Abstract
Advancements in mixed reality (MR) have led to innovative approaches in image-guided surgery (IGS). In this paper, we provide a comprehensive analysis of the current state of MR in image-guided procedures across various surgical domains. Using the Data Visualization View (DVV) Taxonomy, we analyze the progress made since a 2013 literature review paper on MR IGS systems. In addition to examining the current surgical domains using MR systems, we explore trends in types of MR hardware used, type of data visualized, visualizations of virtual elements, and interaction methods in use. Our analysis also covers the metrics used to evaluate these systems in the operating room (OR), both qualitative and quantitative assessments, and clinical studies that have demonstrated the potential of MR technologies to enhance surgical workflows and outcomes. We also address current challenges and future directions that would further establish the use of MR in IGS.
1. Introduction
Augmented Reality (AR), Virtual Reality (VR), and Mixed Reality (MR) technologies are increasingly being studied and used in image-guided surgery (IGS). To briefly differentiate between these terms: AR enhances the real world with digital or virtual content, VR immerses users in a completely virtual environment, and MR which combines elements of both is considered a continuum [Citation1] between the two extremes of a fully real world and a virtual one.
Mixed reality technologies have been studied in several surgical contexts including surgical education, planning, training, procedure rehearsal, and real-time guidance tasks, such as incision planning, navigating to surgical targets, avoiding critical structures, and determining the extent of resection. The integration of MR technologies into IGS systems has led to several benefits including more precise and accurate procedures, reduced risk of errors and complications, enhanced surgical workflows, and improved patient outcomes.
In this paper, we aim to assess the recent progress of MR technologies in IGS. Specifically, we wanted to quantify advancements since a 2013 review paper on the state-of-the-art visualization in MR IGS [Citation2]. The conclusions drawn from the 2013 review highlighted that, despite numerous technological innovations only a limited number of MR systems were tested or used by clinicians in the operating room (OR) during real clinical procedures. In light of the growing popularity of MR IGS systems over the past decade, we aimed to determine if more systems have been used in clinical studies or practice. To ensure comparability with the findings of the Kersten-Oertel et al.’s [Citation2] review, we adopted the use of the DVV Taxonomy [Citation3] for describing MR IGS systems and similar paper inclusion criteria, with one key distinction: our primary focus was on studies that reported testing in the OR. In the 2013 review, the interest in determining the extent of IGS system used in the OR led to a dedicated section covering systems tested in real clinical cases. However, this section consisted of only 15 papers with the majority of described systems only being used in a few surgical cases. Given the surge in recent publications on MR IGS systems, we focus exclusively on papers that describe testing of the MR IGS systems in the OR published in the last 7 years.
Our current search saw a significant rise in the use of MR systems in OR. In the previous review period, the average annual publication rate was 24.6 papers per year. In contrast, the current period had an average of 137.9 papers published per year, reflecting a substantial increase. The summarized search statistics are presented in .
Table 1. Past vs. present: comparison of MR IGS in or.
2. Methods
In the following section, we describe the DVV Taxonomy which was used for data extraction, our search strategy, inclusion and exclusion criteria, and screening process.
2.1. DVV Taxonomy
Kersten-Oertel et al. [Citation3] introduced the Data, Visualization processing, View (DVV) taxonomy with the aim of standardizing the description of IGS systems and establishing a common language to facilitate discussions and comprehension of MR IGS system components by both developers and users. This taxonomy provides a comprehensive breakdown of the main components and sub-components required to develop and evaluate an MR IGS system: the Data visualized, the Visualization processing applied to the data, and the View which encompasses where the data is visualized, the type of display device utilized, and the users’ interaction with the data. As the 2013 review used the DVV Taxonomy we employed this taxonomy in this review.
2.2. Search method
A systematic review was conducted following the Preferred Reporting Items for Systematic Reviews and MetaAnalysis (PRISMA) guidelines as shown in . Specifically, we used Google Scholar with the following search terms: ‘allintitle: reality surgery OR neurosurgery (augmented OR virtual OR extended OR mixed OR enhanced) from 2017’. The search was conducted on 4 July 2023 and resulted in an initial 1014 papers that met the search terms.
