ABSTRACT
With the introduction of the Teaching Excellence Framework a lot of attention is focussed on measuring learning gains. A vast body of research has found that individual student characteristics influence academic progression over time. This case-study aims to explore how advanced statistical techniques in combination with Big Data can be used to provide potentially new insights into how students are progressing over time, and in particular how students’ socio-demographics (i.e. gender, ethnicity, Social Economic Status, prior educational qualifications) influence students’ learning trajectories. Longitudinal academic performance data were sampled from 4222 first-year STEM students across nine modules and analysed using multi-level growth-curve modelling. There were significant differences between white and non-White students, and students with different prior educational qualifications. However, student-level characteristics accounted only for a small portion of variance. The majority of variance was explained by module-level characteristics and assessment level characteristics.
Introduction
Recent calls about the need for clear and transparent measures of learning to assess the value of Higher Education (HE) in the United Kingdom (Department for Business, Innovation & Skills, Citation2016; Gibbs, Citation2010) have promoted the introduction of the Teaching Excellence Framework (TEF). With the TEF a lot of attention is focussed on developing and testing a range of measurement approaches aiming to capture relative improvements in student learning. Learning gains can be defined as ‘distance travelled’ (McGrath, Guerin, Harte, Frearson, & Manville, Citation2015) or more elaborately as students’ growth or change in skills, abilities and knowledge that are related to the learning outcomes of the course (Rogaten et al., Citation2018). Despite the growing interest in research on the measurements of learning gains, the effects of individual differences on learning gains have been largely ignored. Although learning gains within a discipline or institution might provide some insights into the average learning gains that students might make during their university experience, several critics have indicated that these learning gains might not be the same for all students (Boud, Citation2017), in particular for students from ‘disadvantaged’ backgrounds (Ashwin, Citation2017). Therefore, this study aims to address this gap by examining whether or not learning gains, as measured by grade trajectories, are influenced by students’ socio-demographic characteristics.
Assessment practices and individual differences
Assessment forms a substantial part of studying in HE. Almost all universities use some form of assessment to assess whether, what and how students learned on the courses (Boud, Citation2017; Coates, Citation2016; Nguyen, Rienties, Toetenel, Ferguson, & Whitelock, Citation2017). As such, if assessment practices across a range of consecutive modules in a degree are effective one should expect that these grades may reflect a proxy of students’ learning gains. However, if assessments are not aligned well to assess actual students’ learning (i.e. assessments are either too hard or too easy, ‘discriminating’ one type of learner over another), then this will result in a large variation between assessments grades. It goes beyond the scope of this article to address effective assessment practices and policies, but we refer to previous well-established work on effective summative and formative assessment practice in HE (e.g. Bearman et al., Citation2016; Boud, Citation2000, Citation2007; Carless, Citation2009).
The main focus of this study is on how socio-demographic factors may positively or negatively influence students’ learning trajectories. Previous research has found that socio-demographic variables (i.e. gender, ethnicity, Social Economic Status (SES), prior educational qualification) play an important role in predicting students’ attainments, especially in distance learning settings. First, some researchers found that there was a gender attainment gap, in particular in Science, Technology, Engineering and Mathematics (STEM)-related disciplines. In several studies male students were found to be awarded higher final degree classifications than female students (e.g. Mellanby, Martin, & O’Doherty, Citation2000), whereas in other studies the opposite was reported, that is male students had lower initial grades than female students, and the gender gap increased with time (Conger & Long, Citation2010).
A meta-analysis of 502 effect sizes from 369 samples indicated that female students outperformed male students overall, but the effect sizes were small in science education, and these differences disappeared in studies where students were predominantly males (Voyer & Voyer, Citation2014). However, despite a large number of existing studies on gender attainment, most were done in face-to-face education, and it is important to examine if similar patterns exist in distance learning. There is already a wealth of literature that highlights that in distance learning a more diverse body of students participate in HE, in part because of the appeal of flexibility and anytime learning (Richardson, Citation2012; Richardson, Rivers, & Whitelock, Citation2015; Rienties & Toetenel, Citation2016). As such, it was hypothesised that:
(H1) There will be a difference between male and female students in their attainment.
