ABSTRACT
Precision medicine is the selection of appropriate treatments to individual patients on the basis of molecular predictive factors and clinical presentation. In this light, multiple myeloma (MM) represents a challenging area of investigation for its biological complexity and close interplay with cell components of the bone marrow microenvironment. Here, we discuss personalized strategies for MM treatment with specific focus to the integrated molecular profiling, the emerging role of non-coding RNA therapeutics and novel pharmacogenomics tools. Precision medicine of MM is still in its infancy but the rapidly increasing knowledge and technological advancements will offer major opportunities for crucial changes of the treatment paradigm in the next future.
Precision medicine in cancer treatment
The heterogeneity in the inter-individual drug response, which influences both therapeutic efficacy and toxicity, is an important caveat for cancer treatment due to a variety of different factors related to the complex interplay between drug-specific, tumor-specific and patient-specific features. Many efforts have been recently made in the novel field of systems pharmacology and pharmacogenomics (PGs) in order to realize the goal of ‘precision medicine’. Frequently, the terms ‘personalized’ or ‘individualized medicine’ are used interchangeably and the term ‘precision medicine’ indeed represents a new attitude to decision-making in disease management. In fact, the paradigm of ‘precision medicine’ interconnects traditional clinical knowledge with the increasing availability of validated predictive biomarkers. Cancer is a disease of the genome, and growing evidence confirms that specific genomic abnormalities underlie tumor biology and treatment response. The rapid introduction of more effective targeted drugs in clinics has led to novel treatment strategies tailored to the genetic profile of each patient and of its own cancer. In parallel, advancements in genomic and biomarker testing technologies are rapidly leading to extensive use in clinical research, which is made possible also by cost lowering [Citation1,Citation2]. The resulting effect is a huge widening of the availability of personalized treatment approaches in the daily practice [Citation3]. The design of clinical trials based on selection of patients carrying somatic or germline mutations have indeed produced remarkable advances in oncology by maximizing treatment benefits and reducing toxicity. It is now clear that a biomarker must be measurable and linked to relevant clinical outcomes, must be of demonstrated clinical utility and validated for its use as a predictive tool or as surrogate end point in clinical trials. Moreover, a biomarker must be actionable for quality and clinical validity by a diagnostic assay and for treatment decision-making. The goal of precision medicine in oncology is, therefore, to pair patients with a drug on the basis of the specific tumor’s molecular profile and genetic landscape instead of relying just on tumor pathology in order to improve the clinical benefit and minimize treatment side effects. Today, high-throughput ‘omics’ technologies, including next-generation sequencing, transcriptomics and proteomics together with the construction of standardized models for data integration, can help clinicians to identify factors associated with differential drug response, both in terms of tumor’s and carrier’s genetic profile. Although distinct, these research areas complement each other and the integration of genomic and clinical data represents the hallmarks of precision medicine (patients, clinicians, researchers, bioinformatics and clinical laboratories) that together constitute a working network that provides the logic framework to direct decision-making (). An example of newer trials design that considers both intra- or inter-patient tumor molecular heterogeneity is the next-generation ‘platform’ clinical trials, designed on the hypothesis that the presence of a molecular marker predicts response to a targeted therapy independently of tumor histology and permits to test the action of multiple drugs in the study of a single disease. In ‘umbrella trials’, patients with a pathologically characterized cancer are assigned to one of the treatment arms of the single trial, according to the identified tumor genetic mutation. The goal of ‘umbrella trials’ is to test whether the effectiveness of a precision choice leads to better outcomes than standard of care approaches. In ‘basket trials’, patients with different tumors are enrolled in a trial irrespective of tumor histology and treated focusing on few molecular lesions and on the specific targeted treatment [Citation4]. In fact, these studies are designed to investigate the effect of a single drug on a single mutation in several cancer types and offer the possibility to integrate a conventional clinical trial design with the evolving genomic data and contribute therefore to pair a drug with a validated biomarker in a specific patient. However, important caveats and limitations of basket trials are still the impact of disease heterogeneity and complexity and the influence of epigenetic changes on patient response to therapy. It is becoming clear that a molecular lesion can act as driver druggable target because it produces tumor addiction, but can be a passenger lesion in other molecular contexts. The actionability of BRAF and c-Kit mutations appears highly context-dependent, and this crucial finding underlines the need of multilayered analysis tools as integrative genomics [Citation5,Citation6] (see below).
Figure 1. The chart depicts the interaction and integration of knowledge that, with different perspective, role and incentive, may have important prognostic implications and contribute to a personalized therapy. Precision medicine should be considered as a means of providing the best available health care for a population by identifying the needs and improving the outcomes of individual patients.
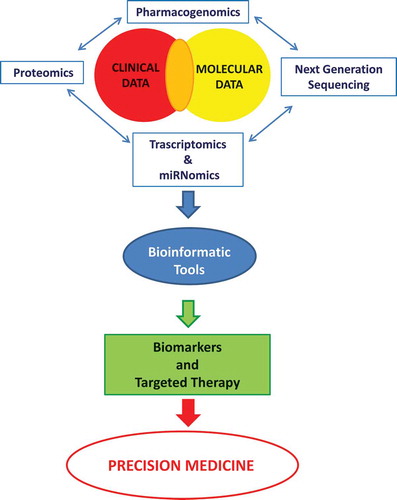
Multiple myeloma, a microenvironment prototypical disease
Multiple myeloma (MM) is a clonal late B-cell malignancy in which plasma cells (PCs) accumulate in the bone marrow (BM). It accounts for 10% of all hematological cancers and discloses a worldwide increasing incidence. MM is a heterogeneous disease characterized by a complex clinical presentation ranging from the pre-malignant monoclonal gammopathy of undetermined significance (MGUS), to smoldering MM, to symptomatic MM, and, finally, extra-medullary MM/plasma cell leukemia. Despite the recent remarkable improvement of the treatment armamentarium, the availability of a variety of investigational therapeutics and novel platforms for advanced preclinical studies [Citation7–Citation16], MM has still a poor prognosis in the majority of cases. The human BM microenvironment (HBMM) plays a critical role in the pathogenesis of MM. The close interplay between MM cells and cell components of the HBMM, which includes BM stromal cells (BMSCs), vascular endothelial cells, osteoclasts and osteoblasts, modulates and sustains growth, survival and the development of drug resistance of MM cells. The knowledge of the complex network of interactions within the HBMM provides a prototypical framework for the design of novel therapeutic strategies targeting both tumor cells and the HBMM. So far, many approaches have been used to identify factors within the HBMM and intrinsic to MM cells mediating cell–cell interactions. In addition to direct interaction among these cell components within the HBMM, a network of soluble and druggable factors, including IL-6 (interleukin), IGF-1 (insulin-like growth factor), B-cell activating factor/a proliferation-inducing ligand and others, play a key role in MM–HBMM axis [Citation17–Citation22]. All these factors regulate cell growth, survival and drug response due to both tumor cell binding, cytokines induction and angiogenesis within the HBMM. New therapeutic targets have been identified and validated by in vitro and in vivo studies finalized to delineate the mechanism of MM cell–environmental interactions. All these studies underline the relevance of targeting molecules involved in cell survival and migration, as well as in drug resistance pathways. The knowledge so far accumulated outlines the molecular mechanisms whereby novel and conventional therapies inhibit growth, survival and drug resistance signaling in MM cells and/or in BM environment. The general hypothesis herein is that combination of different classes of drugs can offer the opportunity to eradicate MM disease only if based on validated targets. At this aim, the global view of molecular mechanisms will provide the framework for balanced clinical trials on combinatory approaches to overcome drug resistance and improve patient outcome, as well as for development of the next generation of more selective and less toxic therapies. The genome-wide profiling of MM and normal PCs, as well as of cells from patients with MGUS, has provided knowledge on the pathogenesis and progression of the MM disease and has disclosed molecular signatures associated with the disease. Therefore, it offered the rationale for the development of a new classification system and risk scores in MM and established clinically relevant patient stratification criteria with therapeutic and prognostic inference. So far, the historical staging systems including the Durie–Salmon system and the International Staging System (ISS) have not been used for treatment selection. Several studies have identified novel risk and prognostic factors mostly based on MM tumor biology, tumor burden and patient-related factors. It is important to define the role of these factors in the treatment choice. In fact, the combination of these prognostic factors into a risk-adapted model has generated three risk categories as defined by mSMART guidelines at Mayo Clinic, which are continuously updated [Citation23]. By these criteria, patients with aggressive course and brief disease-free intervals are defined at high risk, whereas others with a more indolent course are considered at standard risk. Moreover, patients with t(4;14) abnormality have an intermediate risk level and are considered responsive to bortezomib-based therapy.