We are aware that the original search query from Kersten-Oertel et al. is conservative and potentially omits papers, e.g. studies published about biopsy or appendectomy do not necessarily include the word surgery in the title. However, we chose to base our query on theirs to obtain a meaningful comparison to the state-of-the-art in 2013. The search terms were refined after several queries and careful initial screening of results to reflect the evolving landscape of the field. For example, we included new terms, e.g. enhanced and extended that were seldom used a decade ago but are commonly used now. Additionally, to simplify the query, all previous explicit exclusions were discarded.
2.3. Screening and data extraction
The 1013 articles that resulted from our Google search underwent collective screening by the five authors (ZA, MA, NK, ÉL, and MKO). From these, we removed 49 duplicate publications leaving us with 964 papers. Among these, 141 articles were identified as literature reviews which were screened by two authors (ÉL and MKO). The research studies were screened by four authors (ZA, MA, NK, and MKO). After comparing the review papers with the research studies we decide to exclude the former from an extensive analysis due to significant overlapping information.
Our inclusion criteria encompassed conference and journal papers, case reports, and clinical trials that employed intraoperative MR technologies for patient treatment. Conversely, we excluded publications falling into several categories: (1) studies where the developed system was not tested in the OR, (2) abstracts, commentaries, letters to the editor, narrative reviews, or chapters, (3) studies exclusively involving phantom, animal, or cadaveric experiments, (4) systems intended solely for educational purposes, training, or surgical simulation, (5) MR systems exclusively used for preoperative planning, and (6) publications not written in English. Any disagreements regarding the fulfillment of inclusion and exclusion criteria were resolved through discussion, resulting in a consensus among all authors. In total, we identified 79 publications that met the predefined inclusion criteria. In , we provide the detailed count per exclusion reason for the past 2 years.
Table 2. Base exclusion reason categories and counts (2022–2024).
For the 79 selected papers, we extracted information into customized spreadsheets that were organized to align with the DVV taxonomy. Under the Data category, we documented the type of medical images used and the specific data visualized within the context of MR IGS systems. For Visualization processing, we recorded how the data was visualized, capturing details, such as color coding, transparency, volume rendering, meshes, and other relevant techniques. The View was further subdivided into perception location, type of display, and interaction methods. Additionally, we recorded information on the type of surgery, clinical study details including results, challenges, and limitations.
2.4. Recent related reviews
Our search yielded 141 literature review papers, which were excluded from the main analysis, but offer valuable supplementary insights into the state of the field. Here, we present a meta-review of these previous reviews. Out of the 141 reviews, 50 were excluded due to reasons, such as being out of scope, a duplicate, or not being peer-reviewed, etc. Among the remaining reviews, 17 were systematic reviews, with many employing the PRISMA methodology and focusing on specific surgical sub-specialties. The remainder consisted of scoping, methodological, and narrative reviews. The most frequently reviewed specialty, by a significant margin, was spine surgery (24). However, a considerable number of reviews also centered on maxillofacial (9), orthopedic (8), liver (6), vascular (5), or plastic (3) surgery. Furthermore, some reviews examined specific display technologies rather than medical specialties, with head-mounted displays (HMDs) being the most common (3). Additionally, certain reviews explored MR in relation to specific surgical methods, particularly robotic or robotically-assisted surgery (11) and minimally invasive surgery (9). It’s worth noting that there is overlap in the numbers presented above, as some reviews cover both methods and specialties or technologies and specialties. For example, a review might explore MR in robotics surgery for spine procedures or investigate the use of HMDs for spinal surgeries.
These reviews converge on several key points. Overall, MR technologies are recognized for having a beneficial impact on surgical procedures. While some reviews noted minimal or no effect, negative impacts were seldom reported. Positive effects primarily consisted of expedited procedure times and reduced surgical trauma, resulting in shorter patient recovery time and hospital stays. Consequently, these benefits contribute to overall cost reduction in healthcare. Furthermore, MR technologies have the potential to replace more expensive systems, leading to additional cost savings. For example, Moga et al. [Citation4] found that utilizing an HMD AR yielded similar clinical outcomes to those achieved with robot-assisted systems, but at a significantly lower cost.
The readiness of MR technology for surgical application is a topic of debate within the reviewed literature. While some reviews assert MR to be ready for clinical use, many argue it is still an immature technology. Moreover, several reviews highlight the limited amount of clinical data gathered on human subjects, precluding drawing meaningful clinical applicability conclusions at this point. In reviews where authors believe MR technologies have not reached clinical readiness, there is a general consensus that it will in the near future. However, it’s important to note that apparent discrepancies in readiness assessments between reviews may partly stem from the scope of our search. Significant technological advancements have occurred during the 7 year span covered by our analysis, potentially leading to differences over the time period.