Second, ethnicity has also continuously been found to be an important factor in academic attainment, with white students having higher attainments at all levels of the educational system than non-White students (Kao & Thompson, Citation2003; Richardson, Citation2015). At the same time, preliminary research at the Open University (OU) seems to indicate that once non-White students have successfully passed their first module, they are able to catch up relatively quickly (Rienties et al., Citation2017). Therefore, we expect that over time non-White students are able to bridge the attainment gap (H2b).
(H2a) White students have higher academic attainments relative to non-White students
(H2b) The initial gap in attainment between white and non-White students will decrease over time
Third, SES has typically been found to have a direct and indirect impact on academic achievement (Richardson, Citation2012; Robbins et al., Citation2004). Typically students from lower SES have been found to underperform relative to students from higher SES (Richardson, Citation2012; Robbins et al., Citation2004). However, given the OU’s widening access agenda (i.e. provision of support to students, designing courses and learning materials that take into account potential ‘weaknesses’ of learners) we expect (and perhaps hope) that students from ‘non-traditional’ backgrounds will be able to keep up with the pace of learning, and narrow the initial gap over time between them and others.
(H3a) Students with higher SES have higher academic attainment
(H3b) The initial gap in attainment between high and low SES students will decrease over time
Finally, research overwhelmingly showed that prior educational achievement is one of the strongest predictor of future educational achievements (Plant, Ericsson, Hill, & Asberg, Citation2005). The last point is of particular importance to the OU, as over 1/3 of the student population has below A levels academic qualifications. As mentioned earlier, OU takes into account these potential weaknesses of newly enrolled students and therefore we would expect that the gap between students with A-levels and above and between students with qualifications below A-level or no qualification will decrease over time as they develop necessary skills for studying in HE. Therefore it was hypothesised that:
(H4a) Students with A-level or above qualifications have higher academic achievement
(H4b) The initial gap in attainment between students with A-level qualifications and above and students with below A-level or no qualification will decrease over time
Method
Setting and participants
The OU practices an open-entry policy, which allows anyone regardless of prior educational achievements and qualifications to enrol in first-year modules. Successful completion of first-year modules allows participants to progress towards obtaining a degree. Practicing this policy brings challenges in relation to students’ skills and abilities to successfully progress through the first-year modules and as such, it is important to understand what contributes to students’ progress in those modules.
We specifically sampled our data on STEM and first-year modules, where historically the largest retention issues have been identified (Rienties & Toetenel, Citation2016). Concentrating on the STEM subjects and in particular, the first-year modules has a number of advantages, like reducing the heterogeneity of the assessment practices, and increased consistency in provision and disciplinary focus. Furthermore, all the modules selected for this study are year-long modules which provide suitable longitudinal data for estimating grade trajectories.
Data were retrieved from the OU database for 4551 first-year STEM undergraduate students across nine core STEM modules, ranging from 104 to 1333 enrolled students, whereby students studied for 32–34 weeks. The sampling method used in this study was a cluster sampling, which comprised of the full cohort of new students who completed one of the nine modules selected for this study. The data was cleaned and all students who had missing data or preferred to withhold information in relation to their gender, ethnicity, prior educational qualification and SES were removed from the sample. Thus, the final sample used in this study was 4222 students studying in nine first-year STEM modules. In relation to age, 86% of the students were between 18 and 39 years old. The composition of students in each module by socio-demographic variables that were used as predictors in the analysis is presented in . Overall, this sample is fairly representative of the OU STEM population as demographic characteristics are similar to students who have been studying in previous years
Table 1. Distribution of students across nine modules based on their socio-demographic characteristics.
Materials and procedure
Ethics clearance was obtained from the OU Human Research Ethics Committee (HREC/2015/2155). Continuous assessment results (Tutor/Computer Marked Assessments in OU jargon) were retrieved from the university database for the academic year 2013/14. Continuous assessments usually comprise of tests, essays, reports, portfolios, workbooks, but do not include final examination scores. In general, continuous assessments grades contribute around 50% towards overall final module grade. In all selected modules there were minimum of three assessments and a maximum of seven assessments per module. As such, achievements on continuous assessment provided sufficient data to estimate students’ learning progress throughout these nine modules. Importantly, although there were differences between the modules in their assessments types and frequency of assessment, there was no variation within assessment within the modules. The between module differences and between assessment differences were accounted for in the analysis.
