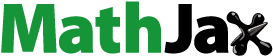
Abstract
Financial inclusion and gender equality are the key constituents of the principle of “leaving no one behind” upheld by the Sustainable Development Goals, which emphasise a holistic approach for the sustainable development of all. Financial inclusion and gender equality are interrelated factors that can influence economic growth in complex ways. In addition to their independent impact on economic growth, there is an active interaction between the two which also plays an important role in determining the level of economic growth. This interaction has been neglected by the existing literature. Based on this gap, the paper investigates the existence and type of this interaction between financial inclusion and gender equality in determining economic growth for a group of 48 developing countries for the period 2004–2019 using the Generalised Method of Moments. The study found a complementary interaction between gender equality and financial inclusion, such that improvements in financial inclusion in the presence of better gender parity have a multiplicative effect on economic growth and vice versa. The study concludes that gender equality creates various pull and push factors for better financial inclusion. Similarly, financial inclusion eliminates gender inequality in access to financial products, exacerbating the influence on economic growth both individually and collectively.
Impact statement
This study looks at the complementary role of financial inclusion and gender equality in promoting economic growth in developing countries. The study employs a panel data analysis of 48 countries from 2004 to 2019 using the Generalized Method of Moments to find a substantial complimentary relationship between financial inclusion and gender equality. The findings show that innovations in financial inclusion, combined with greater gender equality, have a multiplier effect on economic development. This study emphasizes the need to increase financial inclusion and gender equality as interconnected methods for achieving sustainable economic growth. The policy implications are considerable, implying that attempts to increase financial inclusion should be complemented by actions to improve gender equality, assuring inclusive and equitable economic growth. This work adds to the current literature by addressing a previously overlooked interaction, providing new perspectives for policymakers, economists, and development practitioners working to promote economic development in developing countries.
REVIEWING EDITOR:
SUBJECTS:
1. Introduction
Economic growth (henceforth EG) is a dynamic process determined by a multitude of variables highlighted by economists from time to time, ranging from but not limited to investment, capital formation, human capital, and technological improvements. In recent years, various studies have explicated that financial inclusion (henceforth FI) in the form of better availability and easy accessibility of formal financial products and services has a significant and positive impact on EG. Financial inclusion promotes economic growth through eradication of poverty, unemployment and inequality. It also contributes to economic growth through value creation of small business with positive spillover effects on human development indicators such as health, education and reduction of inequality and poverty (Agnello et al., Citation2012; Nanda & Kaur, Citation2016; Park & Mercado, Citation2015). Access to financial services at low and affordable cost reduces vulnerability of poor by improving their living standard (Rajan, 2009). The logic goes in the following ways: Low-cost credit advanced to low income and vulnerable groups initiates organized production activities in rural areas leading to more production of output. This value addition at ground level contributes to national output resulting in high growth at macro level. Additionally, universal access to deposit and insurance products by the excluded people raises fund in the financial market. This helps people park their savings in the financial system, and then the financial market ensures efficient allocation of these funds into long-term investment projects. In this way, financial market secures liquidity risk and encourages more investment. This process also results in more output and employment leading to improvement in the distributions of income and income of the poor. Another strand of literature has found that gender inequality in educational attainments, labour force participation rates, and wage rates has a negative impact on EG. Becker and Lewis (Citation1973) and Becker and Tomes (Citation1976) advocate that gender equality is associated with lower fertility rates and better education of children which increases the human capital embodied in the next generation and hence promotes economic growth in an endogenous growth pattern. Similar results were revealed by Lagerlöf who found that curbing gender inequality in education promotes economic growth by reducing fertility and improving children’s human capital. Klasen (Citation1999) studied the impact of gender inequality in education and employment on average annual compound growth of GDP on a sample of 109 developing countries and found that gender inequality in both dimensions is negatively associated with economic growth. Duflo (Citation2012) report that micro-credit schemes have been directed almost exclusively at women, because, it is argued, women invest the money in goods and services that improve the well-being of families. Greenwood et al. (Citation2005) report that the expansion of technology, which goes hand in hand with economic growth, results in the development of time-saving home appliances which liberate women from the constraints of performing household duties and make it possible for them to participate in growth promoting economic activities.
Drawing from both these factions of literature, this study is an attempt to investigate the interactionary relationship between gender equality (henceforth GEQ) and FI in affecting economic growth. That is, whether the presence of GEQ is essential for better impact of FI on EG. This is essentially because gender inequality in various dimensions determines the extent and inclusiveness of FI (Ghosh & Vinod, Citation2017; Mndolwa & Alhassan, Citation2020), which could weaken the effect of FI on EG. This is because financial exclusion of women exacerbates gender inequality in education, health and employment by limiting women’s access to financial services such as credit, savings and insurance as well as impeding them from taking advantage of market opportunities (Marlow & Patton, Citation2005). Without access to financial resources, women may struggle to start a business or built assets which further perpetuates the cycle of gender inequality. Having said this in background, the present study aims to empirically examine the type of relationship between FI and GEQ vis-à-vis EG. The model hypothesises that FI and GEQ could work either as substitutes or complements in determining EG. If FI and GEQ complement each other, it means that better gender parity via various demand and supply channels magnify the impact of FI on EG. In this case an optimal policy strategy would focus on the promotion of both GEQ and FI for better growth results. On the other hand, if FI and GEQ work as substitutes, then the impact of FI and GEQ on EG are independent of one another. In this case optimal policies in each would be independent of the other. The existing literature on the relationship between gender equality and financial development/inclusion, although highlighting some important dimensions, has neglected their interactionary role in determining economic growth. For example, using data from Botric and Broz (Citation2017) investigated the determinants of gender inequality in financial inclusion in Central and South East European countries. Similarly, using the same database, Antonijević et al. (2022) also revealed significant gender differences in seven indicators of financial inclusion in a sample of 144 developing and developed countries. Cabeza-García et al. (Citation2019) study the impact of female financial inclusion and overall financial inclusion on economic growth, respectively. Others such as Morsy (Citation2020) associate gender inequality in financial inclusion with institutional and regulatory framework. The existing research on EG has extensively explored the impact of FI and GEQ on EG (Haini, Citation2021; Hill & King, Citation1995; Kabeer & Natali, Citation2013; Klasen & Lamanna, Citation2009; Nanda & Kaur, Citation2016; Park & Mercado, Citation2015; Pervaiz et al., Citation2011; Sethi & Acharya, Citation2018; Summers, Citation1994). These studies although being important completely ignore the gender-finance interaction in determining economic growth. Building on this research gap, following the work of Brambor et al. (Citation2006) on conditional hypothesis, we introduce a multiplicative interaction term between FI and GEQ in our regression model to analyse the growth impact of FI contingent upon the level of GEQ. The importance of studying this relationship is justified by the fact that one of the sustainable development goals (SDG-5- ‘achieve GEQ and empower all women and girls’) of the United Nations exclusively relates to GEQ as an essential pre-condition of sustained and inclusive development. In addition to this, seven out of seventeen SDGsFootnote1 emphasise the importance of FI in reducing poverty, gender inequality and promoting economic development.
