ABSTRACT
We surveyed dragonflies (Odonata) at 87 sites in the anthropologically modified Pampa biome of southern Brazil to evaluate how regionally rare and common species form species assemblages in habitats with different water physiochemistry, habitat structures, and other environmental variables. We classified 9 out of the 90 species encountered as regionally common and 59 as regionally rare. A discriminant analysis confirmed that localities with only a few common species were characteristic in the set of rare species present, while localities housing more common species showed no clear pattern. A PCA revealed that a subset of the common species were strongly positively associated with water temperature, turbidity, dissolved O2 and pH but negatively associated with desertification. In contrast, rare species were positively associated with grassland habitat, but negatively with agriculture, salinity, and conductivity. In general, the associations of the rare species were weaker than those of common species. Finally, a correlation suggested that sites with six or more common species present had a reduced number of rare species compared to sites with fewer common species. It is possible that common species reduce the available niche space for weaker competitors among the rare species. We conclude that the original species assemblages in the biome may have been species poor with few regionally common species. Current anthropogenic change has increased the number of common species, which in turn has negative effects on the survival possibilities of rare species.
Introduction
In general, animal communities and their distributions are shaped by environmental factors, biotic interactions, dispersal, and stochasticity [Citation1,Citation2]. The complexity of assemblage structure and distribution is the result of an interplay of patch dynamics, neutral effects, species sorting and dispersal capabilities [Citation3]. Although not always determinant for range size at regional or even global scale [Citation4], dispersal capability is a key component, which also directly influences the local community [Citation5]. At local scale, biotic interactions, such as interspecific competition, have proven to be of great importance in community structuring dynamics [Citation6,Citation7]. Another example is the spatial competition hypothesis discussed in [Citation8]. We therefore know that common species influence the presence of rare species, as shown by studies on several animal groups such as butterflies [Citation9], ants [Citation10], and among vertebrates [Citation11,Citation12].
In aquatic systems, at small spatial scales, environmental conditions are among the most important factors determining community structure [Citation13–15], since the presence or absence of species will depend on the prevailing conditions, e.g. species sorting [Citation16]. Further, the intensification of human disturbances is one of the main triggers of assemblage changes over time [Citation17], resulting in species declines, loss, and changes in assemblage composition [Citation18,Citation19], affecting specialist (more sensitive) and generalist (highly adaptive) species alike [Citation20].
Coexistence mechanisms forming a community of common, moderately common and rare species function in several ways. Chesson [Citation21] suggested that mechanisms acting on densities and environmental factors over time are as essential as resource partitioning and frequency-dependent predation. Coexistence of species with different habitat requirements is possible if they have high enough dispersal rates enabling them to reach possible sites where a superior competitor is not present [Citation22].
All communities are composed of a small number of common species and a larger set of rare ones [Citation23]. According to general theory, this pattern is universal, but many cases have shown that the number of rare species is higher than expected [Citation12]. The term “rare” does not automatically imply that a species is threatened in any way as there are many different reasons for a species to be less common in an area. Rabinowitz [Citation24], working on plants, defined rarity by differences in local abundance (low vs. high for common species), geographic range (narrow vs. wide), and habitat specificity (low vs. high). A species can be considered rare if it is encountered in only a fraction of the localities surveyed or if only a few individuals are found, if it has a narrow geographic range and thus high habitat specificity, and therefore is vulnerable to habitat loss [Citation25]. On the other hand, common species are defined as widespread, locally abundant, and resistant to environmental changes, in many cases also having higher dispersal capabilities [Citation26]. Surveying common species is easy, as they should be present in a large percentage of the samples, but it is always difficult to survey rare species, as has been discussed when selecting indicator species [Citation27] and references therein. Further, most of the known species that tend to be rare occur only in small and restricted geographical ranges [Citation27]. Turnover rates for rare species are high, they disappear more often from sites, and if they are locally extinct, they require longer times to re-immigrate than common species [Citation28]. At the same time, rare species are often more valuable from a conservation point of view, and many decision makers rely on information about rare species alone [Citation29,Citation30]. Hence, it is important to understand how common species might interact with the occurrence of rare species in poorly known aquatic assemblages, as those in the Pampa, southern grassland, in southern Brazil.
We used dragonflies (Odonata) as model organisms; this group has been frequently used in ecological studies and is known to respond to landscape change [Citation2,Citation31], climate change [Citation32] and water chemistry [Citation33]. This order has been suggested to be a useful bioindicator group [Citation34–36], and as the group is semiaquatic with an aquatic larva and a terrestrial adult, both stages are selective in terms of habitat choice cf. [Citation35].
We asked the following questions: 1) How will regionally common species interact with or affect rare ones, and the overall species assemblages in different types of water bodies in the area? 2) What are the relationships between these assemblages and the habitat structures and environmental factors present in and around these water bodies? We expect to be able to increase our understanding of how species interact with their environment and how those interactions shape patterns in community composition.
Materials and methods
Study area
The study was performed in the Pampa biome in southern Brazil. This biome is one of the least known in the Neotropics [Citation37]. Several studies performed in this area have shown that certain groups of organisms have high diversity and high levels of endemism, at least regarding the flora [Citation38,Citation39]. These grasslands are under high pressure from cattle farming, agriculture, and forestry; the latter has resulted in the conversion of vast areas into exotic tree plantations of Eucalyptus, Pinus, and Acacia [Citation37,Citation40]. Data from ten years ago showed that only 36% of the original vegetation remains in a highly fragmented mosaic [Citation38], and only 0.5% of the biome is officially protected [Citation37].
We sampled adult odonates in 87 sites within the Pampa. We selected the sites to reflect the diversity different habitats in the region. Our chosen streams, ponds (temporary or permanent), lakes, and wetlands were clustered in seven regions ranging from 29°24’ to 30°55’ S and 53°07’ to 56°29’ W (). The areas have mean annual temperatures between 13°C and 17°C, altitudes from 50 to 200 m.a.s.l. and mean annual precipitation between 1,200 and 1,600 mm [Citation41] corresponding to temperate climate (Cfb Köppen).
