Abstract
Women and gender minorities are underrepresented in positions of leadership and seniority in academia. Research on gender in higher education (HE) has varied in scale and methodological approach from large-scale global surveys to small-scale projects with interviews and focus groups, with a noticeable gap in the attention given to early career researchers and doctoral students, and the ways in which their experiences can vary significantly from discipline to discipline, institution to institution, and “department-like unit” to “department-like unit.” Drawing on gender theory to link gender to power and gendered organizations, we connect this theoretical perspective to research on gender in HE. This article proposes a new agenda to develop our understanding of gender in HE by drawing together quantitative and feminist geography to focus on the issue at different “palatial” scales within HE. We propose expanding the definition of gender often used in quantitative research and to consider intersectionality, using open source data to provide novel and reproducible insights into the dynamics of gender in HE, and using quantitative geography methods to develop a multiscalar understanding of these dynamics.
在学术界, 妇女和性别群体是领导地位和资历的少数群体。高等教育中的性别研究采用了不同的尺度和方法, 包括全球调查、小型访谈和焦点小组。然而, 这些研究很少探讨职业初期研究人员和博士生, 及其在不同学科、不同机构、不同部门的不同经历。为了融合性别、权力和性别化组织, 本文从性别理论出发, 将理论与高等教育性别研究相互联系起来。本文提议, 为了理解高等教育中的性别问题, 应当结合定量地理和女权主义地理、关注高等教育中的不同“位置”尺度。应当将定量研究经常使用的性别定义拓展到交叉性, 基于开源数据为高等教育的性别变化提供新的、可复制的理解, 并通过定量地理方法去实现对这些变化的多尺度理解。
Las mujeres y las minorías se hallan subrepresentadas en los puestos directivos y de mayor responsabilidad de la academia. La investigación relacionada con género en la educación superior (HE) ha variado en escala y enfoque metodológico, desde los estudios globales a gran escala hasta proyectos limitados de pequeña escala, con entrevistas y grupos de interés, donde se percibe un vacío notable en términos de la atención que se presta a los investigadores principiantes y estudiantes doctorales, y las formas como sus experiencias pueden variar de manera significativa de una disciplina a otra, de institución a institución y de “una unidad similar a un departamento” a otra. Basándonos en la teoría de género para vincular género con organizaciones de poder y género, conectamos esta perspectiva teórica con la investigación sobre género en la educación superior. En este artículo se propone una nueva agenda para avanzar en nuestro entendimiento del género en la educación superior, al combinar las geografías cuantitativa y feminista para que se centren en la cuestión de las diferentes escalas “palaciegas” dentro de la educación superior. Proponemos expandir la definición de género a menudo usada en la investigación cuantitativa y considerar la interseccionalidad, usando datos de código abierto para proveer visiones novedosas y reproducibles dentro de las dinámicas del género en la educación superior, y usando métodos de geografía cuantitativa para desarrollar una comprensión mulltiescalar de estas dinámicas.
It is unsurprising that women and other minoritized genders and groups are underrepresented in positions of power, leadership, and seniority in higher education (HE). We recognize and acknowledge the existence of multiple axes of inequality within academia, including gender, ethnicity, social class, sexuality, religion, citizenship, and age. Although we wish to focus on gender in this article, we do not wish to imply that these other axes and experiences do not exist or do not matter. They do. We believe that the agenda elaborated here for a renewed engagement with a critical, feminist, and quantitative geography of HE has relevance to these other inequalities.
Using UK academia as a lens through which to examine the issues of gender within HE, female academics around the word cite barriers including hiring biases, gender stereotypes, and sexual harassment (American Council on Education and Center for International Higher Education [ACE and CIHE] 2021). For women and minorities working in scientific fields in the United States, the largest global provider of HE, there are inequalities in multiple aspects of the academic career including publishing, peer review, grant submissions, and research impact (Kozlowski et al. Citation2022). When looking at U.S. PhD theses, Hofstra et al. (Citation2020) showed how “women and non-white scholars introduce more novelty but have less impactful novelty when compared to men and white students” (9286). Over in New Zealand, Walker et al. (Citation2020) found that female researchers are more likely to be employed on part-time contracts and that their progress was slower than that of their male colleagues, with the result that “women dominate at the lower levels of the academic scale, while men dominate more senior levels” (7). In India, despite 39 percent of PhDs being awarded to women (in 2015–2016), only 14 percent of the full-time academic staff working in research are women (Paswan and Singh 2020). In Kazakhstan and Kyrgyzstan, conflicting and contradictory viewpoints on labored gender roles have emerged with Soviet views, conservative views (from the revival of Islam), and neo-liberal views all contributing to women’s expectations to fulfill the multiple roles of worker, leader, mother, and partner (Kuzhabekova and Almukhambetova Citation2021).
A lack of diversity, in the United Kingdom and globally, is not just a moral issue, however; it is also a waste of talent. A diverse workforce, with diverse teams composed of people with different interests and pools of knowledge, promotes innovation and responds to tackle challenging research problems in novel ways (Hofstra et al. Citation2020). Diversity therefore not only helps to nourish a fairer and more equal society, but can increase economic productivity as well: The UK Commission for Employment and Skills estimated in 2016 that “the under-utilisation of women’s skills costs the UK economy between 1.3 and 2 percent of GDP every year” and that by eliminating the gender pay gap, it could add £41 billion to the UK economy annually (CitationHouse of Commons Women and Equalities Committee 2016).
Data on staff and students in UK HE collected for 2019–2020 by the Higher Education Statistics Agency (HESA) indicate that 46.6 percent of academic staff are female, and 0.16 percent identified as other (HESA Citation2021a). Of the 22,745 full professors, though, just 6,340 (27.9 percent) identified as female, which is, at best, a modest improvement from 2012 when HESA reported that “only 20.5 percent were women” (Savigny Citation2014, 795; HESA Citation2021a). Across European Union countries, a mere 24 percent of professors in 2020 were female (O’Connor and Irvine Citation2020) and, looking at other international contexts, women made up 36 percent of professors in the United States (American Association of University Women [AAUW] 2022), 36 percent of associate and full professors in Australia, 20 to 25 percent of researchers in Ghana, and only 17.5 percent of professors in Hong Kong (ACE and CIHE Citation2021).
Applying an intersectional approach (Crenshaw Citation1991) and considering ethnicity and gender together raises even more profound issues: Advance HE (Citation2021a) found that, in 2019–2020, just 8.2 percent of UK professors indicated a Black, Asian, and minority ethnic (BAME) background and identified as male, and that a mere 3.6 percent were BAME and female, up from 2.1 percent in the previous year (HESA Citation2021a). We should note, however, that such a broad classification obscures significant disparities within this category: Data from 2020 found that fewer than 1 percent of professors identify as Black—that is 140 individuals in all (Adams Citation2020)—with just 25 Black women across the United Kingdom having roles in “the professoriates” (Rollock Citation2019). In the United States, among higher education institutions (HEIs) awarding undergraduate degrees, “only 5.2 percent of tenured faculty are Black, and 6.6 percent are Latinx” (AAUW Citation2022), and in 2021, only 5 percent of college presidents were “women of colour,” whereas 30 percent of college presidents were women (ACE and CIHE Citation2021). These inequalities persist across other personal characteristics like disability status, with “a lower proportion of professors disclosing that they identify as disabled (3.2 percent) than non-professorial academic staff (4.8 percent)” (Advance HE Citation2021a, 85). These statistics alone suggest the extent and seriousness of the diversity crisis among staff in academia.
Until recently, personal characteristics like gender and race or ethnic group were mainly treated separately in HE, as seen through the separate Athena SWAN award for gender equality and the Race Equality Charter for race equality in HE. At the institutional level, in the United Kingdom, although most HEIs publish equality, diversity, and inclusion (EDI) reports that include data on their staff and student populations (e.g., the universities of Nottingham, Kent, King’s College London, Lancaster, Newcastle, and Dundee), only a few report on how those personal characteristics intersect. A rare exception is King’s College London (Citation2021), which provides a detailed look at actual and target figures for intersectional groupings such as the number of BAME female staff. In general, though, much more work could be done to include data on intersectionality at individual institutions to support and extend the work being done by HESA in its annual reports (see Advance HE Citation2021a).
