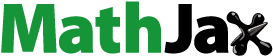
ABSTRACT
Over the past decade, computational modeling based on density functional theory (DFT) calculations provides a deep insight into the catalytic mechanism of single-atom catalysts (SACs) and paves way for high-throughput screening of promising SACs. This review summarizes computational methods for the analysis of the electronic structures and catalytic performance of SACs, as well as introduces the utilization of descriptors for the computational design of SACs. We expect that future advances in computational methods will surely help to identify highly effective SACs for a wide variety of reactions.
Graphical Abstract
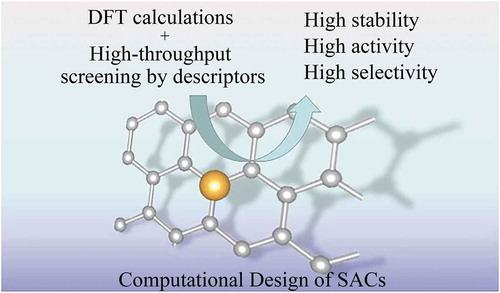
1. Introduction
Widely applied in more than 90% of chemical processes, heterogeneous catalysts have attracted the most extensive attention in the industrial production of the majority of chemicals [Citation1,Citation2]. Traditional heterogeneous catalysts of supported nanoparticles have played an important role in the efficient production of chemicals. However, they may suffer from low atom utilization efficiency and low selectivity for desired products in a lot of reactions. To handle these drawbacks, scientists have turned their eyes to atomically dispersed metal atoms anchored on supports, which are defined as single-atom catalysts (SACs) [Citation3]. The distinctive structures of SACs make them very promising as heterogeneous catalysts. Firstly, the identical active sites of SACs enable them to selectively catalyze only a certain kind of reaction. Moreover, as all of the atoms of SACs are exposed to reactants, their atom utilization efficiency is close to 100%, which can increase the catalytic activity per atom and reduce the consumption of metal resources [Citation4]. In addition, the relatively simple structure of SACs provides scientists with an ideal model to explore the relationship between the active sites and their catalytic performance, paving the way for the rational design of SACs [Citation5]. Upon these advantages, SACs have been experimentally fabricated for various reactions, showing promising prospects in catalysis [Citation6–10].
Although SACs with high catalytic performance have been identified experimentally, the intrinsic factors that determine their catalytic performance remain unclear. Compared with experimental studies, computational modeling by density functional theory (DFT) calculations could offer more explicit insights into the reaction processes. Based on DFT calculations, the whole reaction cycle and the energy barriers of each elementary step can be calculated. Moreover, DFT calculations could be used to analyze the electronic structures of the active sites and reveal their structure–performance relationship. Favored with these advantages, DFT calculations have been widely applied to the study of SACs [Citation11–14].
In this review, we summarize computational methods that open avenues for the rational design of SACs. We first introduce DFT calculations of the electronic structures of SACs. Then, we summarize calculation methods for evaluating the catalytic performance of SACs, including stability, activity, and selectivity. Finally, we describe the development of high-throughput methods for designing SACs based on descriptors, as shown in . It is believed that these advanced computational methods and descriptors could be used to guide the experimental studies and design efficient SACs for a wide variety of reactions.
Figure 1. Computational design of SACs. (a) Spin density distribution of Fe supported on P3-doped graphene SAC. (Reprinted from Ref [Citation47]. Copyright 2018 Elsevier Ltd.). (b) Relative chemical potentials of Au nanoparticles and single atoms at different sites of CeO2 support. (Reprinted from Ref [Citation28]. Copyright 2017 American Chemical Society). (c) Schematic of CO oxidation on MoS2 supported Co SAC. (d) Schematic of titanium nitride supported Pt SAC selectively catalyze oxygen reduction and formic acid oxidation reaction. (Reprinted from Ref [Citation15]. Copyright 2016 WILEY‐VCH Verlag GmbH & Co. KGaA, Weinheim). (e) Volcano relation between adsorption free energy of OOH and limiting potential of SACs for oxygen electroreduction reaction. (Reprinted from Ref [Citation66]. 2019 American Chemical Society). (f) Relation between adsorption free energy of OH and structure descriptor φ of graphene supported transition metals. (Reprinted from Ref [Citation27]. Copyright 2018 The Author(s), under exclusive licence to Macmillan Publishers Ltd, part of Springer Nature). (g) DFT calculated adsorption energy versus predictive adsorption energy for transition metals on oxide supports (γ-Al2O3, MgO, and MgAl2O4). (Reprinted from Ref [Citation16]. Copyright 2019 American Chemical Society)
![Figure 1. Computational design of SACs. (a) Spin density distribution of Fe supported on P3-doped graphene SAC. (Reprinted from Ref [Citation47]. Copyright 2018 Elsevier Ltd.). (b) Relative chemical potentials of Au nanoparticles and single atoms at different sites of CeO2 support. (Reprinted from Ref [Citation28]. Copyright 2017 American Chemical Society). (c) Schematic of CO oxidation on MoS2 supported Co SAC. (d) Schematic of titanium nitride supported Pt SAC selectively catalyze oxygen reduction and formic acid oxidation reaction. (Reprinted from Ref [Citation15]. Copyright 2016 WILEY‐VCH Verlag GmbH & Co. KGaA, Weinheim). (e) Volcano relation between adsorption free energy of OOH and limiting potential of SACs for oxygen electroreduction reaction. (Reprinted from Ref [Citation66]. 2019 American Chemical Society). (f) Relation between adsorption free energy of OH and structure descriptor φ of graphene supported transition metals. (Reprinted from Ref [Citation27]. Copyright 2018 The Author(s), under exclusive licence to Macmillan Publishers Ltd, part of Springer Nature). (g) DFT calculated adsorption energy versus predictive adsorption energy for transition metals on oxide supports (γ-Al2O3, MgO, and MgAl2O4). (Reprinted from Ref [Citation16]. Copyright 2019 American Chemical Society)](/cms/asset/4f92b13b-adcd-48bd-a044-f268187a98e9/tapx_a_1905545_f0001_oc.jpg)
2. DFT calculations of SACs
Through DFT calculations, the electronic structure of SACs can be theoretically revealed. The electronic analysis is useful for the construction of descriptors based on the identification of the intrinsic properties determining the catalytic performance of SACs. Moreover, DFT calculations can predict the stability, activity, and selectivity of SACs. These predictive results are the criteria for the evaluation of the accuracy of identified descriptors. Thus, DFT calculations of the intrinsic properties and catalytic performance of SACs are the prerequisites for the computational design of SACs.
