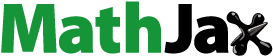
ABSTRACT
We explore the determinants of broadcasting demand for Formula One racing in the United States of America and pay specific attention to the relationship between outcome uncertainty and television viewership figures. Using implied probabilities derived from betting odds, we offer an approach to measuring outcome uncertainty that differs from those currently established in the Formula One broadcasting demand literature. We do not find any evidence to support the uncertainty of outcome hypothesis. On the contrary, viewers display a preference for less closely contested races. We find that scheduling and accessibility to live races are important determinants of viewership. Our results have practical implications and can guide the sport's administrators and television broadcasters seeking to understand a growing national market for F1 broadcasting.
KEYWORDS:
1. Rationale/Purpose
While sports economists have traditionally focused their research on popular team sports such as baseball, basketball and European football, studies considering fan interest in attending and viewing other sports is increasing over time. One such sport that has begun to appeal to researchers in this area is Formula One (F1), with the elite motorsport attracting increasing interest from sports economics and management scholars (e.g. Baecker et al., Citation2022; Budzinski & Feddersen, Citation2020; Garcia-del-Barrio & Reade, Citation2022; Gasparetto et al., Citation2022; Peeters & Wesselbaum, Citation2023; Schreyer & Torgler, Citation2018). Consistent with the wider body of literature considering demand to view live sport, these works have focused on empirically testing the uncertainty of outcome hypothesis – a theoretical cornerstone of the sports economics literature since the contributions of Rottenberg (Citation1956) and Neale (Citation1964).
As the rapid evolution of sports broadcasting continues (see Baecker et al., Citation2022; Buraimo et al., Citation2020, Citation2022; Butler & Massey, Citation2019; Butler & Butler, Citation2023; Butler et al., Citation2022), understanding the motivations of armchair spectators to watch content by appointment has garnered increased attention. Traditionally, most pursuits to model viewer figures concentrated on team sports, but attention has increasingly turned to evaluating the role of outcome uncertainty in individual sports such as tennis (Konjer et al., Citation2017) and hybrid individual-group sports where there is a strong team component such as motor sports and cycling (Larson & Maxcy, Citation2014 Van Reeth, Citation2013). In short, there is mixed empirical support for the outcome uncertainty hypothesis in attendance and viewership data at the individual contest level, with the literature now also considering wider behavioural motivations to consume sport (Buraimo et al., Citation2020; Coates et al., Citation2014; Pawlowski et al., Citation2018).
Our research is nested within the branch of literature studying demand for individual sport and is timely for two reasons. We explore the motivations of fans in F1 for one of its largest national markets for sports broadcasting – the United States of America (USA). The determinants of demand in this market are not well understood in the academic literature. With these viewership data we consider the uncertainty of outcome hypothesis and ask what are the determinants of demand for F1 telecasts in the USA? Specifically, we measure the uncertainty of an outcome in four different ways: (i) the difference in betting odds between drivers, (ii) points differences in the World Drivers’ Championship (WDC) and Constructors’ World Championship (CWC) at any point during the season, (iii) the difference in seconds between the first and third qualified driver and (iv) the difference in time between the first and fifth qualified driver. These different methods offer market and sporting-based measures of uncertainty, between elite drivers and constructors. These are explained in detail in the next section.
While this paper is not the first to model F1 viewership figures, we contribute to the direct demand research for the sport in two specific ways. First, we use a hand-collected dataset from bookmakers to measure driver winning likelihoods. Betting on F1 Grand Prix’s has proved to be a niche pursuit to date, and no F1 research has utilised market (betting) odds probabilities to consider uncertainty of outcome and fan demand. The use of odds to quantity contest balance is a first-rate metric to capture contest outcome uncertainty in the wider literature on sports demand and our use of these measures can augment our understanding of preferences for uncertainty in F1.