On these bases, MM cannot be any more considered one disease but rather a mosaic of different entities, with each one of the subtypes defined by the specific genetic and cytogenetic aberrations. These defined groups are unique entities and unlikely to lose their characteristics over time. Moreover, although morphologically similar, the several subtypes of the disease, identified at genetic and molecular level, are associated with specific clinic-pathological features and different outcome. The 70-gene prognostic signature, developed by the University of Arkansas for Medical Sciences (UAMS) and commercialized under the name ‘MyPRS®’ (Myeloma Prognostic Risk Score; Signal Genetics Inc., Carlsbad, CA, USA) is a standardized method of MM prognostication used to identify high-risk disease [Citation24] and contribute to guide therapeutic interventions [Citation25,Citation26]. The IFM15 (Intergroupe Francophone du Myélome) and the MM Survival Index14 developed from gene expression profiling (GEP) data [Citation27], respectively, identified 15 genes strongly associated with survival and 14 genes associated with low-risk disease. All these innovative tools are of help to redefine prognostic stratification and might be useful for prediction of molecular targeted agents activity. They clearly improve the information provided by conventional MM taxonomy tools, which are basically constructed on clinical or cytogenetic features or staging systems [Citation28].
In the last decade, important advances in molecular cytogenetics and global genomic studies brought about a significant advancement in the comprehension of MM pathogenesis. Recognition of the paradigmatic role exerted by the HBMM has recently allowed the design of therapeutic strategies targeting MM cells together with the nontumor compartment of the HBMM.
Recent findings have clearly demonstrated that MM includes an array of different clones, which may be individually correlated to different clinical features in terms of proliferative activity, relapse or progression [Citation29]. This branched Darwinian evolutionary model is presently under active investigation and will be a main topic for future development in precision medicine of MM [Citation30].
In fact, expression profiling approaches and the analysis of genetic alteration recurrence have identified genes involved in ‘druggable’ pathways and prompted the rationale to profile individual patients and test combined therapies to overcome drug resistance and avoid toxicity.
Novel agents, including immune-modulatory drugs thalidomide and lenalidomide, and the proteasome inhibitor bortezomib, have shown significant antitumor activity in clinical studies involving patients with relapsed or refractory myeloma. The microarray profiling has been used to define the preclinical rationale for combining bortezomib with conventional DNA-damaging agents, stress response inhibitors and with the immune-modulatory analog lenalidomide. Additionally, GEP have revealed how different molecules could trigger resistance to novel therapies in MM [Citation31]. In this light, identification of novel predictive biomarkers is a compelling issue. A variety of recent studies have provided novel information, which might lead to validation of precision approaches to drive MM treatment. It has been reported that a novel mechanism of action of lenalidomide resides in targeting of the activity of E3 ubiquitin ligase. This effect drives to degradation of specific targets [Citation32,Citation33]. Moreover, Moreaux et al. built a DNA methylation score highly predictive of decitabine activity [Citation34]. Delmore et al. disclosed the activity of BET bromodomain inhibitors in myelocytomatosis oncogene (MYC) hyperactivated MM cells [Citation35]. Moreover, Lohr et al. observed frequent mutations in KRAS, NRAS, BRAF, FAM46C, TP53, and DIS3, further underscoring high heterogeneity in activation or inhibition of genes related to targeted therapy activity [Citation36]. Cottini et al. have provided proof of concept of a synthetic lethality approach based on enhancement of oxidative stress and blockade of replicative cell response. This result might lead to a highly promising therapeutic approach [Citation37]. However, despite these recent provocative findings and significant therapeutic advances, MM remains incurable and new drugs or clinical strategies are needed to overcome the HBMM-mediated drug resistance. To this end, an extensive evaluation of molecular data obtained by high-throughput technologies and the development of new approaches for integration in a comprehensive view might provide significant advances to define cell targeting of MM and/or HBMM cells, which finally may result in improved outcome of MM patients.
An enhanced understanding of the molecular pathology of MM could be gained from genomic studies with the final aim to develop treatments that target discrete molecular subclasses of tumors. For example, BRAF V600E mutated MM tumors could take advantage from BRAF inhibitors [Citation38]. Current challenges include how to advance and implement targeted drug development strategies. The precision medicine vision, as previously described, relies on delivering the most appropriate therapy to patients on the basis of clinical and molecular features of their specific disease. The development of therapeutic agents that target molecular mechanisms is driving innovation in clinical trial strategies. Although progress has been made, modifications to existing core paradigms in oncology drug development will be required to fully accomplish the promise of precision medicine. MM clinical trials need at this point to be designed on novel robust validated biomarkers and need to rely on this paradigm change. MM cannot be anymore considered as a unique disease, and clinical trials must rely on this emerging concept. In this context, RNA-based therapy is a promising challenge in MM precision medicine.
miRNA therapy of MM
MicroRNAs (miRNAs) are short RNA molecules, 18–22 nucleotides in length, highly conserved during evolution across different species and with tissue specific biology. Despite the discovery of miRNAs being not recent, only in the last few years the relevance and the therapeutic properties of miRNAs have emerged. miRNAs are post-transcriptional regulators of gene expression, which play a central role in the complex circuitry of normal and aberrant cell biology. Aside from the long-standing recognition of the other categories of tumor genomic alterations, miRNAs dysregulation is recently considered an additional genomic intermediary in cancer development. In fact, following the understanding of how these molecules work and regulate a number of different targets, they can offer the opportunity to finely tune the transcriptome and the proteome [Citation39].
Each miRNA can target hundreds of mRNAs in a coordinate fashion as part of a basic network that regulates a variety of cellular functions. It is now becoming clear that several human diseases are indeed related to dysregulated expression of miRNAs. It is, therefore, reasonable that one approach to the therapy of human cancers should rely on the reconstitution of deficient miRNAs or on the antagonism of aberrantly expressed miRNAs. This rapidly developing field can be defined as ‘miRNAs target therapy’ and could be included in the general scenario of targeted therapy. Although several molecular-targeted agents have produced benefits for the treatment of human cancer, in many cases the length of response has been modest. The miRNAs therapy offers the fascinating expedience to shift from single target to a multiple target-based approach, with the potential to produce major perturbation in tumor cells.
Recently, in addition to insights provided in the genetic classification of MM, specific miRNA signatures have been defined and associated with the different subtypes of the disease [Citation40–Citation42]. The role of this class of epigenetic regulators has been investigated in order to identify their possible role in the malignant transformation of the PCs. Seckinger et al. detected few differences in miRNA profiles of normal and malignant PCs; these differentially expressed miRNAs strongly impact MM survival [Citation43].
Dysregulated miRNA expression in MM cells, which is associated to cytogenetic abnormalities and correlated with gene-expression changes characteristic of MM genetic subtypes, has provided the rationale for the design of new therapeutic strategies in this disease [Citation11,Citation44–Citation47]. In fact, for many malignancies, including MM, recent experimental evidence has demonstrated addiction to oncogenic miRNAs (oncomiRs), and this finding has led to the design of therapeutics specifically directed against these oncomiRs. The alternative approach, which is defined as ‘miRNAs as a drug’, offers the opportunity of reprogramming tumor cells by the delivery of synthetic mature miRNA mimics. For example, in MM, several miRNAs are deeply upregulated or downregulated in the tumor cells and it has been demonstrated that their inhibition [Citation48–Citation56] or reconstituted expression [Citation57–Citation66] affects cell proliferation and survival.