2.1. Electronic structures
The performance of catalysts is in principle determined completely by electronic structures [Citation17]. The eximious performance of SACs in a series of reactions has been elucidated from the analysis at the electronic scale [Citation18–21]. Moreover, revealing the electronic factors that determine the catalytic performance may point out directions for tuning the catalytic performance of SACs.
The analysis of the electronic structures can be used to understand the stability and activity of SACs. As illustrated in , the stability and activity of SACs are in a competitive relationship. The stability of SACs corresponds to the fully occupied bonding orbitals [Citation22]. The more hybridization between the orbitals of the single atoms and the support can lead to stronger binding strength. For example, partial density of states (PDOS) of Pt@nitrogen-doped graphene shows that the discrete 5d-orbitals of Pt interact with the N-2p orbitals around the Fermi level [Citation20]. Charge transfers from Pt to N atom, and the calculated adsorption energy of Pt (5.171 eV) clearly suggests that it is stable, which is in accordance with experiment [Citation20]. The orbital hybridization between the single atom and the support also induces the strong metal–support interactions (SMSIs) within oxide-supported single metal atoms [Citation23]. Due to SMSI, single atoms can stably stay on the supports. For instance, DFT calculations and experiments prove that Au single atoms are ultrastable on iron oxide nanocrystallites [Citation24]. The Au atoms are bound to the support by strong covalent metal–support interactions (CMSI) [Citation24]. Bader charge analysis and calculated valence electron configurations show that the anchored Au single atoms are highly positively charged, which enables them to interact strongly with the negatively charged support [Citation24]. The SMSI and electrostatic interaction thus account for the high stability of Au single atoms against sintering. On the other hand, the activity of SACs corresponds to the free electrons near the Fermi level because of the unsaturated coordination [Citation22]. The free electrons determine the activity of SACs by tuning the binding strength between them and the adsorbates. As it is well-known that the activation of adsorbates can be ascribed to the moderate binding energy, namely, not too weak to make the activation energy of reactants too high or too strong to let the products hard to desorb. For instance, after dilute Ag by Cu, the activation barrier of bulk Cu for H2 formation in methanol reforming is reduced by 0.1 eV [Citation25]. This is partially because the dilution narrows the 3d state of Cu and contributes to the covalent bond formation when Cu interacts with hydrogen. The Cu in the AgCu alloy as the minority element is found to exhibit free-atom like d-state structure, and this phenomenon is also found for several other single-atom alloys (SAAs) – the structure of metal supported single metal atoms, pointing out a new approach to SAAs that may exhibit excellent catalytic performance [Citation25].
Figure 2. Schematic illustration of the d state determines the stability and catalytic activity of SACs. (Reprinted from Ref [Citation22]. Copyright © 2019 Published by Elsevier B.V.)
![Figure 2. Schematic illustration of the d state determines the stability and catalytic activity of SACs. (Reprinted from Ref [Citation22]. Copyright © 2019 Published by Elsevier B.V.)](/cms/asset/c6651a9c-b5da-41f0-8836-4649138966ee/tapx_a_1905545_f0002_oc.jpg)
2.2. Evaluation of stability
Structural stability is the prerequisite for industrial application of an effective SAC. Single atoms are often easy to aggregate into nanoparticles (NPs), which are reported to be inactive in many reactions. Another problem that influences the stability of SACs is the leaching of metal atoms. In this case, the amount of the active site reduces, leading to low activity and selectivity. Thus, stable SACs mean that single atoms stay on the surface without desorbing and diffusing.
Based on DFT calculations, both calculated thermodynamic and kinetic properties are useful in ascertaining the stability of SACs, and overall consideration is necessary [Citation26]. Thermodynamically, the stability of SACs is evaluated by energetics. The ability of SACs against aggregation can be evaluated by comparing the adsorption energy of the metal atom (Ead) on the support and the bulk cohesive energy (Ecoh) defined by Equationequations (1)(1)
(1) and (Equation2
(2)
(2) ), respectively [Citation27].
where n is the number of atoms in the bulk; Esupport+atom, Esupport, Efree-atom, and Ebulk are the energies of the support anchored with a metal atom, the pristine support, the isolated metal atom, and the bulk, respectively. If the adsorption energy is smaller than the bulk cohesive energy, SACs are stable against aggregation and vice versa. With the calculated value of adsorption energy, the ability of SACs against metal being leached can also be facilely evaluated, a stable SAC exhibits negative adsorption energy value. Moreover, the ability of SACs against aggregation can be described by Gibbs free energy. As shown in ), SACs with smaller Gibbs free energy than NPs are stable. For SACs, the chemical potential can be calculated by:
Figure 3. (a) Schematic illustration of the free-energy diagram of sintering and dispersion processes between Au NPs and SAs. (Reprinted from Ref [Citation26]. Copyright 2018 American Chemical Society, 2018 The Author(s). (b) Chemical potential of Au single atoms and NPs on different supports. (Reprinted from Ref [Citation28]. Copyright 2017 American Chemical Society)
![Figure 3. (a) Schematic illustration of the free-energy diagram of sintering and dispersion processes between Au NPs and SAs. (Reprinted from Ref [Citation26]. Copyright 2018 American Chemical Society, 2018 The Author(s). (b) Chemical potential of Au single atoms and NPs on different supports. (Reprinted from Ref [Citation28]. Copyright 2017 American Chemical Society)](/cms/asset/69347a84-c7f7-41cd-8e81-d366222bfcd5/tapx_a_1905545_f0003_oc.jpg)
where μSAC and Esupport+atom represent the chemical potential and energy of SAC, respectively. Esupport represents the energy of the pristine support and Ebulk represents the energy of one atom in bulk.
For NPs, the chemical potentials can be approximated by the formation energy calculated from:
where μNP, γ, and R represent the chemical potential, the surface energy, and the curvature radius of the NPs, respectively. Ω represents the molar volume of one atom in bulk. For instance, the calculated chemical potential of Au single atoms and NPs on different supports are plotted in ), clearly showing the stability of single atoms compared with NPs [Citation28]. In addition, by combined chemical potential calculation and electronic structure analysis, the stability of supported Fe SACs was investigated [Citation29]. The doped N could promote charge transfer and create a different coordinate environment that improves the thermodynamic stability of these SACs.
On the other hand, dynamically, energy barriers are used to evaluate the stability of SACs, as shown in ) [Citation28,Citation30]. If the energy barrier of single atoms aggregating to NPs is high, the SAC is thought to be stable against aggregation. Besides, a high enough energy barrier of the single atoms diffusing away from another site suggests that they are stable against being leached. Based on the calculated static energy barriers, the temperature (T)-related ability of self-diffusion of adatoms can also be evaluated. For example, for in-channel diffusion, the jump rate and diffusion coefficient D can be calculated by:
where represents the prefactor,
represents the activation barrier, a represents the distance between two adjacent sites and
represents the probability of an
-sites jump. Based on these parameters, the ability of self-diffusivity of adatoms on Cu(110), Ag(110) and Au(110) was investigated. The calculated results show that at 450 K, self-diffusion on Cu(110) is activated, while on Ag(110) and Au(110) are similar [Citation31]. At high temperatures, the percentage of long-jumps on Cu(110) and Ag(110) is increased, which can be explained by the energy dissipation rate of the adatom and the multidimensional topology of the potential energy surface [Citation31].