Regarding the evolution of the field, a particularly interesting review that offers a broad perspective is that of Han et al. [Citation5]. This bibliometric review covers a span of nearly twenty years and offers unique insights into significant progress over time. Notably, the authors observe an exponential growth in the number of papers published per year during the period studied. This could in part explain the high level of confidence in other reviews about reaching clinical readiness in the short term.
3. State of the art using the DVV Taxonomy
Our analysis begins with a comprehensive examination of each component within the DVV Taxonomy, followed by an exploration of the surgical domain.
3.1. Data
The first component of the DVV taxonomy, Data, comprises of patient-specific data and visually processed data. Patient-specific data includes demographics, clinical scores, raw imaging data, and similar information, while visually processed data refers to data presented to users through the View component. The purpose of considering data as a component of IGS systems is to underscore the importance of determining which type of data should be displayed during surgery and at what stage of the procedure.
3.1.1. Patient-specific data
Consistent with the 2013 study [Citation2], the majority of the analyzed papers (63.3%) predominantly use preoperative computed tomography (CT) as their primary imaging modality. Approximately 12.7% of the publications use both CT and magnetic resonance imaging (MRI), while MRI alone accounts for 11.4% of the cases. As illustrated in , a significant majority (87.4%) of the publications in this study utilized either CT, MRI, or a combination of these two imaging technologies.
There were a few papers that used other imaging modalities. For example, in the studies by Gorpas et al. [Citation6] and Alfonoso-Garcia et al. [Citation7] Near-Infrared-Fluorescence (NIRF) was used. Molecular imaging with NIRF involves the use of contrast agents exhibiting fluorescent properties within the near-infrared (NIR) window, typically ranging from 700 to 900 nanometers (nm). Cabrilo et al. [Citation8] used Digital Subtraction Angiography (DSA), a fluoroscopic technique used in interventional radiology to visualize blood vessels, with CT and MRI. In the study by Liu et al. [Citation9] Positron Emission Tomography (PET), which involves the use of a radioactive drug (tracer) to visualize both normal and abnormal metabolic activity in tissue, was used in combination with MRI. Lastly, Simone et al. [Citation10] combined PET, CT, MRI, and X-ray to perform an open multivisceral resection surgery for advanced gastric adenocarcinoma.
3.1.2. Visually processed data
The primary focus of visually processed data is its semantic aspect, which pertains to the interpretation of information at a specific surgical stage. This semantic aspect may be anatomical, operational, or strategic. Anatomical semantics relate to the patient’s anatomy, physiology, or pathology, while operational semantics pertain to actions or tasks, and strategic semantics involve planning and guidance. The majority of MR IGS systems employ an anatomical semantic. In other words, they create visual representations of the patient’s anatomy to aid surgeons in locating and navigating toward a designated target.
A new use case that was not seen in the 2013 paper of an operational semantic comes from the use of telesurgery. Lu et al. [Citation11] developed a system that allows the videoconferencing in AR as well as the virtual presence of remote experts for real-time intraoperative guidance. Similarly, Sauer et al. [Citation12] described using the HoloLens for tele-consulting. The system enables a live stream from a surgeon’s HMD, allowing remote experts to view and communicate in real-time with the performing surgeon. The experts could also mark structures within the surgical site using a stylus on a tablet computer that could then be seen by the surgeon.
There are three subclasses of visually processed data: analyzed imaging data, prior knowledge data, and derived data. Analyzed imaging data refers to the data primitive used in an MR IGS system to display information, such as lines, contours, planes, surfaces, wire-frames/meshes, and volumes. Prior knowledge data includes information derived from generic models, such as atlases, prior measurements or plans, tool models, and uncertainty information specific to the IGS system. Derived data is information processed from patient-specific data or prior knowledge data and includes labels, and intraoperative measurements like distances between regions of interest (ROIs).