Species sampling
Our species appear as clusters at a range of sites in a varying landscape, i.e. we do not have a homogeneous landscape, and consequently more of a pattern-based diversity cf. [Citation42]. As pattern-based diversity is fairly insensitive to the intensity of sampling (of rare species), we strived for an average sampling effort per site instead of trying to find every possible species in the habitat. We sampled adult dragonflies from March 2015 to January 2017, visiting sampling sites 1–5 times during this period, often once per season excluding winter months (May–August). The sampling sites included the whole range of different waterbodies in the region, suitable for Odonata: from large river sections, to man-made lakes and temporary water. Our sites were mostly small, often around 100 m in length (for lotic environments) or diameter (for lentic water bodies) up to bigger lakes with a surface area of many hectares. The streams (n = 43) were of different size, from small streamlets with a width of 1 meter up to shoreline sections of major streams in the area (width >25 m). The shorelines often had dense riparian forest, but seven sites lacked forest cover. The ponds, lakes, and wetlands (n = 44) varied in the spectrum from fully overgrown areas with seeping groundwater, via densely vegetated lakes (normally dammed streams) to small water reservoirs with only sparse vegetation. We classified 20 of the localities as temporary, although they had the same variation in habitat structures as the permanent ones. The temporary sites, often rain pools or rivulets fed during rain were, with some exceptions, visited only once, due to them rapidly drying out. Permanent and longer lasting temporary waters were visited more frequently, i.e. up to 5 times, as the species assemblage may differ during the season and between years due to phenological differences. We are aware that, especially on localities that were only sampled on one or two events, the phenology of certain species may have caused an underestimation of the real species numbers, which in turn may have reduced our perception on the proportion of rare/common species. We used the method described by Renner et al. [Citation36]: hand-held insect nets by a team of two people in sunny days during the peak activity of Odonata (09:00 h to 16:00 h). We collected along the edges and marginal zones of waterbodies; distances varying in length according to the size and shape of the water body; the average time spent per site was 30 minutes. For each site, we noted the species present and the number collected. We sampled most specimens encountered, but strong flyers of the family Aeshnidae were visually recorded, which was possible since there are only a few species in the area, and the individuals are easy to detect. We assessed the effectiveness of our sampling efforts through a rarefaction curve. Here, we use a subset of the dataset from a bigger study [Citation43], in which the sampling was extended over larger areas and over a seven-year time-period. We wanted to limit habitat variation for our analyses and also reduce the between year variation. We therefore selected sites visited during three consecutive years situated in the savanna or steppe vegetation types in the Pampa [Citation43], i.e. typical Pampa grassland. The dataset has also been used to evaluate effects on land use on species composition in the area [Citation44].
The majority of species determination was possible by using a reference collection at Museu de Ciências Naturais da Univates, largely established from previous work [Citation43,Citation44], whereas the initial determination to genera used the standard literature available for Latin America [Citation45–50]. In some difficult cases, original species descriptions and external experts were consulted. The specimens were deposited in the MCNU (Museu de Ciências Naturais da Univates). The collection authorization process was issued by ICMBio, through the SISBio system under the number 50,624–1.
Environmental variables
As it was not possible to use GIS mapping for parts of the area, we used recent (2013–2016) satellite images available via Google Earth Pro™, where we quantified seven land use variables inside a circular perimeter of 1 km2 with its center at the midpoint of the sampling site. This method will result in an inconstant area of land surveyed due to a varying amount of water present in the circle (0% to 35% of the area), and water area was therefore chosen as one of the land cover variables (below). Using the area measuring tool in the program, we quantified the cover percentage of each variable inside the perimeter. All areas were visible in the maps without any presence of clouds or haze. The land use variables were chosen as follows:
- forest: mostly riparian forests which are the commonest formations in the area, also isolated gallery forest patches scattered in the landscape as “Islands”;
- forestry: often plantations of exotic trees with commercial purposes, in the Pampa the most common species used are Eucalyptus sp., Pinus sp., and Acacia sp.;
- grassland: areas used for cattle grazing, considered both native and also areas with exotic grass species, e.g. Eragrostis plana (Poaceae), an African grass species;
- agriculture: planted areas for crop production, the most common crops grown in the region are rice, soybeans, and corn;
- water surface: areas covered by the water bodies within the perimeter; some water bodies, e.g. large rivers, continued outside the circle);
- human built structures: roads, buildings, and urbanized areas;
- sand: erosion sites caused by the loss of vegetal layer and ongoing desertification processes which are taking place in several localities of the Pampa.
At each site, and at least on one visit, we measured temperature, pH, conductivity, turbidity, dissolved O2, total dissolved solids, and salinity using a Horiba multi-parameter water quality meter (Horiba Co., Japan). We placed the sensor of the probe at a suitable location representing the most common sub-habitat at each site and near the shore-line, aiming to take measurements in a water column of at least 50 cm. Note that some of the (mostly temporary) locations had very shallow water and at some of these locations (20) these measurements had to be excluded due to extremely low water levels. Values measuring more than once were averaged. All landcover and water chemistry measures are available in Supplementary material 1.
Species numbers and classification
There is no consensus of what constitutes a rare vs. a widespread (common) species and, as pointed out by Hartley and Kunin [Citation51], rarity should be explained using several different factors. This is, however, often not possible when dealing with species where ecological information is scarce [Citation52] such as most of the species in our study area in southern Brazil. We therefore use a simple classification of common vs. rare, similar to that used for dragonflies in two other studies, where the authors used percentages to classify species as “moderately common” [Citation34,Citation53]. We adapted this method based on our dataset, allowing for lower and higher percentages, respectively. In total, our dataset contained 90 species. We classified them into regionally common (occurring at ≥30 localities in the dataset; 9 species in total) and regionally rare (occurring at ≤10; 59). A complete species list with the respective classifications is available as Supplementary material 2. The remaining 22 species, neither common nor rare, were only included in the number per site.