2. Literature review
2.1. Financial development and economic growth
The traces of the debate over the possible linkages between finance and EG can be found in the classical works of Bagehot (Citation1873), Hicks (Citation1969) and Patrick (Citation1966). Schumpeter (Citation1911) describes how well-functioning banks foster technical innovation by identifying and funding entrepreneurs with the greatest potential for successfully implementing novel goods and manufacturing processes. In contrast, Robinson (Citation1952) asserts that "where enterprise leads, finance follows." According to this theory, economic development generates demand for specific forms of financial arrangements, and the financial system responds naturally to these demands. By bridging the gap between those with an excess of funds and those with a deficiency of funds, well-functioning and inclusive financial systems play a crucial role in allocating resources to highly productive investments. This enhances the overall resource efficiency, which generates greater EG. The empirical analysis of the relationship between FI (financial development in a broader sense) and EG has increased in recent years. With the exception of a few studies, the majority of empirical investigations, whether time series studies involving a specific country or panel studies involving a cross-country sample, demonstrate a significant positive association between access to finance and EG. Levine and King (Citation1993) conducted an empirical study on a panel of 80 countries over the period 1960–1989, controlling for a multitude of other factors affecting EG. Their findings indicate that the initial stage of financial development is a reliable predictor of future EG rates. Similarly, Sahay et al. (Citation2015) found that expanding access to a variety of banking services for individuals and businesses, as well as increasing the number of women who use these services, has a substantial positive impact on EG. Sethi and Acharya (Citation2018) used Pedroni Panel cointegration test, FMOLS and Panel VECM causality to investigate the long-run causality between FI and EG across a panel of 31 countries over the period 2004–2010. The empirical findings confirm a long-run relationship between FI and EG across all cross-sections. The estimates also revealed a bi-directional causal relationship between FI and EG. FI contributes to EG by allowing small businesses to create value, which has a beneficial impact on human development indicators such as poverty, health and education (Nanda & Kaur, Citation2016; Park & Mercado, Citation2015). Sharma (Citation2015) examined the impact of FI on EG for the period 2004 to 2013 covering Indian economy. The authors employed vector auto-regression (VAR) models and Granger causality to test the main research question. Empirical results revealed that there is a positive association between EG and various dimensions of FI, specifically banking penetration, availability of banking services and usage of banking services in terms of deposits. Granger causality analysis reveals a bi-directional causality between geographic outreach and economic development and a unidirectional causality between the number of deposits/loan accounts and gross domestic product. Haini (Citation2021) examined the impact of financial institutions access and financial institutions depth on economic growth in 51 low- and lower–middle-income countries from 1996 to 2017.The authors employed generalised least squares model for estimation of regression coefficients. The results showed that both financial access and financial depth are positively corelated to economic growth. However, the marginal impact of financial depth is negative at low levels of financial access, while the finance–growth relationship becomes positive at higher levels of financial access. Results suggest the importance of developing inclusive financial systems that emphasise quality rather than quantity to promote economic growth. Nandi et al. (Citation2021) investigated the relationship between financial development and growth of GDP per capita in 76 developing-countries between 2011 and 2017, using the Granger causality test and Pedroni and Westerlund cointegration tests. The findings suggest that developing nations with high levels of FI will experience robust EG. However, the positive effects for two subsample nations (those with low and moderate levels of FI) are diverse. Ohiomu and Ogbeide-Osaretin (Citation2020) Using GMM estimation, investigated the relationship between gender inequality and FI in several sub-Saharan African nations. The study concluded that FI significantly reduced gender inequality. Accessibility to financial products and services has a greater impact on reducing gender inequality than usage of financial products and services, according to the study.