The majority of systems primarily focus on visualizing analyzed imaging data, and specifically pre-operative patient models obtained from MRI and CT scans. Specifically, most papers described visualizing the target structure (e.g. tumor, vertebrae, liver, etc.), relevant anatomy around the target structures, as well as risk structures (e.g. vascular risk structures, such as optic nerve or carotic arteries) as 3D rendered anatomical objects. A smaller number of papers used lines or contours to depict the margins of objects of interest. For example, researchers who used BrainLab’s Microscope Navigation with Leica ARveo (Brainlab, Munich, Germany) [Citation13–22] were presented with contours delineating anatomical structures of interest that are projected into the surgical microscope. Lastly, some papers used planar data in the form of axial, saggital, or coronal slices. In the works of Butler et al. [Citation23] and Bhatt et al. [Citation24] the FDA approved AR system for spine surgery, xvision-Spine (XVS) system (Augmedics, Ltd, Philadelphia, PA, USA) was used. In this system, not only can the 3D reconstruction of the spine elements be projected in the AR, but also axial and sagittal CT slices can be displayed in the visual field. Another example of planar data being used comes from the work of Borgmann et al. [Citation25] who used smart glasses (Google Glass) during surgery. Due to the limitations of this specific hardware, 3D anatomical models cannot be registered for guidance thus CT slices of the patient were displayed in the upper corner of the glasses ().
Figure 3. Different types of visualized data and processing. (A) CT slice image visualized in corner of Google Glass [Citation25]. (B) Contours and transparency used in BrainLab’s HUD showing lesion (yellow), the carotid arteries (blue), the chiasm (yellow), and the optic nerves (orange) [Citation26]. (C) Wireframe mesh rendering of uterus with tumor shown in yellow [Citation27]. (D) Surface rendering of spine, vasculature, and tumor anatomy as seen through the HoloLens [Citation28]. (E) Volume rendering of vessels in neurovascular surgery with colors representing venous/arterial flow [Citation29].
![Figure 3. Different types of visualized data and processing. (A) CT slice image visualized in corner of Google Glass [Citation25]. (B) Contours and transparency used in BrainLab’s HUD showing lesion (yellow), the carotid arteries (blue), the chiasm (yellow), and the optic nerves (orange) [Citation26]. (C) Wireframe mesh rendering of uterus with tumor shown in yellow [Citation27]. (D) Surface rendering of spine, vasculature, and tumor anatomy as seen through the HoloLens [Citation28]. (E) Volume rendering of vessels in neurovascular surgery with colors representing venous/arterial flow [Citation29].](/cms/asset/64132fbc-714a-4181-8222-ee54c172c0fa/icsu_a_2355897_f0003_c.jpg)
A few papers visualized prior knowledge data. For example, Pratt et al. [Citation30] alongside an AR overlay of the 3D anatomy of the muscles, bones, and joints of the leg, displayed color-coded arrows that are used to identify the subsurface location of vascular perforators, guiding the surgeon in extremity reconstruction surgery. A group from the Karolinska University Hospital (Stockholm, Sweden) who have been studying the clinical impact of AR in spine surgery, used AR to visualize prior knowledge data in the form of surgical plans [Citation31–34]. For example, in Refs. [Citation32,Citation33], the bone entry point identified before surgery is shown using AR for guidance during surgery. Using the same system, Burström et al. also determined the location of adhesive skin markers from video streams [Citation31]. The adhesive markers were highlighted in green, and a virtual reference grid was visualized, connecting the markers around the surgical field to allow the surgeon to understand patient tracking and registration.
A recent study by Lim et al. introduced a new type of data (surgical videos) not previously found in the 2013 review. The authors investigated the ergonomic benefits of using AR glasses vs. traditional monitors in video-assisted surgery. By projecting the laparoscopic video into AR glasses, they observed improved surgeon posture and reduced muscular fatigue compared to the video being displayed on a monitor during surgery.
There were only a couple of papers that reported the use of derived data. The works of Alphonso-Garcia et al. [Citation7] and Gorpas et al. [Citation6] used intraoperative measures of autofluorescence (using NIRF) to determine cancerous/necrotic or other tissue types.
3.2. Visualization processing
The second component of the DVV Taxonomy, Visualization Processing, describes how the data is transformed and visualized. The purpose of considering visualization processing is to emphasize the importance of determining the optimal methods for data transformation and visualization for specific surgical tasks or scenarios. Visualization processing entails determining whether data should be segmented and surface-rendered or volume-rendered, how it should be colored, and determining how, in an MR environment, it should be combined with the real world to allow for intuitive surgical guidance.
Consistent with the findings from 2013, the majority of papers used simple visualization processing techniques.