The theoretical assumption of both these therapeutic approaches has been demonstrated in multiple proof-of-concept preclinical studies by investigating the activity of miRNA mimics or inhibitors on tumor cells. The miRNA replacement has been demonstrated as effective in a variety of preclinical models of human cancers. Among several miRNAs, the study of miR-34a mimics is presently the more advanced in terms of clinical translation [Citation67,Citation68]. However, the most important bottleneck in the development of this approach resides in the need of nanocarriers for systemic delivery.
On the other hand, the inhibition of single and specific miRNAs proceeds from the synthesis of stable modified oligonucleotides with antisense activity. The progress of the chemistry of antisense oligonucleotides leads to the development of molecules with extremely improved stability and very efficient activity as target sequesters [Citation69].
So far, a plethora of miRNA mimics or inhibitors have been investigated in preclinical models of MM and are summarized in .
Table 1. miRNA mimics or inhibitors that have been investigated in preclinical models of MM.
Integrative genomics in MM
The role of different stimuli and how BMSCs may affect MM cell growth, as well as the different activating and/or inhibiting signals associated to specific genetic aberrations cannot be adequately distinguished by individual analysis platforms: GEP and miRNA profiling. At the same time, it is necessary to go deeply to define different levels of interaction between the two classes of actors, MM and HBMM cells, and thus provide a global picture of the molecular patterns across the entire genome. Large-scale study or high-throughput analyses need integrated platforms to bare these tangled biological systems. By these new approaches applied to MM environment studies, it can be attempted to dissect the role of each component of the HBMM simultaneously and identify which cell component expresses a gene of interest. This will lead to the identification of specific molecular events and allow the design of targeted therapies. In addition, a more detailed knowledge of MM and HBMM biology, as well as the understanding of the cross-talk between these two biological compartments, provides essential advantages for discovering new targets and understanding the mechanism of target therapies for best clinical use.
In the recent years, the development and the extensive use of novel technological platforms in molecular biology have led to the production of a large amount of data about different disciplines of the ‘omic’ world. Classical approaches of bioinformatic analysis were focused on the use of single classes of data (i.e. genomic data or proteomic data). It has been made clear that the integration of data at different levels could produce more relevant results. Consequently, many different approaches have focused on such kind of integration, leading to the rise of a novel discipline, often referred to as integromics, or integrated analysis of ‘omic’ data, in which computer science, bioinformatics and network theory play a synergistic role. This discipline focuses on the elucidation of basic principles of the interplay among different biological molecules (such as proteins or genes) [Citation70–Citation72]. It usually employs a common approach for findings that share a common flow of information. The flow starts from gathering data of different data sources from publicly available data sets often available on the web. Then, data may be integrated also with ad hoc performed wet-lab experiments. All data are finally integrated into a single network model and the model is analyzed with different algorithms tailored to the specific application. For instance, a main class of experiments focuses on the elucidation on a system-level scale of mechanisms of the regulation of gene expression. From a biological point of view, it is clear that main actors of this process are mRNAs, miRNAs and transcription factors (TFs) that play an interacting role in the regulation of gene expression that results in variable levels of gene transcripts and proteins.
The integration of such data sets is usually based on the use of the formalism provided from graph theory depicted in . As a result, bioinformatic approaches for the integrated analysis usually build comprehensive graphs in which nodes are mRNAs, miRNAs and TFs (or other molecules) and edges represent the interactions among them. Edges fall into two main categories: (i) activation edges modeling the interplay among molecules among whose one may increase the level of another one, and (ii) inhibition edges that model the action of inhibition. The analysis of such graphs is made with different algorithms tailored to the specific application. For instance, the individuation of small and connected subgraphs with three different classes of nodes is usually employed for the individuation of loops (feedback and feed-forward) in which the regulation of the expression of a gene is a synergistic action of both miRNA and TF.
Figure 2. Researcher use different samples to produce his/her experimental data. In parallel, previous wet lab experiments or computational experiments (e.g., prediction algorithms) have produced different knowledge banks. The network-based integrated analysis takes as input both user experimental data and knowledge banks and produces biologically meaningful knowledge using appropriate theoretical models.
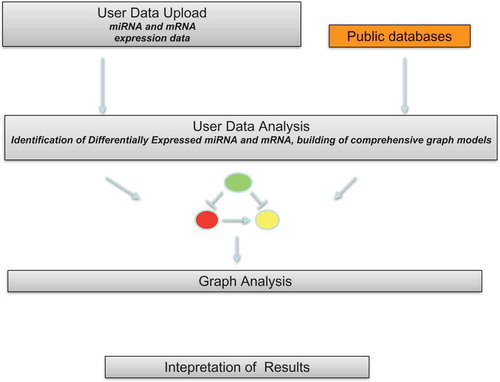
There is a need to present in a systematic catalog all the available methods. Main approaches of analysis share some specific characteristics. First of all, the approaches share the use of an internal knowledge base containing information gathered from literature and databases. The knowledge base usually stores association among mRNAs, miRNAs and TFs modeled as graphs. This internal knowledge base is used for guiding the analysis of experimental data. Second, the approaches enable the user to take external experimental data sets from a pool of samples extracted from patients in case–control or time-series experiments. Then, data of knowledge bases are used to build the association graph from experimental data. This association graph is then mined to extract knowledge.
We here briefly recall some main approaches of analysis focusing only on freely available tools. We should note that the literature also reports an approach of integration available for Ingenuity Pathway Analysis (IPA®, Qiagen, Hilden, Germania) software that we do not report here since it is not freely available.
MAGIA2 is a freely available web server for the integrated analysis of both genes and microRNA. As internal knowledge base, it uses eight different databases of miRNA/mRNA associations used to build the null models, that is, associations that are known by literature, two experimentally validated databases of TF–miRNA interactions and a single TF–gene interaction database. dChip-GemiNi (Gene and miRNA Network-based Integration) [Citation73] is a web server freely available for academic users, which is able to integrate and analyze paired miRNA–mRNA expression data. mirConnX is based on a broader perspective with respect to the previous approaches since it uses a genome-wide approach and it is tailored for human and mouse [Citation74]. The workflow of analysis is based on the comparison of the whole networks of associations among genes, TFs and mRNAs. IntegraMiR is a tool that receives as input mRNA and miRNA expression data, obtained from samples that are subdivided into two classes (e.g. controls vs. cases) [Citation75]. It is integrated with Bioconductor to analyze experimental data by determining differentially expressed genes and to perform gene set enrichment analysis. Then, associations among mRNA and miRNA are derived considering their individual expression levels (i.e. considering pairs of mRNA–miRNA whose regulation is inversely correlated) or through their target interactions via functional analysis through literature and databases.
In a parallel way, literature reports some examples of works that followed a similar approach of analysis that has been demonstrated as proof of principle obtaining relevant results, without the implementation of supporting software. These approaches have some similar characteristics: the use of open-source or available tools in reproducible and standardized workflow of analysis.
The paper by Van Iterson et al. presented a workflow of analysis based on open-source bioinformatics tools and the consequent application to the evaluation of miRNA target predictions [Citation76]. By integrating miRNA and mRNA data, authors were able to improve the prediction of miRNA by analyzing the expression of predicted target genes.
Bryan et al. presented a workflow of analysis of integrated miRNA–mRNA data based on bicluster analysis [Citation77]. Authors used graph theory to integrate expression data in a single graph, then demonstrated that bicluster analysis of graphs may reveal biologically relevant functional modules.
Nazarov et al. presented a novel analysis approach based on the integration of mRNA and miRNA expression levels, miRNA and TF target predictions using different tools, both academic and commercials [Citation78]. The workflow of analysis starts by considering paired miRNA and mRNA data obtained from microarray experiments available in literature. Data, after an initial preprocessing conducted on the Partek GS® platform, were analyzed through the LIMMA package of Bioconductor [Citation79]. Significant differentially expressed (SDE) mRNA and miRNA were then uploaded into the IPA® to build regulatory networks of mRNAs, miRNAs and TFs. The IPA® platform enables the reconstruction of causal networks constructed from individual relationships by providing a set of tools for inferring and scoring upstream regulators of gene expression data [Citation80].