Second, we introduce new viewership data derived from USA audiences. As suggested, this is a critical market for the sport and to our knowledge has yet to be studied. Presently, the bulk of F1 demand research appeals to viewership data from German markets (e.g. Baecker et al., Citation2022; Schreyer & Torgler, Citation2018). This is not surprising given the European origins of the sport and access to rich data from German broadcasters. Likely due to cultural and historic factors, research considering demand for motor sports in the USA has tended to focus on National Association for Stock Car Auto Racing (NASCAR)Footnote1 (Berkowitz et al., Citation2011). Again, it is understandable that the general focus in the USA concerns this format of car racing, rather than the European born F1. However, the USA first held a Grand Prix under the current organisational structure, as early as 1959 and has held 18 races since the start of the twenty-first century. The American market continues to grow, with F1 receiving increased viewership and now seeking an estimated $75 million per year for media rights (Ourand, Citation2022). Therefore, understanding the determinants of demand in this market is deserving of attention.
Our tests not only make an important empirical contribution but also a practical one. The past decade has witnessed an unprecedented concentration of wins in the hands of a limited number of F1 drivers and constructors. Since 2010 just four drivers (Sebastian Vettel, Lewis Hamilton, Nico Rosberg and Max Verstappen) have been crowned winners of the WDC, with just two constructors (Red Bull and Mercedes) winning the CWC in the same period. At no other point in the history of F1 have the WDC and CWC been so concentrated. For example, during the decade of the 1970s, seven different drivers won the WDC title, a further six drivers won the WDC title during the decade of the 1980s, and seven different drivers held the WDC title at some point during the 1990s. While the winning constructor has traditionally been quite concentrated, the reduction in the number of successful drivers over more than the past decade is unprecedented in the history of F1. Budzinski and Feddersen (Citation2020) document this decline in competitive balance, an observation supported by a Herfindahl-Hirschman (HHI) concentration index which reports a HHI of 0.209 for the 2020 season, compared to 0.109 in 2014 and just 0.098 in 2010. As such, this research can offer insight for sports business and F1 administrators tasked with designing rules for the sport and ensuring that a reasonable level of competitive balance exists both within races and within championships.
Past F1 research, insofar as it concerns direct demand, has focused on two related areas: uncertainty of outcome and competitive balance. Kipker (Citation2003), Krauskopf et al. (Citation2010), and most recently Gasparetto et al. (Citation2022) and Baecker et al. (Citation2022) examine uncertainty of outcome. These works have applied sporting-level metrics to capture contest uncertainty. Kipker (Citation2003) measures uncertainty of outcome using the points difference between the first and last placed driver in the WDC table. Krauskopf et al. (Citation2010) proxy outcome uncertainty in a race setting through a concentration index based on Championship points. Both report an unclear relationship between uncertainty of outcome and viewership demand and suggest that there appears to be lower levels of demand when uncertainty of outcome is both too high and too low. Gasparetto et al. (Citation2022) consider a similar strand of the direct demand literature by focusing on race attendance and uncertainty of outcome. The authors find that demand for F1 is a normal good – increasing ticket prices reduces attendance – and that race uncertainty and attendance is non-linear (U-shaped). The performance of a domestic driver at their home Grand Prix has a significant and positive impact on race attendance.
Another strand of F1 demand research considers wider issues of competitive balance within the sport. Mastromarco and Runkel (Citation2009) suggest rule changes normally occur when competitive balance is in decline. The authors note that rule changes primarily intended to increase safety standards in the sport can exert a significant positive impact on competitive balance. Judde et al. (Citation2013) contend that regulatory changes increase outcome uncertainty. Mourão (Citation2017) finds the outcome uncertainty is higher as one goes back in time, a finding that may explain why rule changes are required if the intention is to improve competitive balance. Schreyer and Torgler (Citation2018) report that fans prefer some level of competition between the top drivers, and that race organisers need to ensure that competitive balance remains high to attract strong television audience numbers.
Uncertainty of outcome has continued to attract interest in the most current research and has moved in new directions. Most recently Garcia-del-Barrio and Reade (Citation2022) explore uncertainty of outcome using Google trend indexes. They find that search volumes significantly decline once pennant-race uncertainty is removed however the authors do note that this finding is subject to their model specification. Close to this study, Baecker et al. (Citation2022) consider F1 viewer demand under different TV channels holding non-exclusive media rights, finding that outcome uncertainty can differ between premium or non-premium mediums. The authors contend that gender effects can explain this.