Especially, for electrochemical reactions, the ability of SACs against leaching can be determined by the dissolution potential [Citation32]. The leaching equation for a supported n-atom cluster (Mn/substrate) can be written as:
The corresponding reaction-free energy at potential U is:
where (M2+,aq) can be calculated from the chemical potential of bulk metals G(M,s) and experimental standard redox potential U0:
when ΔG<0, metal leaching proceeds spontaneously, thus the dissolution potential Un for leaching one metal atom can be estimated as:
Based on this method, Liu et al. [Citation33] calculated Un for NC, Fe2O3, and graphdiyne supported Ptn and Nin (n =1-20) clusters as well as NC and Fe2O3 supported Pdn (n =1-20) clusters. The highest leaching equilibrium potential before the formation of atomically dispersed structure and the lowest equilibrium potential for destroying the structure can be used to define the boundaries of the electrochemical potential window within which the SAC is stable against metal leaching. Similarly, the electrochemical potential window for graphdiyne supported Pt3 and Ni3 single cluster catalysts (SCCs) are also plotted. This approach of constructing electrochemical potential windows may also be extended to other supported metal clusters, providing a strategy for the selection of stable SACs and SCCs.
2.2. Evaluation of activity and selectivity
2.2.1. Activity
High catalytic activity and selectivity are the ultimate goals for designing SACs. Guided by DFT calculations, these catalytic properties for several reactions can be predicted, including widely studied reactions such as CO oxidation [Citation30], water gas shift [Citation34], selective hydrogenation [Citation35], half reactions in the fuel cell [Citation27], electrochemical nitrogen [Citation14] and CO2 reduction [Citation36] and other reactions such as aromatic nitroreduction [Citation37], alkane dehydrogenation [Citation38], selective dehalogenation [Citation39] and so on. We first introduce computational methods for the evaluation of the catalytic activity. As examples, we mainly introduce the industrial widely applied gas-solid phase reactions and electrochemical reactions.
2.2.1.1. Gas-solid phase reactions
To theoretically evaluate the activity and selectivity of SACs, unraveling the reaction mechanisms is a crucial job. For gas-solid phase reactions, this process is accomplished by selecting the initial and final stable structures for each elementary step and the transition states between them as well as getting corresponding energies for these structures. With these energies, activation barriers can be calculated, and reaction cycles during the reaction process can be easily plotted. As shown in , CO oxidation goes through a Mars-van Krevelen (Mvk) mechanism on Pt1/CeO2. The oxygen atom of the support participates in the catalytic cycle, after the formation of two CO2 molecules, the support is reoxidized by an adsorbed O2. The potential energies of the corresponding structures are plotted within one diagram, showing the energy changes and reaction barriers along the reaction process.
Figure 4. (a) Potential energy diagrams and reaction cycle for CO oxidation on Pt1@CeO2. (Reprinted from Ref [Citation46]. Copyright 2019, Springer Nature)
![Figure 4. (a) Potential energy diagrams and reaction cycle for CO oxidation on Pt1@CeO2. (Reprinted from Ref [Citation46]. Copyright 2019, Springer Nature)](/cms/asset/723013ad-4917-4df9-8a5c-7a9bb52be3b7/tapx_a_1905545_f0004_oc.jpg)
To evaluate activity, microkinetic models are then established by solving equations describing the coverage of intermediates, with the rate constant (k) calculated through harmonic transition state theory by equations (12) and (13) [Citation40–45].
where kB represents the Boltzmann constant, T represents the absolute temperature, Ea represents the activation barrier, q represents partition functions and vi represents the vibrational frequency. Through this method, kinetic models for several gas-phase reactions have been established, and different SACs have been selected as potential catalysts, such as Au/TiO2 [Citation40], Ag/MgO, and Ag/Fs-defect MgO [Citation45] for CO oxidation, and Pt/Mo2C [Citation43] and Pt/TiO2 [Citation42] for water-gas shift reaction.
2.2.1.2. Electrochemical reactions
For electrochemical reactions, the activity of SACs is determined by the potential-determining step (PDS), which is defined as the step with the most maximum value of free energy change through the reaction process [Citation47]. The PDS is the thermodynamic bottleneck for a reaction and the degree of difficulty of this step well describes the activity of the SACs [Citation48,Citation49]. The corresponding potential of the PDS is called the limiting potential (UL), defined as Equationequation (14)(14)
(14) :
where ΔGPDS is the free energy change of the PDS.
In the case of HER, the free energy of H adsorption () is used to evaluate the activity of SACs [Citation50–52]. An effective SAC for HER should have
close to zero to lower the reaction barriers of hydrogen adsorption and desorption steps [Citation27]. For example, by investigating
on a series of SACs, as shown in ), Hossain et al. [Citation53] proposed Co@N-doped graphene as a promising SAC for HER with the
value close to zero, which is proved by their further theoretical analysis and experimental results. For multi-step electrochemical reactions with several intermediates, free energy diagrams along reaction pathways are plotted, as shown in ) for oxygen evolution reaction (OER). A SAC with lower free energy change of the PDS is proved to be able to exhibit higher activity in the reaction. Based on these analyses, the PDSs on different SACs are highlighted in ) and Ni embedded in nitrogen-doped holey graphene frameworks stands out with the lowest free energy change (0.42 eV) for the PDS [Citation7]. Besides, with the value of the UL, overpotential defined as the difference between it and the equilibrium potential for an ideal catalyst can also be used to evaluate the activity of SACs for electrochemical reactions [Citation54]. A smaller overpotential indicates higher activity of the corresponding SAC.