A similar approach has been presented in a previous work by Di Martino et al. and applied to the analysis of MM data [Citation81]. With respect to the prior work, the authors first applied the integrated analysis into a clinical relevant scenario by applying results to the profiling of MM patients. The workflow of analysis was based on the use of publicly available published by Wu et al. [Citation82]. Data were initially preprocessed by using Affymetrix proprietary software and filtered using the e-freely available tool DChip. Through the use of DChip, the authors identified SDE miRNA and mRNA in two subgroups of MM patients: hyperdyploids (HD) MM versus nonhyperdyploids (nHD) MM. These data (SDE-genes and SDE-miRNAs) were then integrated into a single model by using the approach of Kramer et al. implemented into the IPA® software [Citation80]. This approach also enabled them to consider the role of TFs and to extract causal relationships among them. The authors also analyzed data into a functional space looking at canonical pathways and biofunctions, carried out by SDE-genes and miRNAs. The main result of this analysis is the identification of different biological events related to the two MM subtypes. Finally, upstream regulator analysis enabled the identification of URs related to the identified transcription events.
Starting from the first findings provided by Di Martino et al., Calura et al. presented a pipeline of analysis based on the use of MAGIA web server and other public tools to the same but more extended data set integrated with more clinical data [Citation83]. The authors used a parallel combination of two workflow of analysis, based on MAGIA2 and Micrographite computational procedures. Through the use of MAGIA2, the authors were able to reconstruct miRNA-mRNA-TF circuits into a single graph model. These data were then analyzed through topological analysis on Micrographite that is able to analyze relations among genes and miRNA by the automatic analysis of literature in stratified samples. The analysis identified the most significantly modulated pathways for each data set. The sequential use of the above-mentioned tools has been recently described in a methodological report [Citation46].
New taxonomy of MM
Several subtypes of the MM can be differentiated at the genetic and molecular level. Although morphologically similar, distinct genetic subtypes may be associated with unique clinic pathological features and different outcome, with a more favorable outcome for the HD group. At major hierarchical level, MM has been divided into HD and nHD subtypes. HD subtype, which included approximately 55–60% of MM patients, is characterized by the presence of copy number alterations, such as trisomies of odd chromosomes (3,5,7,9,11,15,19,21). The remaining cases of MM are referred as nHD and are identified for the constitutive activation of genes, such as CCND1 (11q13), CCND3 (6p21), MAF (16q23), MAFB (20q11) or FGFR3/MMSET (4p16.3), as a result of chromosomal translocations involving the immunoglobulin heavy chain locus on chromosome 14q32. This HD and nHD MM subclassification has well established at both mRNA or miRNA levels, based on clustering approaches of microarray expression profiles [Citation82,Citation84–Citation87]. Even if these two main groups of MM disclose specific genomic and transcriptomic features, an algorithm adequate for prognosis and drug response cannot be defined. Li et al. described a method for MM classification taking the view that chromosome alterations imprint the gene expression by gene-dosage effect [Citation88]. Although the authors confirm the effectiveness of the classification method, they conclude that biological and analytical causes account for misclassified samples reinforcing the rationale for an integrated and deep analysis of whole molecular profiling data to disclose transcriptional networks and achieve comprehension of the disease biology. The original integrative approach developed by Di Martino et al. and described above clearly supports the hypothesis that HD and nHD are two different diseases not only by means of early promoting events [Citation29], but evolve by different pathways that finally depict a highly divergent genetic architecture, highlighting several druggable targets predominant in HD. In this study, aberrant STAT signaling was detected with specific hyperactivity of STAT3 in HD, suggesting a role as important therapeutic target for several STAT3 inhibitors, including peptide-mimetic, small-molecule inhibitors and oligonucleotides, presently in preclinical and clinical development [Citation89,Citation90]. Moreover, the authors pointed TGFβ as therapeutic target to disrupt the pathognomonic skewed cellular interactions in MM-HBMM in the aim to antagonize bone destruction and MM-related bone disease [Citation81].
In conclusion, integrative genomics in MM was able to recognize master regulators of tumor processes and offered a reliable powerful tool to select molecular targets for therapeutic intervention indicating new therapeutic opportunities to be pursued in the next future in the era of precision medicine.
A parallel approach has been the development of the HM-metascore defined as the sum over the weighted factors gene expression-based risk-assessment (UAMS-, IFM-score), proliferation and ISS-stage, expression of prognostic target genes, delineates three significantly different MM groups with different survival [Citation91].
Pharmacogenomics in MM: a general view
There is strong evidence that genetic factors are involved in MM pathogenesis. Polymorphisms in several genes, belonging to different pathways, although with controversial results, were associated with MM risk by genome-wide association studies. The most common type of inherited genetic variation is single-nucleotide polymorphisms (SNPs), a DNA sequence variation in which a single nucleotide can be changed in a nucleotide sequence and so differs between members of a species. SNPs can be present in coding, noncoding or intergenic regions. Most SNPs have no effect on health or development, but some of them can act as biological markers: they can help researchers to identify genes associated with disease and to predict individual’s response to specific drugs, susceptibility to environmental factors such as toxins, and risk of developing specific diseases. In the HBMM and B-cell development, the highly polymorphic cytokine-encoding genes seem to exercise an important role [Citation92]. In particular, the most studied are tumor necrosis factor alpha (TNFα) (−308G/A), IL6 (−174G/C) and IL1B (+3954T/C) genes as well as a variable number tandem repeat polymorphism in the IL1RN gene involvement. Controversial results were obtained on the association between TNFα −308G/A haplotype and MM risk, while it seems to confirm its correlation to a decreased risk to develop MM [Citation93,Citation94]. Weinhold and Chubb have demonstrated that SNP at 2p23.3 (rs6746082), 3p22.1 (rs1052501), 3q26.2 (rs10936599), 6p21.33 (rs2285803), 7p15.3 (rs4487645), 17p11.2 (rs4273077) and 22q13.1 (rs877529) influence MM and MGUS risk [Citation95,Citation96].