Complementing the “big picture” available from data collected by statistical agencies and global studies on academic publications (Larivière et al. Citation2013; Holman, Stuart-Fox, and Hauser Citation2018) are an array of smaller studies—typically with a handful of participants—centered on experiences within singular universities or departments (Wall Citation2008; Cidlinská Citation2019; Hillier Citation2021). Missing from this framing, however, is a strong conceptualization of place: Critiquing academia as a whole erodes disciplinary, institutional, and departmental differences that shape individual experiences of oppression and opportunity. Westerholt, Mocnik, and Comber (Citation2020) described places as “often intimate and subjective, making them open to value assignment” (811), and it is important to understand how place can affect people’s everyday lives. In this work, we consider place to be a specific location, and particular area, such as a university campus or department, institute, or center within the university structure. It is also important, however, to understand place through the wider embedding of the institution in, for example, a rural, semiurban, or urban setting. For example, HEIs in larger and more diverse cities such as Manchester, Liverpool, and London, will have a different platial context to those in smaller towns and rural settings such as Cranfield University, Royal Agricultural University (Cirencester), and St. Andrew’s.
We seek here to argue for the utility of advances in machine learning and data science, together with the use of new forms of data for furthering the platial approach to academia using quantitative feminist geography. In one sense this is not new, as feminist geography has always responded to the neglected experiences of women and to the biases in knowledge production in the discipline (Kwan Citation2002). Moreover, it has also long sought to ensure that women are included “as subjects in geographic research using … quantitative methods” (McDowell Citation1992, 2). Of course, we are not the first to note the scalar aspects to the gendered differences in experiences in HE: from microaggressions at the department level, to parental leave policies at the institutional level, and on up to funding decisions across disciplines. We note, too, Franklin et al.’s (Citation2022) argument that with our “ever-increasing computational capacity, more rigorous methods, and expanding data availability, [we can] addresses the particular needs of under-represented and marginalized groups” (2). What we want to explore is how these threads might be knitted together into a larger agenda that brings geography and geographers into the conversation.
We know that our proposed research agenda will not hear the individual voices, stories, and experiences of staff and students, but it will help us to develop a more nuanced understanding of how the dynamics of inequality play out not just at different scales but also in unique, singular places. We believe that there are opportunities here to appropriate tools often used to oppress and control (Eubanks Citation2018; Noble Citation2018) in ways that help to hold existing power structures to account and, as such, hope to position our work alongside the broader critique mounted by D’Ignazio and Klein (Citation2020) in Data Feminism.
The structure of this article is as follows: We start by briefly theorizing gender, power, and gendered organizations before placing our critiques within the current literature on women in academia. The final section outlines our agenda and discusses reconceptualizing the definition of gender in quantitative work with a focus on intersectionality, as well as how we can collect data through new sources and detail how data science techniques and quantitative geographical methods can be useful in examining this in new ways. We use UK academia as a lens to examine these issues but seek to show how this approach transfers to other national contexts.
Theorizing Gender and Gendered Organizations
Before exploring the literature on women’s experiences and challenges of working in HE, we begin by defining the key term of gender, and how it relates to power and organizations.
Defining Gender
Gender is traditionally constructed as the binary of man and woman, masculine and feminine, and “is one of the most widespread classification systems in the world today” (D’Ignazio and Klein Citation2020, 100). The gender binary allows only two rigid and distinct groups, usually based on a person’s sex assigned at birth, showing how strongly society links sex and gender (Gilbert Citation2008; Lindqvist, Gustafsson Sendén, and Renström Citation2020). Gender is socially constructed, varies between cultures, changes over time, and relates to societal norms, roles, and behaviors (World Health Organization Citation2021). Lindqvist, Gustafsson Sendén, and Renström (Citation2020) argued that gender should be thought of in terms of four parts: bodily (traditionally thought of as sex), legal, expression, and identity. It is also important to recognize that the gender binary dismisses the experiences of nonbinary, genderfluid, and transgender people whose gender identity is not simply woman or man or is different from the one they were given at birth (Mihaljević et al. Citation2019). Drawing on key texts, such as Butler (Citation1990), West and Zimmerman (Citation1987), and de Beauvoir (Citation1949), we can define gender as fluid, beyond the binary of woman and man, a continuous performance, and as socially constructed. By extension, gender can vary over a person’s lifetime and is a spectrum of identities.
Power and Gendered Organizations
Bearing in mind the complex nature of gender, a person’s perceived gender identity influences how others view them and consequently shapes their experience of bias and structural discrimination, depending on whether they are within the dominant and privileged group (D’Ignazio and Klein Citation2020). In Data Feminism, D’Ignazio and Klein (Citation2020) defined power as:
the current configuration of structural privilege and structural oppression, in which some groups experience unearned advantages—because various systems have been designed by people like them and work for people like them—and other groups experience systematic disadvantages—because those same systems were not designed by them or with people like them in mind. (24)
Clearly, these systems exist and perpetuate themselves in and through networks of institutional and organizational power. Acker (Citation1990) argued that the resulting “gendered organizations” are
[a] means that advantage and disadvantage, [of] exploitation and control, [of] action and emotion, meaning and identity, are patterned through and in terms of a distinction between male and female, masculine and feminine. (146)
Acker (Citation1990)’s theory of gendered organizations outlines that through the construction of symbols and social structures, the gendered division of labor, and interactions between different genders, gendered organizations arise and interact with the ways power is distributed through organizational hierarchies (Acker Citation1990; Britton Citation2017), which includes within the HE sector.
What We Know about Women in Academia
This section explores the literature on the experiences and challenges of female staff and students within academia. We start with the widely used, but increasingly dated, “leaky pipeline” metaphor.
Framing the Problem
The leaky pipeline is an extensively used metaphor (e.g., Berryman Citation1983; Bennett Citation2011; Gasser and Shaffer Citation2014; Kloxin Citation2019) to describe gendered differences along the academic career path, especially in the sciences (Goulden, Mason, and Frasch Citation2011). The pipeline metaphor describes how women are disproportionately affected by the barriers and challenges in academia and, consequently, either leak out or opt out due to horizontal (quantity in the pipeline) or vertical (progression along the pipeline) segregation (Berryman Citation1983; Bennett Citation2011).
The pipeline model, however, has also been robustly critiqued as an overly narrow and linear model that assumes that all academic careers follow the same trajectory from doctoral student to professor (as shown in ). There is a clear “mismatch between a linear career path and the reality of many women’s professional lives” (Bennett Citation2011, 164), though, especially with the shift away from jobs for life to portfolio careers, hence why other models have been suggested. Recently, Berhe et al. (Citation2022) proposed the metaphor of the hostile obstacle course, arguing that the passivity of the leaky pipeline metaphor “betrays” the actual experiences of people in gender, race, class, and ability minorities. They placed this analogy “in the context of scientific racism, colonial legacies and systemic biases that permeate our disciplines and institutions” (Berhe et al. Citation2022, 2).
Figure 1 The traditional UK academic career path with the percentage of females at each stage of the UK pipeline (HESA Citation2021a, 2022).
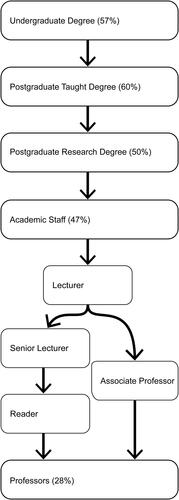
Blickenstaff (Citation2005) also argued that women are actively filtered out of the research pipeline by a “sex-based filter” and do not passively “leak away,” even if “no single issue can be called the primary cause” (384). The multiple pathway composite model suggests multiple ways for an individual to get from A to B and proposes the inclusion of a researcher’s sociocultural context because the leaky pipeline model is “inadequate to illuminate differences across STEM sub-fields” (Cannady, Greenwald, and Harris Citation2014, 447). A different take is the “Vanish Box phenomenon,” to which Etzkowitz and Ranga (Citation2011) argued that althouh women appear to be leaving traditional science careers, they are instead using their skills and knowledge in related fields—in the case of science, technology, engineering, and medicine (STEM) this might be technology transfer—which is seen to give employees more flexibility in managing their work–life balance. Collectively, these models point to an urgent need for a better understanding of women’s life courses in academia, where experiences could differ dramatically.
Building Scale into Discussions on Women in Academia
When accounting for the structural inequalities in academia, researchers have put forward a range of reasons for why women are underrepresented in senior positions; these include the “chilly” climate, the lack of female role models, the unequal caring responsibilities, and disciplinary differences and the stereotypes associated with them. The examples that follow have been chosen to highlight the pernicious, but often inadvertent, ways that organizations put up barriers to progression by women and minority groups. We think it is helpful to view these as occurring at different, but compounding and overlapping, scales: the micro, meso, and macro.