Aiming to obtain a general overview on the species diversity on each sampling site, we further classified the localities into three categories based on the number of common species encountered: 1) 0–2 species present; 2) 3–5 species present; and 3) 6–8 species present; no locality had all 9 common species present. Based on this, we classified the rare species according to their occurrence at localities with different numbers of common species: a) species present only at localities with up to 2 common species; b) present at localities with up to 5 common species, and c) present at localities with up to 8 common species; this classification enables us to see what we might call the “tolerance” of rare species to the presence of common species in the assemblages.
Data analyses
We tested the spatial independence of the 87 sampling sites related to species composition using a Moran’s I analysis [Citation54]. We used individual species occurrence as variables in a Principal Components Analysis (PCA) where the first axis was used as response variable to the Moran’s I with coordinate variables for 10 different distance classes. The global Moran’s I analysis did not detect any significant spatial structure of the species composition for any distance classes (minimal distance class average: 0.01 degrees; Moran’s I = 2.29; p = 0.61), and, hence, spatial structure was not included in further statistical analysis.
We performed a discriminant analysis to investigate whether the distribution of the rare species at sites with different numbers of common species present was random or not. By using a set of independent variables (here: the species) a discriminant analysis is searching for linear combinations of those variables (aka: discriminant functions) which separates the groups of cases most clearly [Citation55]. Our collecting method, where we actively search for different species, will favor those which are not abundant at the sites, whereas all specimens of the abundant species are not always collected. To correct for this possible error, we used relative abundance of the species at all sites as independent variables, using ln(n + 1) transformation to level out high differences in numbers of collected specimens. We used the three categories of common species (above) as grouping variable. This procedure results in two discriminant functions, each with an Eigenvalue informing of the efficiency of the function and a Wilks’ lambda value measuring how well the function separate cases into groups. The latter value corresponds to the proportion of the total variance not explained by differences among the groups, meaning that a smaller value mirrors a higher discriminant ability of the function. The classification results indicate how well the three groups formed by the presence of a number of common species can be predicted by the species assemblage of rare species. The analysis was carried out in SPSS v20.
In a second analysis, we wanted to verify which of the environmental variables had the highest impact over the Odonata assemblages to investigate whether common and rare species reacted differently. To do this, all environmental variables were entered in a PCA using the varimax method, first to investigate any interrelationships between them and also to reduce the number of variables for the CCA analyses (below), we followed a similar procedure as that in Zhu et al. [Citation56]. We used the scree method to determine the number of factors retained. We used ln(n + 1) transformed data to normalize variation within and between variables. Associations with eigenvectors higher than 0.65 (positive or negative) were considered relevant in the evaluation. The analysis was carried out in SPSS v20.
We used the principal components derived from the environmental variables for two CCA analyses: In the first, we used the ln(n + 1) transformed relative abundance values for the rare species to identify species or species groups, which are strongly impacted on the PCs. The second analysis used the same type of dataset but for the common species. The CCA model used forward selection with 999 permutations. As the relationships between species and environmental variables are assumed to be unimodal in a CCA, rare species suffer by not occurring in a high enough number of sites [Citation56]. For the evaluation of the rare species, we therefore focused on species found in at least three sites in the dataset, removing the others from the analysis. The analyses were carried out in PAST v3.14 [Citation57].
Finally, we ran partial Spearman correlations between the number of common and rare species present at the sites, separated according to the span of occurrence of the rare species using the groups above. Data were controlled for the total number of species observed at the site. The objective here was to see whether the number of common species at a locality was correlated to the number of co-occurring rare species.
Results
The average number of common species at all sites was 3.90 ± 2.01 (standard deviation), while the average number of rare species was 2.43 ± 1.74 sd. The most species poor site with both species categories present had two (one rare and one common) species. The most species rich site had 13 (7, 6). We found at least one common and one rare species at 86% of the sites (N = 75). We found no common species at three of the sites (3.4%) and no rare species at nine sites (10.3%).
The discriminant analysis used two discriminant functions of which the first was significant (Eigenvalue = 5.134, Wilks’ lambda = 0.085, χ2 = 139.1, df = 112, p = 0.042) and explained 84.9% of the variance. In total, 89.7% of all habitats could be classified according to the number of common species present based on their rare species assemblage structures. Our classification separated all sites with 0–2 common species present (group 1) from the rest, while groups 2 and 3 were slightly mixed (with 9 of the localities wrongly classified; ). Sites with only a few common species could thereby be identified based on the rare species occurring, while this pattern becomes unclear for sites with a higher number of common species.
Figure 2. Discriminant analysis for three groups of common species using the relative abundance, (ln (n + 1) transformed) of the rare species as independent variables. 1 (black circles) = 0–2 common species, 2 (open circles) = 3–5 common species and 3 (black squares) = 6–8 common species at the locality. Group centroids marked as “x”. Several localities appear on top of each other and are not visible in the figure. Localities with only a few common species have a more distinct composition of rare species than localities with a higher number of common species.
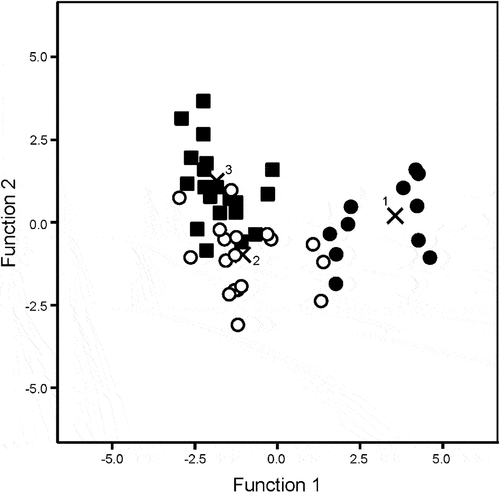
The scree method extracted four factors from the PCA explaining 68% of the total variance. Principal component 1 (PC1) explained 31.4% of the variance and was strongly associated with temperature and pH, but also to conductivity, turbidity, and dissolved O2. Further, it was negatively associated with sand (). PC2 explained 15.6% of the variance and was negatively associated with conductivity and salinity. PC3 explained 12.7% of the variance and was strongly associated with grassland and negatively associated with agriculture. The last factor, PC4, explained 8.4% of the variance and was associated with forestry (). There were no associations to the four original variables forest, water surface, human structures, and total dissolved solids.