This paper continues as follows. The next section presents the research method. It explains where data is sourced from and how the empirical estimations are conducted. Descriptive statistics on selected variables are also presented. Section 3 presents our results and findings. In total, eight estimations are run across four different measures of uncertainty of outcome. Section 4 discusses the implications of our work considering the general findings. We offer thoughts on what we believe is driving these results and suggest policy advice for race organisers and broadcasters of F1 races seeking to increase viewership in the USA. Section 5 concludes the paper.
2. Design/Methodology/approach
We explore the uncertainty of outcome hypothesis and the factors associated with broadcasting demand in the USA market between 2016 and 2020. Our dataset includes 81 F1 Grand Prix races from round eleven of the 2016 season to the final round of the 2020 season, broadcasted in the USA across the ESPN, NBC, CNBC, and ABC networks. While the 2020 season was impacted by Covid-19, the July 2020 United States Grand Prix, along with races in Brazil, Canada and Mexico, were all cancelled due to rising numbers of Covid-19 infections and restrictions in the Americas. Therefore, the estimations that follow are not affected by a possible substitution of fans who normally attend the USA Grand Prix in-person watching on their televisions, as no race was held in 2020. This time-period is also selected due to constraints imposed by access to viewer data and gambling odds. Nine Grand Prix races are excluded as they were not broadcast on television in the USA or viewership data was unavailable for these races. The dependent variable – viewership figures – is obtained from showbuzzdaily.com where all USA sports broadcasts’ viewership figures are uploaded on a weekly basis. Data is collected manually, with the viewership and network broadcaster of each Grand Prix recorded for all 81 races. It is our understanding that this viewership data relates to cable and satellite television viewership only for all persons aged three years and older. No race was sold on a pay-per-view basis. Summary statistics on viewership are presented in .
Table 1. Descriptive statistics.
2.1 Measuring uncertainty of outcome
Four measures of uncertainty of outcome are considered. The first – and most novel measure – is the difference in betting odds (DIBO) of F1 drivers placed in positions 1–5 on the starting grid for each Grand Prix. To calculate this, we infer probabilities from the odds. Given that little is known about the overall book value we cannot adjust for the overround. As per the traditional outcome uncertainty hypothesis, one could expect a negative relationship between increasing differences in adjusted betting odds and viewer demand. We obtain betting odds from a variety of sources online including metro.co.uk (2016), bleacherreport.com (2018), sportsbettingdime.com (2019) and paddypower.com (2020). We have no reason to believe there are significant variations in odds across providers. The average odds for the driver in pole position are 1.96 (2016), 2.26 (2017 and 2018), 2.30 (2019) and 1.58 (2020). Typically, there is a clear favourite for each race. Lower average odds for the car starting first on the grid suggest a less competitive World Championship for both drivers and constructors.
The second measure of uncertainty of outcome is the points difference in WDC and CWC at any point during the season. This is captured by a running total of the drivers and constructors’ championship points. Two methods have been created to capture this. DRIVERPTSDIFF measures the difference in points between the first placed driver and the tenth placed driver in the WDC at the start of any given Grand Prix. Similarly, CONSTRUCTORPTSDIFF captures the difference between the first and the third placed constructor in the CWC. Therefore, while the difference in betting odds allows us to measure for race-specific competitive balance from race-to-race, the variations of our points difference measures attempt to explain season-long competitive balance as these are a running total until the final weekend of the season.
The third and fourth measures of outcome uncertainty are inspired by the work of Schreyer and Torgler (Citation2018) and allow us to check the robustness of our first two measures of uncertainty of outcome. The authors quantify uncertainty of outcome through the difference in seconds between the first and third qualified driver (DQT3) and the difference in time between the first and fifth qualified driver (DQT5). The closer the time difference between the top three/ five qualifying drivers, the higher the level of uncertainty of outcome.
