Abstract
Background
Antibiotic treatment is a well-known risk factor for Clostridioides difficile infection (CDI). The time from start of antibiotic exposure to onset of CDI for different antibiotics is sparsely studied. CDI with onset in the community is often treatable without in-hospital care while CDI patients treated in hospital need isolation, resulting in higher costs and infection control measures.
Objectives
To determine the time from start of antibiotic exposure to onset of healthcare facility-associated CDI for different antibiotics.
Methods
Time between antibiotic exposure and disease onset was evaluated retrospectively with chart reading in a two-centre Swedish setting. A case was attributed to an antibiotic group if this represented more than 2/3 of total antibiotic exposure 30 days before onset of CDI.
Results
Cephalosporins caused CDI faster (mean 7.6 days), and more often during ongoing antibiotic therapy (81% of the cases) than any other antibiotic group. All other common agents had between 2–3 times longer period between start of exposure to onset of CDI (quinolones more than 3 times).
Conclusions
The time gap between antibiotic exposure and onset of CDI is markedly different between different antibiotics. Decreased cephalosporin use could delay onset of healthcare facility-associated CDI and limit infections with onset within the hospital. This might decrease costs for inpatient care, need of infection control measures and shortage of beds in the hospital.
Introduction
Clostridioides difficile infection (CDI) is a potentially severe infection, often following antibiotic exposure [Citation1–3]. The infection is most commonly healthcare-associated (HA-CDI) [Citation4], even though the incidence of community-acquired infections is rising [Citation5]. CDI causes severe suffering for patients and great costs, since there is need for isolation during hospital stay [Citation6,Citation7]. The severity of infection is related to different strains of bacteria, age, co-morbidities and varies from infections with long hospital stays and significant mortality to mild infections, not in need of hospital care [Citation8]. Other important risk factors for developing CDI are renal insufficiency, high comorbidity scores, advanced age, proton pump inhibitor treatment, different malignancies and inflammatory bowel disease [Citation3,Citation9–12].
Many studies have evaluated antibiotic treatment as a risk factor, examining which antibiotics that have the greatest ability to cause CDI. Second and third-generation cephalosporins, clindamycin and quinolones are more prone to cause CDI than other antibiotics [Citation4]. Antibiotic stewardship programs, focused on limiting use of these antibiotics, are effective in decreasing CDI rates [Citation13,Citation14].
The time between antibiotic exposure and the debut of CDI has been sparsely studied. One study concluded that patients suffer the greatest risk for CDI during the first month after the end of antibiotic treatment [Citation15]. Another study evaluated time to cause CDI for different antibiotics as a secondary endpoint. Meropenem had the shortest median time (5 days) cephalosporins between 6–8, clindamycin 8, quinolones 9 and piperacillin/tazobactam 15 days. However, the issue of multiple antibiotic exposure was not addressed, meaning that a patient could receive unlimited antibiotic types and still be included in the analysis [Citation16].
Our study was conducted primarily to examine the time-dependent ability of different antibiotics to cause CDI.
Materials and methods
Setting and design
This was a retrospective two centre cohort study based on chart reading, designed to compare the time lag between antibiotic treatment and CDI onset for different antibiotic classes and if the onset of CDI occurred during or after antibiotic exposure.
The setting was two hospitals in the same administrative region of western Sweden, Skaraborg hospital and Södra Älvsborg hospital. The hospitals together serve an uptake area of 465,000 people and are similar in size and management of CDI patients. There were no recognised outbreaks of HA-CDI during the study period. Both hospitals participated in a national surveillance of CDI conducted by the Public Health Agency of Sweden. According to this report, the most common ribotypes during the study years were 014, 023, 002 and 001 [Citation17].
Inclusion and definitions
Patients were included for chart review during the years 2007, 2012–2016. Patients from 2007 were included, and data was collected, in our project studying an antibiotic stewardship intervention in 2008 [Citation14]. Inclusion criteria were age ≥ 18 years, symptoms of CDI, and a positive test for C. difficile toxin A or B (EIA n = 279, or NAAT n = 106). Onset of the disease was the day of positive CDI test. A complete list of all positive tests during the study years was provided from the hospital laboratories.