2.2. Gender inequality and economic growth
Promoting GEQ is important not only from a social, humanistic, or ethical point of view but also from an economic point of view as well. Correcting gender inequality in one or more dimensions has been a focus of the international community in all of its development plans since 1990, starting from the International Development Goals (IDGs) of the Development Assistance Committee (DAC) through the global promise of the Millennium Development Goals (MDGs) to the more recent and much more inclusive Sustainable Development Goals (SDGs). Despite these efforts, gender inequality in varying dimensions remains a huge challenge in the world. There is a vast body of literature that elucidates various direct and indirect means through which gender inequality affects growth. Klasen (Citation1999) using two stage least squares regression (2SLS) in the study of 109 industrialised and developing countries, explicates that if talents are uniformly distributed within gender, then gender inequality in education directly amounts to an artificial market distortion on the available pool of human capital. Among the indirect channels, studies have found that GEQ in education leads to reductions in fertility rates and child mortality, both of which ensure better quality human capital in the next generation (Hill & King, Citation1995; Murthi et al., Citation1995; Pervaiz et al., Citation2011; Summers, Citation1994). Volart (Citation2000) evaluates the association between the ratios of female to male enrolment rates on real GDP growth using data from 87 countries for the period 1965–1989. Employing dummy variable regression, her study concludes that both increases in education and reductions in gender inequality increase growth. McGillivray and Baliamoune-Lutz (Citation2007) also found a negative and statistically significant relationship between GDP growth and gender inequality in primary and secondary school enrollment and literacy rates. However, some earlier cross-country studies, such as Barro and Lee (Citation1994) and Barro et al. (Citation1995), who found a negative impact of female education on EG, were later challenged on methodological grounds for not accounting for multicollinearity and endogeneity (Forbes, Citation2000; Klasen, Citation1999; Klasen & Lamanna, Citation2009; Lorgelly & Owen, Citation1999). In case of the relationship between gender inequality in labour markets and EG, Kabeer and Natali (Citation2013) report that first, there is scarcity of literature on this relationship, and second, the results of whatever studies there are, point in different directions, owing to the lack of comparable cross-country data and the difficulty in dealing with the problems of unobserved heterogeneity and endogeneity. In his study Klasen (Citation1999), cited earlier, found that although the impact of the female share of formal employment on growth was significantly large and positive, nevertheless, it became statistically insignificant. Volart (Citation2004), in her study of 16 Indian states found that gender inequality in labour markets in terms of female to male composition of the labour force and female to male share in managerial positions had a positive and significant impact on total output per capita. As far as the impact of gender wage inequality on EG is concerned, Seguino (Citation2000a) and Seguino (Citation2000b) using least square dummy variables (LSDV) regression deduced that gender wage inequality in the manufacturing sector had a positive and significant impact on growth by giving a cost advantage in investment and exports; this however was true only in the case of semi-industrialized economies (SIEs). In the case of agricultural economies, GEQ rather than inequality was supposed to promote growth. However, this hypothesis was not empirically tested due to the absence of appropriate data (Seguino et al., Citation2010). This positive association between gender wage inequality and enhanced growth via exports was also confirmed by Busse and Spielmann (Citation2006). Schober and Winter-Ebmer (Citation2009) replicated the study of Seguino (Citation2000a) using more reliable data and found that the impact of the gender wage gap either confirmed the negative sign or became zero.
As revealed by the literature review above, the existing literature on EG completely neglects the role of interaction between GEQ and FI as a potential determinant of EG. This means that a statistically significant interaction coefficient would imply omitted variable bias in the previous studies as well as question the authenticity of their policy relevance. The current study aims to address this potential gap in the existing literature and make necessary policy recommendations. Accordingly, we propose the following research hypothesis to be empirically tested based on the data on a group of 48 developing countries for the period 2004–2019 using the Generalised Method of Moments:
H0: The interaction effect between FI and GEQ is insignificant or the level of GEQ does not affect the impact of FI in determining EG.
H1: The level of GEQ does affect the impact of FI in determining EG.
3. The empirical model and the estimation method
The study is based on a panel analysis of 48 developing economies for the time period 2005–2019. The primary goal of this empirical work is to examine the impact of the interaction between FI and GEQ on EG in these countries. We start our analysis by estimating a panel model specified as follows.
(1)
(1)
Since EG, measured by GDP per capita, has a certain amount of inertia, its lag is also considered a regressor. FII and GEI are measures of FI and GEQ, respectively. The GEQ Index (GEI) is measured by (1-GII), where GII is the Gender Inequality Index. GEI ranges from 0 to 1, with higher values corresponding to better gender parity. The variable () represents the interaction effect between FI and GEQ. Following earlier methodologies, we included a vector
of four additional control variables, namely trade openness (TRADE), inflation (INF) and Gross capital formation (GCF) and Government expenditure. The coefficient of trade is expected to exhibit a positive sign (Liu et al., Citation2009) while inflation is expected to have a negative effect on EG (Bruno & Easterly, Citation1998). Similarly, the effect of gross capital formation and government expenditure on EG is expected to be positive (Uneze, Citation2013). The coefficients
and
are the parameters to be evaluated. A statistically significant and positive sign for
means that higher levels of FI are conducive to EG. Similarly, a positive sign for
will mean that higher levels of GEQ are associated with higher EG. For the interaction term a statistically significant positive sign for
reveals that the FI and GEQ are complements i.e. FI promotes GEQ, and together (interaction term) they produce a positive effect on EG. Conversely, a negative sign for
means that the GEQ-enhancing effects of FI are smaller in countries where GEQ is already high than in countries with lower GEQ. This means that finance and GEQ substitute for each other’s effects. The presence of a statistically insignificant
however, means that the impact of FI on GEQ is independent of the level of FI. For illustration purposes, the coefficients of the primary variables of importance, as well as their signs and meanings, are shown in . It is crucial to note that if the interaction between FI and GEQ is statistically significant, then any model of EG that neglects the interaction may be mis-specified and may lead to omitted variable bias.
Table 1. Direction and meaning of the coefficients of variables of interest.
Note that since FI is a multidimensional concept and has a number of sub-indices like financial availability, financial accessibility, and financial usage, putting it all into a single index means losing some important information about the impact of the individual components. Based on this, we have run four different models corresponding to every dimension of FI. Model 1 measures FI on the basis of financial access, measured by the number of ATMs available per 100,000 adults. Model 2 measures FI on the basis of availability of financial services, using the number of bank branches per 100,000 of population as its proxy. Model 3 measures FI on the basis of financial usage, using bank deposits to GDP ratio as a proxy for financial usage. Model 4 is an extension of Model 3, which is based on another proxy of financial usage, i.e., the credit-deposit ratio. The selection of these proxies, corresponding to every dimension of FI, is backed by the empirical literature (Kodan & Chhikara, Citation2011; Maity & Sahu, Citation2022).
3.1. Methodology
The study investigates the following dynamic panel model equation:
(2)
(2)
Where represents the dependent variable for country i in year t,
is the country-specific fixed effect,
represents the coefficient of autoregressive dependent variable.
is the independent variable and its lag
also used as a regressor with
&
as their corresponding coefficients.