Table 1. Results of principal component analysis on environmental variables measured at the collecting sites. Bold digits indicate high eigenvector values
Only the second axis in our CCA for the rare species was significant. It explained 30.25% (Eigenvalue 0.351, P = 0.042) of the cumulative variance (). Along axis 2, PC2 and 3 (positive to grassland, negative to agriculture, salinity, and conductivity) had the biggest effect on the occurrence of the rare species. The species Neoneura leonardoi Machado, 2005, Coryphaeashna perrensi McLachlan, 1887, Cyanallagma bonariense Ris, 1913, Oxyagrion hempeli Calvert, 1909, Macrothemis imitans Karsch, 1890, Miathyria marcella Selys in Sagra 1857 were positively correlated to these components, while Micrathyria longifasciata Carlvert, 1909, Orthemis attenuata Erichson, 1848, Macrothemis lutea Calvert, 1909, Telebasis theodori Navás, 1934, Tholymis citrina Hagen, 1867, Erythemis vesiculosa Fabricius, 1775, Rhionaeschna planaltica Calvert, 1952, as well as an undescribed species of Coenagrionidae are negatively correlated.
Figure 3. CCA plot based on the relative abundance of rare species and the four principal components. Most common species (empty circles) appear near the centre of the ordination and are only moderately affected by the PCs. A small set of species, e.g. Micrathyria longifasciata, Orthemis aequilibris and Dasythemis venosa are strongly (negatively) affected by PC1 and PC3 while the rest of the rare species (filled circles) are moderately affected.
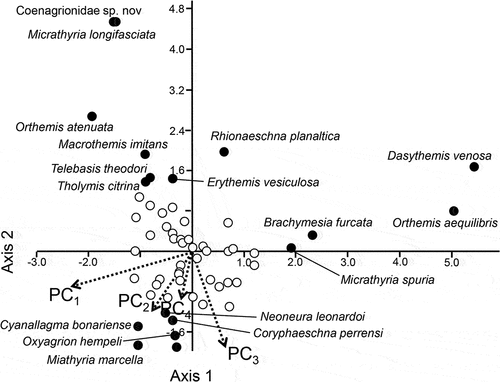
In the CCA for the common species, the first two axes were significant (Axis 1 explaining 62.32% of the cumulative variance, Eigenvalue 0.050, P = 0.001; axis 2 explaining 21.71%, Eigenvalue 0.017, P = 0.043; ). PC1 had the most pronounced effect on the occurrence of species, with higher values corresponding to high relative abundance of Ischnura fluviatilis Selys, 1876, Acanthagrion gracile Rambur, 1842, and Perithemis tenera (Say, 1839). Further, Pantala flavescens was negatively affected by PC1. PC1 corresponds to high temperature, pH, turbidity, and dissolved O2 and also to low amounts of sand. PC2 had the same direction as PC1 but the effects were much weaker. PC3 and 4 correlates positively to Erythrodiplax atroterminata Ris, 1911, and Erythrodiplax sp. (1), and negatively to Perithemis tenera. These components correspond to a high amount of forestry and grassland and a low amount of agriculture.
Figure 4. CCA plot based on the relative abundance of the common species and the four principal components. The small damselflies I. fluviatilis and A. gracile are positively associated with PC1 and 2 while the P. flavescens is negatively associated. Further, two Erythrodiplax species are positively associated with PC3 and 4, with P. tenera (Say, 1839) negatively associated. Erythrodiplax media is not affected by any of the variables corresponding to the PCs.
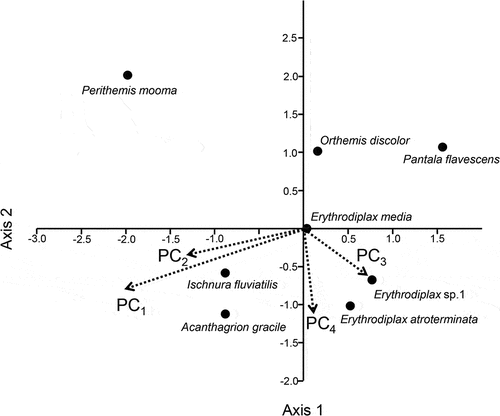
The number of rare species per site was not correlated with the number of common species (Spearman correlation; p = 0.115) when controlling for total species number. Looking at the abundance groups of common vs. rare species, we noted that when the number of common species per site was high (6–8 species; group 3) there was a negative correlation with the number of rare species at the sites (group c; Spearman correlation, p = 0.014, r = −0.49) when controlling for total species number. There was no correlation between the groups of few common/few rare species (1 vs. a; p = 0.57) or between groups 2 and b (p = 0.86). There were fewer rare species present when there was a higher number of common species present.
Discussion
In this study, we show two main findings: First, when only few common species occur at a site, the rare species composition differed from that at locations with a higher number of common species present. Second, localities with more than five common species harbored fewer rare species than localities with a low number of common species. Hence, the Odonata communities in the Pampa biome seems to a big extent be shaped according to the presence or absence of a small set of regionally common species.