Figure 5. (a) for HER on a series of SACs. (Reprinted from Ref [Citation53]. Copyright 2019 WILEY‐VCH Verlag GmbH & Co. KGaA, Weinheim). (b) Free energy diagram at 1.23 V for OER over Fe, Co, and Ni embedded in nitrogen-doped holey graphene frameworks. (Reprinted from Ref [Citation7]. Copyright 2018 The Author(s))
![Figure 5. (a) ΔGH∗ for HER on a series of SACs. (Reprinted from Ref [Citation53]. Copyright 2019 WILEY‐VCH Verlag GmbH & Co. KGaA, Weinheim). (b) Free energy diagram at 1.23 V for OER over Fe, Co, and Ni embedded in nitrogen-doped holey graphene frameworks. (Reprinted from Ref [Citation7]. Copyright 2018 The Author(s))](/cms/asset/a6464066-de6b-4934-b0df-987458bc7026/tapx_a_1905545_f0005_oc.jpg)
2.2.2. Selectivity
By plotting reaction cycles and free energy diagrams, the selectivity of SACs can also be readily determined. Generally, for competitive steps, the reaction would go through the step with a lower activation energy or free energy barrier. As shown in , on Mo-based MOF, CO2 reduction reaction (CO2RR) chooses the way with a lower free energy barrier for each step and results in the selective production of CH4. As adsorption is the first step for surface reactions, the difference between the adsorption free energy of the main adsorbates can also be used as a descriptor for evaluating their selectivity [Citation55,Citation56]. For instance, to reduce the competition of HER in nitrogen reduction reaction (NRR), the adsorption of H at the active sites should be suppressed. Therefore, by comparing the adsorption energy of N2 and H, the defective graphene-based SACs such as Mo@C3, Nb@C3, V@C3, and Mn@N3 have been proved to show high selectivity with smaller adsorption energy of N2 [Citation55]. This is in accordance with the ensemble effect in SACs that H can only be adsorbed at the top site of the single atoms [Citation55]. Besides, Bader charge analysis and charge density difference show that the Ti in Ti@N4 is positively charged, which also contributes to the reduced H adsorption by electrostatic repulsion [Citation55].
Figure 6. Free energy diagram for CO2RR on Mo-based MOF. (Reprinted from Ref [Citation60]. Copyright 2019 Elsevier B.V. All rights reserved.)
![Figure 6. Free energy diagram for CO2RR on Mo-based MOF. (Reprinted from Ref [Citation60]. Copyright 2019 Elsevier B.V. All rights reserved.)](/cms/asset/c56bbb79-be03-449a-8554-e438ba8dacf7/tapx_a_1905545_f0006_oc.jpg)
On considering the whole reaction processes, the step that determines the kinetic activity of SACs in gas-solid phase reactions or PDSs in electrochemical reactions is used to evaluate the selectivity of SACs. The reaction process with lower reaction barriers will be selectively chosen. For example, in the gas-solid phase selective hydrogenation of 3-nitrostyrene, only the H atoms at the top site of the active atom can be added to substrates; thus, the energy barrier of H diffusion from the fcc-hollow site to the top site (denoted as Ea-react) is used as a descriptor for the catalytic activity [Citation57]. Subsequently, the selectivity of hydrogenation of 3-nitrostyrene on Ni(111), Pt1/Ni(111), and Pt(111) was investigated. The selectivity of Pt(111) is low because the nitro groups of 3-nitrostyrene molecule interact with the support too weakly to be activated. While on Ni(111), a high Ea-react value (above 0.5 eV) indicates that the hydrogenation is hard to proceed. Only the Pt1/Ni(111) SAC can selectivity hydrogenate 3-nitrostyrene with a low Ea-react value (0.17 eV). Besides, in the electrochemical reduction of oxygen, the two-electron transfer oxygen reduction reaction (ORR) toward H2O2 competes with four-electron transfer ORR toward H2O. At 0.6 V, the free energy change of PDSs at each active site on Fe@N doped graphene for four-electron transfer ORR are negative, much lower than the free energy change of PDSs for the two-electron transfer processes, which are all positive [Citation58]. Therefore, by comparing the PDSs, Fe@N doped graphene is proved to be selective for H2O production with four-electron transfer ORR. Moreover, for electrochemical reactions in a protic solvent, HER is easy to become a side reaction. In this case, SACs with lower free energy barriers for the main reactions than HER are thought to be selective [Citation59].
3. Computational design of SACs
Though of fine accuracy, the predictive results given by DFT calculations in Section 2 usually cannot be used directly for the rational design of SACs, as they do not reflect the intrinsic properties of SACs. However, based on these calculated catalytic performances and electronic analysis, effective descriptors can be identified. The descriptors can act as bridges between intrinsic properties and the catalytic performance of SACs. Starting from the desired catalytic performance, the corresponding value ranges of descriptors are determined, pointing out favored intrinsic properties of effective SACs. Identifying easily calculated and accurate descriptors thus opens an approach for the high-throughput screening and computational design of SACs.
3.1. Usage of energy descriptors
In some reactions, the catalytic performances of SACs have been described by a series of energy-related parameters, which are called the energy descriptors [Citation14,Citation61]. The identified energy descriptors are much easier to be figured out compared to the whole reaction process, facilitating the screening of effective SACs. Besides, the correspondence between energy descriptors and catalytic performance usually can be explained by electronic factors, which can help to understand the catalytic mechanisms and prove the reliability of identified energy descriptors.
An effective way of identifying energy descriptors starts from the Sabatier principle that an efficient catalyst should bind key reaction intermediates moderately. Thus, the adsorption energies or adsorption Gibbs free energies (ΔGad) of key intermediates can be used as predictive descriptors. In general, for reactions containing more than one key intermediates, there may be several descriptors. At this point, the scaling relationships between their adsorption energies or adsorption Gibbs free energies provide guidance in limiting the number of independent variables and thus simplifies the descriptors. In fact, as the variations in adsorption energies and transition state energies follow the same basic physics, transition state energies also correlate with adsorption energies. Thus, scaling relations called Brønsted–Evans–Polanyi (BEP) relations exist between activation (free) energies and reaction (free) energies, which is exactly what Sabatier principle describes [Citation54,Citation61]. Following these relations, several predictive descriptors can be identified for different reactions, and representative examples are demonstrated as follows.
In ORR and OER, scaling relations exist between adsorption Gibbs free energies of reaction intermediates and OH, as shown in ), which means that Sabatier principle works for these systems [Citation62]. The change in adsorption Gibbs free energies is explained by the d-band center. It means that the higher the εd, the stronger the adsorption energy of intermediates [Citation54]. Through the reaction equation of each step, reaction free energies can be expressed by the adsorption free energy of intermediates, which are in correlation with OH adsorption Gibbs free energy. Correlating with the overpotential for ORR, a contour plot with OH adsorption Gibbs free energy as energy descriptor is discovered as ), and candidates can then be easily chosen [Citation54]. Similarly, for NRR, scaling relations between adsorption energies of *NNH, *NNH2, *NH, *NH2, and *N (,
,
,
, and
) are discovered [Citation61]. The change in
is explained by projected crystal orbital Hamilton population (pCOHP) and integrated COHP (ICOHP) analyses, and the value of
is used as the rate-limiting steps [Citation61]. As all the rate-limiting steps are related with *NNH and *NH2, their Gibbs free energy (
and
) are discovered as energy descriptors for the limiting potential, and contour plots are plotted.