Is a vector of other exogenous variables, and
is the random error.
3.1.1. Panel unit-root tests
If the series exhibits the behaviour of random walk or is close to a unit root process, it will be impossible to identify the parameters or the parameters will be very weak. The employment of the first-differenced GMM estimator can, therefore, lead to substantial finite-sample biases (Blundell & Bond, Citation1998). In series with unit root, the typical orthogonality criteria of the first-differenced GMM estimator fail to hold (Lee & Azali, Citation2009). Therefore, unit root tests must be carried out on the series before any further analysis can begin. Levin, Lin, & Chu devised a panel unit root test, often known as the LLC, which examines the null hypothesis of non-stationarity or the presence of unit root. When doing panel unit root tests, the Augmented Dickey-Fuller (ADF) test is used to ensure that the series is stationary. As an example, LLC looks at the following ADF specification:
(5)
(5)
Here y represents the variable to be tested for the presence of unit-root, t = [1, T) represents the time periods, and i = [1, N) denotes the cross sectional units of the panel. The lag lengths are determined on the basis of three different information criteria: Akaike, Schwarz, and Hannan-Quinn. The are assumed to be same across all the members of panel. The null hypothesis under the LLC test assumes that panel is non stationarity i.e.
=0 for all i. The alternative hypothesis can be formulated as
for all cross-sectional units showing the panel data stationarity.
3.1.2. The generalized method of moments (GMM) estimation
Although panel data offers many advantages over the cross sectional or time series data in terms of more degrees of freedom and reduced collinearity among regressors thus improving overall efficiency of econometric estimates, using panel data may also bring in problems like heteroscedasticity and autocorrelation owing to heterogeneity in the characteristics of different cross sectional units in the model. Moreover, due to autoregressive nature of dependent variable the problem of endogeneity also creeps in when the lagged value of the dependent variable used as a regressor gets correlated with lagged error term. The estimation of such dynamic panel data models using OLS will result in biased and inconsistent estimators.
Since EG necessarily depends upon its past values, this autoregressive nature creates the problem of endogeneity in the process of removing time invariant cross sectional heterogeneity as shown in EquationEquation (3)(3)
(3) below. The simultaneous presence of the first lag of dependent variable (
and the error term (
on the right side of the equation cause endogeneity hence neither fixed nor random effects model can be used.
(3)
(3)
(4)
(4)
To overcome these problems, Arellano and Bond (Citation1991) developed the Generalized Method of Moments for the estimation of dynamic panels. This technique runs a system of two equations, one in levels and the other in first-differences. Estimators employ IV instruments, or 'internal’ instruments for the two equations, by using the lagged values of the explanatory variables in both levels and first differences. GMM estimator effectively adjusts for unobserved country-specific effects and reduces measurement error. In addition, system GMM yields consistent parameter estimates when the right-hand side of the equation contains a lagged dependent variable which the within estimate is unable to do. The instruments employed in the estimating method must meet the population moment criteria. The Hansen test of over-identifying limitations can be used to test this assumption empirically. The null hypothesis supporting the instrumental validity should not be rejected in this case. To get reliable GMM estimates, it is necessary that the error term must display no serial correlation greater than order 1, which is an extra requirement that must be met. The Arellano and Bond test can be used to examine this hypothesis.
3.1.3. System GMM
First-difference GMM estimation cannot be employed if the panel unit root tests indicate signs of non-stationarity in the levels series. The system GMM estimator, introduced by Blundell and Bond (Citation1998), makes use of extra moment limits to reduce the downward bias induced by processes with near unit roots. According to the system GMM estimator, the bias is greatly reduced by utilising ‘acceptable stationary limitations on the initial condition processes’ (Lee & Azali, Citation2009). The system GMM estimation employs a set of equations in first differences with adequately lagged levels as instruments, with another set of levels equations with adequately lagged first differences as instruments. In a system with both first-differenced and level equations, the GMM estimator uses a linear GMM estimator to merge the two sets of moment conditions (Lee & Azali, Citation2009). For this analysis, we will employ both levels and differences of all variables of interest, as well as lagged levels, following Blundell and Bond (Citation1998) paradigm if unit roots are found in the series.
To check the overall validity of the instrumental variables used in the regression analysis, Arellano and Bond (Citation1991) and Arellano and Bover (Citation1995) suggested two specification tests. The Sargan test of over-identifying restrictions is used to check the endogeneity of the instrumental variables. The null hypothesis under the test is that instrumental variables used are not correlated with error terms and are thus strictly exogenous. Failure to reject the null hypothesis implies that there is no correlation between an instrument and an error term, which means instrumental variable estimations based on the instruments chosen are valid. The second specification test checks the hypothesis of first and second order autocorrelation of the differenced error terms. Under the null hypothesis of no serial autocorrelation between the differenced error terms in an ideal model, the hypothesis related to AR (1) should be rejected, while that of AR (2) should be retained.
3.2. Data
provides the definition and sources of each variable used in the empirical estimation of the model.
Table 2. Definition and source of variables.
3.2.1. Dependent variable
The real GDP per capita (GDP) is used as the dependent variable in this empirical study. The data were extracted from the World Development Indicators (WDI) published by the World Bank.
3.2.2. Explanatory variables
Financial inclusion: In accordance with the standard definition of FI, three broad measures of FI covering all the three dimensions i.e. accessibility, availability, and usage of formal financial services are employed. The accessibility of financial services is measured by the number of Automated Teller Machines (ATM) per 100,000 adults. Availability of financial services is captured by the number of bank branches per 100,000 adults and usage of financial services is gauged by the volume of bank credit and deposits as a percentage of GDP by commercial banks. The data for these variables was derived from the World Development Indicators (World Bank) and the Global Findex Database. Since an improvement in the level of FI promotes EG, the coefficient of FII is expected to be positive.