Regarding immune-related genes, an increased risk of MM was found for several SNPs in the IL6R, IL1B, IL1A, IL1RN [Citation93], IL4R and FCGR2A genes, but these findings were not confirmed in other studies [Citation92] limiting the translatability of these findings. Polymorphisms in growth factors, cell signaling genes, DNA repair, cell cycle and apoptosis genes have been deeply investigated for their role in the genetic susceptibility to MM. It was demonstrated that the activation of the nuclear TF nuclear factor kappa-light-chain-enhancer of activated B cells (NF-κB) seems to be involved in the enhancement of cell proliferation in MM pathogenesis [Citation97], while the IκBα (rs2233406, rs3138054, rs2233419), an inhibitor of NF-κB pathway and the transcriptional activator TRAF3 (rs12147254) have been associated with a protective effect on MM development [Citation98]. As regard to polymorphisms related to insulin metabolism, the IGF1 genes (rs7965399, rs2195239, rs2373722) and the IGFBP3 (rs3110697), and two in the IRS1 gene were associated with increased or decreased MM risk. Furthermore, polymorphisms in SERPINE I, CCR7, HGF, JAK3, CD4 [Citation99], RIPK1 and HPSE immunity-related and adhesion/growth genes have been associated with MM risk even if these studies should not be validated [Citation100,Citation101]. Recently, for selected polymorphisms in HGF, CCR7, IL1B, TRAF3, MTR, TNF-α, CASP9, BAX, mEH and MTHFR, genotyped in patients enrolled in the context of the International Multiple Myeloma Research consortium, was excluded a role as major MM risk factors [Citation102]. To uncover a role in the genetic susceptibility in MM, many SNPs in genes of the DNA repair system were investigated on the basis of the hypothesis that an alteration of the class switch recombination process could be involved in the recurrence of translocation in 14q32.3 cytogenetic band in MM patients. This genetic event is considered the first step leading to the malignant transformation of the PCs in a subset of patients. Polymorphisms in XRCC4 (rs963248) and XRCC5 (rs1051685) SNPs were related to an increased risk to develop MM, supporting the hypothesis that changes in DNA repair genes influence susceptibility to MM, while in another study no association was found for SNPs in ERCC1, PPP1R13L, ERCC2 and XRCC3 [Citation93]. Moreover, SNPs in BAX, CASP3 and CASP9 genes were found to be associated with MM risk and could have an important role in its etiology as well as SNPs in XRCC3 and ERCC2 genes, while no association was found for the GSTM1, GSTT1 and GSTP1 of the glutathione-S-transferase gene and the variant codon 72 p53 allele. The potential role in genetic susceptibility to MM was investigated also for SNPs in adsorption distribution metabolism and excretion genes, but with modest evidence of association with MM risk [Citation92,Citation97]. Scientific data demonstrated an association with an increased MM risk in the GSTT1 null genotype, the homozygote genotype in the rs662 in the PON-1 genes and NAT2 rapid/slow phenotype [Citation93], while polymorphisms in genes involved in benzene metabolism were associated to an increased susceptibility to MM for carriers of ‘high-risk genotypes/phenotypes’ of GSTT1 (null), NQO1 (187PS/SS, rs1800566), mEH (high activity) and for the mEH H139R (rs2234922) genes polymorphism [Citation103]. Nevertheless, in another study no evidence could be demonstrated for association with MM risk for SNPs in NQO1 (rs1800566), PON-1 (rs622) and in rs2234922 [Citation92]. Also, folate-metabolizing enzymes were explored in a meta-analysis for involvement in MM risk and the MTHFR gene, implicated in folate homeostasis regulation, was not associated to increased MM risk [Citation104]. Also, rs1805087, the enzyme methionine synthase was associated to a higher risk for MM in a mixed Caucasian and African-American population while was associated to decreased risk in an Asian population [Citation92]. Moreover, several genetic variants were associated to treatment benefit in relapsed and refractory MM. The TNFα −238A allele was correlated with prolonged progression-free survival (PFS) and overall survival (OS) in patients treated with thalidomide and dexamethasone [Citation94]. Shaw et al. reported the evidence that a polymorphism in GSTP1 can modulate outcome of MM patients treated with a low-dose, but not high-dose melphalan (HDM) containing regimen; polymorphisms in transporters of melphalan into the cell could not be correlated with toxicity or pharmacokinetic changes [Citation105]. Patients’ outcome after dexamethasone/adriamycin/vincristine (DAV) induction therapy was correlated to SNPs in ABCB1, CYP3A4 and TP53BP2, which were associated with response; SNPs in ALDH2, GSTT2 and BRCA1 were associated with response to HDM, whereas polymorphisms in CYP1A1, RAD51 and PARP were associated with disease progression following the same regimen; polymorphisms in ALDH2 and CYP1A1 were, instead, correlated with prolonged OS [Citation106]. Moreover, the rs1695 in GSTP1 and rs2790 in thymidylate synthase (TYMS) were significantly associated with a poor response to DAV regimen followed by a conditioning regimen and autologous stem cells transplantation (ASCT); on the other hand, a worse response to ASCT was related to the rs699517 in TYMS [Citation107]. In MM patients, folate transporters SLC19A1 gene (rs1051296) was found correlated to an improved outcome after HDM and ASCT [Citation92]. Finally, among the drug transporters, the gene coding the ABCB1 has been widely studied. In particular, the rs1045642 and rs2032582 in ABCB1 gene were associated with outcome after different treatments in MM, and the T allele of the ABCB1 C3435T has been associated with a better response to DAV treatment [Citation106], bortezomib treatment of relapsed and/or refractory myeloma patients together with a better PFS (T/T homozygotes) and OS of MM patients [Citation108]. The rare T allele of the tri-allelic ABCB1 SNP G2677T/A was associated to a better response to DAV and a prolonged OS in MM patients [Citation106]. Instead, controversial results were found for SNPs in genes belonging to the cytochrome P450 family (i.e. CYP2C19, CYP2D6, CYP3A4) [Citation92]. The poor metabolizer phenotype of CYP2C19 gene was associated with a poor response to thalidomide and an individual’s risk of developing a peripheral neuropathy appears to be mediated by polymorphisms in genes governing repair mechanisms and inflammation in the peripheral nervous system [Citation109]. All these findings demonstrate that PG advances have led to the discovery of factors influencing the individual risk to MM, the response to chemotherapy and survival or to adverse reaction to drugs. In the era of precision medicine, biomarker discovery and improvement of PGs knowledge by new genomic technologies might shade new light in the genetic complexity of MM and lead to a better identification of groups of patients that may differ in their clinical outcome and offer therefore a highly valuable tool for personalized treatment selection.
Profiling and bioinformatics tools
Here, we recall some of the main bioinformatics tools for the analysis of PGs data.
DMET-Analyzer is a freely available tool for the association analysis among the variation of the patient genomes and the clinical conditions of patients [Citation110]. It is able to verify the association of the allelic variant of each probe to the clinical condition under investigation through the use of the well-known Fisher’s test improved by Bonferroni and false discovery rate corrections. PGxClean [Citation111] is a web application that performs quality control analyses for data produced by the Affymetrix DMET array or other candidate gene technologies [Citation112,Citation113]. It performs principal component analysis and provides tools for characterizing and visualizing population structure. The most complete bioinformatic tool for PGs data analysis is certainly the pharmacogenomics knowledge base (PharmGKB) contains genomic, phenotype and clinical information collected from ongoing PG studies [Citation114]. PharmGKB provides, through a web-based interface, a suite of tools to easily manage such information. Examples of functions provided are browsing, querying and downloading of such information. The current release of PharmGKB regards over 150 genes under study, 14 Coriell populations and a large ontology of PGs concepts. A set of relations and crosslinks are used to relate PG concepts to the experimental data in order to form knowledge base information for PG researchers. Regarding pathways, the PharmGKB creates and stores manually annotated pathways that are centered around a particular drug that has PG associations. These can be further subdivided into pharmacokinetic or pharmacodynamic pathways. Regarding clinical interpretation of data stored in PharmGKB, it is realized through knowledge annotation, aggregation and integration in a manual way. The curators of the knowledge base review the variant annotations for a particular genetic variant–drug association pair and aggregate these to write a summary of the association between the drug and each genotype of the genetic variant. The resulting aggregated data are stored into clinical annotation sections. Each clinical annotation is given a level of evidence depending on specific criteria, including study size and statistical relevance of the association. The deep description of PharmGKB is beyond the scope of this review, where we focus on the opportunity to extract relevant information for clinical application.
Expert commentary
Recent advances have increased the understanding of molecular pathology of MM generating the framework for novel therapeutic approaches. A novel trial methodology is, however, eagerly awaited in order to validate the targeted approaches based on molecular rationales. The multilayered knowledge produced by the integrative genomics is the most attractive tool to identify the crucial nodes that might represent the optimal targets for innovative approaches. The complex disease scenario, with the basic role of the cellular interplay within the HBMM, makes, in our opinion, whole-genome or -exome sequencing alone not adequate to capture the molecular features of relevance for treatment tailoring in this specific disease. Although the genetic polymorphism rely on a robust methodology, the profiling technologies as well as next-generation sequencing or RNA-sequencing technologies need often important improvement efforts in order to be translated from pure investigational approaches in robust tools for clinical investigation and for generating biomarkers. Nevertheless, the rising evidence that MM is indeed a composite disease that includes different and parallel clinical entities produces the basic information to translate into a new investigational and therapeutics development approach. A parallel individualization strategy relies on genetic polymorphisms predictive of drug activity and toxicity.
Five-year view
Novel drugs and novel immunotherapy approaches are enriching the therapeutic scenario of MM. We predict that in the next five years a novel vision of clinical research in MM will emerge and it needs to be based on a personalized medicine view. The clinical trials will be designed taking advantage from the robust knowledge of MM biopathology in terms of patient selection but also in terms of sequence and continuous care principles. A wise integrated approach has the potential to gain major advantages in terms of patient survival and quality of life.