After the chart review, patients who did not met criteria for HA-CDI were excluded. The definition of HA-CDI by McDonald et al. was used which was symptom onset (loose stool) either ≥48 h after admission or symptom onset within 4 weeks after inpatient care [Citation18]. Patients with pronounced multiple antibiotic exposure, within 30 days before onset, were also excluded. This was defined as less than 2/3 of the total antibiotic exposure from one single antibiotic type. Patients were then assigned to their dominant exposing antibiotic for comparison between groups.
The definition of severe CDI from Swedish guidelines at the study period was used. Fulfilling one of following criteria for categorised as severe CDI: leukocyte count > 15 x 109/L, s-albumin level ≤ 25 g/L, fever ≥ 38.5 °C, CRP ≥ 150 mg/L, s-creatinine > 130 µmol/L or 50% over baseline, s-urea > 15 mmol/L, or radiologic or endoscopic evidence of severe pseudomembranous colitis [Citation19].
Data collection
The charts were reviewed, by a senior specialist in infectious diseases (J.K.) or a specialist in clinical microbiology (J.E.W.), for exclusion of patients and collection of the variables. The variables collected were patient characteristics and known risk factors for CDI apart for antibiotic exposure (gender, age, duration of in-hospital stay 30 days before onset of CDI, haematological malignancy, inflammatory bowel disease, renal insufficiency, diabetes mellitus, corticoid treatment at admission, proton pump inhibitor treatment at admission (PPI), number of drugs at admission) and outcome variables (mortality (30 days after onset), recurrence (new episode 2-8 weeks after onset), severe CDI, and stay (days) in ICU).
Detailed information was collected regarding duration of exposure of different antibiotics, time to onset of CDI from start of antibiotic exposure and onset of CDI in relation to antibiotic exposure (during or after).
Statistical methods
To compare the time from start of antibiotic exposure to onset of CDI, Kaplan-Meier curves were used. Onset of CDI was set as endpoint. The five most common CDI-causing antibiotic groups, henceforth referred to as the big five, are shown in . For comparison of these curves head-to-head, log rank was used.
Figure 1. Kaplan-Meyer Curve showing the number of days elapsed from start of antibiotic exposure to CDI. For comparison between curves see below.
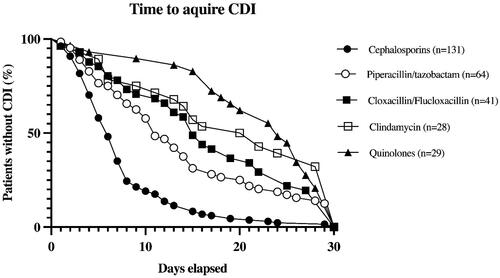
All antibiotic groups were then compared to the group causing CDI fastest (cephalosporins) using Cox regression. The difference between the groups were presented as mean with standard deviation and HR with 95% CI for the comparison.
To adjust for other possible confounding factors for CDI (risk factors other than antibiotic exposure), the risk factors that differed between any of the five major antibiotic groups were included in the cox regression. To choose variables included in the cox regression as possible confounders, Chi-squared test was used head-to-head between the big five, comparing categorical variables with more than n = 10 in each group, otherwise, Fisher’s exact test was used. To compare qualitative variables, the Mann–Whitney U-test was used. All risk factors that differed significantly in any of the comparisons between groups were included and thereby adjusted for possible confounders.
Onset during ongoing antibiotic exposure for the different antibiotic groups was presented as numbers and percentages. The antibiotic groups were compared using binary logistic regression described with OR and 95% CI in comparison to the group where onset during ongoing antibiotic exposure was most prevalent (cephalosporins). This was also adjusted for the significantly differing risk factors as possible confounders, including them for multivariable comparison in the binary logistic regression.
The outcome variables severe CDI, mortality, recurrence, and length of ICU stay were also compared between the antibiotic exposure groups; the categorical variables with binary logistic regression and the qualitative variable with cox regression. These comparisons were also adjusted for the same possible confounders as above.