A Lack of Female Role Models and Mentors. Posselt, Porter, and Kamimura (Citation2018) and Howe-Walsh and Turnbull (Citation2016) identified the paucity of female role models within departments as a barrier to women entering and progressing in academia. Howe-Walsh and Turnbull (Citation2016) stated that “the absence of ‘top tier’ women perpetuates the dominant male culture in academia and more women are needed in senior roles to encourage others to aspire to senior-level positions” (418). Having a senior female role model to support and guide doctoral students and early career researchers (ECRs) might help to alleviate the feelings of “otherness,” especially in male-dominated disciplines and departments such as those in STEM (Posselt, Porter, and Kamimura Citation2018).
The absence of women is often experienced at the microscale within departments: In one study undertaken by Posselt, Porter, and Kamimura (Citation2018), an interviewee noted that when there are female role models, “[female students] realise that this is a place where, as a female they will not stand out. And I think that makes a huge difference to them in terms of the environment” (397). Kloxin (Citation2019) supported this view that mentees from minority groups benefit from having mentors who have experienced similar life events to help with feelings of isolation, imposter syndrome and being “out of place.”
Kloxin (Citation2019) described how support from role models, mentors, and advocates is “crucial for shaping career paths and retaining talent from diverse backgrounds” (287). It should therefore not be surprising that greater gender diversity among staff in a university department often correlates with increasing the gender diversity of incoming students as well (Posselt, Porter, and Kamimura Citation2018). This is critical during the ECR phase when informal mentoring outside the supervisor–student relationship helps students to build professional relationships at conferences and while teaching on courses (Kloxin Citation2019).
A Chilly Climate. Often by default, office temperatures are often set to levels that suit the metabolic rates of men and create, quite literally, a chilly climate. As a result, Kingma and Van Marken Lichtenbelt (Citation2015) suggested that buildings need to reduce their gender biases in “thermal comfort” by setting slightly hotter office temperatures. The term is also used metaphorically, however, to describe a research environment and culture that is more suited to male researchers and that is unwelcoming to women. The effect was first described by Hall and Sandler (Citation1982) when discussing the subtle ways that students of different genders were treated and how this can affect the educational attainment and progress of female students in particular.
The metaphor can be linked to Acker’s (Citation1990) concept of the gendered organization, and Britton (Citation2017) framed it as patterns of “inequitable treatment that, as they accumulate, inhibit women’s confidence, self-esteem, and accomplishment” (7). More subtle patterns of inequities might include traditions of visits to the pub or nights out, whereas more obvious microaggressions might include verbal and nonverbal behaviors such as eye rolling and deep sighs by male colleagues when female staff are speaking and using “girls” to refer to female colleagues (Blithe and Elliott Citation2020). Cumulatively these contribute to a work environment that is every bit as uncomfortable as one set several degrees too cold.
Unequal Caring Responsibilities and a Lack of Work–Life Balance. Across the world, “75 percent of unpaid work is done by women,” which equates to between three and six hours a day (Criado Perez Citation2019, 70). Despite changing expectations and attitudes, women and mothers still take on an unequal amount of care work (for children and elderly relatives), household work and emotional labor within the family unit (Morgan et al. Citation2021). This work is known to affect women’s careers, with many women choosing to work in lower paid and part-time roles after having children (Criado Perez Citation2019). This extends the effects of gender on the experience of academic working beyond the boundaries of the department and its expectations to include wider mesoscale dynamics across workplaces and institutions.
Women have also been disproportionately affected by the COVID-19 pandemic (United Nations Citation2021), whether through the gendered differences in job losses, through working in undervalued, low-paid, and feminized jobs, or through school closures and the pressure of home schooling (Viglione Citation2020). For women working in academia, the challenges of the pandemic have played out in a decrease in research output and academic productivity as well as the pressure, felt by all academics, of changing their teaching from in-person to online formats.
Mothers in academia who take periods of leave can also expect negative effects on their career trajectory and, when they return, working arrangements and practices that are not well-suited to their needs (Morgan et al. Citation2021). Parenthood “sharply decreases” the academic productivity of mothers in the short term and there is a consensus in the literature that parenting has a larger effect on women’s careers than men’s (Morgan et al. Citation2021). Women working in academia surveyed by Goulden, Mason, and Frasch (Citation2011), Seierstad and Healy (Citation2012), and Morgan et al. (Citation2021) reported issues ranging from uneven support in the parental leave policies of their departments, a lack of flexibility regarding child care, and the unfair consequences of taking maternity leave.
Disciplinary Differences. It is critical to note that gender disparities are stronger in some disciplines than others: Those associated with care and health are disproportionately female, whereas those associated with logical thought and problem solving are predominately male (Meyer, Cimpian, and Leslie Citation2015). Women are overrepresented in the life (especially health-related areas such as the medical sciences and nursing), psychological, and social sciences, whereas they remain underrepresented in geological sciences, engineering, mathematics, and physics (Ceci et al. Citation2014).
In UK mathematics departments, women made up just 6 percent of professors in 2013, whereas the proportion of female PhD students remained stable at 30 percent between 2000 and 2010 (Mihaljević-Brandt, Santamaría, and Tullney Citation2016). The engineering and technology fields showed greater disparities, with females accounting for only one fifth of academic staff in 2018–2019 (HESA Citation2021a), whereas in medicine, dentistry, and other health disciplines the female to male staff ratio in 2018–2019 was 3:2 (HESA Citation2021a).
Globally, these effects also play out in Larivière et al.’s (Citation2013) examination of collaboration across 5 million (mainly) science papers authored by more than 27 million people. There are fewer citations of papers written by women, fewer international collaborations for women, and fewer first authorship positions for female researchers at a ratio of 1:1.93 (Larivière et al. Citation2013). It is therefore unsurprising that, within STEM, there is substantive literature on the deeply entrenched underrepresentation of women (Ceci et al. Citation2014; Holman, Stuart-Fox, and Hauser Citation2018).
Closer to home, Schurr, Müller, and Imhof (Citation2020) assessed the membership and editorship of handbooks, progress reports, and journals across geography, finding “a strong correlation between the share of female editors and the share of female authors” (324). Similarly, Franklin et al. (Citation2021) noted that the gender diversity of editorial boards affects the production of geographical knowledge because it affects the range of voices and experiences that are heard. Of particular relevance is Franklin et al.’s (Citation2021) focus on the role of gender and gatekeeping within quantitative human geography: 31.5 percent of the editorial roles were filled by women, with a noticeable lack of “senior” women even if more balance is observed at the “younger academic age” (52).
Demonstrating, however, the macrodynamics in play even where finer grained work might suggest significant improvement, in terms of international diversity, board members from North America and Europe dominate the eight quantitative human geography journals with only 32.6 percent of the team members coming from non-Anglophone countries (Franklin et al. Citation2021). In line with Schurr, Müller, and Imhof’s (Citation2020) work on the discipline, Franklin et al. (Citation2021) also urged geographers to “be proactive in forcing ourselves to make changes today for the benefit of generations to come and geography to come” (56).
Women as Early Career Researchers and PhD Students
Returning to the longitudinal view of , we can see that 57 percent of students at the undergraduate level and 60 percent of postgraduate students in the United Kingdom identify as female (Advance HE Citation2021b; HESA Citation2021b). If there are so many female HE students, though, why has this not yet translated into senior academic positions? Within academic careers there is a lot of latency—the time needed for improvements earlier in the training and development process to feed through the academic ranks—but the doctor of philosophy (PhD) marks the point where academic careers start and yet remains grossly underexamined. The PhD is more than simply an academic qualification; it encompasses transferable research, written, and oral communication skills, and it is critical to the student’s professional preparation and development.
In the 2020–2021 academic year, 36,095 postgraduate research (PGR) students—those studying for a PhD, professional doctorates, and research master’s degrees—began their studies in the United Kingdom (HESA Citation2022). Approximately 12 percent of PGR students disclosed that they have a disability and the largest ethnic groups PGR students identified as were White (76.3 percent), followed by Asian (8.8 percent), and then Black (5.1 percent; HESA Citation2022). Slightly more people identifying as females (50.6 percent) began their PGR study than people identifying as males (48.9 percent), and the number of enrolled females has been gradually increasing over the past five years. Indeed, the 2019–2020 academic year was the first in which female students overtook males as first-year PGR students (HESA Citation2022). The share of PGR students identifying as the sex category “other” is 0.5 percent, which would include people identifying as nonbinary or another gender-nonconforming identity, but that level of granularity is unavailable in the reported data.