Financial & competing interests disclosure
This paper was supported with funding from the AIRC (Italian Association for Cancer Research, PI: PT. “Special Program Molecular Clinical Oncology- 5 per mille” n.9980, 2010/15) a non-profit organization. The authors have no other relevant affiliations or financial involvement with any organization or entity with a financial interest in or financial conflict with the subject matter or materials discussed in the manuscript apart from those disclosed.
Key issues
Multiple myeloma relies on a complex interplay between malignant plasma cells and the bone marrow microenvironment.
Multiple myeloma is not a unique disease but includes different parallel diseases.
Integrative genomics represent an important tool for a multilayered vision of the disease and for identifying crucial nodes for biomarker selection and new drug development.
Genetic polymorphism identified by single-nucleotide polymorphisms genomic profiling represent an additional tool for treatment individualization.
RNA therapeutics is evolving as a new generation targeted therapy of multiple myeloma.
Novel drugs coupled to novel biological information need to be translated into novel trial design in multiple myeloma.
References
- Jameson JL, Longo DL. Precision medicine–personalized, problematic, and promising. N Engl J Med. 2015;372:2229–2234.
- Rosland GV, Engelsen AS. Novel points of attack for targeted cancer therapy. Basic Clin Pharmacol Toxicol. 2015;116:9–18.
- Kalia M. Biomarkers for personalized oncology: recent advances and future challenges. Metabolism. 2015;64:S16–S21.
- Biankin AV, Piantadosi S, Hollingsworth SJ. Patient-centric trials for therapeutic development in precision oncology. Nature. 2015;526:361–370.
- Chapman PB, Hauschild A, Robert C, et al Improved survival with vemurafenib in melanoma with BRAF V600E mutation. N Engl J Med. 2011;364:2507–2516.
- Arkenau HT, Kefford R, Long GV. Targeting BRAF for patients with melanoma. Br J Cancer. 2011;104:392–398.
- Rossi M, Di Martino MT, Morelli E, et al Molecular targets for the treatment of multiple myeloma. Curr Cancer Drug Targets. 2012;12:757–767.
- Rossi M, Botta C, Correale P, et al Immunologic microenvironment and personalized treatment in multiple myeloma. Expert Opin Biol Ther. 2013;13(Suppl 1):S83–S93.
- Cottini F, Anderson K. Novel therapeutic targets in multiple myeloma. Clin Adv Hematol Oncol. 2015;13:236–248.
- Mimura N, Hideshima T, Anderson KC. Novel therapeutic strategies for multiple myeloma. Exp Hematol. 2015;43:732–741.
- Rossi M, Amodio N, Di Martino MT, et al From target therapy to miRNA therapeutics of human multiple myeloma: theoretical and technological issues in the evolving scenario. Curr Drug Targets. 2013;14:1144–1149.
- Misso G, Zappavigna S, Castellano M, et al Emerging pathways as individualized therapeutic target of multiple myeloma. Expert Opin Biol Ther. 2013;13(Suppl 1):S95–S109.
- Calimeri T, Battista E, Conforti F, et al A unique three-dimensional SCID-polymeric scaffold (SCID-synth-hu) model for in vivo expansion of human primary multiple myeloma cells. Leukemia. 2011;25:707–711.
- Tassone P, Neri P, Burger R, et al Mouse models as a translational platform for the development of new therapeutic agents in multiple myeloma. Curr Cancer Drug Targets. 2012;12:814–822.
- Blotta S, Jakubikova J, Calimeri T, et al Canonical and noncanonical Hedgehog pathway in the pathogenesis of multiple myeloma. Blood. 2012;120:5002–5013.
- Hamasaki M, Hideshima T, Tassone P, et al Azaspirane (N-N-diethyl-8,8-dipropyl-2-azaspiro [4.5] decane-2-propanamine) inhibits human multiple myeloma cell growth in the bone marrow milieu in vitro and in vivo. Blood. 2005;105:4470–4476.
- Rosean TR, Tompkins VS, Tricot G, et al Preclinical validation of interleukin 6 as a therapeutic target in multiple myeloma. Immunol Res. 2014;59:188–202.
- Walker RE, Lawson MA, Buckle CH, et al Myeloma bone disease: pathogenesis, current treatments and future targets. Br Med Bull. 2014;111:117–138.
- Yasui H, Hideshima T, Hamasaki M, et al SDX-101, the R-enantiomer of etodolac, induces cytotoxicity, overcomes drug resistance, and enhances the activity of dexamethasone in multiple myeloma. Blood. 2005;106:706–712.
- Tassone P, Galea E, Forciniti S, et al The IL-6 receptor super-antagonist Sant7 enhances antiproliferative and apoptotic effects induced by dexamethasone and zoledronic acid on multiple myeloma cells. Int J Oncol. 2002;21:867–873.
- Yap TA, Olmos D, Molife LR, et al Targeting the insulin-like growth factor signaling pathway: figitumumab and other novel anticancer strategies. Expert Opin Investig Drugs. 2011;20:1293–1304.
- Hengeveld PJ, Kersten MJ. B-cell activating factor in the pathophysiology of multiple myeloma: a target for therapy? Blood Cancer J. 2015;5:e282.
- Mikhael JR, Dingli D, Roy V, et al Management of newly diagnosed symptomatic multiple myeloma: updated Mayo Stratification of Myeloma and Risk-Adapted Therapy (mSMART) consensus guidelines 2013. Mayo Clin Proc. 2013;88:360–376.
- Van Laar R, Flinchum R, Brown N, et al Translating a gene expression signature for multiple myeloma prognosis into a robust high-throughput assay for clinical use. BMC Med Genomics. 2014;7:25.
- Zhan F, Huang Y, Colla S, et al The molecular classification of multiple myeloma. Blood. 2006;108:2020–2028.
- Shaughnessy JD Jr, Zhan F, Burington BE, et al A validated gene expression model of high-risk multiple myeloma is defined by deregulated expression of genes mapping to chromosome 1. Blood. 2007;109:2276–2284.
- Chen T, Berno T, Zangari M. Low-risk identification in multiple myeloma using a new 14-gene model. Eur J Haematol. 2012;89:28–36.
- Palumbo A, Avet-Loiseau H, Oliva S, et al Revised international staging system for multiple myeloma: a report from International Myeloma Working Group. J Clin Oncol. 2015;33:2863–2869.
- Morgan GJ, Walker BA, Davies FE. The genetic architecture of multiple myeloma. Nat Rev Cancer. 2012;12:335–348.
- Kryukova E, Kryukov F, Hajek R. Centrosome amplification and clonal evolution in multiple myeloma: short review. Crit Rev Oncol Hematol. 2015;98:116–121. DOI:10.1016/j.critrevonc.2015.10.019
- Johnson SK, Heuck CJ, Albino AP, et al The use of molecular-based risk stratification and pharmacogenomics for outcome prediction and personalized therapeutic management of multiple myeloma. Int J Hematol. 2011;94:321–333.
- Kronke J, Udeshi ND, Narla A, et al Lenalidomide causes selective degradation of IKZF1 and IKZF3 in multiple myeloma cells. Science. 2014;343:301–305.
- Lu G, Middleton RE, Sun H, et al The myeloma drug lenalidomide promotes the cereblon-dependent destruction of Ikaros proteins. Science. 2014;343:305–309.
- Moreaux J, Reme T, Leonard W, et al Development of gene expression-based score to predict sensitivity of multiple myeloma cells to DNA methylation inhibitors. Mol Cancer Ther. 2012;11:2685–2692.
- Delmore JE, Issa GC, Lemieux ME, et al BET bromodomain inhibition as a therapeutic strategy to target c-Myc. Cell. 2011;146:904–917.
- Lohr JG, Stojanov P, Carter SL, et al Widespread genetic heterogeneity in multiple myeloma: implications for targeted therapy. Cancer Cell. 2014;25:91–101.
- Cottini F, Hideshima T, Suzuki R, et al Synthetic lethal approaches exploiting DNA damage in aggressive myeloma. Cancer Discov. 2015;5:972–987.
- Andrulis M, Lehners N, Capper D, et al Targeting the BRAF V600E mutation in multiple myeloma. Cancer Discov. 2013;3:862–869.