Data analyses
The data was analysed using linear growth-curve modelling estimated in the MLWiN 3.3 software package (Rasbash, Steele, Browne, & Goldstein, Citation2009). The suitability of the data for estimating multi-level model was satisfactory in terms of the sample size for each level of the model (Maas & Hox, Citation2005). Firstly, a linear regression model was estimated to test the model fit on the continuous assessment data. Secondly, the regression model was compared to the 2- and 3-level random intercept and slope models, where continuous assessments were at level 1, the level 2 variable was the student, and level 3 variable was the respective module students were enrolled in. The nested 3-level model is presented in . The dependent variable was students’ attainment at each continuous assessment throughout the module, with a minimum score of 0 and a possible maximum score of 100. There were a number of instances where some continuous assessment scores were missing, which is acceptable because growth-curves could be estimated on existing data (Rasbash et al., Citation2009).
Figure 1. A three-level data structure with repeated measures (continuous assessment scores) at level-1, students at level-2 and modules at level-3.
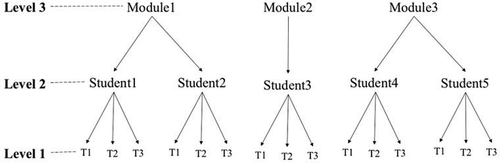
The advantage of using multilevel modelling when working with this type of data is that it takes into account the differences in the assessment practices such as frequency, timing, types of assessment, etc. By specifying ‘Module’ as a level in the growth-curve model we are able to account for the lack of independence between grades for each assessment, student and module. As such, this way of analysing data provides more accurate estimates of errors and avoids the problem of aggregating diverse data (Rasbash et al., Citation2009). Thirdly, our core socio-demographic variables (gender, ethnicity, SES, prior education) were entered first in the model and were nested at student and module levels. For the analysis of the effects of the socio-demographic variables the weighted means were used, and homogeneity of variance was acceptable for this type of analysis. Finally, in order to examine whether socio-demographic predictors had an impact on learning trajectories, interactions between time and socio-demographic predictors were tested.
Results
As a first step, we explored the overall trends from our multi-level model and the underlying structure of our data. Module average continuous assessments’ means, median and standard deviations are presented in . On average, participants obtained continuous assessment scores that were substantially above the passing threshold of 40. presents the growth curves for each of the nine science modules, whereby three modules had positive grade trajectories, while six modules had negative grade trajectories. The average grade increase across the 4222 students was 2.6 grade points (SD = 11.3), which indicated that increase in performance in three modules was proportionally larger, and compensated for the modules that showed an overall decrease in performance. This was further supported by the high dispersion of progress scores across the nine modules.
Table 2. Mean and standard deviations across all continuous assessments for each of the nine level-1 modules.
In order to examine whether the data had a hierarchical structure, subsequent growth-curve models were estimated. Thus, regression, 2-level and 3-level growth curve models were compared with their fit to the data. The results are presented in , whereby the 2-level model fitted the data significantly better than the regression model, and the 3-level model fitted the data better than the 2-level model. This suggests that the present dataset had a 3-level hierarchical structure, and the effects of any predictor variables including socio-demographics should be tested taking the multi-level data structure into account.
Table 3. Intercept, slope and deviance statistics for model comparison.
The partitioning of the variance in the 3-level growth curve model showed that the largest variance in students’ learning gains could be attributed to the respective module student were enrolled in (i.e. level 3). Over half of the variance of how students were progressing over time was due to so-called ‘module characteristics’. For example, learning design and frequency of assessments within each of these nine modules influenced how students’ continuous assessment scores developed over time. In other words, students were put into a ‘module straight-jacket’, whereby half of their variance was related to how a respective module was structured.