2.2 Control variables
Various controls are included in our model. Race-specific factors include the number of Grand Prix races that have been held at a track to capture tradition as a motivation to view (HERITAGE), the number of turns (TURNS), race distance (RACEDISTANCE), drag-reduction-system zones (DRSZONES), highest recorded speed in kilometres per hour (MAXKPH) and grid-to-turn-one-distance (GRID). These additional measures are aimed at capturing the appealing aspects of alternative tracks. In addition, we include race location fixed effects to account for unexplained heterogeneity. We obtained all race data from formula1.com.
Various non-race factors can also explain audience demand. Television ratings (TVR) for the broadcast prior to the live Grand Prix is recorded. We also include a control for broadcasts near a public holiday (PH) (within one day before or after), with the prior expectation being that viewership increases if a Grand Prix is broadcasted the day before or after a USA public holiday. The start time of the broadcast is captured as the Programme Start Time (PST). A similar timing effect is expected, particularly in the case of European and Oceanic races with fans in the USA potentially less likely to watch broadcasts at unsociable hours of the day. Dummy variables are employed to capture the effect, if any, that major sport substitutes have on viewership figures of F1 broadcasts.
Table 2. Television demand with alternative uncertainty of outcome measures.
We incorporate a vector of dummy variables for motor sport broadcasts at the same time as the F1 broadcast (MOTORSPORTDIRECT), and motorsport earlier or later in the day of the F1 broadcast (MOTORSPORTDAILY). Additionally, we control for competing broadcasts including the English Premier League (EPL), Tour De France (TDF), Major League Baseball (MLB), Tennis (ATP), Golf (PGA), and the National Football League (NFL). Finally, we include dummy variables (SEASONAL) and (SEASONALCONS) to capture the point in the season at which the WDC and CWC are won.
2.3 Empirical model
Due to the skewness of the dependent variable, we estimate a Huber regression. One of the key benefits of using this regression is that it allows for a trade-off between robustness to outliers and efficiency. Considering the distribution of our viewer data, this approach allows us to mitigate the influence of extreme observations. We also include location-fixed effects in each model. The regression models employed are as follows:
(1)
(1)
(2)
(2)
(3)
(3)
(4)
(4) where i is the F1 race and
is a vector of controls for pre-race and within-races factors as previously listed.
Equation (1) explores the relationship between viewership figures using odds (DIBO) as the measure of race uncertainty of outcome. Equation (2)–(4) alternate the measure of uncertainty of outcome with the same control variables. Equation (2) uses both DRIVERPTSDIFF and CONSTRUCTORPTSDIFF. Equations (3) and (4) employ DQT3 and DQT5, as used by Schreyer and Torgler (Citation2018). Both are found to be useful predictors of Grand Prix demand in Germany. However, in the context of this research their use acts as a check against both the DIBO and points difference measures of outcome uncertainty. As betting odds are widely seen as the gold standard of outcome uncertainty measures in sports demand literature, we believe this is a useful addition to measuring competitive balance in F1. Additionally, DIBO allows us to measure for race-specific competitive balance that changes from race to race, whereas the points difference measures account for season long competitive balance as they are a running total until the final weekend of the season.
3. Findings
presents the empirical results for the first sets of estimations, utilising four different measures of uncertainty of outcome. Column 1 analyses the effect of viewer demand for using the odds probability measure of uncertainty of outcome. DIBO is statistically significant. An additional 176,890 viewers can be expected for every extra unit increase in the difference in betting odds between the first and fifth qualified driver. We also explore this effect using drivers one to four only. Again, a statistically significant effect is observed at the 1% confidence level, with more than 190,000 additional viewers. This finding is contrary to expectations and indicates that fans do not appear to be overly concerned about a lack of uncertainty of outcome. This could be because some fans in the USA are passionate about F1, uncertainty of outcome in the sport is not, or less of, a concern to viewers. This finding differs from the most recent research on German markets (Baecker et al., Citation2022). This is understandable given the different market we examine, and it could be explained by a variety of cultural and historic reasons beyond the scope of this research. However, finding a preference for dominance among fans is not uncommon in the general literature, with mixed evidence when it comes to empirical applications of the uncertainty of outcome hypothesis.