Gender equality: GEQ has been measured by subtracting the value of the Gender Inequality Index (GII) from 1. GII has been developed by the United Nations Development Programme (UNDP). It is a composite index that measures gender inequality in three important dimensions: (1) Health, comprising Maternal Mortality Rate and Adolescent Birth Rate. (2) Empowerment is composed of female and male population with at least secondary education and female and male shares of parliamentary seats. (3) Labour markets which account for female and male labour force participation rate. The value of the final index ranges from 0 to 1, where 0 represents perfect GEQ and 1 represents perfect gender inequality in the underlying indicators. Its data has been extracted from the UNDP database on gender inequality.
Control variables: Trade openness, inflation, gross capital formation, and government final consumption expenditure have been used as the control variables. The data on these variables was obtained from the World Bank’s World Development Indicators database.
4. Results and discussion
4.1. Descriptive statistics and correlation matrix
presents descriptive statistics for various economic indicators across a dataset of 768 observations. The variables include metrics such as ATMs per 100,000 adults, bank branches per 100,000 adults, bank credit to bank deposits as a percentage of GDP, bank deposits to GDP percentage, GDP per capita in constant 2015 US dollars, the Gender Inequality Index, government final consumption expenditure, gross fixed capital formation as a percentage of GDP, inflation rates (consumer prices annual percentage change), and trade as a percentage of GDP. The mean, standard deviation, minimum, and maximum values are provided for each variable, offering insights into their central tendencies, variability, and range. The data reveals a spectrum of financial and economic characteristics, encompassing accessibility to banking services, economic output, gender inequality, government spending, investment patterns, inflation trends, and trade openness within the analysed dataset.
Table 3. Descriptive statistics.
presents a matrix of correlations among different variables. Each row and column correspond to a specific variable, such as ATM (ATMs per 100,000 of adults), BBR (bank branches per 100,000 of adults), CDR (bank credit to bank deposits as a percentage of GDP), BDPO (bank deposits to GDP percentage), GDP (GDP per capita), GII (Gender Inequality Index), GOVT (government final consumption expenditure), GFC (gross fixed capital formation as a percentage of GDP), INF (inflation, consumer prices annual percentage), and TRADE (trade as a percentage of GDP). The correlation values range from -1 to 1, where 1 indicates a perfect positive correlation, -1 indicates a perfect negative correlation, and 0 indicates no correlation. Positive correlations suggest variables moving in the same direction, while negative correlations indicate variables moving in opposite directions. For example, ATM and GDP have a positive correlation of 0.711, implying that higher ATM availability is associated with higher GDP per capita. Conversely, GII and ATM have a negative correlation of -0.598, indicating that regions with higher gender inequality tend to have fewer ATMs per 100,000 adults.
Table 4. Matrix of correlations.
4.2. Panel unit root results
presents the LLC panel unit root test results with trend and intercept at both levels and the first difference. Since not all of our variables are stationary at levels, the first-difference GMM based on standard orthogonality conditions cannot provide efficient estimation due to downward bias in finite samples, which paves the way for the use of a system GMM in the estimation of the model.
Table 5. LLC Unit root tests (akaike information criteria).
4.3. GMM estimation results
presents the results of such estimation for GDP per capita as a measure of EG. The coefficient of the first lag of GDP is significant and less than 1 which approves the structural stability of the models. For Model 1 which measures FI by the demographic penetration of ATMs, the Coefficient of FII is -0.003. For model 2, measuring availability of financial services by geographic penetration of banking services, the coefficient is .006 and for models 3 and 4, which measure the usage of financial services by deposits and credit usage, the coefficients are 0.004 and 0.255, respectively. Therefore it can be concluded from the results that FI produces pro-growth effects through all of its sub-components. These results are in line with those of Inoue and Hamori (Citation2016). This happens because FI increases the supply of loanable funds in the financial markets by mobilising untapped idle financial resources from hitherto unbanked populations, improves allocative efficiency in the distribution of financial services and helps entrepreneurs to avail credit facilities to start their own enterprises which collectively exert a profound impact on EG. Similarly, GEQ in all models (Model 1 to 4) bears a significant and positive relationship with EG. The corresponding coefficients in Models 1, 2, 3 and 4 are 0.95, 2.15, 2.67, and 0.49, respectively, which means that higher equality between men and women serves as an engine of EG (Hill & King, Citation1995; Lorgelly & Owen, Citation1999). This might happen through a number of channels. A highly functional, educated and healthy female labour force contributes to EG through their labour and human capital. Higher GEQ also leads to a reduction in the fertility rates (Klasen & Lamanna, Citation2009) which increases the education of children and hence future embodied growth. Also a higher score on gender inequality index means that a country is forgoing some growth by keeping the large female population uneducated, unhealthy, and unrepresented in the political sphere and out of the labour force. Regarding the interaction effect between FI and GEQ vis-a-vis EG, the empirical estimates lend credence to the existence of complementary effects between the two. The coefficients for all models are positive and statistically significant. This happens because as the participation of women in economic activities increases, it creates both demand pull and supply push factors. On the demand side, increased economic participation of women would result in higher demand for financial products and services and on the supply side a higher pool of financial funds would enable the financial system to reap the necessary economies of scale that could further reduce the cost of financial services to those outside the ambit of the formal financial system.
Table 6. System GMM results.