In earlier papers, we showed how landscape variables, habitat types and ecoregions affect species diversity of Odonata in the Pampa [Citation40,Citation44]. Rivers and streams bordered by riparian forests and native grasslands harbored more complex communities than man-made lakes and agricultural areas. Community composition could to a big extent be explained by ecoregion, land cover and a small set of water quality variables. In the present study, we could establish that the species themselves have a big part in shaping and affecting the species assemblages. The fact that the density or number of common species is influencing the occurrence of the rare species in the ecosystem has been shown by e.g. Kunin and Gaston [Citation10] and Siqueira, Bini and Roque [Citation58]. There are several aspects that differentiate common from rare species; dispersal capabilities are generally higher in common species [Citation59,Citation60], their life-history strategies involve more competition and are often faster due to utilizing resources, which are temporary [Citation61–63], and in general, they have a wider environmental preference [Citation64]. Patch occupancy has also been considered one of the major factors regulating the proportion of rare vs. common species, since rare (and specialized) species are known to require a set of specific ecological features to inhabit a location [Citation65,Citation66] and with increasing anthropogenic impacts, specialized species are declining [Citation67]. If niche overlap between common and rare species is high at a location, the competition for resources affects foremost the rare species negatively, as these are inferior competitors and more sensitive to both intra- and interspecific competition [Citation68], as well as to changes in the environment [Citation69].
In an ecosystem with a high number of common species most niches will be occupied, making it difficult for populations of rare species to establish or persist; this was shown by us in the lower number of rare species at locations with six or more common species. Further, many common and generalist species grow more rapidly and have shorter life cycles than specialized species and are able to have several generations each year. One such example was shown in arid regions in southern Africa by Suhling, Jödicke, and Schneider [Citation70], who noted that big, highly mobile and multivoltine species (e.g. Pantala, Sympetrum, and Crocothemis) impaired the occurrence of rare and specialized species and prevailed over many slow-growing and rare species. Similar patterns were observed in other studies comparing rare to common species, e.g. [Citation12, Citation71]. These authors suggested that the balance of common vs. rare insect species might to a great extent be affected by the inflow of migrants; this may also be the case in our study area as many of our common species are known to be good dispersers (i.e. genera such as Pantala, Orthemis, and Tramea).
Besides the rare/common relation in the communities, we also report, for the first time, the effects of land use and environmental variables on the Odonata assemblages. We noted that common species are, to a higher degree than rare species, affected by environmental variables, while only the rare species reacted on the amount of grassland in relation to agriculture. We deduct that the factors influencing the common species would all be apparent in open, windy areas where species would need to tolerate a high mineral content and open lake areas (a man-made habitat in the area) with high nutrient concentration. These types of habitats are less common in areas with desertification. If we infer that most of the ancient/original land cover of the Pampa resembled the remaining grasslands we see today albeit changed due to many anthropogenic activities; cf. [Citation72], we may hypothesize that these habitats would be the most suitable for the original species composition in the biome. This has been shown in other studies comparing dragonfly assemblages from original patches of the environment to altered ones [Citation43,Citation73], and also in other taxonomic groups such as soil microbes [Citation74], birds [Citation75] and among groups occurring together: birds, amphibians, reptiles, and lepidopterans [Citation76]. The basic pattern we revealed here is that most of the assemblages seem to be composed of just a small selection of species and could even be considered species poor (2–13 species per locality in the area). This number may be compared to northern European environments [Citation34,Citation77], where the regional species pool is as low as 30–40 species compared to the 90 registered for the Pampa region in this study. It is therefore likely that a “normal” species assemblage in this biome is only a few species. As the original dominating type of environment here is the natural grasslands, we can also infer that the openness of these by itself is more favorable to species with high dispersal capabilities cf. [Citation35]; several such species were also common in our study. Thus, if anthropogenic changes favor species adapted to open environments they would easily spread and colonize the original Pampa habitat.
Previous studies on dragonfly species composition to test the effects of environmental changes caused by humans [Citation78] and on dragonflies with different life-histories affected differently by environmental changes [Citation79] corroborate our results, leading us to some speculation about species-specific ecological needs. Many rare species have been shown to be negatively affected by local scale changes [Citation69,Citation78], as well as by large scale human modifications of the environment. Also, the migratory or long-range dispersers depend on the quality of the features in the terrestrial landscape [Citation78], not only for migration purposes, but also for feeding and roosting [Citation35,Citation80]. There is still a severe shortage of ecological knowledge on most Neotropical dragonflies [Citation46], and, hence, we need to discuss environmental factors instead of specific requirements. Further, there is a similar lack of information whether the rare species also are threatened, since there are big gaps in species assessments compared to other regions [Citation81]. A first IUCN red list assessment has recently been made for all Neotropic odonates [Citation82], and all species in our study listed as Least concern except two, which are Data deficient (Heteragrion triangulare and Archaeogomphus densus). We should assume that some species may be threatened locally while not being threatened as a species, but until such assessments are presented we should infer protection measures based on threats to habitat.
Assessing the status of rare species is difficult also from another point: a rare species is disproportionately vulnerable to extinction in the short term [Citation83], due to human interference, habitat loss, degradation, or other factors. Further, the sampling methods used in this study may result in an incomplete detection of rare species at sites visited only a few times, e.g. in just one season. But rare species are always difficult to detect, and it is often impossible to detect all of them at any survey [Citation84]. Nevertheless, rare species may also be affected by factors, which are more difficult to quantify, mainly biotic ones; an example is our main result, which shows that the abundance of common species directly affects the density of the rare ones. Common species on the other hand respond to more conspicuous environment variables as shown by several authors looking at birds, fishes, and forest mammals [Citation25,Citation85,Citation86]. The higher susceptibility to biotic factors of rare species and their higher risk of extinction stimulates the choice of selecting them as study or reference species for conservation purposes, as shown in many publications e.g. [Citation87–89]. In this context, comparative studies of the interaction between rare and common species are important and could help in acquiring the knowledge needed regarding rare species survival. Given the vast number of rare species and how little is known about them [Citation10] vs. the generally small number of common ones, this is a promising field for further studies.