Severe CDI could be seen as both an outcome variable and a possible marker for an unknown significant risk factor, we therefore conducted a sensitivity analysis including severe CDI as a variable to adjust for this potential risk factor in all regressions. This analyse is labelled multivariate model B.
SPSS version 28.0.1.1 (15) was used for the statistical analyses and GraphPad Prism version 10.1.1 (270) was used to create figures.
Results
751 patients were included for the chart reading. After the review, 385 patients with HCF-CDI could be assigned to one specific major antibiotic and included in the analysis. The antibiotic groups with most assigned cases were cephalosporins (n = 131), piperacillin/tazobactam (n = 64), cloxacillin/flucloxacillin (n = 41), quinolones (n = 29) and clindamycin (n = 28).
The mean age of the study population was 75 years and 53% were female. 44% had severe CDI according to definitions used, 16% had recurrent infection and the 30-days mortality rate was 17%. All patient characteristics for the total study population, and for the five largest antibiotic groups separately, are described in .
Table 1. Patient characteristics.
Head-to-head comparisons between all risk factors collected and all antibiotic groups in the big five showed a significant difference in at least one comparison in the following variables: gender, in-hospital days 30 days before onset, haematological malignancy, and renal insufficiency. Therefore, these risk factors were included and adjusted for in the multivariable model A. There were also differences between groups in severe CDI, this variable was further added to the other risk factors in the sensitivity analysis (multivariate model B).
The results from the Kaplan-Meier curves showed that one antibiotic group, cephalosporins, caused CDI earlier (mean 7.6 days) after start of exposure compared to all the other groups. Also, piperacillin/tazobactam caused CDI earlier than both clindamycin and quinolones, .
The first comparison, days to onset of CDI from start of antibiotic exposure, confirmed the observation that all other antibiotics in the big five caused CDI after longer time than cephalosporins. The differences were quantified with HR and 95% CI. The results were solid after adjusting for possible confounders (multivariable model A) as well as after the sensitivity analysis (multivariable model B). The second most used antibiotic, piperacillin/tazobactam (also the antibiotic second most associated with CDI in the study) showed a HR 0.48 compared to cephalosporins with a 95% CI (0.35–0.66). Notably, quinolones (0.23 (0.15–0.35)) and clindamycin (0.22 (0.14–0.34)) showed the lowest HR of the big five. All other antibiotic groups are described in .
Table 2. Days from start of antibiotic exposure to CDI and onset during ongoing antibiotic treatment.
Onset during ongoing antibiotic exposure was markedly more common when exposed to cephalosporins than any other antibiotic in the big five. The difference to piperacillin/tazobactam (OR 95% CI 0.16 (0.081–0.32)) was even more pronounced in this comparison. Quinolones (0.04 (0.013–0.13)) and clindamycin (0.16 (0.064–0.38)) showed the lowest ORs in this comparison too. Even here, the results were solid after adjusting for possible confounders as well as after the sensitivity analysis. Carbapenems and amoxicillin/clavulanic acid showed non-significant differences already in the univariate analysis and several of the smaller antibiotic groups had too low numbers to be applicable, see .
Outcome variables that were described in were also compared in the big five antibiotic groups. Severe CDI was less common in the quinolone group compared to cephalosporins (OR 95% CI 0.37 (0.15–0.91)), both univariately and when adjusted for confounders, see . 30-days mortality, recurrent CDI and days in ICU didn’t differ significantly in the big five.
Table 3. Severe CDI.
Discussion
Cephalosporins caused CDI faster, and more often during ongoing antibiotic therapy, than any other antibiotic class in our study. This finding contributes to the facts about the tendency of cephalosporins (especially third generation) to cause CDI. It is already known that they cause more CDI than most other antimicrobial agents [Citation4,Citation14]. In a clinical context our findings, may motivate further measures in limiting cephalosporin use through antibiotic stewardship.