Figure 7. (a) Scaling relations between adsorption Gibbs free energies of reaction intermediates and OH. (b) Contour plots of the limiting potential as a function of the adsorption Gibbs free energy of NNH and NH2. ((a) and (b) are reprinted from Ref [Citation54]. Copyright 2018 Elsevier B.V. All rights reserved). (c) The relationship between the energy of CO adsorption and the energy of oxygen vacancy formation on doped oxide. (d) The relationship between the energy of O2 adsorption and the energy of oxygen vacancy formation on doped oxide. ((c) and (d) are reprinted from Ref [Citation63]. Copyright 2008 American Chemical Society)
![Figure 7. (a) Scaling relations between adsorption Gibbs free energies of reaction intermediates and OH. (b) Contour plots of the limiting potential as a function of the adsorption Gibbs free energy of NNH and NH2. ((a) and (b) are reprinted from Ref [Citation54]. Copyright 2018 Elsevier B.V. All rights reserved). (c) The relationship between the energy of CO adsorption and the energy of oxygen vacancy formation on doped oxide. (d) The relationship between the energy of O2 adsorption and the energy of oxygen vacancy formation on doped oxide. ((c) and (d) are reprinted from Ref [Citation63]. Copyright 2008 American Chemical Society)](/cms/asset/9434b1f2-374c-4b37-b23b-f647629361b8/tapx_a_1905545_f0007_oc.jpg)
Apart from using Sabatier analysis and scaling relations, several energy descriptors have been discovered through the analysis of reaction mechanisms. For instance, all the reaction mechanisms for NRR contains two ‘stable to instable’ transitions: *N2 + H+ + e− = *N2H and *NH2 + H+ + e− = *NH3 [Citation14]. Besides, the first and final steps of the chemisorption of N2 and desorption of produced NH3, respectively, are also crucial. Hence, the free energy change of the two key elementary steps ( and
), N2 adsorption energy (
) and NH3 desorption free energy (
) are identified as energy descriptors in NRR [Citation14]. Another example is the identification of energy descriptors for CO oxidation on rutile TiO2(110) supported SACs. The reaction goes through MvK mechanism, and only the energy changes of CO reaction with the surface oxygen atom and O2 adsorption at the vacancy site vary with different dopants. Additionally, the calculated energy change of the former step is a linear function of
while that of the latter step decays linearly with
, as shown in . Therefore, the energy of oxygen vacancy formation is identified as an energy descriptor [Citation63].
3.2. Utilizing structure descriptors
Energy descriptors have been proved to be successful for the rational design of SACs. However, they do not include the intrinsic structural factors determining catalytic properties. Hence, it is usually hard to directly tune the values of energy descriptors such as adsorption energies of SACs to engineer their performance to the top of the volcano plot. In this sense, aided by the analysis of electronic structures and other intrinsic properties, structure descriptors relating the catalytic properties with the intrinsic structural properties have been identified.
In our previous work, a universal structure descriptor that can describe the activity for several reaction systems was presented for ORR, OER, and HER reactions by the graphene-based SACs [Citation27]. A total of 112 graphene-based SACs were constructed and calculated [Citation27]. Though and
correlate well with ORR, OER, and HER activity, they do not reflect the intrinsic characteristics of the active center. Based on the analysis of the electronic structure of the active sites, the structural parameter φ, which is defined as
(θd is the d orbital of the metal atom; EM, EO, EH, EN, and EC are electronegativity of the metal atom, O, H, N and, C, respectively; nN and nC are the number of nearest N and C atoms and
is correction coefficient), was identified as a structure descriptor. As plotted in , SACs owning a φ value of 30–32, 30–35, and around 17 or 39 are prone to exhibit higher activity than precious-metal-based commercial catalysts for ORR, OER, and HER, respectively [Citation27]. This work opens an avenue for identifying universal structure descriptors in the usage of predicting the performance of catalysts for several reactions through one readily obtained parameter.
Figure 8. Theoretical and corresponding experimental onset potentials for (a) ORR and (b) HER versus structure descriptor φ. (Reprinted from Ref [Citation27]. Copyright 2018 the Author(s), under exclusive license to Macmillan Publishers Ltd, part of Springer Nature). (c) Overpotential for CO2RR as a function of structure descriptor Φ. (d) Relationship between the Bader charge of the transition metal ions and descriptor Φ. ((c) and (d) are reprinted from Ref [Citation64]. Copyright 2019 WILEY-VCH Verlag GmbH & Co. KGaA, Weinheim)
![Figure 8. Theoretical and corresponding experimental onset potentials for (a) ORR and (b) HER versus structure descriptor φ. (Reprinted from Ref [Citation27]. Copyright 2018 the Author(s), under exclusive license to Macmillan Publishers Ltd, part of Springer Nature). (c) Overpotential for CO2RR as a function of structure descriptor Φ. (d) Relationship between the Bader charge of the transition metal ions and descriptor Φ. ((c) and (d) are reprinted from Ref [Citation64]. Copyright 2019 WILEY-VCH Verlag GmbH & Co. KGaA, Weinheim)](/cms/asset/7a17d4b3-d7c7-4064-8634-45017a5f8e7a/tapx_a_1905545_f0008_oc.jpg)
In another work, structure descriptor Φ =VM×EM/rM (VM, EM and, rM represent the valence electron number of metals, the electronegativity of central metals, and the radius of metal ions, respectively) was discovered which well correlates with the reaction overpotential for M-N-C SACs for CO2RR [Citation64]. As the volcano relationship in ) shows, in the regime of Φ <5.0 the reaction favors HER while in other regimes favors CO2RR. Besides, in different regimes, the reaction goes through different pathways labeled η1(C) (CO2 → *COO → *COOH → *CO → CO) or η2(C, O) (CO2 → O*CO → O*COH → O*C → CO) [Citation64]. These suggest that the descriptor Φ is an effective descriptor that can well distinguish different reaction mechanisms, intermediates, reaction pathways, and final products. In addition, the Bader charge of the metal ions is in relation to descriptor Φ, as shown in ), indicating its power to reflect intrinsic factors. It is expected that Φ can be used as a universal structure descriptor of M-N-C SACs for wider applications.
Other attempts have also been made to the rational design of SACs through the construction of structure descriptors. Based on the calculated magnetic moment and adsorption energy as well as the analysis of electronic structure, 0.62X-0.21εd-5.04 was identified as a structure descriptor for O adsorption energy on graphene-based SACs [Citation65], where X represents the electronegativity and εd represents the d-band center of the catalytic surface. This descriptor can be used for the high-throughput screening of SACs [Citation66].