As Hodgson [Citation90] showed, the number of attributes determining species abundance is high and ecological factors therefore often complex. For Amazonian trees, environmental and spatial variables could explain a set of attributes of common species but not for rare ones [Citation91]. But certain sets of common and rare species have been shown to react to similar environmental gradients [Citation58], suggesting that species sorting indeed is the dominant process structuring communities. We showed that the number of common species seems to influence the rare species’ presence or absence in the assemblage, thus adding more support to the findings of Siqueira et al. [Citation58]. As rare species are more prone to go locally extinct and take longer time to re-establish [Citation28] and also react more on stochastic events [Citation92], further research on the role of common species in this process would be interesting, as well at investigating the partitioning between effects caused by the habitat and landscape versus effects of species composition.
We see a need for conservation to focus on creating heterogeneous landscapes, as odonate richness is affected by the different scales of land-use [Citation93]. Although the species we encountered are not threatened per se, we fear an ongoing homogenization, replacing the original grassland and riparian forest species with common generalists in the anthropogenic landscape. Therefore, when assessing the creation of new conservation areas, we should prioritize localities with only a small number of common species, where rare species would be more abundant. By providing this information derived from the distribution and occurrence of a freshwater group (the Odonata) in the Pampa biome, we expect to contribute to the maintenance and conservation of the aquatic environment.
Authors contributions
SR collected the field data. SR, EP, MD, and GS jointly developed the study concept and wrote the manuscript.
Acknowledgments
We thank UNIVATES for funding and logistical support, ICMBio for the collection permit and the landowners who kindly let us carry out our study on their properties.
Disclosure statement
No potential conflict of interest was reported by the author(s).
Additional information
Funding
References
- Leibold MA, Holyoak M, Mouquet N. The metacommunity concept: a framework for multi-scale community ecology. Ecol Lett. 2004;7(7):601–613.
- Brasil LS, Vieira TB, Oliveira-Júnior JMB, et al. Elements of metacommunity structure in Amazonian Zygoptera among streams under different spatial scales and environmental conditions. Ecol Evol. 2017;7(9):01–11.
- Soberón J. Grinnellian and Eltonian niches and geographic distributions of species. Ecol Lett. 2007;10(12):1115–1123.
- Lester SE, Ruttenberg BI, Gaines SD, et al. The relationship between dispersal ability and geographic range size. Ecol Lett. 2007;10(8):745–758.
- Taylor AD. Metapopulations, dispersal, and predator-prey dynamics: an overview. Ecol. 2009;71(2):429–433.
- Hanski I. Dynamics of regional distribution: the core and satellite species hypothesis. Oikos. 1982;38(2):210–221.
- Gutiérrez EE, Boria RA, Anderson RP. Can biotic interactions cause allopatry? Niche models, competition, and distributions of South American mouse opossums. Ecography. 2014;37(8):741–753.
- Rosindell J, Cornell SJ. Species-area curves, neutral models, and long-distance dispersal. Ecol. 2009;90(7):1743–1750.
- Thomas CD, Mallorie HC. Rarity, species richness and conservation: butterflies of the Atlas Mountains in Morocco. Biol Conserv. 1985;33(2):95–117.
- Kuning WE, Gaston KG. The biology of rarity: patterns, causes and consequences. Tree. 1993;8(8):298–301.
- Berg A, Tjernberg M. Common and rare Swedish vertebrates – distribution and habitat preferences. Biodiv Conserv. 1996;5(1):101–128.
- Magurran AE, Henderson PA. Explaining the excess of rare species in natural species abundance distribution. Lett Nat. 2003;422(6933):714–716.
- Novelo-Gutiérrez R, Gómez-Anaya JA. A comparative study of Odonata (Insecta) assemblages along an altitudinal gradient in sierra de Coalcomán Mountains, Michoacán, Mexico. Biodiv Conserv. 2009;18(3):679–698.
- Monteiro-Junior CS, Juen L, Hamada N. Effects of urbanization on stream habitats and associated adult dragonfly and damselfly communities in central Brazilian Amazonia. Landsc Urb Plan. 2014;127:28–40.
- Oliveira-Junior JMB, Shimano Y, Gardner TA. Neotropical dragonflies (Insecta: Odonata) as indicators of ecological condition of small streams in the eastern Amazon. Austr Ecol. 2015;40(6):733–744.
- van der Gucht K, Cottenie K, Muylaert K, et al. The power of species sorting: local factors drive bacterial community composition over a wide range of spatial scales. Proc Nat Acad Sci USA. 2007;104(51):20404–20409.
- Wagner H, Wildi O, Ewald KC. Additive partitioning of plant species diversity in an agricultural mosaic landscape. Landsc Ecol. 2000;15(3):219–227.
- Benton TG, Vickery JA, Wilson JD. Farmland biodiversity: is habitat heterogeneity the key? Trend in Ecol Evol. 2003;18(4):182–188.
- Hendrickx F, Maelfait JP, van Wingerden W, et al. How landscape structure, land-use intensity and habitat diversity affect components of total arthropod diversity in agricultural landscapes. J Appl Ecol. 2007;44(2):340–351.
- Renner S, Périco E, Sahlén G. Testing dragonflies as species richness indicators in a fragmented subtropical Atlantic forest environment. Neot Entomol. 2016;43(3):231–239.
- Chesson P. Mechanisms of maintenance of species diversity. Ann Rev Ecol Syst. 2000;31(1):343–366.
- Tilman D. Competition and biodiversity in spatially structured habitats. Ecology. 1994;75(1):2–16.
- Bulmer MG. On fitting the poisson lognormal distribution to species-abundance data. Biometrics. 1974;30(1):101–110.
- Rabinowitz D. Seven forms of rarity. In: Synge H, editor. The biological aspects of rare plant conservation. New York (NY): Wiley; 1981. p. 205–217.
- Lennon JJ, Koleff P, Greenwood JJD, et al. Contribution of rarity and commonness to patterns of species richness. Ecol Lett. 2004;7(2):81–87.
- Mouillot D, Graham NAJ, Villéger S. A functional approach reveals community responses to disturbances. Trends Ecol Evol. 2013;28(3):167–177.