Among the control variables used in the study, the coefficient on trade is positive and significant, revealing the importance of international trade in EG. Economically speaking, one unit hike in trade increases EG by 0.007%, 0.001%, 0.001%, and 0.0025% for models I, 2, 3, and 4, respectively. These results validate the classical and neoclassical doctrine that international trade serves as the ‘Engine of EG’. International trade via increased specialisation in the production of goods and services improves efficiency in the allocation of resources among various productive ends. Also, trade helps to reap the static and dynamic gains of economies of large scale production. These findings are in line with the studies of Liu et al. (Citation2009) and Uneze (Citation2013), who also reported the positive impact of international trade on EG. Similarly, the coefficient of inflation is negative and statistically significant for all models. More specifically, a unit hike in inflation reduces EG by -0.0009%, -0.001%, 0.002%, and 0.004% in models 1, 2, 3, and 4, respectively. This is because inflation erodes the real value of cash balances, affects consumption and investment decisions, and creates uncertainty in the economy, having a detrimental impact on EG. These findings are in tandem with the results of Bruno and Easterly (Citation1998); Adaramola & Dada who also reported the deleterious effects of inflation on EG. Moreover, gross capital formation has a coefficient that is both positive and significant. This means that more capital formation is good for EG. The specific coefficients are 0.05%, 0.03%, 0.045%, and 0.015% for models 1, 2, 3, and 4, respectively. Higher capital formation by increasing the availability of capital per worker in capital scarce developing economies significantly improves productivity of labour and consequently EG. These findings are in line with that of Uneze (Citation2013) and Adhikary (Citation2010) who also found a positive link between investment rates and higher EG. However, government expenditure exhibited a negative impact on EG. An increase in government expenditure is found to reduce EG by 0.015%, 0.022%, and 0.013% for models 1, 2, and 3, respectively. According to Easterly and Rebelo (Citation1993) this is largely due to the prevalence of unproductive government expenditure in developing countries. This is also because government expenditure, as used here by definition includes expenditure on national defence and security and excludes military expenditures that are a part of government capital formation.
5. Conclusion
In the extant literature on EG, a plethora of work has been done on the effects of FI and GEQ on EG. These studies have generally provided evidence in favour of pro-growth effects from increased access to finance as well as from greater gender parity. Notwithstanding these results, the interaction effect between FI and GEQ has been left out. The goal of this paper is consequently to reassess the causal link between GEQ, FI, and EG for the set of chosen developing economies while allowing an interaction effect between FI and GEQ, which we believe may be responsible for omitted variable bias.
We have applied the endogeneity expunging technique of the system GMM for the empirical estimation of the underlying panel model. The main results of the study can be summarised as follows: First, we find that all measures of FI except ATMs have a positive and statistically significant impact on EG. Secondly, the empirical results also revealed that better gender parity produces significant pro-growth effects. However, the interaction of FI and GEQ is found to have a positive and statistically significant impact on EG. This means that the pro-growth effect of GEQ is stronger in countries with higher levels of FI and weaker in countries with higher levels of financial exclusion. The same is affirmed by the following arguments:
Firstly, improved gender parity would create both demand-side and supply-side push factors for FI. On the demand side, the increased participation of women in economic activities on par with men would mean more demand for financial products and services. On the supply side, this would enable the financial system to reap enough economies of scale in providing credit and insurance services to the population, especially the vulnerable ones. This would enhance EG as better gender parity would lead to the inclusion of women in income-generating activities, which would necessarily promote growth.
Secondly, since some of the disparities between men and women are with respect to their use of financial products, FI also complements gender parity by correcting the financial deprivations of the population, including women. This is primarily because financial access provides women with income-generating capabilities, which clearly provides favourable avenues for increased economic participation of socially excluded groups, including women. Hence, an all-inclusive financial system would promote growth directly as well as via positive spillovers, especially in correcting the financial dichotomy between men and women.
The modern development literature supports the relevance of financial access to inclusive development. In terms of policy implications, our results indicate that higher growth rates are obtained from allocating resources to both the building of financial infrastructure and promoting policies targeting greater gender parity. This is particularly true in countries where gender inequality is rampant and a large fraction of women are unbanked and outside the ambit of the formal financial system. A proper allocation of funds between building an inclusive financial system and improving gender parity is then a key to sustain EG.
Finally, although the study adds a new dimension into the relationship between economic growth, financial inclusion and gender equality, the study is not free from limitations which open way for future research in this direction. Firstly, the study is based only on 48 developing countries due to the restrictions imposed by data availability. The future research could expand the study to a wider set of countries controlling for the effects of human capital, infrastructure, institutional quality, inequality and other economic variables. Secondly, the study uses gender inequality index to measure gender inequality while as the future research could use factor analysis to construct a measure of gender inequality based on a more diverse set of variables. Lastly, our measures of financial inclusion are solely bank based and completely ignore the importance of financial markets in determining financial development.
Correction Statement
This article has been corrected with minor changes. These changes do not impact the academic content of the article.
Appendix 1.docx
Download MS Word (12.5 KB)Disclosure statement
No potential conflict of interest was reported by the author(s).
Additional information
Notes on contributors
Inayat Ullah Wani
Inayat Ullah Wani is a Senior Research Fellow (SRF) at the Department of Economics, Aligarh Muslim University, Aligarh, India. He completed his Master’s in Economics from the University of Kashmir, Srinagar, India. His areas of research interest include gender inequality, economic growth, environmental economics, financial development and applied econometrics. His research is based on time series and panel data analysis using numerous econometric techniques. He has published many research articles in top-quartile journals.
Majed Alharthi
Dr. Majed Alharthi is an Assistant Professor in Economics and Finance at King Abdulaziz University, College of Business, Rabigh, Saudi Arabia. His research interests are sustainability, economics, energy, environment and finance. Moreover, Dr. Alharthi is reviewing papers for some high-ranked journals. Dr. Alharthi is a distinguished researcher with papers published in well-reputed SSCI and SCI journals.
Ishfaq Nazir Khanday
Ishfaq Nazir Khanday is a Senior Research Fellow (SRF) in the Department of Economics at Aligarh Muslim University, Aligarh, India. He holds a Master’s in Economics from the University of Kashmir, Srinagar, India. His research interests encompass financial inclusion, poverty, environmental economics, financial development, and applied econometrics. He specialises in both primary and secondary data analysis. He has authored several research articles published in top-quartile journals.
Mohammad Subhan
Mohammad Subhan Department of Commerce, Aligarh Muslim University, Aligarh, India. Mohammad Subhan is currently pursuing a PhD from the Department of Commerce at Aligarh Muslim University, Aligarh, India. His areas of research are energy economics, financial management and international trade. He completed his bachelor’s and master’s degree from Aligarh Muslim University. He published more than 15 research papers in reputed indexed journals. He has also attended and presented at four conferences, seminars, and symposiums. His expertise in secondary data analysis techniques mainly includes the NARDL, ARDL, wavelet coherence analysis, and other econometrics models.