Table 3. Television demand excluding Monaco Grand Prix.
In line with Schreyer and Torgler (Citation2018), various race-specific controls are significant when explaining viewership figures. Although it has a small effect on viewership, when compared with other variables, track characteristics play an important role in shaping the demand for F1 broadcasts in the USA. Channels that require subscription access to races attract significantly fewer viewers. This is consistent with expectations. Despite being a free-to-air broadcaster, ABC does not attract significantly more viewers across three of the four models. It is clear from these results that US F1 fans prefer Grand Prixs that start later in the day, as opposed to those broadcasts at unsociable hours. This differs from a non-linear scheduling effect associated with the German market (Baecker et al., Citation2022).
Column 2 uses the Points Difference model to measure uncertainty of outcome. This model has two alternative explanatory variables with DRIVERPTSDIFF and CONSTRUCTORPTSDIFF representing both the continued changes in the WDC and CWC. The results in model 2 largely align with model 1. Both DRIVERPTSDIFFELITE and CONSTRUCTORPTSDIFFELITE are statistically significant, but the coefficient magnitudes suggest the increase or decrease in viewership is marginal.
As a check, both measures of uncertainty of outcome were tested under different parameters. Instead of the difference between the first-placed driver and the tenth-placed driver in the WDC at the start of any given Grand Prix (DRIVERPTSDIFF), we estimated the model using the difference between the first and twentieth-placed driver in the WDC at the start of any given Grand Prix. Similarly, with CONSTRUCTORPTSDIFF, rather than the difference between the first and the third-placed constructor in the CWC, we estimated the difference between the first and fifth-placed team. The original results are robust to these alternative measures.
Column 3 presents the DQT3 model. DQT3 is not statistically significant. This finding is not surprising and, while not corroborating previous research, this variable is introduced to a new market with a unique history and relationship with F1. Column 4 presents the DQT5 model. Like the three prior models, most of the channel variables remain significant. DQT5, like DQT3, is not statistically significant.
While our findings concerning outcome uncertainty are mixed, being dependent upon measurement, fans don’t appear to display a preference for a more competitive race. This is in line with the findings of Gooding and Stephenson (Citation2017) and Kang et al. (Citation2018). Our findings are different to Dang et al. (Citation2015) and Mizak et al. (Citation2007) who both report a preference for greater outcome uncertainty to increase sports viewership. However, we propose that our use of betting odds offers an improved measure of balance.
The lack of statistical significance of the outcome uncertainty measures DQT3 and DQT5 across the models is important in the wider context. Running four different uncertainty of outcome models, each with different outcome uncertainty variables, highlights the effectiveness of each outcome uncertainty measure. The significance of the DIBO and both points difference models when compared to DQT3 and DQT5, suggests they may be superior measures of outcome uncertainty and should be used by others continuing in this line of research. Despite the growing depth of sports economics literature, the lesser explored field of F1 deserves further attention given its appeal and paucity of work sports economics work to date.
To test the robustness of these results, we removed the three Monaco Grand Prix races from the dataset. This race is unique and consistently posts the highest rating and viewership figures within this dataset. The results are presented in . The removal of Monaco does impact the results. ESPN, ABC, RACEDISTANCE and WEATHER change significantly, and can be attributed to the difference in viewership between the Monaco Grand Prix and less popular races. Importantly however, all four measures of uncertainty of outcome remain robust to the removal of the Monaco Grand Prix, across columns 5–8 in . The robustness of the positive coefficient across both models suggests that the DIBO and points difference models capture the die-hard US fans that are willing to tune into a race broadcast regardless of increasing differences in betting odds across the top five drivers.