- Zhang Q, Yan W, Bai Y, et al Synthetic miR-145 mimic inhibits multiple myeloma cell growth in vitro and in vivo. Oncol Rep. 2015;33:448–456.
- Agnelli L, Tassone P, Neri A. Molecular profiling of multiple myeloma: from gene expression analysis to next-generation sequencing. Expert Opin Biol Ther. 2013;13(Suppl 1):S55–S68.
- Lionetti M, Agnelli L, Lombardi L, et al MicroRNAs in the pathobiology of multiple myeloma. Curr Cancer Drug Targets. 2012;12:823–837.
- Lionetti M, Musto P, Di Martino MT, et al Biological and clinical relevance of miRNA expression signatures in primary plasma cell leukemia. Clin Cancer Res. 2013;19:3130–3142.
- Seckinger A, Meissner T, Moreaux J, et al miRNAs in multiple myeloma - a survival relevant complex regulator of gene expression. Oncotarget. 2015;6:39165–39183.
- Tagliaferri P, Rossi M, Di Martino MT, et al Promises and challenges of microRNA-based treatment of multiple myeloma. Curr Cancer Drug Targets. 2012;12:838–846.
- Rossi M, Amodio N, Di Martino MT, et al MicroRNA and multiple myeloma: from laboratory findings to translational therapeutic approaches. Curr Pharm Biotechnol. 2014;15:459–467.
- Di Martino MT, Amodio N, Tassone P, et al. Functional analysis of microRNA in multiple myeloma. Methods Mol Biol. 2015:S125–S137. DOI:10.1517/14712598.2013.796356
- Amodio N, Di Martino MT, Neri A, et al Non-coding RNA: a novel opportunity for the personalized treatment of multiple myeloma. Expert Opin Biol Ther. 2013;13(Suppl 1):S125–S137.
- Gulla A, Di Martino MT, Gallo Cantafio ME, et al. A 13 mer LNA-i-miR-221 inhibitor restores drug-sensitivity in melphalan-refractory multiple myeloma cells. Clin Cancer Res. 2013;19(8):2096–2106. DOI:10.1158/1078-0432.CCR-12-3325
- Leone E, Morelli E, Di Martino MT, et al Targeting miR-21 inhibits in vitro and in vivo multiple myeloma cell growth. Clin Cancer Res. 2013;19:2096–2106.
- Di Martino MT, Gulla A, Gallo Cantafio ME, et al In vitro and in vivo activity of a novel locked nucleic acid (LNA)-inhibitor-miR-221 against multiple myeloma cells. PLoS One. 2014;9:e89659.
- Di Martino MT, Gulla A, Cantafio ME, et al In vitro and in vivo anti-tumor activity of miR-221/222 inhibitors in multiple myeloma. Oncotarget. 2013;4:242–255.
- Zhang Y, Roccaro AM, Rombaoa C, et al LNA-mediated anti-miR-155 silencing in low-grade B-cell lymphomas. Blood. 2012;120:1678–1686.
- Zhao JJ, Lin J, Zhu D, et al miR-30-5p functions as a tumor suppressor and novel therapeutic tool by targeting the oncogenic Wnt/beta-catenin/BCL9 pathway. Cancer Res. 2014;74:1801–1813.
- Du J, Liu S, He J, et al MicroRNA-451 regulates stemness of side population cells via PI3K/Akt/mTOR signaling pathway in multiple myeloma. Oncotarget. 2015;6:14993–15007.
- Feng M, Luo X, Gu C, et al Seed targeting with tiny anti-miR-155 inhibits malignant progression of multiple myeloma cells. J Drug Target. 2015;23:59–66.
- Leotta M, Biamonte L, Raimondi L, et al A p53-dependent tumor suppressor network is induced by selective miR-125a-5p inhibition in multiple myeloma cells. J Cell Physiol. 2014;229:2106–2116.
- Amodio N, Bellizzi D, Leotta M, et al miR-29b induces SOCS-1 expression by promoter demethylation and negatively regulates migration of multiple myeloma and endothelial cells. Cell Cycle. 2013;12:3650–3662.
- Raimondi L, Amodio N, Di Martino MT, et al Targeting of multiple myeloma-related angiogenesis by miR-199a-5p mimics: in vitro and in vivo anti-tumor activity. Oncotarget. 2014;5:3039–3054.
- Di Martino MT, Campani V, Misso G, et al In vivo activity of miR-34a mimics delivered by stable nucleic acid lipid particles (SNALPs) against multiple myeloma. PLoS One. 2014;9:e90005.
- Roccaro AM, Sacco A, Thompson B, et al MicroRNAs 15a and 16 regulate tumor proliferation in multiple myeloma. Blood. 2009;113:6669–6680.
- Shen X, Guo Y, Yu J, et al. miRNA-202 in bone marrow stromal cells affects the growth and adhesion of multiple myeloma cells by regulating B cell-activating factor. Clin Exp Med. 2015:1–10. DOI:10.1007/s10238-015-0355-4
- Yang Y, Li F, Saha MN, et al miR-137 and miR-197 induce apoptosis and suppress tumorigenicity by targeting MCL-1 in multiple myeloma. Clin Cancer Res. 2015;21:2399–2411.
- Palagani A, Op De Beeck K, Naulaerts S, et al. Ectopic microRNA-150-5p transcription sensitizes glucocorticoid therapy response in MM1S multiple myeloma cells but fails to overcome hormone therapy resistance in MM1R cells. PLoS One. 2014;9:e113842.
- Jagannathan S, Vad N, Vallabhapurapu S, et al MiR-29b replacement inhibits proteasomes and disrupts aggresome+autophagosome formation to enhance the antimyeloma benefit of bortezomib. Leukemia. 2015;29:727–738.
- Morelli E, Leone E, Cantafio ME, et al Selective targeting of IRF4 by synthetic microRNA-125b-5p mimics induces anti-multiple myeloma activity in vitro and in vivo. Leukemia. 2015;29:2173–2183.
- Misiewicz-Krzeminska I, Sarasquete ME, Quwaider D, et al Restoration of microRNA-214 expression reduces growth of myeloma cells through positive regulation of P53 and inhibition of DNA replication. Haematologica. 2013;98:640–648.
- Misso G, Di Martino MT, De Rosa G, et al Mir-34: a new weapon against cancer? Mol Ther Nucleic Acids. 2014;3:e194.
- Di Martino MT, Leone E, Amodio N, et al Synthetic miR-34a mimics as a novel therapeutic agent for multiple myeloma: in vitro and in vivo evidence. Clin Cancer Res. 2012;18:6260–6270.
- Lennox KA, Behlke MA. Chemical modification and design of anti-miRNA oligonucleotides. Gene Ther. 2011;18:1111–1120.
- Kristensen VN, Lingjaerde OC, Russnes HG, et al Principles and methods of integrative genomic analyses in cancer. Nat Rev Cancer. 2014;14:299–313.
- Guzzi PH, Agapito G, Milano M, et al. Methodologies and experimental platforms for generating and analysing microarray and mass spectrometry-based omics data to support P4 medicine. Brief Bioinform. 2015. DOI:10.1093/bib/bbv076
- Roy S, Guzzi PH. Biological network inference from microarray data, current solutions, and assessments. Methods Mol Biol. 2015:1–13. DOI:10.1007/7651_2015_284
- Yan Z, Shah PK, Amin SB, et al Integrative analysis of gene and miRNA expression profiles with transcription factor-miRNA feed-forward loops identifies regulators in human cancers. Nucleic Acids Res. 2012;40:e135.
- Huang GT, Athanassiou C, Benos PV. mirConnX: condition-specific mRNA-microRNA network integrator. Nucleic Acids Res. 2011;39:W416–W423.
- Afshar AS, Xu J, Goutsias J. Integrative identification of deregulated miRNA/TF-mediated gene regulatory loops and networks in prostate cancer. PLoS One. 2014;9:e100806.
- Van Iterson M, Bervoets S, De Meijer EJ, et al Integrated analysis of microRNA and mRNA expression: adding biological significance to microRNA target predictions. Nucleic Acids Res. 2013;41:e146.