3.3. Machine-learning aided catalyst design
Utilizing descriptors has indeed promoted the rational design of SACs. However, up to now, universal descriptors that are suitable for various types of SACs and reaction systems are still insufficient. As a result, a vast amount of DFT calculations still need to be carried out to screen different descriptors for each reaction system. At this point, machine-learning based methods can help to break the bottleneck [Citation67]. Based on DFT calculations providing basic data, statistical learning could directly point out the accurate correlations between the intrinsic factors and catalytic performance, giving effective function forms to predict the catalytic properties. Recently, successful attempts by combined DFT and statistical learning or machine-learning methods have been reported to study SACs [Citation68].
An example is to identify different descriptors that can predict interaction strengths between single atoms and the oxide support by combined DFT calculations and statistical learning approach [Citation68]. Through DFT calculations, the oxide formation enthalpy (ΔHf, ox) of metal adatom and the oxygen vacancy formation energy (ΔEvac) of support correlate well with the binding energy of single metal atoms, which can be explained by the electronic interactions as introduced in Section 2.1 [Citation68]. In the following, the feature space was expanded to containing 333,932 descriptors that include parameters of the atomic properties of the adatom and support. Through these descriptors, binding energy was predicted, and their comparison to DFT calculated data is shown in . By LASSO+l0 analysis, the robustnesses of the descriptors are examined, and results show that the most accurate descriptors giving the lowest RMSE are made up of |ΔHf, ox/ΔEvac | multiplied by a parameter describing the property of surface metal or together with the adatom. This work explained the trends by intrinsic factors and provided a successful model for screening metal/support combinations that produce stable SACs against sintering. Besides, machine-learning has been used to predict the activity and selectivity of SACs. Guo et al. [Citation66] employed a feature set containing 8 intrinsic descriptors by machine-learning and found that the oxide formation enthalpy (Hf, ox) and the adjusted electron numbers of d/p orbital (edp’) show the highest feature importance of 0.59 and 0.20, respectively, in determining ΔG (O*) thus selectivity of certain two-dimensional SACs, as shown in ). Their predicted ΔG (O*) data by machine-learning versus DFT calculated data are plotted in ) [Citation66]. In addition, Lin et al. [Citation69] developed three models for ORR, OER, and HER, respectively, using machine-learning, which successfully predicted their limiting potentials on graphene-supported SACs. Similarly, Fung et al. [Citation70] identified Equationequation (15)(15)
(15) to accurately predict ΔGH on M-N-C-based SACs, which is a general descriptor for HER activity.
Figure 9. (a) One and (b) three-element-containing descriptors predicted versus DFT calculated binding energy between a metal adatom and its support. (Reprinted from Ref [Citation68]. Copyright Macmillan Publishers Limited, part of Springer Nature 2018). (c) Machine-learning predicted versus DFT computed values. (d) Feature importance of random forest model for
. ((c) and (d) are reprinted from Ref [Citation66]. Copyright American Chemical Society 2019)
![Figure 9. (a) One and (b) three-element-containing descriptors predicted versus DFT calculated binding energy between a metal adatom and its support. (Reprinted from Ref [Citation68]. Copyright Macmillan Publishers Limited, part of Springer Nature 2018). (c) Machine-learning predicted versus DFT computed ΔGO∗ values. (d) Feature importance of random forest model for ΔGO∗. ((c) and (d) are reprinted from Ref [Citation66]. Copyright American Chemical Society 2019)](/cms/asset/f5348da6-b2f2-4497-9859-1cc49ec0073c/tapx_a_1905545_f0009_oc.jpg)
4. Summary and outlook
In conclusion, SACs emerge as a new candidate that can cut down noble metal consumption in catalysis. They have shown exceptional performance for many important reactions, and their relatively simple structure makes them ideal models to discover the underlying mechanisms for catalysis. To reduce the time and economical-consuming work of complicated experiments, computational efforts have been paid to investigate the SACs. Density functional theory (DFT) calculations can reveal the properties of the active sites, and help to understand the exceptional performance of SACs. In addition, the catalytic performance including stability, activity, and selectivity of SACs can also be predicted by DFT calculations. Here, based on these DFT calculated information, we highlight the identification and usage of energy and structure descriptors to describe the performance of SACs which can guide the high-throughput screening of SACs. Energy descriptors are identified as a series of energy-related parameters that can well reflect the DFT calculated catalytic performance of SACs, which are more readily to be figured out than the whole reaction processes. Compared with energy descriptors, structure descriptors can directly relate the performance of SACs with intrinsic structural factors, making the properties of active sites more explicit and thus directly guide the rational design of SACs. Moreover, the advanced machine-learning methods have also been used for the identification of effective descriptors. Through machine-learning, effective functional forms can be readily constructed consisting of parameters selected from a large feature set. With the development of computational techniques, we are expecting the combined DFT and machine-learning methods will provide us with general strategies for identifying universal and effective structure descriptors to guide the rational design of SACs.
Apart from the effective usage in the design of SACs, computational methods are also expected to be of great help to the research progress of other atomic-scale dispersed catalysts, such as double atom catalysts (DACs), single cluster catalysts (SCCs), and so on [Citation71,Citation72]. For example, Guo et al. [Citation73] performed large-scale DFT calculations and obtained an activity map for NRR on bimetal catalysts supported by two-dimensional expanded phthalocyanine. The adsorption energy of N2H* was identified as a descriptor in the map, which has been used for the screening of promising bimetal catalysts. Besides, first-principles calculations have been applied for the demonstration of in situ dynamic generation of multinuclear sites from single atoms implicated as active sites in O2 activation [Citation74]. The dynamic formation of multinuclear sites is discovered as distinct from traditional heterogeneous catalysts, providing a more thorough understanding of the mechanisms of catalysis. With the rapid development of computational skills, it is expected that universal descriptors and computational methods will open new avenues for the rational design of catalysts in wider applications.
Acknowledgments
This work is supported by the National Natural Science Foundation of China (Grant Nos. 21822801 and 22078005), China Postdoctoral Science Foundation (2019TQ0021) and the Fundamental Research Funds for the Central Universities (XK1802-1 and XK180301).
Disclosure statement
No potential conflict of interest was reported by the authors.
Additional information
Funding
References
- Li X, Yang X, Huang Y, et al. Supported noble-metal single atoms for heterogeneous catalysis. Adv Mater. 2019;31:e1902031.
- Han J, Lu J, Wang M, et al. Single atom alloy preparation and applications in heterogeneous catalysis. Chin J Chem. 2019;37:977.
- Qiao B, Wang A, Yang X, et al. Single-atom catalysis of CO oxidation using Pt1/FeOx. Nat Chem. 2011;3:634.
- Wang A, Li J, Zhang T. Heterogeneous single-atom catalysis. Nat Rev Chem. 2018;2:65.