4. Practical implications
Our results add to the literature on direct demand for F1 by exploring a growing and important market. The results allow us to offer new insights to the governing body of the sport and sports broadcasters, aiming to capture viewers in the USA market. Our novel use of betting odds – which has not been used to date – suggests that armchair fans prefer less competitive races. This is not overly surprising and as mentioned at the outset, the past decade or more has witnessed a concentration of championship wins – both drivers and constructors – in the hands of a very small group. Fans are tuning in to watch drivers and constructors dominating races, rather than watching races where the likely winner is more difficult to predict. Of course, viewers could be watching the race in the hope of a shock result. The present supremacy of Red Bull and Mercedes, and that of drivers such as Lewis Hamilton, and more recently Max Verstappen, should not be of major concern to race organisers and administrators seeking to increase competitive balance and, by extension, outcome uncertainty. It is of note that this finding is dependent on our measurement of outcome uncertainty. However, none of the four measures adopted imply an association between greater balance and significant increases in television audiences.
Our results regarding the level of uncertainty of outcome differ across models and deviate from past literature. Albeit we study a different market, we believe this difference highlights the importance of using betting odds – which are commonly accepted to be one of the most appropriate ways to quantify how competitive a contest is – to measure race outcome uncertainty. Traditionally, administrators within the sport have initiated rule changes when one driver or constructor was seen to be creating a position of dominance or competitive balance was being eroded. The most obvious example of this started in 2010 when dramatic cost-cutting measures were introduced by the FIA to allow smaller teams to compete. These rules were extended further at the start of the 2013 season, and at the start of the 2021 campaign, the governing body introduced a budget cap of $140 million per season. There are obvious sporting reasons for such rule changes, but our results suggest that in the short to medium run, an erosion of outcome uncertainty did little to effect viewership. In fact, the opposite is found when measuring this using betting odds (DIBO). Therefore, the governing body could not appeal to American viewership demand to justify rules to improve competitive balance. Rule changes could be motivated by other reasons, mainly those related to sporting competition, and the need to ensure constructors remain interested in competing in the sport in the long run.
We show across most of the models that other motor sport broadcasts on the day of the race – once not in direct competition with the screening – stimulate demand. These appear to be complimentary to what happens later at the racetrack and race organisers and broadcasters should keep this in mind when races are scheduled to take place. The timing of these races is important and needs to be considered. Grand Prix’s that start at unsociable times for the audience in the USA result, as expected, in significantly fewer viewers. Some races take place in countries with significantly different time zones to the USA, such as Australia, Japan, and Singapore, but race organisers could consider time schedules for European Grand Prix that are more amenable to the large American audience. Given the long summer days in Europe, late afternoon starts would not necessarily change race dynamics and could attract a greater audience in the USA. Generally, we find longer races are associated with fewer viewers – they may become tedious if the race forms a stable pattern, with little overtaking or incident. It may be the case that fans are uninterested in races that last too long. However, while this could be taken into consideration by race organisers, the findings are not robust to the exclusion of the Monaco Grand Prix.
Our research has several limitations. Future studies would benefit from a larger dataset of Grand Prix races and betting odds, which includes viewers on mobile devices or those who can stream Grand Prixs. This would give further insights to administrators and broadcasters alike. Furthermore, complementary products could be stimulating demand. For example, numerous documentaries, such as the popular Netflix show Drive to Survive, have been released in the recent past, directing significantly more attention towards the sport. This could be attracting casual fans, or those who have never watched F1 previously, to watch a Grand Prix when it is live on television. Future studies could benefit greatly from considering how indirect channels impact demand and quantifying how external events (e.g. docuseries and esports) affect Grand Prix viewership. A significant competition change was also initiated in the 2021 season, which saw the addition of sprint races as a form of qualifying across three Grand Prixs. The rule change was instigated to increase interest in the sport and has had a ripple effect – qualifying moved from Saturday to Friday. Future studies would benefit from investigating the effect that an additional race across the weekend can have on viewership.
5. Research contribution
This paper uses various measures of uncertainty of outcome in F1 to understand the determinants of demand for television viewership in the USA over four seasons. Significantly, we introduce two new measures that have yet to be used in the F1 demand literature to measure race uncertainty of outcome – betting data that essentially offers a market-generated prediction of the outcome.