Our new data highlights that HA-CDI, in a cephalosporin dominated setting, will in most cases occur during the hospital stay, causing prolonged hospital care and need of isolation. All other common agents had between 2–3 times longer period between start of exposure to onset of CDI (quinolones more than 3 times). With these significantly longer time gaps more infections would be treatable at home since the patients had recovered from their first infection before having CDI. Of course, some of them would still need readmission for in-hospital care of their CDI depending on its severity.
The findings in our study could contribute to more cost-efficient healthcare (since isolation care is expensive), facilitate infection control (room cleaning and hygiene measures), and contribute to curb the shortage of hospital beds. These findings must be confirmed in future studies, measuring appropriate time-dependant outcomes.
An explanation to these pronounced differences could be the cephalosporins dual potential to cause CDI. Both colonisation of bacteria and opportunity of growth and toxin production, facilitated by a disrupted bowel flora, is needed for acquiring CDI. Antibiotic treatment in general is not associated with increased colonisation with C. difficile [Citation20]. When divided into groups though, cephalosporin treatment is associated with increased colonisation while other antibiotics, for example piperacillin/tazobactam seems to prevent it [Citation21,Citation22]. Increasing colonisation and effectively disrupting gut flora at the same time could be multiplying factors for cephalosporins, when causing CDI.
Antibiotics is also often used as prophylaxis during and after surgery and already after prolonging the prophylaxis (all antibiotics) from one to three days the rate of CDI rises [Citation23]. Cephalosporins are a common choice due to its broad coverage but according to the findings in this study other agents would perhaps be more suitable. In the chart reading we noted several cases due to only antibiotic prophylaxis with cephalosporins during and after surgery, an observation consistent with recent studies about colonisation [Citation22].
Clindamycin and quinolones, traditionally seen as risk antibiotics, had onset of CDI remarkably late compared to cephalosporins. Only 14% of quinolone-exposed patients had onset during ongoing antibiotic exposure and the mean time from start of exposure to CDI was 22 days. There are examples of low CDI rates in fluoroquinolone treated patients [Citation24] but resistance to quinolones and spread of hypervirulent strains like 027 is also evident [Citation25,Citation26]. Although, during the study period, the surveillance of strains showed that 027 was uncommon and we had no outbreaks in the hospitals. This could have contributed to the favourable result of the quinolones, also clindamycin to some extent.
The quinolones were also the only group that had less infections categorised as severe CDI compared to cephalosporins (24% compared to 47%). The overall frequency of severe CDI was 44%. This high rate could be partly due to the very including definitions of severe CDI from the national Swedish guidelines during the study years [Citation19]. Nevertheless, the difference between cephalosporins and quinolones in this cohort is still evident.
This study had several strengths and limitations. Even though we knew the strain types circulating, we did nottype all strains in the study. Strain specific factors unknown to us, such as different resistance for the antibiotics used, could have influenced the results. Also, the results from this study might not be applicable to a setting where 027 strains are common.
We used Kaplan-Meyer curves and Cox-regression to compare the antibiotic groups which is usually used when not all included patients had experienced the event (in this case CDI) within the timeframe. However, this was the most reasonable way to do the comparison according to statistical consultants.
A strength is that we have adjusted for other possible risk factors as confounders in multivariable model A and B. Still, there are possible risk factors that were not recorded in the chart review, e.g. corticosteroid doses, the severity of the primary infection needing antibiotic treatment, and dose of antibiotics. We chose days of antibiotic exposure instead of DDD’s since it was time to CDI that was our outcome variable but perhaps dosing of antibiotics could be important as well. We cannot exclude the possibility of remaining unknown confounders causing bias by indication.
Another strength of our study is that the data was retrieved from charts manually. The chart review allowed us to ensure accurate data on antibiotic exposure in detail and to separate CDI from colonisation.
We also tried to address the problem with multiple antibiotic exposure by only including the patients that received over 2/3 of their total exposure by one antibiotic group. The problem is still evident to some extent though. Some antibiotics are more plausible innocent bystanders, and some could be important even if their exposure was less than a third of the total. If we would have included only patients with single antibiotic exposure, the study would have been underpowered since multiple antibiotic exposure is common in hospitalised patients. Also, studies have suggested that combinations of antibiotic exposures can increase CDI risk [Citation27], which might be of importance for the timeframe as well.