HESA data point toward a persistent gender divide between STEM and non-STEM subjects at the PGR level (HESA Citation2021b): More males studied STEM subjects than females—and the reverse is true for non-STEM subjects—with the physical sciences, engineering, and mathematical sciences being noticeably more male-dominated. More female-dominated disciplines included medicine, psychology, biological, and social sciences. Looking specifically at geography PGR students, although more female students were studying both physical and human geography than males in the United Kingdom (HESA Citation2021b), in the United States Kaplan and Mapes (Citation2016) found that “female PhDs are negatively affected by the GIS [geographic information systems] composition of the faculty and positively affected by the human and environment composition” (433). Kaplan and Mapes (Citation2016) authored one of only a few studies to use quantitative data to assess the gender diversity of a discipline at a PhD level.
When comparing female academic staff to ECRs, who tend to be in the first few years of their academic career, “[they] have quite different experiences as they have not (yet) faced the same structural barriers and are still paving their academic path” making this an important phase of the academic career to examine (Bourabain Citation2020, 5). In their research, Bourabain (Citation2020) highlighted the need for specific policies that target the “microclimates” of the workplace, which does not mean simply hiring more women or introducing diversity policies, but improving the research environment so that female ECRs are properly encouraged and supported throughout their careers. It can be harder for female researchers to build an academic identity than for their male colleagues because of the typically masculine characteristics that are expected of excellent researchers (Cidlinská Citation2019). The barriers to ECR careers are both individual and structural and as the current academic system is “masculine and androcentric,” there is a need for a deeper and wider transformation within academia culture to effect long-lasting changes (Cidlinská Citation2019, 379).
Promisingly, some departments have already moved toward gender parity among PhD students: Posselt, Porter, and Kamimura (Citation2018) conducted a comparative case study analysis of one chemistry and one civil and environmental engineering (CEE) department, from a U.S. university context, demonstrating that change can happen quite quickly. Facing a need for change thanks to low national rankings and a declining national reputation, the chemistry department made conscious changes to encourage females to apply and to shape departmental policies that were more gender inclusive so as to attract more female staff (Posselt, Porter, and Kamimura Citation2018). They found that, as the staff body became more diverse, the student community became more diverse as well because it was seen as providing a more welcoming environment for female PhD students (Posselt, Porter, and Kamimura Citation2018). Strategies employed by the CEE department included mentoring and encouraging high-performing undergraduate female students to apply for the PhD program, as well as promoting a gender-neutral admissions and recruitment process for doctoral programs (Posselt, Porter, and Kamimura Citation2018).
Arguing for a Quantitative Feminist Geography Approach
In a special issue on this topic in The Professional Geographer, McLafferty (Citation1995) argued that “quantitative methods are well suited to describe and probe the measurable aspects of women’s lives, to analyze spatial associations, and to document spatial and temporal inequalities” (438), and Ropers-Huilman and Winters (Citation2011) stressed that “quantitative feminist methodology can provide invaluable information about gendered lives” and answer “important questions that need further investigation” (672) within gender research. Quantitative methods are therefore “particularly helpful for providing a broad ‘picture’ of the social, spatial, or temporal inequalities women experienced at various spatial scales” (Kwan Citation2002, 8). The feminist critique of gendered organizations in general, and of academia in particular, however, has been largely rooted in qualitative and case study approaches, and this limits the range of evidence about what can and should be changed here and now.
There is good reason for this state of affairs: Spierings (Citation2012) argued that “in mainstream gender studies, quantitative research is still seen as bad” (332), and noted that little quantitative feminist social science was conducted in the preceding decade. It is to some extent inherent to feminist critiques that quantitative methods cannot understand, measure, or count women’s experiences; however, a more nuanced argument is that quantitative methods simply are not suitable for, or capable of, answering every research question and so should not be used as a blanket or default approach (Kwan Citation2002). A quantitative feminist approach to geography is not new, with Kwan (Citation2002) noting that “quantitative methods have been used in feminist geographic research since the early days of feminist geography” (2). We would concur with Scott (Citation2010) that “there is no such thing as a best method for researching gender inequalities” (226), as a mix of methods “leads to the most insight, because different methods are complementary, not substitutions of each other” (Spierings Citation2012, 344).
Papers such as Larivière et al. (Citation2013), Holman, Stuart-Fox, and Hauser (Citation2018), and Ceci et al. (Citation2014) highlight the importance of quantitative research on gender in HE and have provided critical insights into the dynamics of gender in STEM. For other research modes to be as effective as possible, it is important to have a benchmark against which to compare strategies, research, and policy recommendations and to systematically monitor phenomena at different scales within HE. For example, if we know that certain departments within universities have a better balance of people from different genders, races, ages, and backgrounds, then we can seek to understand how that has come about and evaluate the transferability of these strategies to other settings.
Is the study of gendered relations within HE and the use of quantitative data and research methods not already quantitative feminist geography? Well, yes; however, many researchers in the field tend to be primarily interested in examining the gendered dynamics within their own discipline, and do not necessarily have place in mind. We would argue that disconnecting the discipline from its institutional context weakens our understanding of the gender dynamics in HE. Spatial approaches typically model “the fact that nearby observations depend on one another regardless of regional boundaries” (Wolf et al. Citation2021, 1), but a platial model examines relationships among people from the same place. So what geographers contribute here is a conceptualization of space and place as part of the problem: Building on Acker’s (Citation1990) gendered organizations theory, we conceptualize academia as a set of multiscalar platial interactions within the hierarchical structure of HE (see ). The flowchart in shows why it is important to consider how gender—or, indeed, any other type of diversity—could be affected by differing platial processes.
Figure 2 The multiple scales—discipline, institution, department, and researcher—of exclusionary processes within higher education.
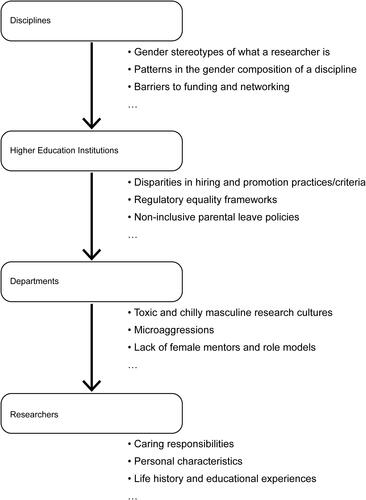
Combining quantitative and feminist geography perspectives, we can develop a more nuanced view of the overall challenge. We propose three pillars for a new agenda:
Reconceptualizing the definition of gender and reaffirming the importance of intersectionality within data collection and quantitative research.
Using and developing new sources of open data such as university Web sites, journal publications, social media, and thesis metadata services.
Using geographical modeling approaches to better understand the interaction of place(s) with gender.
These three pillars of this quantitative feminist geographical approach to gender in HE are elaborated and discussed in the sections that follow.
Reconceptualizing Gender and Reaffirming the Importance of Intersectionality
We highlight the need to bring feminist thinking into quantitative geographical research by broadening the definition of gender and reaffirming the importance of intersectionality. In her recent paper on uncertainty in quantitative geographical methods, Franklin (Citation2022) highlighted how the social construction of classifications, such as gender and race, often fails to allow for people who do not neatly fit into a single box. Similarly, D’Ignazio and Klein (Citation2020) invited us “to challenge the gender binary, along with other systems of counting and classification that perpetuate oppression” (97). Yet, within quantitative geography, it is unusual for research to consider gender identities beyond the gender binary when measuring and collecting data; as Franklin et al. (Citation2022) noted, “our understanding of gender identity and biological sex is rapidly evolving—a gender binary is hopelessly inadequate to capture gendered experiences—and our data and methods inevitably lag” (4).
Much of the quantitative data from censuses, surveys, and administrative sources are collected as one of three categories: female, male, and other (Westbrook and Saperstein Citation2015; D’Ignazio and Klein Citation2020). As Lindqvist, Gustafsson Sendén, and Renström (Citation2020) argued, however, there are numerous ways in which gender data could be collected in a more inclusive way that does not “other” people including, for example, the provision of a free text response for people to self-identify their gender. Reconceptualizing the definition of gender in quantitative research to allow for a spectrum of identities that go beyond the man–woman binary would help us to develop a much more nuanced understanding of the broad spectrum of staff and students who do not simply identify as female or male (Lindqvist, Gustafsson Sendén, and Renström Citation2020).