Furthermore, the second largest portion of variance was attributed to the continuous assessment level. In other words, students obtained substantially different assessment scores during their module, which may indicate that there was a lack of alignment between the assessments, different motivational efforts by a student for each respective assessment, or a combination of these two. Thus, even though students were put into the same module straight-jacket, students progressed relatively unevenly through the module. The variance partition coefficients are presented in .
Table 4. Unstandardised beta coefficients for the main effects of each socio-demographic predictor (Model 2) and interactions between socio-demographic predictors and time (Model 3).
Influence of socio-demographic variables on learning trajectories
As a second step and primarily focusing on testing our hypotheses, we specifically tested the impact of individual differences on students’ grade trajectories. Even though the smallest proportion of variance in our multi-level modelling (i.e. 8.5%) could be attributed to individual differences, of course it would be essential to determine whether there were implicit or explicit factors influencing the learning trajectories of students. On the one hand, the relatively low influence of socio-demographics and other student characteristics could be seen as something positive. In contrast to a range of studies highlighting inequalities in academic achievement due to socio-demographic factors (e.g., Conger & Long, Citation2010; Richardson, Citation2015), the OU open access policy seemed not to structurally disadvantage particular widening access groups. On the other hand, individual differences did seem to influence learning trajectories, and therefore in we illustrated the respective impacts of our socio-demographic variables.
There was no difference in gender attainment (B2 Male = − 0.221, p > 0.05), and the addition of gender into the model had no effect on the overall model fit. As such, no support was found for H1 and gender was removed from the model. All other remaining socio-demographic predictors individually made significant improvements to the model fit. As can be seen from the , ethnicity, SES and prior educational level reduced the variance in the model by 10%, and all interactions further reduced it by additional 0.1%. As such, socio-demographic variables made a modest yet significant contribution to explaining the variance.
There were significant differences between students from white and non-White ethnic backgrounds, whereby as expected non-White students had lower academic achievements that white students (H2a). Interactions between ethnicity and time showed that the gap between white and non-White students increased slightly throughout the module rather than reduced (-H2b), but this increase was not significant. In relation to SES, students from low SES background had lower attainments in comparison to students from non-low SES backgrounds (H3a). The results of the interaction showed that the gap in the attainment increased slightly rather than decreased (-H3b), but again this was not significant throughout the model.
Finally, there were significant differences in terms of prior qualifications, whereby as hypothesised students with prior A-level or equivalent qualification had higher attainment than students with the qualifications below A-levels. Students with HE qualification had higher attainments than students who only had A-level qualification or equivalent (H4a). Furthermore, there was a significant interaction between prior academic qualification and time, whereby the gap in attainment between students with A-level qualification or equivalent and those with degree qualification decreased, and the gap between students with A-level qualification or equivalent and those with qualifications below A-level increased (-H4b). The graphical representation of the interaction is presented in . In sum, white students, students from non-low SES and those who had A-levels or equivalent or HE qualification prior to the start of their degree showed high attainments, and students with A-levels or equivalent showed the highest-grade increase in comparison to students with other education qualifications.
Discussion
The main aim of this study was to examine the effects of socio-demographic factors on students’ learning trajectories. Based on the review of the existing literature, this study hypothesised that there would be initial differences in learning attainment based upon differences in gender, ethnicity and socio-economic status, but the expectation was that these individual differences would disappear as students were becoming more familiar with studying online. Taking into account that OU has open access policy, this study was in particular interested how prior educational qualifications impacted students’ learning trajectories.
Overall, our results showed that only 8.5% of the variance in students’ learning trajectories could be attributed to student characteristics. Although some research indicated gender differences in STEM performance (Mellanby et al., Citation2000), no gender differences in initial achievements and learning trajectories were found, in line with previous research with OU students (Rogaten, Rienties, & Whitelock, Citation2017). This in a way provides an optimistic outlook for STEM subjects as the OU seemed to have mitigated gender inequality in first-year module attainments.
Previous research had also identified that non-White students underperformed in comparison to white students (Kao & Thompson, Citation2003; Richardson, Citation2015). This study found that indeed non-White students showed on average lower attainments, but the differences in learning trajectories were small. This finding has important implications in relation to widening participation and access to HE, as STEM is primarily consisting of white students. Students from a low SES background had lower academic attainment, and the gap in attainment did not change over time. Taking into account that OU invests substantial resources to help students from diverse backgrounds to obtain a HE qualification, we anticipated that over time SES and non-White students would keep up and possibly catch up with white students and students from non-low SES, which does not seem to be supported by our analyses.