- Pimm SL, Jenkins CN, Abell R, et al. The biodiversity of species and their rates of extinction, distribution, and protection. Sci. 2014;344:1246752.
- Volkov I, Banavar JR, Hubbell SP, et al. Neutral theory and relative species abundance in ecology. Nat. 2003;424(6952):1035–1037.
- Gärdenfors U. Classifying threatened species at national versus global levels. Trend in Ecol Evol. 2001;16(9):511–516.
- Gauthier P, Debussche M, Thompson JD. Regional priority setting for rare species based on a method combining three criteria. Biol Conserv. 2010;143(6):1501–1509.
- Juen L, Cabette HSR, de Marco P. Odonate assemblage structure in relation to basin and aquatic habitat structure in Pantanal wetlands. Hydrobiol. 2007;579(1):125–134.
- de Block M, Pauwels K, van den Broeck M, et al. Local genetic adaptations generates latitude-specific effects of warming in predator-prey interactions. Glob Ch Biol. 2013;19(3):689–696.
- Al Jawaheri R, Sahlén G. Negative impact of lake liming programmes on the species richness of dragonflies (Odonata): a study from southern Sweden. Hydrobiol. 2016;788(1):99–113.
- Sahlén G, Ekestubbe K. Identification of dragonflies (Odonata) as indicators of general species richness in boreal forest lakes. Biodiv Conserv. 2001;10(5):673–690.
- Corbet PS. Dragonflies: behavior and ecology of Odonata. Ithaca, USA: Cornell University Press; 1999.
- Renner S, Périco E, Sahlén G, et al. Dragonflies (Odonata) from the Taquari River valley region, Rio Grande do Sul, Brazil. Check List. 2015;11(5):1740.
- Overbeck GE, Hermann JM, Andrade BO, et al. Restoration ecology in Brazil – time to step out of the forest. Nat Conserv. 2013;11(1):92–95.
- MMA - Ministério do Meio Ambiente. Maps of plant distribution in the Brazilian biomes [Inetnet]. Brasília (DF): Governo do Brasil; 2009 [Cited 2019 Feb 25]. Available from: http://www.mma.gov.br/estruturas/sbf_chm_rbbio/_arquivos/mapas_cobertura_vegetal.pdf
- Behling H, Pillar VP, Orlóci L, et al. Late Quaternary Araucaria forest, grassland (campos), fire and climate dynamics, studied by high-resolution pollen, charcoal and multivariate analysis of the Cambará do Sul core in southern Brazil. Palaeogeogr Palaeoclim Palaeoecol. 2004;203(3–4):277–297.
- Bencke GA, editor. Brasília (DF): Ministério do Meio Ambiente; 2009. Pillar VP, Müller SC, Castilhos ZMS, et al. editors. Diversidade e conservação da fauna dos campos do sul do Brasil. Campos Sulinos - conservação e uso sustentável da biodiversidade. p. 101–121.
- INPE - Instituto Nacional de Pesquisas Espaciais [Internet] Brasília (DF): Centro de Previsão de Tempo e Estudos Climáticos, Banco de Dados Meteorológicos. Ministério da Ciência e Tecnologia; 2009 [cited 2020 mar 19]. Available from: http://bancodedados.cptec.inpe.br/
- Scheiner SM. Measuring pattern diversity. Ecology. 1992;73(5):1860–1867.
- Renner S, Périco E, Dalzochio MS, et al. Ecoregions within the Brazilian Pampa biome reflected in Odonata species assemblies. Austr Ecol. 2018;44(3):1–12.
- Renner S, Périco E, Dalzochio MS, et al. Water body type and land cover shape the dragonfly communities (Odonata) in the Pampa biome, Rio Grande do Sul, Brazil. J Ins Conserv. 2018;22(1):113–125.
- Garrison RW, von Ellenrieder N, Louton JA. Dragonfly genera of the new world: an illustrated and annotated key to the Anisoptera. Baltimore (USA): The John Hopkins University Press; 2006.
- Garrison RW, von Ellenrieder N, Louton JA. Damselfly genera of the new world: an illustrated and annotated key to the Zygoptera. Baltimore (USA): The John Hopkins University Press; 2010.
- Heckman CW. Encyclopedia of South American aquatic insects: Odonata - Anisoptera. Dordrecht (The Netherlands): Springer; 2006.
- Heckman CW. Encyclopedia of South American aquatic insects: Odonata - Zygoptera. Washington (DC): Springer; 2010.
- Lencioni FAA. The damselflies of Brazil: an illustrated identification guide II - coenagrionidae family. São Paulo (Brazil): All Print Editora; 2006.
- Lencioni FAA. Damselflies of Brazil: an illustrated identification guide, Southeast region. Jacareí (Brazil): Editora do Autor; 2017.
- Hartley S, Kunin WE. Scale dependency of rarity, extinction risk, and conservation priority. Conserv Biol. 2003;17(6):1559–1570.
- Fattorini S. Relations between species rarity, vulnerability, and range contraction for a beetle group in a densely populated region in the Mediterranean Biodiversity Hotspot. Conserv Biol. 2013;28(1):169–176.
- Suhling F, Sahlén G, Martens A, et al. Dragonfly assemblage composition and diversity in arid tropical environments: a case study from western Namibia. Biodiv Conserv. 2006;15(1):311–332.
- Moran PAP. Notes on continuous stochastic phenomena. Biometrika. 1950;37(1–2):17–23.
- Press SJ, Wilson S. Choosing between logistic regression and discriminant analysis. J Am Stat Assoc. 1978;73(364):699–705.
- Zhu X, Huang Z, Shen HT, et al. Dimensionality reduction by mixed kernel canonical correlation analysis. Patt Recogn. 2012;45(8):3003–3016.
- Hammer Ø. PAST, PAleontological STatistics, Version 3.06. Oslo: Natural History Museum; 2015.
- Siqueira T, Bini LM, Roque FO. Common and rare species respond to similar niche processes in macroinvertebrate metacommunities. Ecogr. 2012;35(2):183–192.