Although an intersectional approach can give us new ways of conceptualizing inequality and bias, we need to recognize that a proliferation of genders might also create a situation in which quantitative models lose explanatory power because of small sample sizes and disclosure restrictions. In other words, the deployment of models through which we can both learn from, and challenge, each other could be inhibited by privacy-related restrictions on access to data about exactly those groups that are most likely to be encountering bias in their everyday lives. To date, there seems to have been little consideration of how we might begin to navigate the trade-off between model power and model inclusivity. We return to this issue later on.
Examining this tension—we do not propose a unique resolution—is important in developing quantitative intersectional approaches to tackle the multiple systems of discrimination and oppression at play in academia (ACE and CIHE Citation2021; Brockman Citation2021; Castro and Collins Citation2021). The work of Black feminist scholars such as Patricia Hill Collins and Kimberlé Crenshaw in the 1980s and 1990s showed the importance of considering and understanding how a person’s multiple identities affect their experiences in certain settings (Crenshaw Citation1991; D’Ignazio and Klein Citation2020). It is difficult to understand the dynamics and patterns of minoritized groups when looking at one characteristic, such as gender, in isolation as, for example, Black able-bodied women will experience different discriminations to White women with a disability. The HESA data, presented previously, showed the stark differences in the number of professors by gender and race and highlighted the underrepresentation of female BAME academics in particular.
Reconceptualizing gender and focusing on the intersection of personal characteristics in these ways allows for a more inclusive quantitative geography that recognizes the need for a broader categorization as well as acknowledging the uncertainty of current social categories.
Using New and Open-Source Data
The second pillar proposes an expansion of the sources and types of data, as well as the scales at which they are available, used in this field of research. HESA produces large amounts of data on UK HE (e.g., Advance HE Citation2021b; HESA Citation2021a, Citation2021b, 2022) but it is typically aggregated to separate institutional, disciplinary, and national scales. This is understandable given the way that ethical and privacy considerations are normally understood, but the absence of more granular scales—such as departments and even individuals—and any sense of the links between them creates a significant gap in our understanding of the place-based dynamics affecting women in academia.
The work of Larivière et al. (Citation2013), Mihaljević-Brandt, Santamaría, and Tullney (Citation2016), Jadidi et al. (Citation2017), and Holman, Stuart-Fox, and Hauser (Citation2018), among others, shows the potential benefits of gender research on the authorship of academic publications at the individual level. With the continued growth in “accidental and open” (Arribas-Bel Citation2014) data on people working and studying in HE—including sources such as LinkedIn profiles, university Web sites, social media, funding applications, and thesis metadata services (like the British Library’s [Citation2021] EThOS metadata and DART Europe)—many data sources that were not created with gender research in mind can nonetheless be used—with care—to further examine the field.
Thinking critically, and taking a data feminist (D’Ignazio and Klein Citation2020) approach in thinking about the data that we use, encourages us to consider biases inherent to the data as part of the research process: Who is being included or excluded, how were the data collected, and for what purpose? For example, disciplinary differences in the frequency and type of publications means that using only data from journal publications provides, at best, a partial portrait of those working and studying in the academic sector. Inherent biases and discriminatory practices in grant funding, time available for research, teaching responsibilities, career breaks, and reviewing practices could already be affecting the data that are available before data analysis and modeling begin. We would argue that data could be used alongside other sources of data to create a fuller picture of HE. For example, data from inside the academy and from individual institutions on things like PhD student completion rates could be used as a proxy for gender inequality and to indirectly assess departments within universities and disciplines; however, it is unknown how widely these data are reported, and it is assumed to be inaccessible to the wider research community.
Using open and accessible data brings new risks and different ethical implications and challenges compared to traditional sources of data, like surveys and censuses, which have tended to go through an ethical approval procedure prior to collection. Therefore, we need to consider issues of data privacy and how to ethically use personal data such as an individual’s name and information about their work. The current approach of institutional review boards and ethics panels tends to prioritize factors such as the legal risks to the institution, the preservation of individual anonymity, and alignment with the purposes for which data were originally collected. Missing from this is the perspective of affected individuals and groups whose perspectives might differ quite substantially from those of senior academics. To put it another way, the privacy of Black female professors is compromised not by poor ethical practices, but by the fact that there are so few of them that anonymity is effectively impossible.
Quantitative Geographical Methods: Multilevel Modeling, Machine Learning, and Data Science
The final pillar of this research agenda is the greater inclusion of newer quantitative methods in research on HE. Gender inferencing algorithms (GIAs), facial recognition algorithms (FRAs), and multilevel models (MLMs) will be used here as examples to illustrate the potentials and pitfalls of such an approach. GIAs, alongside FRAs, can be used to assign a gender when the information is not present in the data; for example, when examining gendered patterns over space and time in publications, doctoral theses, and conference proceedings. MLMs can be used to address questions of “where” when it comes to gendered inequalities within the hierarchy of higher education of individuals within departments within institutions through examining the platial inequities and identifying specific institutions and disciplines that do well or do badly in terms of gender parity.
Data Science Tools. Text analysis techniques can be used to infer gender from names and pronouns in text (where a language uses them); these approaches might sometimes be the simplest—and indeed only—way of ascribing gender (Santamaría and Mihaljević Citation2018), and several papers cited in this article used GIAs for this purpose (Larivière et al. Citation2013; Mihaljević-Brandt, Santamaría, and Tullney Citation2016; Holman, Stuart-Fox, and Hauser Citation2018). GIAs typically use a database of name-to-gender combinations and the frequency at which they occur to predict the likelihood that a name is female, male, or unknown (Wais Citation2016; Sebo Citation2021). Among the biases that this perpetuates are the reinforcement of the two-gender binary, the systematic inference of Asian names as unknown, and the generation of both false positives and false negatives. In addition, some of the services are expensive and require knowledge of programming to use (Santamaría and Mihaljević Citation2018).
In a similar vein, FRAs are also being widely deployed for commercial and research use, and their more obvious ethical impacts are coming to the forefront of debates (Khalil et al. Citation2020). Despite their creative use in augmenting GIAs to improve overall prediction accuracy in the context of examining bias (Karimi et al. Citation2016), FRAs also have inherent gender and racial biases, as documented in Buolamwini and Gebru’s (Citation2018) Gender Shades work. FRAs are known to perform worse on darker skin and female faces than lighter skin and male faces (Buolamwini and Gebru Citation2018). Therefore, with “public scandals emerging over the lack of transparency, misuse of data, and the propagation of systemic racism,” there is a need for increased scrutiny of these techniques to mitigate potential harm and damage to communities and individuals (Brown, Davidovic, and Hasan Citation2021, 1). Since Buolamwini and Gebru’s (Citation2018) initial investigation, Microsoft has improved its gender recognition software Face Detect, but more needs to be done to remove bias and promote inclusivity in these algorithms (Raji et al. Citation2020).
Again, we must engage with the inherent tension between the opportunity to add valuable predictor variables and interaction effects to our models of bias, and the ongoing ethical challenges raised by such methods. Both would obviously be improved with more representative training data: from a wider range of cultures and countries (especially for GIAs), and from a larger range of skin colors and gender identities (for FRAs). This is not going to solve the problem, however, and we will be left with difficult trade-offs: Can the known biases of the methods be set against the potential benefits of the knowledge created by their use? Do we have an overriding obligation to examine and uncover the oppression and biases that continue to occur to hold power and the people and organizations who benefit from it to account? Feminist geography has always engaged with the ethics of methodological practice, and this is a new opportunity for quantitative feminist geographers to engage with the ethics of machine learning and artificial intelligence in ways that raise substantively new questions about their role in research. Can we employ techniques that have been strongly critiqued by many in feminist geography in ways that support the emergence of novel quantitative feminist geography critiques of institutions and disciplines?
Returning briefly to the model power and inclusivity trade-off question, a more inclusive construction of data science methods—such as widening the scope of gender identities in data collection—is necessarily one in which we are less confident in our results thanks to the interactions between small sample sizes and more complex model specifications. So an inclusive data science is one in which there is likely to be a decline in explanatory power, and this has usually been seen as a weakness—with p value hacking and the drive for “good results” being the result—but in another framing it draws attention to what we do not know and suggests the need for greater humility before our data. Stressing diversity and uncertainty might be an uncomfortable outcome, but it is also an important one!