To conclude, limiting cephalosporin use will not only decrease rates of HA-CDI, as previously shown. In a non-outbreak setting like ours, it will also markedly delay onset of HA-CDI. This could be an opportunity to decrease costs for inpatient care, need of infection control measures and shortage of beds in the hospital. Further research is warranted to verify our findings in larger cohorts, and to estimate the actual savings in real-life outcome.
Ethical approval
The research was conducted in accordance with the Declaration of Helsinki and national and institutional standards. The study was approved by the regional ethical review board at the University of Gothenburg, Sweden (reference number: 227-16). Informed consent was waived because of the retrospective observational nature of the study.
Authors’ contributions
J.K. and G.J. conceived, planned, and designed the study. J.K and J.E.W. read all journals and collected the data. J.K. conducted the statistical analyses and wrote the manuscript. The manuscript was reviewed by all authors. All authors read and approved the final manuscript.
Acknowledgements
We would like to thank Salmir Nasic, Statistician, Research and Development Centre, Skaraborg Hospital, Skövde, Sweden for valuable assistance regarding statistical methods.
Disclosure statement
All authors report no conflicts of interest relevant to this article.
Data availability statement
Data will be available upon reasonable request to corresponding author.
Additional information
Funding
References
- Brown K, Valenta K, Fisman D, et al. Hospital ward antibiotic prescribing and the risks of Clostridium difficile infection. JAMA Intern Med. 2015;175(4):626–633. doi: 10.1001/jamainternmed.2014.8273.
- Webb BJ, Subramanian A, Lopansri B, et al. Antibiotic exposure and risk for hospital-associated clostridioides difficile infection. Antimicrob Agents Chemother. 2020;64(4):e02169-19. doi: 10.1128/AAC.02169-19.
- Slimings C, Riley TV. Antibiotics and hospital-acquired Clostridium difficile infection: update of systematic review and meta-analysis. J Antimicrob Chemother. 2014;69(4):881–891. doi: 10.1093/jac/dkt477.
- Leffler DA, Lamont JT. Clostridium difficile infection. N Engl J Med. 2015;372(16):1539–1548. doi: 10.1056/NEJMra1403772.
- Fu Y, Luo Y, Grinspan AM. Epidemiology of community-acquired and recurrent Clostridioides difficile infection. Therap Adv Gastroenterol. 2021;14:17562848211016248. doi: 10.1177/17562848211016248.
- Heimann SM, Cruz Aguilar MR, Mellinghof S, et al. Economic burden and cost-effective management of Clostridium difficile infections. Med Mal Infect. 2018;48(1):23–29. doi: 10.1016/j.medmal.2017.10.010.
- Lessa FC, Mu Y, Bamberg WM, et al. Burden of Clostridium difficile infection in the United States. N Engl J Med. 2015;372(9):825–834. doi: 10.1056/NEJMoa1408913.
- Walker AS, Eyre DW, Wyllie DH, et al. Relationship between bacterial strain type, host biomarkers, and mortality in Clostridium difficile infection. Clin Infect Dis. 2013;56(11):1589–1600. doi: 10.1093/cid/cit127.
- Bignardi GE. Risk factors for Clostridium difficile infection. J Hosp Infect. 1998;40(1):1–15. doi: 10.1016/s0195-6701(98)90019-6.
- Mehta P, Nahass RG, Brunetti L. Acid suppression medications during hospitalization as a risk factor for recurrence of Clostridioides difficile infection: systematic review and meta-analysis. Clinical Infect Dis. 2020;73(1):e62–e68. doi: 10.1093/cid/ciaa545.
- Vaishnavi C. Established and potential risk factors for Clostridum difficile infection. Indian J Med Microbiol. 2009;27(4):289–300. doi: 10.4103/0255-0857.55436.
- Hebbard AIT, Slavin MA, Reed C, et al. The epidemiology of Clostridium difficile infection in patients with cancer. Expert Rev Anti Infect Ther. 2016;14(11):1077–1085. doi: 10.1080/14787210.2016.1234376.