We find that the behaviour of American television fans does not support the uncertainty of outcome hypothesis when using our novel approach. Rather, fans have no problem watching races where the likely winner is strongly predicted by the market in advance of the race. Fan loyalty to the sport or motivations to watch the spectacle of these elite cars and drivers appear to be enough for fans to watch live broadcasts. Several other variables in our model prove to be important determinants of demand and remain robust across different estimations. The type of broadcasting channels (free-to-air or subscription), the race distance and the start time of a race prove to be the most consistent and robust determinants of viewer demand. When removing the Monaco Grand Prix, our results are largely consistent with earlier findings and we continue to report that viewers prefer to watch races with a dominant driver and team. These findings are important for race organisers considering instigating rule changes in the sport, and broadcasters seeking to maximise viewership demand.
Disclosure statement
No potential conflict of interest was reported by the author(s).
Notes
1 NASCAR was founded in the United States in 1948 and is headquartered in Daytona Beach, Florida, sanctioning more than 1,500 races annually mainly in the USA (see Abere et al., Citation2012 for the economics of NASCAR).
References
- Abere, A., Bronsteen, P., & Elzinga, K. G. (2012). The Oxford handbook of sports economics. The Oxford Handbook of Sports Economics Volume 1: The Economics of Sports, 1, 318. https://doi.org/10.1093/oxfordhb/9780195387773.013.0017
- Baecker, N., Ansari, P., & Schreyer, D. (2022). Formula 1 Grands Prix demand across different distribution channels. Managing Sport and Leisure, 1–14. https://doi.org/10.1080/23750472.2022.2115395
- Berkowitz, J. P., Depken, C. A., & Wilson, D. P. (2011). When going in circles is going backward: Outcome uncertainty in NASCAR. Journal of Sports Economics, 12(3), 253–283. https://doi.org/10.1177/1527002511404778
- Budzinski, O., & Feddersen, A. (2020). Measuring competitive balance in Formula One racing. In Outcome uncertainty in sporting events (pp. 5–26). Edward Elgar Publishing.
- Buraimo, B., Forrest, D., McHale, I. G., & Tena, J. D. (2020). Unscripted drama: Soccer audience response to suspense, surprise, and shock. Economic Inquiry, 58(2), 881–896. https://doi.org/10.1111/ecin.12874
- Buraimo, B., Forrest, D., McHale, I. G., & Tena, J. D. (2022). Armchair fans: Modelling audience size for televised football matches. European Journal of Operational Research, 298(2), 644–655. https://doi.org/10.1016/j.ejor.2021.06.046
- Butler, D., & Butler, R. (2023). Ghost games and ex-post viewing preferences for the English premier league: Evidence from YouTube highlights. Sports Economics Review, 100012. https://doi.org/10.1016/j.serev.2023.100012.
- Butler, D., Butler, R., & Maxcy, J. (2022). New insights on the Louis-Schmeling paradox: Determinants of demand for subscription and pay-per-view boxing. European Sport Management Quarterly, 22(4), 588–608. https://doi.org/10.1080/16184742.2020.1820063.