Geographically Aware Tools. Finally, geographically aware models bring new ideas to the study of gender in academia and a few examples of models we could use include geographically weighted regression, point pattern analysis, spatial error models, spatial interaction modeling, and multilevel modeling. The last has been widely used in quantitative geography (Goldstein Citation2003, Citation2011; Owen, Harris, and Jones Citation2016), with Wolf et al. (Citation2021) stressing their popularity for accounting for group heterogeneity and “provid[ing] a notion of ‘platial’ dependence,” in which individuals and observations at the same place “are modelled as similar to one another” (1). In other disciplines, MLMs are used in fields such as health and education research to understand place-based and context-specific inequalities. For example, Evans et al. (Citation2018) used MLMs to examine “systems of oppression and privilege” in health inequalities across society to assess between- and within-category differences. Leckie (Citation2009) and Rasbash et al. (Citation2010) used MLMs to assess the relative effects of families, schools, and neighborhoods on pupil’s educational attainment to examine where within the educational hierarchy inequalities lie.
MLMs are not geographical per se but they are geographically aware and can be used to explore questions of a geographical nature by helping to avoid both the ecological (drawing conclusions for individuals based on aggregated group-level data) and atomistic (drawing incorrect conclusions for higher level groups using the data at the lower level of the individual) fallacies (Owen, Harris, and Jones Citation2016). In a review paper on quantitative methods in feminist research, Spierings (Citation2012) noted that “when studying individuals, researchers can use multilevel models to see whether societal factors have an important role in causing differences” (339).
One reason that MLMs are often used in health and education research is that the data in these fields are usually hierarchical in nature with data for individuals nested in neighborhoods, and pupils nested in classrooms that are, in turn, nested in schools (Goldstein Citation2003, Citation2011; Leckie Citation2009). Applying this to an HE context, staff and students can, speaking very broadly, be nested in departments that are nested in universities that are nested within disciplines. This allows us to frame a multiscalar world in which the microlevel of researchers interacts with the meso- and macroscales of departments and universities to form a hierarchy (see ).
MLMs could therefore be used to examine how places affect gender diversity in HE in ways that that cannot be explained by simply assessing the individuals in isolation from the structures in which they are situated. Examining how the residual variance is split across different intellectual scales could help us to understand how gender diversity compares for the same departments in different institutions and disciplines, as well as how the departments within a single university vary in their diversity. Breaking down the problem of diversity into the micro-, meso-, and macroscales allows us to consider the effect of micro-, meso-, and macroscale processes () that occur at each part of the hierarchy of HE, thus creating a benchmark on which future research can build.
Conclusion
Gender inequality and a lack of diversity in HE, alongside other forms of oppression and discrimination, are global, historic, and systemic. Things do change, however, and with the move from jobs for life to portfolio careers, for instance, aspatial—and a-platial—framings such as the leaky pipeline (and even more recent metaphors, such as the hostile obstacle course; see Berhe et al. Citation2022) no longer seem to fully capture how academic careers are structured or experienced. Conceptualizing academia as a hierarchical, platial structure of disciplines, institutions, and departments (set in particular geographical contexts) seems an important way to further our understanding of the gendered dynamics within HE. We argue that this multiscalar approach helps us to more appropriately contextualize the reasons why women, nonbinary people, and other minorities leave academia, whether it is through the experience of microaggressions (inherent sexism, racism, ableism, ageism, etc.), mesoscale (often toxic) masculine work environments, or the macroscale distribution of caring responsibilities; or as seems rather more likely, some combination of all these and more.
Working at the intersection of quantitative and feminist geography, we can gain a new understanding of, and create a new approach to monitoring and benchmarking, the HE sector through a more inclusive and detailed lens with a sector-wide and multiscalar understanding. Using the context of the United Kingdom and data from HESA as a lens through which to explore the challenges that our sector faces exemplifies what is available—and what is needed—to take a quantitative feminist geographical approach to this area of research.
Indeed, this is the program in which we are currently engaged, the experience of which prompted these wider reflections. The three pillars that we have set out here highlight important steps that need to become staple parts of our ongoing research. We not only need to reconceptualize gender, but to further develop our understanding of intersectionality in a quantitative research setting. Expanding the social categories used in everyday life will, we hope, allow us to include groups who have been historically overlooked and left out of mainstream research. As well, underutilized sources of data, such as thesis metadata, social media, and university Web sites, hold a wealth of data that move us beyond the structural biases inherent in the analysis of academic publications already being used to examine patterns of diversity within individual disciplines. Finally, using data science approaches such as text- and image-based methodologies alongside geographically aware models can help us to understand if there is something about specific places that affects gender diversity in HEIs that cannot be explained by looking at individuals, institutions, or disciplines in isolation.
This calls for a multidisciplinary approach to maximize the available resources—theories, data, and methods—but we would position geography as a discipline, and place as an analytical concept, as the critical locus for engaging with multiple national and subnational contexts. This step is critical for diversifying HE for the people working and studying within it: Academia will not become as diverse and as inclusive as it should be without a recognition of the importance of place and the monitoring and benchmarking of the sector as a whole. Academia ultimately reflects a country’s society and crucial research takes places on behalf of the public, therefore we need to represent the people who fund and benefit from our research.
Acknowledgments
We would like to thank the reviewers for their very thoughtful and encouraging feedback on the earlier version of this research, which is part of Laura Sheppard’s CASE PhD project on gender in UK higher education. The work is funded by the Economic and Social Research Council (part of UKRI) through the UBEL Doctoral Training Partnership, and by the British Library as industry partner. We would like to thank them for their continued support of this research.
Additional information
Notes on contributors
Laura Sheppard
LAURA SHEPPARD is a PhD Student in the Centre for Advanced Spatial Analysis, University College London, 90 Tottenham Court Road, London, W1T 4TJ, UK. E-mail: [email protected]. Her research interests include using quantitative human geography methods and data science to examine social and spatial inequalities.
Jonathan Reades
JONATHAN READES is an Associate Professor of Spatial Data Science in the Centre for Advanced Spatial Analysis, University College London, 90 Tottenham Court Road, London, W1T 4TJ, UK. E-mail: [email protected]. His interests include economic geography, housing and neighborhoods, open data and teaching, reproducible research, and emerging quantitative methods.
R. P. J. Freeman
R. P. J. FREEMAN is an Associate Professor of Doctoral Education in the IOE, UCL’s Faculty of Education and Society, University College London, 20 Bedford Way, London, WC1H 0AL, UK. E-mail: [email protected]. His interests include doctoral education; diversity, equality, and inclusion within higher education; and higher education professionals.
References
- Acker, J. 1990. Hierarchies, jobs, bodies: A Theory of gendered organizations. Gender and Society 4 (2):139–58. doi: 10.1177/089124390004002002.
- Adams, R. 2020. Fewer than 1 percent of UK university professors are black, figures show. The Guardian, February 27. Accessed July 5, 2022. https://www.theguardian.com/education/2020/feb/27/fewer-than-1-of-uk-university-professors-are-black-figures-show.
- Advance HE. 2021a. Staff statistical report 2021. Advance HE, York, UK.
- Advance HE. 2021b. Students statistical report 2021. Advance HE, York, UK.
- American Association of University Women (AAUW). 2022. Fast facts: Women working in academia. Accessed August 2, 2022. https://www.aauw.org/resources/article/fast-facts-academia/.
- American Council on Education and Center for International Higher Education (ACE and CIHE). 2021. Women’s representation in higher education leadership around the world. Accessed September 3, 2021. https://www.acenet.edu/Documents/Womens-Rep-in-Higher-Ed-Leadership-Around-the-World.pdf.
- Arribas-Bel, D. 2014. Accidental, open, and everywhere: Emerging data sources for the understanding of cities. Applied Geography 49:45–53. doi: 10.1016/j.apgeog.2013.09.012.
- Bennett, C. 2011. Beyond the leaky pipeline: Consolidating understanding and incorporating new research about women’s science careers in the UK. Brussels Economic Review 54:149–76.
- Berhe, A. A., R. T. Barnes, M. G. Hastings, A. Mattheis, B. Schneider, B. M. Williams, and E. Marín-Spiotta. 2022. Scientists from historically excluded groups face a hostile obstacle course. Nature Geoscience 15 (1):2–4. doi: 10.1038/s41561-021-00868-0.
- Berryman, S. E. 1983. Who will do science? Trends, and their causes in minority and female representation among holders of advanced degrees in science and mathematics: A special report. The Rockefeller Foundation, New York.
- Blickenstaff, J. C. 2005. Women and science careers: Leaky pipeline or gender filter? Gender and Education 17 (4):369–86. doi: 10.1080/09540250500145072.
- Blithe, S. J., and M. Elliott. 2020. Gender inequality in the academy: Microaggressions, work–life conflict, and academic rank. Journal of Gender Studies 29 (7):751–64. doi: 10.1080/09589236.2019.1657004.
