ABSTRACT
We recently developed and validated a bedside tissue-to-diagnosis pipeline using stimulated Raman histology (SRH), a label-free optical imaging method, and deep convolutional neural networks (CNN) in prospective clinical trial. Our CNN learned a hierarchy of interpretable histologic features found in the most common brain tumors and was able to accurately segment cancerous regions in SRH images.
Conventional methods for intraoperative tissue diagnosis have remained largely unchanged for nearly 100 years in surgical oncology.Citation1 Standard light microscopy is used in combination with hematoxylin and eosin (H&E) staining to provide image contrast for interpretation by a clinical pathologist. The conventional method for intraoperative histology is cumbersome, requiring tissue transport to a remote pathology laboratory, processing by skilled laboratory technicians, and interpretation by a board-certified pathologist. Each of these steps is a potential barrier to providing efficient, reproducible, and accurate intraoperative cancer diagnosis. Moreover, there is currently a discrepancy between the number of board-certified pathologists and the number of medical centers performing cancer surgery.Citation2 In the setting of neurosurgical oncology, this imbalance is expected to increase in the coming years, with up to 40% of neuropathology fellowship positions remaining vacant.Citation3
To augment the existing intraoperative pathology workflow and address the contracting workforce, we recently developed a parallel diagnostic pipeline that combines fiber-laser-based optical imaging and deep learning to provide near-real time brain tumor diagnosis.Citation4 Stimulated Raman histology (SRH) is a label-free, optical imaging method that provides submicron-resolution images of fresh, unprocessed biological tissues. SRH uses the intrinsic vibrational properties of biological macromolecules (e.g., proteins, lipids, nucleic acids) to generate image contrast.Citation5 We have previously shown that SRH is able to capture classic diagnostic image features seen in brain tumors (e.g., microvascular proliferation in glioblastoma, glandular formation in metastatic adenocarcinoma), in addition to histologic findings not seen in conventional H&E histology, including myelin-rich axons and lipid droplets.Citation2 Because SRH requires no tissue processing, image interpretation is not complicated by freezing and section artifact that results from conventional processing in intraoperative H&E histology.
SRH images are natively digital and we had previously demonstrated that SRH is ideally suited for computer-augmented diagnostic techniques(refs).Citation2,Citation6 Our previous methods required manual feature engineering due to limited data size. Advances in computer vision for classification tasks have demonstrated that explicit feature engineering can result in decreased accuracy.Citation7 Therefore, armed with 2.5 million SRH images, we aimed to train a CNN, composed of a trainable feature extractor for optimal performance. Human-level accuracy has been achieved on simulated diagnostic tasks with deep neural networks across several clinical domains, including ophthalmology,Citation8 radiology,Citation9 and dermatology.Citation10
Our pipeline consisted of three steps: 1) image acquisition, 2) image processing, and 3) CNN diagnostic prediction (). An unprocessed surgical specimen are passed off the surgical field and a small sample (e.g., 3 mm3) is compressed into a customized microscope slide. Images are then acquired at two Raman shifts, 2,845 cm−1 and 2930 cm−1. Second, a dense sliding window algorithm is used to generate semi-overlapping, high-resolution and high-magnification SRH patches to be used at both training and inference. We implemented and trained the benchmarked Inception-ResNet-v2 architecture with randomly initialized weights to classify 13 histologic classes.
Figure 1. The SRH-CNN pipeline for automated intraoperative brain tumor diagnosis. (1) A patient newly diagnosed with a brain lesion undergoes a brain biopsy or planned resection. Fresh tissue is loaded directly into a stimulated Raman histology (SRH) imager for image acquisition. Images are acquired at two Raman shifts, 2,845 cm−1 and 2,930 cm−1, and a third image channel is generated via pixel-wise subtraction. Time to acquire a 1 × 1-mm2 SRH image is approximately 2 min. (2) A dense sliding window algorithm generates image patches that are preprocessed to optimize image contrast. (3) Each patch undergoes a feedforward pass through the network. Our inference algorithm is designed to retain the patches with high probability of being diagnostic, filtering the regions that are normal or nondiagnostic. Patch-level predictions from tumor regions are then summed and renormalized to generate a patient-level probability distribution over the diagnostic classes. Our pipeline can provide tissue diagnoses in <2.5 min using a 1 × 1-mm2 image, decreasing time to diagnosis by a factor of 10 compared with conventional intraoperative histology.
MRI, magnetic resonance imaging; H&E, hematoxylin and eosin; CNN, convolutional neural network.
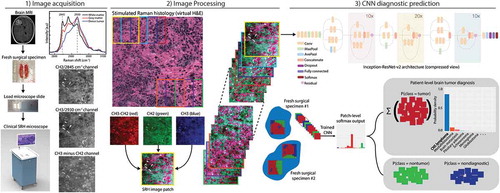
To rigorously test the diagnostic performance of our trained CNN, we performed a multicenter, prospective, non-inferiority, randomized clinical trial comparing conventional H&E histology with pathologist interpretation (control arm) versus SRH with CNN-based interpretation (experimental arm). A total of 278 patients from three tertiary medical centers were included in the study. Overall diagnostic accuracy was 93.9% in the control arm and 94.6% in the SRH-CNN arm, demonstrating that our parallel tissue-to-diagnosis pipeline was noninferior to the current standard of care. Additionally, diagnostic errors were mutually exclusive; errors made by study pathologists in the control arm were correctly classified by the SRH-CNN pipeline, and vice versa. These results indicate that two diagnostic pathways are complementary and the combination of human expertise and artificial intelligence has the potential to improve intraoperative decision making in surgical oncology.
