journal of Public Affairs, 22(3), e2561. https://doi.org/10.1002/pa.2561
- Onifade, S. T., Çevik, S., Erdoğan, S., Asongu, S., & Bekun, F. V. (2020). An empirical retrospect of the impacts of government expenditures on economic growth: New evidence from the Nigerian economy. Journal of Economic Structures, 9(1), 1–13. https://doi.org/10.1186/s40008-020-0186-7
- Pavlínek, P. (2018). Global production networks, foreign direct investment, and supplier linkages in the integrated peripheries of the automotive industry. Economic Geography, 94(2), 141–165. https://doi.org/10.1080/00130095.2017.1393313
- Rodríguez, X. A., & Pallas, J. (2008). Determinants of foreign direct investment in Spain. Applied Economics, 40(19), 2443–2450. https://doi.org/10.1080/00036840701367606
- Saini, N., & Singhania, M. (2018). Determinants of FDI in developed and developing countries: A quantitative analysis using GMM. Journal of Economic Studies, 45(2), 348–382. https://doi.org/10.1108/JES-07-2016-0138
- Sargan, J. D. (1958). The estimation of economic relationships using instrumental variables. Econometrica, 26(3), 393–415. https://doi.org/10.2307/1907619
- Sekuloska, J. D. (2015). Innovation oriented FDI as a way of improving the national competitiveness. Procedia – Social and Behavioral Sciences, 213, 37–42. https://doi.org/10.1016/j.sbspro.2015.11.400
- Shan, S., Lin, Z., Li, Y., & Zeng, Y. (2018). Attracting Chinese FDI in Africa: The role of natural resources, market size and institutional quality. Critical Perspectives on International Business, 14(2/3), 139–153. https://doi.org/10.1108/cpoib-11-2016-0055
- Solow, R. M. (1956). A contribution to the theory of economic growth. The Quarterly Journal of Economics, 70(1), 65–94. https://doi.org/10.2307/1884513
- UNCTAD. (2023). Foreign direct investment: Inward and outward flows and stock, annual. https://unctadstat.unctad.org/wds/TableViewer/tableView.aspx
- Vernon, M. M., Danley, C. M., & Yang, F. M. (2021). Developing a measure of innovation from research in higher education data. Scientometrics, 126(5), 3919–3928. https://doi.org/10.1007/s11192-021-03916-z
- Wang, S., He, Y., & Song, M. (2021). Global value chains, technological progress, and environmental pollution: Inequality towards developing countries. Journal of Environmental Management, 277, 110999. https://doi.org/10.1016/j.jenvman.2020.110999
- Wang, Y., Kang, Y., Wang, J., & Xu, L. (2017). Panel estimation for the impacts of population-related factors on CO2 emissions: A regional analysis in China. Ecological Indicators, 78, 322–330. https://doi.org/10.1016/j.ecolind.2017.03.032
- WIPO. (2021). Global Innovation Index 2021: Tracking Innovation through the COVID-19 Crisis (14th ed.). Geneva: World Intellectual Property Organization.
- Xaypanya, P., Rangkakulnuwat, P., & Paweenawat, S. W. (2015). The determinants of foreign direct investment in ASEAN: The first differencing panel data analysis. International Journal of Social Economics, 42(3), 239–250. https://doi.org/10.1108/IJSE-10-2013-0238