Mamdouh Abdulaziz Saleh Al-Faryan
Mamdouh Abdulaziz Saleh Al-Faryan is a Board Member and Head of the Scientific Committee at The Saudi Economic Association. Mamdouh is a member of more than forty national and international professional associations. He has worked tirelessly to expand his experience and financial acumen, which would guide him down the path into leadership and higher level of responsibility. So far, he has several publications in the field of economics, finance, corporate governance, and accounting, which have been published in top international journals. Mamdouh also serves as a reviewer for a number of international journals and holds academic and research distinctions.
Notes
1 Sustainable Development Goals 1, 2, 5, 8,9,10 and 17 enshrined in targets 1.4, 2.3, 5.6B, 8.3, 9.3, 10.5 and 17.16 respectively.
References
- Adhikary, B. K. (2010). FDI, trade openness, capital formation, and economic growth in Bangladesh: A linkage analysis. International Journal of Business and Management, 6(1), 16. https://doi.org/10.5539/ijbm.v6n1p16
- Agnello, L., Mallick, S. K., & Sousa, R. M. (2012). Financial reforms and income inequality. Economics Letters, 116(3), 583–587. https://doi.org/10.1016/j.econlet.2012.06.005
- Arellano, M., & Bond, S. (1991). Some tests for specification for panel data: Monte Carlo evidence and an application to employment equations. Review of Economic Studies, 277–297.
- Arellano, M., & Bover, O. (1995). Another look at the instrumental variable estimation of erro- component models. Journal of Econometrics, 68(1), 29–51.): https://doi.org/10.1016/0304-4076(94)01642-D
- Bagehot, W. (1873). A description of the money market. London: HS King.
- Barro, R., & Lee, J. W. (1994). Sources of economic growth. Carnegie-Rochester Conference Series on Public Policy, 40, 1–46. https://doi.org/10.1016/0167-2231(94)90002-7
- Barro, R., Sala, X., & Martin, I (1995). Economic Growth. McGraw-Hill.
- Becker, Gary S., &Lewis, H. Gregg. (1973). On the Interaction between the Quantity and Quality of Children. Journal of Political Economy, 81(part 2)
- Becker, G. S., &Tomes, N. (1976). Child Endowments and the quantity and quality of children. Journal of Political Economy, 84(4, Part 2), S143–S162.
- Blundell, R. W., & Bond, S. (1998). Initial conditions and moments restrictions in dynamic panel data models. Journal of Econometrics, 87(1), 115–143. https://doi.org/10.1016/S0304-4076(98)00009-8
- Botric, V., & Broz, T. (2017). Gender differences in financial inclusion: Central and South Eastern Europe. South-Eastern Europe Journal of Economics, 15(2)
- Brambor, T.,Clark, W. R., &Golder, M. (2006). Understanding interaction models: Improving empirical analyses. Political Analysis, 14(1), 63–82. https://doi.org/10.1093/pan/mpi014
- Bruno, M., & Easterly, W. (1998). Inflation crises and Long-run growth. Journal of Monetary Economics, 41(1), 3–26. https://doi.org/10.1016/S0304-3932(97)00063-9
- Busse, M., & Spielmann, C. (2006). Gender Inequality and Trade. Review of International Economics, 14(3), 362–379. https://doi.org/10.1111/j.1467-9396.2006.00589.x
- Cabeza-García, L.,Del Brio, E. B., &Oscanoa-Victorio, M. L. (2019). Female financial inclusion and its impacts on inclusive economic development. Women's Studies International Forum, 77, 102300. https://doi.org/10.1016/j.wsif.2019.102300
- Duflo, E. (2012). Women empowerment and economic development. Journal of Economic Literature, 50(4), 1051–1079. https://doi.org/10.1257/jel.50.4.1051
- Easterly, W., & Rebelo, S. (1993). Fiscal policy and economic growth. Journal of Monetary Economics, 32(3), 417–458. https://doi.org/10.1016/0304-3932(93)90025-B
- Forbes, K. (2000). A reassessment of the relationship between inequality and growth. American Economic Review, 90(4), 869–887. https://doi.org/10.1257/aer.90.4.869
- Ghosh, S., & Vinod, D. (2017). What constrains financial inclusion for women? Evidence from Indian micro data. World Development, 92, 60–81. https://doi.org/10.1016/j.worlddev.2016.11.011
- Greenwood, J.,Seshadri, A., &Yorukoglu, M. (2005). Engines of liberation. The Review of Economic Studies, 72(1), 109–133. https://doi.org/10.1111/0034-6527.00326
- Haini, H. (2021). Financial access and the finance-growth nexus: Evidence from developing economics. International Journal of Social Economics, 48(5), 693–708. (Emerald Publishing Limited) https://doi.org/10.1108/IJSE-08-2020-0549
- Hicks, J. (1969). Automatists, Hawtreyans, and Keynesians. Journal of Money, Credit and Banking, 1(3), 307. https://doi.org/10.2307/1991183
- Hill, M. A., & King, E. (1995). Women’s education and economic well-being. Feminist Economics, 1(2), 21–46. https://doi.org/10.1080/714042230
- Inoue, T., & Hamori, S. (2016). Financial access and economic growth: ecidence from Sub-saharan Africa. Emerging Markets Finance and Trade, 52(3), 743–753. https://doi.org/10.1080/1540496X.2016.1116282
- Kabeer, N., & Natali, L. (2013). Gender equality and economic growth: Is there a win-win. Institute of Development Studies Working Paper,
- Klasen, S. (1999). Does gender inequality reduce growth and development? Evidence from cross-country regressions. Policy Research Report on Gender and Development.