We used neuron activation maximization, a method to qualitatively evaluate the learned latent representations of deep neural networks, to improve the interpretability of our trained CNN. This revealed a hierarchy of SRH feature representations with increasingly complex cytologic and histoarchitectural structures being detected in higher-layers of our CNN. Myelinated axons, high nuclear-cytoplasmic ratios, lipid droplets, pleomorphism, and chromatin organization were differentially detected across brain tumor subtypes. These demonstrate that our CNN learned the diagnostic importance of specific histomorphologic, cytologic, and nuclear features classically used by pathologists to diagnose brain cancer.
Finally, we developed a SRH semantic segmentation method designed to identify the diagnostic and cancerous regions with whole-slide SRH images. By using a semi-overlapping, sliding window method for patch generation, every pixel in an SRH image has an probability distribution over the diagnostic classes that is a function of the local overlapping patch-level predictions. A red-green-blue transparency indicating tumor tissue, normal/non-neoplastic tissue and nondiagnostic regions, respectively, allows for image overlay of pixel-level CNN predictions. Our semantic segmentation method achieved a mean intersection-over-union value of 61.6 ± 28.6 for the ground truth diagnostic class and 86.0 ± 19.2 for the tumor inference class, for patients in our prospective study cohort.
In our study, we demonstrated how combining SRH with deep neural networks can be used to rapidly evaluate fresh surgical specimens and provide intraoperative brain tumor diagnosis. Our pipeline provides a validated means of delivering expert-level intraoperative diagnosis where neuropathology resources are scarce, and augmenting diagnostic accuracy in resource-rich centers. The workflow allows surgeons to access histologic data in near real-time, enabling more seamless use of histology to inform surgical decision-making based on microscopic evaluation of intraoperative specimens.
Disclosure of potential conflicts of interest
No potential conflicts of interest were disclosed.
References
- Gal AA, Cagle PT. The 100-year anniversary of the description of the frozen section procedure. JAMA. 2005;294:1–3. doi:10.1001/jama.294.24.3135.
- Orringer DA, Pandian B, Niknafs YS, Hollon TC, Boyle J, Lewis S, Garrard M, Hervey-Jumper SL, Garton HJL, Maher CO, et al. Rapid intraoperative histology of unprocessed surgical specimens via fibre-laser-based stimulated Raman scattering microscopy. Nat Biomed Eng. 2017;1. doi:10.1038/s41551-016-0027.
- Robboy SJ, Weintraub S, Horvath AE, Jensen BW, Alexander CB, Fody EP, Crawford JM, Clark JR, Cantor-Weinberg J, Joshi MG, et al. Pathologist workforce in the United States: I. Development of a predictive model to examine factors influencing supply. Arch Pathol Lab Med. 2013;137:1723–1732. doi:10.5858/arpa.2013-0200-OA.
- Hollon TC, Pandian B, Adapa AR, Urias E, Save AV, Khalsa SSS, Eichberg DG, D’Amico RS, Farooq ZU, Lewis S, et al. Near real-time intraoperative brain tumor diagnosis using stimulated Raman histology and deep neural networks. Nat Med. 2020;26:52–58. doi:10.1038/s41591-019-0715-9.
- Freudiger CW, Min W, Saar BG, Lu S, Holtom GR, He C, Tsai JC, Kang JX, Xie XS. Label-free biomedical imaging with high sensitivity by stimulated Raman scattering microscopy. Science. 2008;322:1857–1861. doi:10.1126/science.1165758.
- Hollon TC, Lewis S, Pandian B, Niknafs YS, Garrard MR, Garton H, Maher CO, McFadden K, Snuderl M, Lieberman AP, et al. Rapid intraoperative diagnosis of pediatric brain tumors using stimulated raman histology. Cancer Res. 2018;78:278–289. doi:10.1158/0008-5472.CAN-17-1974.
- Krizhevsky A, Sutskever I, Hinton GE. ImageNet classification with deep convolutional neural networks. In: Pereira F, Burges CJC, Bottou L, Weinberger KQ, editors. Advances in neural information processing systems 25. Red Hook (NY): Curran Associates, Inc.; 2012. p. 1097–1105.
- Gulshan V, Peng L, Coram M, Stumpe MC, Wu D, Narayanaswamy A, Venugopalan S, Widner K, Madams T, Cuadros J, et al. Development and validation of a deep learning algorithm for detection of diabetic retinopathy in retinal fundus photographs. JAMA. 2016;316:2402–2410. doi:10.1001/jama.2016.17216.
- Titano JJ, Badgeley M, Schefflein J, Pain M, Su A, Cai M, Swinburne N, Zech J, Kim J, Bederson J, et al. Automated deep-neural-network surveillance of cranial images for acute neurologic events. Nat Med. 2018;24:1337–1341. doi:10.1038/s41591-018-0147-y.
- Esteva A, Kuprel B, Novoa RA, Ko J, Swetter SM, Blau HM, Thrun S. Dermatologist-level classification of skin cancer with deep neural networks. Nature. 2017;542:115–118. doi:10.1038/nature21056.