- Butler, R., & Massey, P. (2019). Has competition in the market for subscription sports broadcasting benefited consumers? The case of the English Premier League. Journal of Sports Economics, 20(4), 603–624. https://doi.org/10.1177/1527002518784121
- Coates, D., Humphreys, B. R., & Zhou, L. (2014). Reference-dependent Preferences, loss aversion, and live game attendance. Economic Inquiry, 52(3), 959–973. https://doi.org/10.1111/ecin.12061
- Dang, T. M., Booth, R., Brooks, R., & Schnytzer, A. (2015). Do TV viewers value uncertainty of outcome? Evidence from the Australian Football League. Economic Record, 91(295), 523–535. https://doi.org/10.1111/1475-4932.12206
- Garcia-del-Barrio, P., & Reade, J. J. (2022). Does certainty on the winner diminish the interest in sport competitions? The case of Formula One. Empirical Economics, 63(2), 1059–1079. https://doi.org/10.1007/s00181-021-02147-8
- Gasparetto, T., Orlova, M., & Vernikovskiy, A. (2022). Same, same but different: Analyzing uncertainty of outcome in Formula One races. Managing Sport and Leisure, 1–15. https://doi.org/10.1080/23750472.2022.2085619
- Gooding, C., & Stephenson, E. F. (2017). Superstars, uncertainty of outcome, and PGA tour television ratings. Journal of Sports Economics, 18(8), 867–875. https://doi.org/10.1177/1527002516637649
- Judde, C., Booth, R., & Brooks, R. (2013). Second place is first of the losers. Journal of Sports Economics, 14(4), 411–439. https://doi.org/10.1177/1527002513496009
- Kang, B., Salaga, S., Tainsky, S., & Juravich, M. (2018). NCAA college basketball television viewership: Does preference for outcome uncertainty change throughout the season? International Journal of Sport Finance, 13(4), 373–391.
- Kipker, I. (2003). Determinanten der kurzfristigen TV-Nachfrage in der Formel 1–Superstar-vs. Spannungseffekte und Implikationen für die Wettbewerbspolitik. Globalisierung des Wirtschaftlichen Wettbewerbes im Sport, 85–104.
- Konjer, M., Meier, H. E., & Wedeking, K. (2017). Consumer demand for telecasts of tennis matches in Germany. Journal of Sports Economics, 18(4), 351–375. https://doi.org/10.1177/1527002515577882
- Krauskopf, T., Langen, M., & Bünger, B. (2010). The search for optimal competitive balance in Formula One (No. 38). CAWM Diskussion Papier/Centrum für Angewandte Wirtschaftsforschung Münster.
- Larson, D. J., & Maxcy, J. (2014). Uncertainty of outcome and radio policy in professional road cycling. Journal of Sport Management, 28(3), 311–323. https://doi.org/10.1123/jsm.2012-0295
- Mastromarco, C., & Runkel, M. (2009). Rule changes and competitive balance in Formula One motor racing. Applied Economics, 41(23), 3003–3014. https://doi.org/10.1080/00036840701349182
- Mizak, D., Neral, J., & Stair, A. (2007). The adjusted churn: An index of competitive balance for sports leagues based on changes in team standings over time. Economics Bulletin, 26(3), 1–7.
- Mourão, P. (2017). The economics of motorsports: The case of Formula One. Springer.
- Neale, W. C. (1964). The peculiar economics of professional sports: A contribution to the theory of the firm in sporting competition and in market competition. The Quarterly Journal of Economics, 78(1), 1–14. https://doi.org/10.2307/1880543
- Ourand, J. (2022). Sports Media: F1 goes to market expecting big increase in rights next year as U.S. interest continues growth. Sportsbusinessjournal.com. https://www.sportsbusinessjournal.com/Journal/Issues/2022/04/11/Insiders/Sports-media.aspx (Accessed 12 June 2022)
- Pawlowski, T., Nalbantis, G., & Coates, D. (2018). Perceived game uncertainty, suspense and the demand for sport. Economic Inquiry, 56(1), 173–192. https://doi.org/10.1111/ecin.12462
- Peeters, R., & Wesselbaum, D. (2023). Competitiveness in Formula One. Sports Economics Review, 100007. https://doi.org/10.1016/j.serev.2022.100007.
- Rottenberg, S. (1956). The baseball players’ labor market. Journal of Political Economy, 64(3), 242–258. https://doi.org/10.1086/257790
- Schreyer, D., & Torgler, B. (2018). On the role of race outcome uncertainty in the TV demand for Formula 1 Grands Prix. Journal of Sports Economics, 19(2), 211–229. https://doi.org/10.1177/1527002515626223
- Van Reeth, D. (2013). Television demand for the Tour de France: The importance of outcome uncertainty, patriotism and doping. International Journal of Sport Finance, 8, 39–60.