- Bryan K, Terrile M, Bray IM, et al Discovery and visualization of miRNA-mRNA functional modules within integrated data using bicluster analysis. Nucleic Acids Res. 2014;42:e17.
- Nazarov PV, Reinsbach SE, Muller A, et al Interplay of microRNAs, transcription factors and target genes: linking dynamic expression changes to function. Nucleic Acids Res. 2013;41:2817–2831.
- Wettenhall JM, Smyth GK. limmaGUI: a graphical user interface for linear modeling of microarray data. Bioinformatics. 2004;20:3705–3706.
- Kramer A, Green J, Pollard J Jr, et al Causal analysis approaches in ingenuity pathway analysis. Bioinformatics. 2014;30:523–530.
- Di Martino MT, Guzzi PH, Caracciolo D, et al Integrated analysis of microRNAs, transcription factors and target genes expression discloses a specific molecular architecture of hyperdiploid multiple myeloma. Oncotarget. 2015;6:19132–19147.
- Wu P, Agnelli L, Walker BA, et al Improved risk stratification in myeloma using a microRNA-based classifier. Br J Haematol. 2013;162:348–359.
- Calura E, Bisognin A, Manzoni M, et al. Disentangling the microRNA regulatory milieu in multiple myeloma: integrative genomics analysis outlines mixed miRNA-TF circuits and pathway-derived networks modulated in t(4;14) patients. Oncotarget. 2015;7(3):2367–2378.
- Amin SB, Yip WK, Minvielle S, et al. Gene expression profile alone is inadequate in predicting complete response in multiple myeloma. Leukemia. 2014;28(11):2229–2234. DOI:10.1038/leu.2014.140
- Lionetti M, Biasiolo M, Agnelli L, et al Identification of microRNA expression patterns and definition of a microRNA/mRNA regulatory network in distinct molecular groups of multiple myeloma. Blood. 2009;114:e20–e26.
- Fonseca R, Bergsagel PL, Drach J, et al International Myeloma Working Group molecular classification of multiple myeloma: spotlight review. Leukemia. 2009;23:2210–2221.
- Agnelli L, Fabris S, Bicciato S, et al Upregulation of translational machinery and distinct genetic subgroups characterise hyperdiploidy in multiple myeloma. Br J Haematol. 2007;136:565–573.
- Li Y, Wang X, Zheng H, et al Classify hyperdiploidy status of multiple myeloma patients using gene expression profiles. PLoS One. 2013;8:e58809.
- Furqan M, Akinleye A, Mukhi N, et al STAT inhibitors for cancer therapy. J Hematol Oncol. 2013;6:90.
- Nelson EA, Sharma SV, Settleman J, et al A chemical biology approach to developing STAT inhibitors: molecular strategies for accelerating clinical translation. Oncotarget. 2011;2:518–524.
- Meissner T, Seckinger A, Reme T, et al Gene expression profiling in multiple myeloma–reporting of entities, risk, and targets in clinical routine. Clin Cancer Res. 2011;17:7240–7247.
- Martino A, Sainz J, Buda G, et al Genetics and molecular epidemiology of multiple myeloma: the rationale for the IMMEnSE consortium (review). Int J Oncol. 2012;40:625–638.
- Vangsted A, Klausen TW, Vogel U. Genetic variations in multiple myeloma I: effect on risk of multiple myeloma. Eur J Haematol. 2012;88:8–30.
- Du J, Yuan Z, Zhang C, et al Role of the TNF-alpha promoter polymorphisms for development of multiple myeloma and clinical outcome in thalidomide plus dexamethasone. Leuk Res. 2010;34:1453–1458.
- Weinhold N, Johnson DC, Rawstron AC, et al Inherited genetic susceptibility to monoclonal gammopathy of unknown significance. Blood. 2014;123:2513–2517; quiz 2593.
- Chubb D, Weinhold N, Broderick P, et al Common variation at 3q26.2, 6p21.33, 17p11.2 and 22q13.1 influences multiple myeloma risk. Nat Genet. 2013;45:1221–1225.
- Salazar L, Kashiwada T, Krejci P, et al Fibroblast growth factor receptor 3 interacts with and activates TGFbeta-activated kinase 1 tyrosine phosphorylation and NFkappaB signaling in multiple myeloma and bladder cancer. PLoS One. 2014;9:e86470.
- Du J, Huo J, Shi J, et al Polymorphisms of nuclear factor-kappaB family genes are associated with development of multiple myeloma and treatment outcome in patients receiving bortezomib-based regimens. Haematologica. 2011;96:729–737.
- Lee KM, Baris D, Zhang Y, et al Common single nucleotide polymorphisms in immunoregulatory genes and multiple myeloma risk among women in Connecticut. Am J Hematol. 2010;85:560–563.
- Purdue MP, Lan Q, Menashe I, et al Variation in innate immunity genes and risk of multiple myeloma. Hematol Oncol. 2011;29:42–46.
- Greenberg AJ, Rajkumar SV, Vachon CM. Familial monoclonal gammopathy of undetermined significance and multiple myeloma: epidemiology, risk factors, and biological characteristics. Blood. 2012;119:5359–5366.
- Martino A, Campa D, Jurczyszyn A, et al Genetic variants and multiple myeloma risk: IMMEnSE validation of the best reported associations–an extensive replication of the associations from the candidate gene era. Cancer Epidemiol Biomarkers Prev. 2014;23:670–674.
- Lajin B, Alachkar A. The NQO1 polymorphism C609T (Pro187Ser) and cancer susceptibility: a comprehensive meta-analysis. Br J Cancer. 2013;109:1325–1337.
- Ma L-M, Ruan L-H, Yang H-P. Meta-analysis of the association of MTHFR polymorphisms with multiple myeloma risk. Sci Rep. 2015;5:10735.
- Shaw PJ, Nath CE, Lazarus HM. Not too little, not too much-just right! (Better ways to give high dose melphalan). Bone Marrow Transplant. 2014;49:1457–1465.
- Dumontet C, Landi S, Reiman T, et al Genetic polymorphisms associated with outcome in multiple myeloma patients receiving high-dose melphalan. Bone Marrow Transplant. 2010;45:1316–1324.
- Simeon V, Todoerti K, La Rocca F, et al Molecular classification and pharmacogenetics of primary plasma cell leukemia: an initial approach toward precision medicine. Int J Mol Sci. 2015;16:17514–17534.
- Buda G, Ricci D, Huang CC, et al Polymorphisms in the multiple drug resistance protein 1 and in P-glycoprotein 1 are associated with time to event outcomes in patients with advanced multiple myeloma treated with bortezomib and pegylated liposomal doxorubicin. Ann Hematol. 2010;89:1133–1140.
- Johnson DC, Corthals SL, Walker BA, et al Genetic factors underlying the risk of thalidomide-related neuropathy in patients with multiple myeloma. J Clin Oncol. 2011;29:797–804.
- Guzzi PH, Agapito G, Di Martino MT, et al DMET-analyzer: automatic analysis of Affymetrix DMET data. BMC Bioinformatics. 2012;13:258.
- Rotroff D, Jack J, Campbel N, et al. PGxClean: a quality control GUI for the Affymetrix DMET chip and other candidate gene studies with non-biallelic alleles. BioData Mining. 2014;7:24.
- Di Martino MT, Arbitrio M, Leone E, et al Single nucleotide polymorphisms of ABCC5 and ABCG1 transporter genes correlate to irinotecan-associated gastrointestinal toxicity in colorectal cancer patients: a DMET microarray profiling study. Cancer Biol Ther. 2011;12:780–787.
- Di Martino MT, Arbitrio M, Guzzi PH, et al A peroxisome proliferator-activated receptor gamma (PPARG) polymorphism is associated with zoledronic acid-related osteonecrosis of the jaw in multiple myeloma patients: analysis by DMET microarray profiling. Br J Haematol. 2011;154:529–533.
- Klein TE, Altman RB. PharmGKB: the pharmacogenetics and pharmacogenomics knowledge base. Pharmacogenomics J. 2004;4:1.