- Stevens V, Dumyati G, Fine LS, et al. Cumulative antibiotic exposures over time and the risk of Clostridium difficile infection. Clin Infect Dis. 2011;53(1):42–48. doi: 10.1093/cid/cir301.
- Karp J, Edman-Waller J, Toepfer M, et al. Clostridioides difficile incidence related to in-hospital cephalosporin use: a tale of two highly comparable hospitals. J Antimicrob Chemother. 2019;74(1):182–189. doi: 10.1093/jac/dky408.
- Hensgens MP, Goorhuis A, Dekkers OM, et al. Time interval of increased risk for Clostridium difficile infection after exposure to antibiotics. J Antimicrob Chemother. 2012;67(3):742–748. doi: 10.1093/jac/dkr508.
- Thabit AK, Varugehese CA, Levine AR. Antibiotic use and duration in association with Clostridioides difficile infection in a tertiary academic medical center: a retrospective case-control study. Anaerobe. 2019;59:126–130. doi: 10.1016/j.anaerobe.2019.06.016.
- Rizzardi K, Norén T, Aspevall O, et al. National surveillance for Clostridioides difficile Infection, Sweden, 2009–2016. Emerg Infect Dis. 2018;24(9):1617–1625. doi: 10.3201/eid2409.171658.
- Mcdonald LC, Coignard B, Dubberke E, et al. Recommendations for Surveillance of Clostridium difficile-associated Disease. Infect Control Hosp Epidemiol. 2007;28(2):140–145. doi: 10.1086/511798.
- Norén T. Clostridioides difficile infection, guidelines on behalf of Swedish society of phisicians in infectious diseases https://infektion.net/wp-content/uploads/2017/05/vardprogram_cdi_infektionslakarforeningen_slutversion.pdf.
- Privitera G, Scarpellini P, Ortisi G, et al. Prospective study of Clostridium difficile intestinal colonization and disease following single-dose antibiotic prophylaxis in surgery. Antimicrob Agents Chemother. 1991;35(1):208–210. doi: 10.1128/AAC.35.1.208.
- Dubberke ER, Reske KA, Seiler S, et al. Risk factors for acquisition and loss of Clostridium difficile colonization in hospitalized patients. Antimicrob Agents Chemother. 2015;59(8):4533–4543. doi: 10.1128/AAC.00642-15.
- Anjewierden S, Han Z, Brown AM, et al. Risk factors for Clostridioides difficile colonization among hospitalized adults: a meta-analysis and systematic review. Infect Control Hosp Epidemiol. 2021;42(5):565–572. doi: 10.1017/ice.2020.1236.
- Branch-Elliman W, O'Brien W, Strymish J, et al. Association of duration and type of surgical prophylaxis with antimicrobial-associated adverse events. JAMA Surg. 2019;154(7):590–598. doi: 10.1001/jamasurg.2019.0569.
- Patel N, Gorseth A, Belfiore G, et al. Fluoroquinolone-associated adverse events of interest among hospitalized veterans affairs patients with community-acquired pneumonia who were treated with a fluoroquinolone: a focus on tendonitis, Clostridioides difficile infection, and aortic aneurysm. Pharmacotherapy. 2023;44(1):49–60. doi: 10.1002/phar.2877.
- Sholeh M, Krutova M, Forouzesh M, et al. Antimicrobial resistance in Clostridioides (Clostridium) difficile derived from humans: a systematic review and meta-analysis. Antimicrob Resist Infect Control. 2020;9(1):158. doi: 10.1186/s13756-020-00815-5.
- Razavi B, Apisarnthanarak A, Mundy LM. Clostridium difficile: emergence of hypervirulence and fluoroquinolone resistance. Infection. 2007;35(5):300–307. doi: 10.1007/s15010-007-6113-0.
- Davies K, Lawrence J, Berry C, et al. Risk factors for primary clostridium difficile infection; results from the observational study of risk factors for Clostridium difficile Infection in hospitalized patients with infective diarrhea (ORCHID). Front Public Health. 2020;8:293. doi: 10.3389/fpubh.2020.00293.