As expected, students with a previous HE degree and those who had A levels had significantly higher attainments than those who had below A-levels academic qualification. The observed relationships in a way are unfortunate but expected, in particular for the students who had below A-levels qualifications as those students are on a much steeper learning curve. It may take them longer to develop the skills necessary for making faster progress in learning, and hopefully catch up with those students who started their degree with more advanced basic academic skills.
Limitations and future research
Although the results of our study are important for understanding STEM students’ learning progress in first-year modules, there are three main limitations that should be taken into account when considering these findings. Firstly, using assessment scores as a proxy for learning gains relies heavily on the assumption that assessment scores are valid and reliable indicators of learning. As with many other UK universities, the OU spends substantial effort to ensure that assessments are valid and reliable, are rooted in the learning design, aligned with the Quality Assurance Agency (QAA) framework, supported by appropriate moderation and double marked by external examiners.
Secondly, these results were estimated on the part-time distance-learning students, and as such may have limited generalisability in other educational environments. Therefore, future research should examine learning trajectories of students from different socio-demographic backgrounds in ‘traditional’ full-time face-to-face university contexts. Further testing is needed to see if similar results are also observed in other non-science disciplines.
Finally, there seems to be an uneven distribution in different socio-demographics characteristics across the nine focussed modules. Some modules had proportionally a larger number of students who had above A-level qualifications than others, and in some modules, there were proportionally more students from low SES, which would impact the trajectory within the module. In future research a wider set of modules needs to be taken into consideration to offset any imbalances in initial starting conditions.
In conclusion, the results of this study make an important contribution to our understanding of students’ progress in their first-year undergraduate distance education in STEM modules. The results overall showed a relatively optimistic picture, with student-level characteristics accounting for a relatively small portion of the variance. The majority of the variance lied at the module level and assessment level. As such, it is within university power to intervene and improve students’ learning trajectories by further strengthening our individual support structures, and where needed provide additional support for students with low prior educational qualifications.
Acknowledgments
This research was conducted as part of a “Longitudinal mixed-method study of learning gains: applying ABC framework” project funded by Higher Education Funding Council for England (HEFCE) as part of its wider work on learning gain (to find out more see the HEFCE website: http://www.hefce.ac.uk/lt/lg). The authors are also grateful for the excellent feedback from the reviewers.
Additional information
Funding
References
- Ashwin, P. (2017). Making sense of the Teaching Excellence Framework (TEF) results (Centre for Global Higher Education policy briefings). Lancaster. Retrieved from http://www.researchcghe.org/publications/making-sense-of-the-teaching-excellence-framework-tef-results/
- Bearman, M., Dawson, P., Boud, D., Bennett, S., Hall, M., & Molloy, E. (2016). Support for assessment practice: Developing the assessment design decisions framework. Teaching in Higher Education, 21(5), 545–556.
- Boud, D. (2000). Sustainable assessment: Rethinking assessment for the learning society. Studies in Continuing Education, 22(2), 151–167.
- Boud, D. (Ed.). (2007). Rethinking assessment in higher education: learning for the longer term (1 edition ed.). London: Routledge.
- Boud, D. (2017). Standards-based assessment for an era of increasing transparency. In Carless, D., Bridges, S., Chan, C., & Glofcheski, R. (Eds.), Scaling up assessment for learning in higher education. The enabling power of assessment (Vol. 5, pp. 19–31). Singapore: Springer.
- Carless, D. (2009). Trust, distrust and their impact on assessment reform. Assessment & Evaluation in Higher Education, 34(1), 79–89.
- Coates, H. (2016). Assessing student learning outcomes internationally: Insights and frontiers. Assessment & Evaluation in Higher Education, 41(5), 662–676.
- Conger, D., & Long, M.C. (2010). Why are men falling behind? Gender gaps in college performance and persistence. The ANNALS of the American Academy of Political and Social Science, 627(1), 184–214.