- Bourabain, D. 2020. Everyday sexism and racism in the ivory tower: The experiences of early career researchers on the intersection of gender and ethnicity in the academic workplace. Gender, Work and Organization 28 (1):248–67. doi: 10.1111/gwao.12549.
- The British Library. 2021. Ethos: E-thesis online service. Accessed January 10, 2021. https://ethos.bl.uk/.
- Britton, D. M. 2017. Beyond the chilly climate: The salience of gender in women’s academic careers. Gender and Society 31 (1):5–27. doi: 10.1177/0891243216681494.
- Brockman, A. J. 2021. “La crème de la crème”: How racial, gendered, and intersectional social comparisons reveal inequities that affect sense of belonging in STEM. PLoS ONE 91 (4):1–8. doi: 10.1080/00330124.2020.1828944.
- Brown, S., J. Davidovic, and A. Hasan. 2021. The algorithm audit: Scoring the algorithms that score us. Big Data and Society 8 (1):1–8.
- Buolamwini, J., and T. Gebru. 2018. Gender shades: Intersectional accuracy disparities in commercial gender classification. In Proceedings of Machine Learning Research, 81:1–15. Conference on Fairness, Accountability, and Transparency.
- Butler, J. 1990. Gender trouble: Feminism and the subversion of identity. London and New York: Routledge.
- Cannady, M. A., E. Greenwald, and K. N. Harris. 2014. Problematizing the STEM pipeline metaphor: Is the STEM pipeline metaphor serving our students and the STEM workforce? Science Education 98 (3):443–60. doi: 10.1002/sce.21108.
- Castro, A. R., and C. S. Collins. 2021. Asian American women in STEM in the lab with White men named John. Science Education 105 (1):33–61. doi: 10.1002/sce.21598.
- Ceci, S. J., D. K. Ginther, S. Kahn, and W. M. Williams. 2014. Women in academic science: A changing landscape. Psychological Science in the Public Interest 15 (3):75–141. doi: 10.1177/1529100614541236.
- Cidlinská, K. 2019. How not to scare off women: Different needs of female early-stage researchers in STEM and SSH fields and the implications for support measures. Higher Education 78 (2):365–88. doi: 10.1007/s10734-018-0347-x.
- Crenshaw, K. 1991. Mapping the margins: Intersectionality, identity politics, and violence against women of color. Stanford Law Review 43 (6):1241–99. doi: 10.2307/1229039.
- Criado Perez, C. 2019. Invisible women: Exposing data bias in a world designed for men. 1st ed. London: Chatto and Windus.
- de Beauvoir, S. 1949. The second sex, trans. and ed. H. M. Parshley. New York: Vintage Books.
- D’Ignazio, C., and L. Klein. 2020. Data feminism. Cambridge, MA: The MIT Press.
- Etzkowitz, H., and M. Ranga. 2011. Gender dynamics in science and technology: From the “leaky pipeline” to the “vanish box.” Cahiers Économiques de Bruxelles 54:131–47.
- Eubanks, V. 2018. Automating inequality: How high-tech tools profile, police, and punish the poor. New York: St. Martin’s Press.
- Evans, C. R., D. R. Williams, J. Onnela, and S. V. Subramanian. 2018. A multilevel approach to modelling health inequities at the intersection of multiple social identities. Social Science & Medicine 203:64–73. doi: 10.1016/j.socscimed.2017.11.011.
- Franklin, R. 2022. Quantitative methods I: Reckoning with uncertainty. Progress in Human Geography 46 (2):689–97. doi: 10.1177/03091325211063635.
- Franklin, R. S., E. C. Delmelle, C. Andris, T. Cheng, S. Dodge, J. Franklin, A. Heppenstal, M. P. Kwan, W. Li, S. McLafferty, et al. 2022. Making space in geographical analysis. Geographical Analysis:1–17. doi: 10.1111/gean.12325.
- Franklin, R. S., V. Houlden, C. Robinson, D. Arribas-Bel, E. C. Delmelle, U. Demšar, H. J. Miller, and D. O’Sullivan. 2021. Who counts? Gender, gatekeeping, and quantitative human geography. The Professional Geographer 73 (1):48–61. doi: 10.1080/00330124.2020.1828944.
- Gasser, C. E., and K. S. Shaffer. 2014. Career development of women in academia: Traversing the leaky pipeline. The Professional Counselor 4 (4):332–52. doi: 10.15241/ceg.4:332.
- Gilbert, M. A. 2008. Defeating bigenderism: Changing gender assumptions in the twenty-first century. Hypatia 24 (3):93–112. http://www.jstor.org/stable/20618166. doi: 10.1111/j.1527-2001.2009.01047.x.
- Goldstein, H. 2003. Multilevel modelling of educational data. Dordrecht, The Netherlands: Springer.
- Goldstein, H. 2011. Multilevel statistical models. 4th ed. Hoboken, NJ: Wiley.
- Goulden, M., M. A. Mason, and K. Frasch. 2011. Keeping women in the science pipeline. The Annals of the American Academy of Political and Social Science 638 (1):141–62. [Database] doi: 10.1177/0002716925.
- Hall, R. M., and B. Sandler. 1982. The classroom climate: A chilly one for women? In The classroom climate: A chilly one for women?, 1–24. Washington, DC: Association of American Colleges, Project on the Status and Education of Women.
- HESA. 2021a. All staff (excluding atypical) by equality characteristics 2014/15 to 2019/20. Accessed December 2, 2021. https://www.hesa.ac.uk/data-and-analysis/staff/table-27.
- HESA. 2021b. Who’s studying in HE? Accessed December 2, 2021. https://www.hesa.ac.uk/data-and-analysis/students/whos-in-he.
- HESA. 2022. Higher education student statistics: UK, 2020/21—Student numbers and characteristics. Accessed February 2, 2022. https://www.hesa.ac.uk/news/25-01-2022/sb262-higher-education-student-statistics/numbers.
- Hillier, K. M. 2021. Academia and motherhood: A narrative inquiry of Ontario academic mothers’ experiences in university graduate programs. Journal of Family Issues. Advance online publication. doi: 10.1177/0192513X211064864.
- Hofstra, B., V. V. Kulkarni, S. Munoz-Najar Galvez, B. He, D. Jurafsky, and D. A. McFarland. 2020. The diversity–innovation paradox in science. Proceedings of the National Academy of Sciences of the United States of America 117 (17):9284–91. doi: 10.1073/pnas.1915378117.
- Holman, L., D. Stuart-Fox, and C. E. Hauser. 2018. The gender gap in science: How long until women are equally represented? PLOS Biology 16 (4):e2004956. doi: 10.1371/journal.pbio.2004956.
- House of Commons Women and Equalities Committee. 2016. The link to productivity. https://publications.parliament.uk/pa/cm201516/cmselect/cmwomeq/584/58406.htm.
- Howe-Walsh, L., and S. Turnbull. 2016. Barriers to women leaders in academia: Tales from science and technology. Studies in Higher Education 41 (3):415–28. doi: 10.1080/03075079.2014.929102.
- Jadidi, M., F. Karimi, H. Lietz, and C. Wagner. 2017. Gender disparities in science? dropout, productivity, collaborations and success of male and female computer scientists. Advances in Complex Systems 21 (3&4):1750011.
- Kaplan, D. H., and J. E. Mapes. 2016. Where are the women? Accounting for discrepancies in female doctorates in U.S. geography. The Professional Geographer 68 (3):427–35. doi: 10.1080/00330124.2015.1102030.
- Karimi, F., C. Wagner, F. Lemmerich, M. Jadidi, and M. Strohmaier. 2016. Inferring gender from names on the Web: A comparative evaluation of gender detection methods. In WWW’16 Companion Proceedings of the 25th International Conference Companion on World Wide Web, 53–54. doi: 10.1145/2872518.2889385.
- Khalil, A., S. G. Ahmed, A. M. Khattak, and N. Al-Qirim. 2020. Investigating bias in facial analysis systems: A systematic review. IEEE Access.8:130751–61. doi: 10.1109/ACCESS.2020.3006051.
- Kingma, B., and W. Van Marken Lichtenbelt. 2015. Energy consumption in buildings and female thermal demand. Nature Climate Change 5 (12):1054–56. doi: 10.1038/nclimate2741.
- King’s College London. 2021. Equality, diversity & inclusion annual report 2020–21. Accessed November 15, 2022. https://www.kcl.ac.uk/hr/diversity/di-at-kings/edi-annual-report-2020-21.pdf.