- Li Y. Single-atom catalysts: the role of intrinsic intermediate. Green Energy Environ. 2020;5:4.
- Li Y, Wu ZS, Lu P, et al. High-valence nickel single-atom catalysts coordinated to oxygen sites for extraordinarily activating oxygen evolution reaction. Adv Sci (Weinh). 2020;7:1903089.
- Fei H, Dong J, Feng Y, et al. General synthesis and definitive structural identification of MN4C4 single-atom catalysts with tunable electrocatalytic activities. Nat Catal. 2018;1:63.
- Chen Z, Vorobyeva E, Mitchell S, et al. A heterogeneous single-atom palladium catalyst surpassing homogeneous systems for Suzuki coupling. Nat Nanotechnol. 2018;13:702.
- Nie L, Mei D, Xiong H, et al. Activation of surface lattice oxygen in single-atom Pt/CeO2 for low-temperature CO oxidation. Science. 2017;358:1419.
- Shin J, Lee YJ, Jan A, et al. Highly active and thermally stable single-atom catalysts for high-temperature electrochemical devices. Energy Environ Sci. 2020;13:4903.
- Gao H. CO oxidation mechanism on the γ-Al2O3 supported single Pt atom: first principle study. Appl Surf Sci. 2016;379:347.
- Hao Z, Guo S, Guo L. Mechanisms investigation of the WGSR catalyzed by single noble metal atoms supported on vanadium oxide clusters. Appl Organomet Chem. 2019;33:e4960.
- Wu L, Guo T, Li T. Rational design of transition metal single-atom electrocatalysts: a simulation-based, machine learning-accelerated study. J Mater Chem A. 2020;8:19290.
- Ling C, Ouyang Y, Li Q, et al. A general two-step strategy–based high-throughput screening of single atom catalysts for nitrogen fixation. Small Methods. 2018;3:1800376.
- Yang S, Kim J, Tak YJ, et al. Single-atom catalyst of platinum supported on titanium nitride for selective electrochemical reactions. Angew Chem Int Ed Eng. 2016;55:2058.
- Tan K, Dixit M, Dean J, et al. Predicting metal–support interactions in oxide-supported single-atom catalysts. Ind Eng Chem Res. 2019;58:20236.
- Norskov JK, Bligaard T, Rossmeisl J, et al. Towards the computational design of solid catalysts. Nat Chem. 2009;1:37.
- Qiu HJ, Ito Y, Cong W, et al. Nanoporous graphene with single-atom nickel dopants: an efficient and stable catalyst for electrochemical hydrogen production. Angew Chem Int Ed Eng. 2015;54:14031.
- Daelman N, Capdevila-Cortada M, Lopez N. Dynamic charge and oxidation state of Pt/CeO2 single-atom catalysts. Nat Mater. 2019;18:1215.
- Cheng N, Stambula S, Wang D, et al. Platinum single-atom and cluster catalysis of the hydrogen evolution reaction. Nat Commun. 2016;7:13638.
- Ren Y, Tang Y, Zhang L, et al. Unraveling the coordination structure-performance relationship in Pt1/Fe2O3 single-atom catalyst. Nat Commun. 2019;10:4500.
- Wang Y, Song E, Qiu W, et al. Recent progress in theoretical and computational investigations of structural stability and activity of single-atom electrocatalysts. Prog Nat Sci. 2019;29:256.
- Pan C-J, Tsai M-C, Su W-N, et al. Tuning/exploiting Strong Metal-Support Interaction (SMSI) in Heterogeneous Catalysis. J Taiwan Inst Chem Eng. 2017;74:154.
- Qiao B, Liang J, Wang A, et al. Ultrastable single-atom gold catalysts with strong covalent metal-support interaction (CMSI). Nano Res. 2015;8:2913.
- Greiner MT, Jones TE, Beeg S, et al. Free-atom-like d states in single-atom alloy catalysts. Nat Chem. 2018;10:1008.
- Liu J, Tang Y, Wang Y, et al. Theoretical understanding of the stability of single-atom catalysts. Natl Sci Rev. 2018;5:638.
- Xu H, Cheng D, Cao D, et al. A universal principle for a rational design of single-atom electrocatalysts. Nat Catal. 2018;1:339.
- Liu JC, Wang YG, Li J. Toward rational design of oxide-supported single-atom catalysts: atomic dispersion of gold on Ceria. J Am Chem Soc. 2017;139:6190.
- Yang W, Zhao M, Ding X, et al. The effect of coordination environment on the kinetic and thermodynamic stability of single-atom iron catalysts. Phys Chem Chem Phys. 2020;22:3983.
- Li F, Li Y, Zeng XC, et al. Exploration of high-performance single-atom catalysts on support M1/FeOx for CO oxidation via computational study. ACS Catal. 2014;5:544.
- Montalenti F, Ferrando R. Jumps and concerted moves in Cu, Ag, and Au(110) adatom self-diffusion. Phys Rev B. 1998;59:5881.
- Xu H, Cheng D. First-principles-aided thermodynamic modeling of transition-metal heterogeneous catalysts: a review. Green Energy Environ. 2020;5:286.
- Liu JC, Xiao H, Li J, et al. Constructing high-loading single-atom/cluster catalysts via an electrochemical potential window strategy. Chem Soc. 2020;142:3375.
- Song W, Hensen EJM. Mechanistic aspects of the water–gas shift reaction on isolated and clustered au atoms on CeO2(110): a density functional theory study. ACS Catal. 2014;4:1885.
- Lin R, Albani D, Fako E, et al. Design of single gold atoms on nitrogen-doped carbon for molecular recognition in alkyne semi-hydrogenation. Angew Chem Int Ed Eng. 2019;58:504.
- Zhang H, Li J, Xi S, et al. A graphene-supported single-atom FeN5 catalytic site for efficient electrochemical CO2Reduction. Angew Chem Int Ed Eng. 2019;58:14871.
- Li J, Zhong L, Tong L, et al. Atomic Pd on graphdiyne/graphene heterostructure as efficient catalyst for aromatic nitroreduction. Adv Funct Mater. 2019;29:1905423.
- Borrome M, Gronert S. Gas-phase dehydrogenation of alkanes: c−H activation by a graphene-supported nickel single-atom catalyst model. Angew Chem Int Ed Eng. 2019;58:14906.
- Wu W, Cui E, Zhang Y, et al. Involving single-atom silver(0) in selective dehalogenation by AgF under visible-light irradiation. ACS Catal. 2019;9:6335.
- Wang YG, Cantu DC, Lee MS, et al. CO oxidation on Au/TiO2: condition-dependent active sites and mechanistic pathways. J Am Chem Soc. 2016;138:10467.