- Department for Business, Innovation & Skills. (2016). Higher education: success as a knowledge economy - white paper (No. 28041602 2809873 05/16). UK. Retrieved from https://www.gov.uk/government/publications/higher-education-success-as-a-knowledge-economy-white-paper.
- Gibbs, G. (2010). Dimensions of quality. York: Higher Educational Academy.
- Kao, G., & Thompson, J.S. (2003). Racial and ethnic stratification in educational achievement and attainment. Annual Review of Sociology, 29, 417–442.
- Maas, C.J.M., & Hox, J.J. (2005). Sufficient sample sizes for multilevel modeling. Methodology, 1(3), 86–92.
- McGrath, C.H., Guerin, B., Harte, E., Frearson, M., & Manville, C. (2015). Learning gain in higher education. Retrieved from http://www.rand.org/content/dam/rand/pubs/research_reports/RR900/RR996/RAND_RR996.pdf
- Mellanby, J., Martin, M., & O’Doherty, J. (2000). The ‘gender gap’ in final examination results at Oxford University. British Journal of Psychology, 91(3), 377–390.
- Nguyen, Q., Rienties, B., Toetenel, L., Ferguson, R., & Whitelock, D. (2017). Examining the designs of computer-based assessment and its impact on student engagement, satisfaction, and pass rates. Computers in Human Behavior, (Early Access), 76, 703–714.
- Plant, E.A., Ericsson, K.A., Hill, L., & Asberg, K. (2005). Why study time does not predict grade point average across college students: Implications of deliberate practice for academic performance. Contemporary Educational Psychology, 30(1), 96–116.
- Rasbash, J., Steele, F., Browne, W.J., & Goldstein, H. (2009). A user’s guide to MLwiN. Centre for multilevel modelling, University of Bristol (pp. 296).
- Richardson, J.T.E. (2012). The attainment of white and ethnic minority students in distance education. Assessment & Evaluation in Higher Education, 37(4), 393–408.
- Richardson, J.T.E. (2015). The under-attainment of ethnic minority students in UK higher education: What we know and what we don’t know. Journal of Further and Higher Education, 39(2), 278–291.
- Richardson, J.T.E., Rivers, B.A., & Whitelock, D. (2015). The role of feedback in the under-attainment of ethnic minority students: Evidence from distance education. Assessment & Evaluation in Higher Education, 40(4), 557–573.
- Rienties, B., Rogaten, J., Nguyen, Q., Edwards, C., Gaved, M., Holt, D., … Ullmann, T. (2017, June 1). Scholarly Insight Spring 2017: A Data Wrangler Perspective. Open University UK. Retrieved from http://article.iet.open.ac.uk/D/Data%20Wranglers/Scholarly%20Insight%20Report%20Spring%202017/DW_scholarly_insight_31_05_2017.pdf
- Rienties, B., & Toetenel, L. (2016). The impact of learning design on student behaviour, satisfaction and performance: A cross-institutional comparison across 151 modules. Computers in Human Behavior, 60, 333–341.
- Robbins, S.B., Lauver, K., Le, H., Davis, D., Langley, R., & Carlstrom, A. (2004). Do psychosocial and study skill factors predict college outcomes? A meta-analysis. Psychological Bulletin, 130(2), 261–288.
- Rogaten, J., Rienties, B., & Whitelock, D. (2017). Assessing learning gains. In D. Joosten-ten brinke & M. Laanpere (Eds.), Technology enhanced assessment. tea 2016. communications in computer and information science (Vol. 653, pp. 117–132). Cham: Springer.
- Rogaten, J., Rienties, B., Sharpe, R., Cross, S.J., Whitelock, D., Lygo-Baker, S., & Littlejohn, A. (2018). Reviewing affective, behavioural, and cognitive learning gains in higher education. Assessment and evaluation in higher education. Manuscript submitted for publication. Assessment and Evaluation in Higher Education.
- Voyer, D., & Voyer, S.D. (2014). Gender differences in scholastic achievement: A meta-analysis. Psychological Bulletin, 140(4), 1174–1204.