- Suhling I, Suhling F. Thermal adaptation affects interactions between a range-expanding and a native odonate species. Freshw Biol. 2013;58(4):705–714.
- Borthagaray AI, Barreneche JN, Sebastian A, et al. Modularity along organism dispersal gradients challenges a prevailing view of abrupt transitions in animal landscape perception. Ecogr. 2014;37(6):564–571.
- Nylin S, Gotthard K. Plasticity in life-history traits. Ann Rev Entomol. 1998;43(1):63–83.
- Johansson F, Rowe L. Life history and behavioural responses to time constraints in a damselfly. Ecol. 1999;80(4):1242–1252.
- Therry L, Nilsson-Örtmann V, Bonte D, et al. Rapid evolution of larval life history, adult immune function and flight muscles in a poleward-moving damselfly. J Evol Biol. 2014;27(1):141–152.
- Cornwell WK, Ackerly DD. A link between plant traits and abundance: evidence from coastal California woody plants. J Ecol. 2010;98(4):814–821.
- Kassen R. The experimental evolution of specialists, generalists, and the maintenance of diversity. J Evol Biol. 2002;15(2):173–190.
- Paulson D. The importance of forests to neotropical dragonflies. In: Cordero-Rivera A, editor. Forest and Dragonflies. Sofia: Pensoft Publishers; 2006:79–101.
- Clavel, Julliard R, Devictor V. Worldwide decline of specialist species: toward a global functional homogenization? Front Ecol Env. 2011;9(4):222–228.
- Yenni G, Adler PB, Ernest KM. Do persistent rare species experience stronger negative frequency dependence than common species? Gl Ecol Biogeogr. 2017;26(5):513–523.
- Renner S, Périco E, Sahlén G. Man-made lakes form species-rich dragonfly communities in the Brazilian Atlantic Forest (Odonata). Odonatologica. 2016;45(3–4):135–154.
- Suhling F, Jödicke SW. Odonata of African arid regions – are there desert species. Cimbebasia. 2003;18:207–224.
- Southwood TER. The croonian lecture 1995. Natural communities: structure and dynamics. Phil Trans Royal Soc London. 1996;351(1344):1113–1129.
- Santos S, Silva LG. Mapeamento por imagens de sensoriamento remoto evidencia o bioma Pampa Brasileiro sob Ameaça. Boletim geográfico. 2007;29(2):49–57.
- Juen L, Shimano Y, Gardner TA. Neotropical dragonflies (Insecta: Odonata) as indicators of ecological condition of small streams in the eastern Amazon. Austr Ecol. 2015;40(6):733–744.
- Lupatini M, Jacques RJS, Antoniolli ZI. Land-use change and soil type are drivers of fungal and archaeal communities in the Pampa biome. W J Microbiol Biotech. 2013;29(2):223–233.
- Mörtberg UM. Resident bird species in urban forest remnants; landscape and habitat perspectives. Landsc Ecol. 2001;16(3):193–203.
- Atauri JA, de Lucio JV. The role of landscape structure in species richness distribution of birds, amphibians, reptiles and lepidopterans in Mediterranean landscapes. Landsc Ecol. 2001;16(2):147–159.
- Koch K, Wagner C, Sahlén G. Farmland versus forest: comparing changes in Odonata species composition in western and eastern Sweden. Ins Conserv Div. 2014;7(1):22–31.
- Raebel EM, Merckx T, Feber RE, et al. Multi-scale effects of farmland management on dragonfly and damselfly assemblages of farmland ponds. Agric Ecosyst Env. 2012;161:80–87.
- Kadoya T, Suda S, Tsubaki Y, et al. The sensitivity of dragonflies to landscape structure differs between life-history groups. Landsc Ecol. 2008;23(2):149–158.
- Samways MJ, Steytler NS. Dragonfly (Odonata) distribution patterns in urban and forest landscapes, and recommendations for riparian management. Biol Conserv. 1996;78(3):279–288.
- Clausnitzer V, Kalkman VJ, Ram M. Odonata enter the biodiversity crisis debate: the first global assessment of an insect group. Biol Conserv. 2009;142(8):1864–1869.
- IUCN. The IUCN red list of threatened species. Version 2021-3. [cited 2022 Jan 16]. https://www.iucnredlist.org
- Gaston KJ. Biodiversity and extinction: the importance of being common. Progr Phys Geogr. 2008;32(1):73–79.
- Mao CX, Colwell RK. Estimation of species richness: mixture models, the role of rare species, and inferential challenges. Ecol. 2005;86(5):1143–1153.
- Sheldon AL. Conservation of stream fishes: patterns of diversity, rarity, and risk. Conserv Biol. 1998;2(2):149–156.
- Arita HT, Robinson G, Redford KH. Rarity in neotropical forest mammals and its ecological correlates. Conserv Biol. 1990;4(2):181–192.
- Caughley G, Gunn A. Conservation biology in theory and practice. Oxford (UK): Blackwell Science; 1995.
- Gaston KJ, Blackburn TM. Pattern and process in macroecology. Oxford (UK): Blackwell Science; 2000.
- Baillie JEM, Hilton-Taylor C, Stuart SN. IUCN red list of threatened species. a global species assessment. Switzerland: Gland; 2004.
- Hodgson JG. Commonness and rarity in British butterflies. J Appl Ecol. 1993;30(3):407–427.
- Bispo PC, Balzter H, Malhi Y, et al. Drivers of metacommunity structure diverge for common and rare Amazonian tree species. PloS ONE. 2017;1(11):e0188300.
- Zhang X, Pu Z, Li Y, et al. Stochastic processes play more important roles in driving the dynamics of rarer species. J Plant Ecol. 2015;9(3):328–332.
- Foster SE, Soluk DA. Protecting more than the wetland: the importance of biased sex ratios and habitat segregation for conservation of the Hine’s emerald dragonfly, Somatochlora hineana Williamson. Biol Conserv. 2006;127(2):158–166.