- Kloxin, A. M. 2019. Addressing the leaky pipeline through mentoring and support: A personal perspective. Nature Review Materials 4:287–89. doi: 10.1038/s41578-019-0109-0.
- Kozlowski, D., V. Larivière, C. R. Sugimoto, and T. Monroe-White. 2022. Intersectional inequalities in science. Proceedings of the National Academy of Sciences of the United States of America 119 (2):1–8. doi: 10.1073/pnas.2113067119.
- Kuzhabekova, A., and A. Almukhambetova. 2021. Women’s progression through the leadership pipeline in the universities of Kazakhstan and Kyrgyzstan. Compare: A Journal of Comparative and International Education 51 (1):99–117. doi: 10.1080/03057925.2019.1599820.
- Kwan, M.-P. 2002. Quantitative methods and feminist geographic research. In Feminist geography in practice: Research and methods, ed. P. J. Moss, K. F. Al-Hindi, and H. Kawabata, 1–17. Blackwell.
- Larivière, V., C. Ni, Y. Gingras, B. Cronin, and C. R. Sugimoto. 2013. Bibliometrics: Global gender disparities in science. Nature 504 (7479):211–13. doi: 10.1038/504211a.
- Leckie, G. 2009. The complexity of school and neighbourhood effects and movements of pupils on school differences in models of educational achievement. Journal of the Royal Statistical Society: Series A (Statistics in Society) 172 (3):537–54. doi: 10.1111/j.1467-985X.2008.00577.x.
- Lindqvist, A., M. Gustafsson Sendén, and E. Renström. 2020. What is gender, anyway: A review of the options for operationalising gender. Psychology and Sexuality 12 (4):332–44. doi: 10.1080/19419899.2020.1729844.
- McDowell, L. 1992. Doing gender: Feminism, feminists, and research methods in human geography. Transactions of the Institute of British Geographers 17 (4):399–416. doi: 10.2307/622707.
- McLafferty, S. 1995. Counting for women. The Professional Geographer 47 (4):436–42. doi: 10.1111/j.0033-0124.1995.00436.x.
- Meyer, M., A. Cimpian, and S. J. Leslie. 2015. Women are underrepresented in fields where success is believed to require brilliance. Frontiers in Psychology 6:235. doi: 10.3389/fpsyg.2015.00235.
- Mihaljević, H., M. Tullney, L. Santamaría, and C. Steinfeldt. 2019. Reflections on gender analyses of bibliographic c1orpora. Frontiers in Big Data 2:29. doi: 10.3389/fdata.2019.00029.
- Mihaljević-Brandt, H., L. Santamaría, and M. Tullney. 2016. The effect of gender in the publication patterns in mathematics. PLoS ONE 11 (10):e0165367–23. doi: 10.1371/journal.pone.0165367.
- Morgan, A. C., S. F. Way, M. J. D. Hoefer, D. B. Larremore, M. Galesic, and A. Clauset. 2021. The unequal impact of parenthood in academia. Science Advances 7 (9):1–8. doi: 10.1126/sciadv.abd1996.
- Noble, S. U. 2018. Algorithms of oppression: How search engines reinforce racism. New York: New York University Press.
- O’Connor, P., and G. Irvine. 2020. Multi-level state interventions and gender equality in higher education institutions: The Irish case. Administrative Sciences 10 (4):98. doi: 10.3390/admsci10040098.
- Owen, G., R. Harris, and K. Jones. 2016. Under examination: Multilevel models, geography and health research. Progress in Human Geography 40 (3):394–412. doi: 10.1177/0309132515580814.
- Paswan, J., and V. K. Singh. 2020. Gender and research publishing analysed through the lenses of discipline, institution types, impact and international collaboration: a case study from India. Scientometrics 123:497–515. doi: 10.1007/s11192-020-03398-5.
- Posselt, J., K. B. Porter, and A. Kamimura. 2018. Organizational pathways toward gender equity in doctoral education: Chemistry and civil engineering compared. American Journal of Education 124 (4):383–410. doi: 10.1086/698457.
- Raji, I. D., T. Gebru, M. Mitchell, J. Buolamwini, J. Lee, and E. Denton. 2020. Saving face: Investigating the ethical concerns of facial recognition auditing. In Proceedings of the AAAI/ACM Conference on AI, Ethics, and Society. http://arxiv.org/abs/2001.00964. doi: 10.1145/3375627.3375820.
- Rasbash, J., G. Leckie, R. Pillinger, and J. Jenkins. 2010. Children’s educational progress: Partitioning family, school, and area effects. Journal of the Royal Statistical Society: Series A (Statistics in Society) 173 (3):657–82. doi: 10.1111/j.1467-985X.2010.00642.x.
- Rollock, N. 2019. Staying power: The career experiences and strategies of UK Black female professors. Technical report, University and College Union. Accessed August 2, 2022. https://www.ucu.org.uk/media/10075/Staying-Power/pdf/UCU_Rollock_February_2019.pdf.
- Ropers-Huilman, R., and K. T. Winters. 2011. Feminist research in higher education. The Journal of Higher Education 82 (6):667–90. http://www.jstor.org/stable/41337166. doi: 10.1353/jhe.2011.0035.
- Santamaría, L., and H. Mihaljević. 2018. Comparison and benchmark of name-to- gender inference services. PeerJ Computer Science 4:e156. doi: 10.7717/peerj-cs.156.
- Savigny, H. 2014. Women, know your limits: Cultural sexism in academia. Gender and Education 26 (7):794–809. doi: 10.1080/09540253.2014.970977.
- Schurr, C., M. Müller, and N. Imhof. 2020. Who makes geographical knowledge? The gender of geography’s gatekeepers. The Professional Geographer 72 (3):317–31. doi: 10.1080/00330124.2020.1744169.
- Scott, J. 2010. Quantitative methods and gender inequalities. International Journal of Social Research Methodology 13 (3):223–36. doi: 10.1080/13645579.2010.482258.
- Sebo, P. 2021. Performance of gender detection tools: A comparative study of name-to-gender inference services. Journal of the Medical Library Association 109 (3):414–21. https://jmla.pitt.edu/ojs/jmla/article/view/1185. doi: 10.5195/jmla.2021.1185.
- Seierstad, C., and G. Healy. 2012. Women’s equality in the Scandinavian academy: A distant dream? Work, Employment and Society 26 (2):296–313. doi: 10.1177/0950017011432918.
- Spierings, N. 2012. The inclusion of quantitative techniques and diversity in the mainstream of feminist research. European Journal of Women’s Studies 19 (3):331–47. doi: 10.1177/13505068124436215.
- United Nations. 2021. Goal 5: Achieve gender equality and empower all women and girls. Accessed January 15, 2021. https://sdgs.un.org/goals/goal5.
- Viglione, G. 2020. Are women publishing less during the pandemic? Here’s what the data say. Nature 581 (7809):365–66. doi: 10.1038/d41586-020-01294-9.
- Wais, K. 2016. Gender prediction methods based on first names with genderizeR. R Journal 8 (1):17–37. doi: 10.32614/rj-2016-002.
- Walker, L., I. Sin, C. Macinnis-Ng, K. Hannah, and T. McAllister. 2020. Where to from here? Women remain absent from senior academic positions at Aotearoa New Zealand’s universities. Education Sciences 10 (6):152. doi: 10.3390/educsci10060152.
- Wall, S. 2008. Of heads and hearts: Women in doctoral education at a Canadian university. Women’s Studies International Forum 31 (3):219–28. doi: 10.1016/j.wsif.2008.04.007.
- West, C., and D. H. Zimmerman. 1987. Doing gender. Gender & Society 1 (2):125–51. http://www.jstor.org/stable/189945. doi: 10.1177/0891243287001002002.
- Westbrook, L., and A. Saperstein. 2015. New categories are not enough: Rethinking the measurement of sex and Gender & Society 29 (4):534–60. doi: 10.1177/0891243215584758.
- Westerholt, R., F. B. Mocnik, and A. Comber. 2020. A place for place: Modelling and analysing platial representations. Transactions in GIS 24 (4):811–18. doi: 10.1111/tgis.12647.
- Wolf, L., L. Anselin, D. Arribas-Bel, and L. Mobley. 2021. On spatial and platial dependence: Examining shrinkage in spatially dependent multilevel models. Annals of the American Association of Geographers 111:1–13. doi: 10.1080/24694452.2020.1841602.
- World Health Organization. 2021. Gender and health. https://www.who.int/health-topics/gender#tab=tab_1.