- Klasen, S., & Lamanna, F. (2009). The impact of gender inequality in education and employment on economic growth: New evidence for a panel of countries. Feminist Economics, 15(3), 91–132. https://doi.org/10.1080/13545700902893106
- Kodan, A. S., & Chhikara, K. S. (2011). Status of financial inclusion in Haryana: an evidence of commercial banks. Management and Labour Studies, 36(3), 247–267. https://doi.org/10.1177/0258042X1103600303
- Lee, G. H. Y., & Azali, M. (2009). The endogeneity of the Optimum Currency Area Criteria in East Asia. Economic Modelling, 27(1), 165–170. (elsevier.com). https://doi.org/10.1016/j.econmod.2009.08.004
- Levine, R., & King, R. G. (1993). Finance and growth: Schumpeter might be right. The Quarterly Journal of Economics, 108(3), 717–737. https://doi.org/10.2307/2118406
- Liu, X., Shu, C., & Sinclair, P. (2009). Trade, foreign direct investment and economic growth in Asian economies. Applied Economics, 41(13), 1603–1612. https://doi.org/10.1080/00036840701579176
- Lorgelly, P. K., & Owen, P. D. (1999). The effect of female and male schooling on economic growth in the Barro-Lee model. Empirical Economics, 24(3), 537–557. https://doi.org/10.1007/s001810050071
- Maity, S., & Sahu, T. N. (2022). Financial inclusion in north-eastern region: An investigation in the state of Assam. Vilakshan-XIMB Journal of Management, 19(2), 206–221.
- Marlow, S., &Patton, D. (2005). All credit to men? Entrepreneurship, finance, and gender. Entrepreneurship Theory and Practice, 29(6), 717–735. https://doi.org/10.1111/j.1540-6520.2005.00105.x
- McGillivray, M., & Baliamoune-Lutz, M. (2007). "Gender Inequality and Growth in Sub-Saharan African and Arab Countries." ICER working papers 25-2007.
- Mndolwa, F. D., & Alhassan, A. L. (2020). Gender disparities in financial inclusion: Insights from Tanzania. African Development Review, 32(4), 578–590. https://doi.org/10.1111/1467-8268.12462
- Morsy, H. (2020). Access to finance – Mind the gender gap. The Quarterly Review of Economics and Finance, 78, 12–21. https://doi.org/10.1016/j.qref.2020.02.005
- Murthi, M., Guio, A.-C., & Dreze, J. (1995). Mortality, fertility, and gender bias in India: A. Population and Development Review, 21(4), 745–782. https://doi.org/10.2307/2137773
- Nanda, K., & Kaur, M. (2016). Financial inclusion and human development: A cross country evidence. Management and Labour Studies, 41(2), 127–153. https://doi.org/10.1177/0258042X16658734
- Nandi, B. K., Hasan, G. Q., & Kabir, M. H. (2021). A tale of the financial inclusion- growth nexus and the degree of financial inclusion: a dynamic panel approach on selected developing countries. Journal of Financial Economic Policy, 14(3), 381–402. https://doi.org/10.1108/JFEP-03-2021-0071
- Ohiomu, S., & Ogbeide-Osaretin, E. N. (2020). Financial inclusion and gender inequality reduction: Evidence from Sub-Saharan Africa. The Indian Economic Journal, 67(3-4), 367–372. https://doi.org/10.1177/0019466220946411
- Park, C. Y., & Mercado, R. V. (2015). Financial inclusion, poverty, and income inequality in developing Asia. Asian Development Bank, 1–17.
- Patrick, H. T. (1966). Financial development and economic growth in underdeveloped countries. Economic Development and Cultural Change, 14(2), 174–189. https://doi.org/10.1086/450153
- Pervaiz, Z., Chani, M. I., Ahmad Jan, S., & Chaudhary, A. R. (2011). Gender inequality and economic growth: A time series analysis for Pakistan. MPRA, 434–439.
- Robinson, J. (1952). The generalization of the general theory the rate of interest and other essays (Vol. A). Macmillan.
- Sahay, R., Cihak, M., N'Diaye, P., Barajas, A., Ayala Pena, D., Bi, R., Gao, Y., Kyobe, A., Nguyen, L., Saborowski, C., Svirydzenka, K., & Yousefi, R. (2015). Rethinking financial deepening: Stability and growth in emerging markets. Staff Discussion Notes, 15(8), 1. https://doi.org/10.5089/9781498312615.006
- Schober, T., & Winter-Ebmer, R. (2009). Gender wage inequality and economic growth: Is there really a Puzzle?" IZA discussion Paper 4323., institute for Study of Labour.
- Schumpeter, J. A. (1911). The theory of development. Harvard University Press.
- Seguino, S. (2000a). Gender inequality and economic growth: A cross-country analysis. World Development, 28(7), 1211–1230. https://doi.org/10.1016/S0305-750X(00)00018-8
- Seguino, S. (2000b). Accounting for Asian economic growth: Adding gender to the equation. Feminist Economics, 6(3), 27–58. https://doi.org/10.1080/135457000750020128
- Seguino, S., Berik, G., & Van der Meulen Rodgers, Y. (2010). An investment that pays off: Promoting gender equality as a means to finance development. FES Gender Study.
- Sethi, D., & Acharya, D. (2018). Financial inclusion and economic growth linkages: Some cross country evidence. Journal of Financial Economic Policy, 10(3), 369–385. https://doi.org/10.1108/JFEP-11-2016-0073
- Sharma, D. (2015). Nexus between financial inclusion and economic growth Evidence from the emerging Indian economy. Journal of Financial Economic Policy, 8(1), 13–36. https://doi.org/10.1108/JFEP-01-2015-0004
- Summers, L. (1994). Investing in all the people. The World Bank.
- Uneze, E. (2013). The relation between capital formation and economic growth: Evidence from sub-Saharan African countries. Journal of Economic Policy Reform, 16(3), 272–286. https://doi.org/10.1080/17487870.2013.799916
- Volart, B. E. (2000). Sex discrimination and growth. IMF Working Paper.
- Volart, B. E. (2004). "Gender Discrimination and Growth: Theory and Evidence from India." DEDPS working paper 1–64.