- Clay JP, Greeley JP, Ribeiro FH, et al. DFT comparison of intrinsic WGS kinetics over Pd and Pt. J Catal. 2014;320:106.
- Ammal SC, Heyden A. Water-gas shift activity of atomically dispersed cationic platinum versus metallic platinum clusters on titania supports. ACS Catal. 2016;7:301.
- Li Q, Ma Z, Sa R, et al. Computation-predicted, stable, and inexpensive single-atom nanocatalyst Pt@Mo2 C – an important advanced material for H2 production. J Mater Chem A. 2017;5:14658.
- Jing H, Li Q, Wang J, et al. Theoretical study of the reverse water gas shift reaction on copper modified β-Mo2 C(001) surfaces. J Phys Chem C. 2018;123:1235.
- Xu H, Xu C-Q, Cheng D, et al. Identification of activity trends for CO oxidation on supported transition-metal single-atom catalysts. Catal Sci Technol. 2017;7:5860.
- Wang H, Liu JX, Allard LF, et al. Surpassing the single-atom catalytic activity limit through paired Pt-O-Pt ensemble built from isolated Pt1 atoms. Nat Commun. 2019;10:3808.
- Guo X, Huang S. Tuning nitrogen reduction reaction activity via controllable Fe magnetic moment: a computational study of single Fe atom supported on defective graphene. Electrochim Acta. 2018;284:392.
- Koper MTM. Analysis of electrocatalytic reaction schemes: distinction between rate-determining and potential-determining steps. J Solid State Electrochem. 2012;17:339.
- Zhao J, Chen Z. Single Mo atom supported on defective boron nitride monolayer as an efficient electrocatalyst for nitrogen fixation: a computational study. J Am Chem Soc. 2017;139:12480.
- He T, Zhang C, Du A. Single-atom supported on graphene grain boundary as an efficient electrocatalyst for hydrogen evolution reaction. Chem Eng Sci. 2019;194:58.
- Chen W, Pei J, He CT, et al. Rational design of single molybdenum atoms anchored on N-doped carbon for effective hydrogen evolution reaction. Angew Chem Int Ed Eng. 2017;56:16086.
- Gao G, Bottle S, Du A. Understanding the activity and selectivity of single atom catalysts for hydrogen and oxygen evolution via ab initial study. Catal Sci Technol. 2018;8:996.
- Hossain MD, Liu Z, Zhuang M, et al. Rational design of graphene-supported single atom catalysts for hydrogen evolution reaction. Adv Energy Mater. 2019;9:1803689.
- Hwang J, Noh SH, Han B. Design of active bifunctional electrocatalysts using single atom doped transition metal dichalcogenides. Appl Surf Sci. 2019;471:545.
- Choi C, Back S, Kim N-Y, et al. Suppression of hydrogen evolution reaction in electrochemical N2 reduction using single-atom catalysts: a computational guideline. ACS Catal. 2018;8:7517.
- Zhou HY, Li JC, Wen Z, et al. Tuning the catalytic activity of a single Mo atom supported on graphene for nitrogen reduction via Se atom doping. Phys Chem Chem Phys. 2019;21:14583.
- Peng Y, Geng Z, Zhao S, et al. Pt single atoms embedded in the surface of Ni nanocrystals as highly active catalysts for selective hydrogenation of nitro compounds. Nano Lett. 2018;18:3785.
- Yang L, Cheng D, Xu H, et al. Unveiling the high-activity origin of single-atom iron catalysts for oxygen reduction reaction. Proc Natl Acad Sci USA. 2018;115:6626.
- Chen Z, Zhao J, Cabrera CR, et al. Computational screening of efficient single-atom catalysts based on graphitic carbon nitride (g-C3N4) for nitrogen electroreduction. Small Methods. 2018;3:1800368.
- Cui Q, Qin G, Wang W, et al. Novel two-dimensional MOF as a promising single-atom electrocatalyst for CO2 reduction: a theoretical study. Appl Surf Sci. 2020;500:143993.
- Liu X, Jiao Y, Zheng Y, et al. Building up a picture of the electrocatalytic nitrogen reduction activity of transition metal single-atom catalysts. J Am Chem Soc. 2019;141:9664.
- Bligaard T, Nørskov JK, Dahl S, et al. The Brønsted–Evans–Polanyi relation and the volcano curve in heterogeneous catalysis. J Catal. 2004;224:206.
- Kim HY, Lee HM, Pala RGS, et al. CO oxidation by Rutile TiO2 (110) Doped with V, W, Cr, Mo, and Mn. J Phys Chem C. 2008;112:12398.
- Gong L, Zhang D, Lin CY, et al. Catalytic mechanisms and design principles for single-atom catalysts in highly efficient CO2 conversion. Adv Energy Mater. 2019;9:1902625.
- Yang W, Xu S, Ma K, et al. Geometric structures, electronic characteristics, stabilities, catalytic activities, and descriptors of graphene-based single-atom catalysts. Nano Mater Sci. 2020;2:120.
- Guo X, Lin S, Gu J, et al. Simultaneously achieving high activity and selectivity toward two-electron O2 electroreduction: the power of single-atom catalysts. ACS Catal. 2019;9:11042.
- Kitchin JR. Machine learning in catalysis. Nat Catal. 2018;1:230.
- O’Connor NJ, Jonayat ASM, Janik MJ, et al. Interaction trends between single metal atoms and oxide supports identified with density functional theory and statistical learning. Nat Catal. 2018;1:531.
- Lin S, Xu H, Wang Y, et al. Directly predicting limiting potentials from easily obtainable physical properties of graphene-supported single-atom electrocatalysts by machine learning. J Mater Chem A. 2020;8:5663.
- Fung V, Hu G, Wu Z, et al. Descriptors for hydrogen evolution on single atom catalysts in nitrogen-doped graphene. J Phys Chem C. 2020;124:19571.
- Cao Y, Zhao J, Zhong X, et al. Building highly active hybrid double-atom sites in C2N for enhanced electrocatalytic hydrogen peroxide synthesis. Green Energy Environ. (In press, DOI: https://doi.org/10.1016/j.gee.2020.12.006).
- Liu JC, Ma XL, Li Y, et al. Heterogeneous Fe3 single-cluster catalyst for ammonia synthesis via an associative mechanism. Nat Commun. 2018;9:1610.
- Guo X, Gu J, Lin S, et al. Tackling the activity and selectivity challenges of electrocatalysts toward the nitrogen reduction reaction via atomically dispersed biatom catalysts. J Am Chem Soc. 2020;142:5709.
- Paolucci IKC, Parekh AA, J. shih A, et al. Dynamic multinuclear sites formed by mobilized copper ions in NOx selective catalytic reduction. Science. 2017;357:898.