Surface-rendering with color-coding and/or transparency was the most common approach for rendering virtual objects. In the selected publications, as illustrated in , over half of the papers (54.4%) used surface-rendering and color-coding of anatomical data, while 22.8% mentioned surface-rendering without specifying color-coding. Only 5.1% used surface-rendering with both color coding and transparency. Interestingly, unlike the 2013 findings where approximately one-third of the publications did not detail the visualization methods, only 3.8% of the selected papers failed to specify (or it was not apparent from the included figures) some form of visualization processing. This suggests a growing recognition among researchers of the importance of thoughtful data visualization for providing effective guidance to the end-users.
Surface-rendering was the most prevalent method for rendering virtual data. Typically, researchers segment relevant anatomical data for the surgical procedure and apply color-coding to different anatomical objects for easy identification. For example, in Golse et al.’s work [Citation35] which describes an AR system for open liver surgery, the authors combine surface rendering of the liver (rendered khaki) with transparency to reveal the biliary tree and vessels (green and blue) within the liver.
One exemplary use of transparency comes from the studies that used the BrainLab’s Navigation platform with the Zeiss Microscopes HUD or Leica ARveo (Brainlab, Munich, Germany). Here semi-transparent 3D representations of pre-operative data, such as tumors, tractography, vertebrae are color-coded and projected into the surgeon’s view (see ). Studies that used this system include the work of Carl et al. [Citation17] who used the HUD of the Pentero operating microscope (Zeiss, Oberkochen, Germany) with the BrainLab system to project color-coded semi-transparent vertebrae (and occasionally spinal nerves) into the microscope view. The vertebrae could be either surface rendered, i.e. (3D mode) or displayed as contours (line-mode). In linemode, contours of the extent of the anatomy perpendicular to the viewing axis of the microscope in the focal plane are visualized and a dotted line indicates the maximum extent of the object beyond the focal plane. The same system and visualization processing were used by Carl et al. [Citation36] in transphenoidal surgery to visualize anatomical data, such as tumors, carotid arteries, optic nerves, and the chiasm. In similar work [Citation16], the authors highlighted the flexibility of the visualization, noting that the color of the object could be easily adjusted for better contrast if the background color begins to resemble the object’s color too closely.
A small subset of papers used wireframe/mesh rendering (see ) to visualize anatomical data. For example, in the study by Bourdel et al. [Citation37], which examined an AR system for gynecologic surgery to visualize adenomyomas, the authors employed mesh rendering. In this approach the virtual object is represented as a mesh rather than a surface-rendered object, allowing the viewers to perceive the real anatomy through the mesh. Similarly, Golse et al. [Citation35] used a virtual surface mesh overlaid on the liver in their work. The mesh is deformed based on a physics-based elastic model of the liver, to guide the surgeons during open liver surgery.
Despite the advancements in graphics hardware, only a small fraction of papers (<2%) used volume-rendering for visualizing the virtual objects. One example of volume rendering comes from Wierzbicki et al. [Citation38] who performed a study with eight patients using the HoloLens2 for guiding of microwave ablation in the context of gastrointestinal tumors. The authors provided holograms of volume rendered thoracic images to facilitate planning and serve as a decision support tool during the operation. They concluded that the 3D visualization of the volume rendered anatomy aided in tumor localization and planning the optimal access to the pathology.
3.3. View
The third component of the DVV taxonomy, View, encompasses three components: perception location, display, and interaction tools.
3.3.1. Perception location
Perception location pertains to the specific area within the user’s environment where the MR visualization is presented. categorizes the papers based on the perception location used in the system. Whereas the 2013 review found that 43% of papers identified the patient/surgical field as the perception location, the majority of the studies (63.3%) now used the patient/surgical field. This shift reflects a trend toward incorporating visual information directly into the surgical field through techniques, such as projecting data onto the patient using HMDs or integrating it within the microscope view.
Table 3. Categorization of the papers based on the settings used for data visualization.
In the 2013 review [Citation2], there were three papers (3.4%) that allowed for perception location to be both a display and/or the patient, our review identified a larger subset of papers (15.6%) that adopted a hybrid approach, utilizing both the surgical field and/or monitor. This combination has both the advantages of direct visualization within the surgical field while also having the benefits of external screen-based display systems visible to all personnel in the OR. Moreover, this configuration provides a high degree of flexibility in visualizing augmented information, enabling tailored presentations based on the unique demands of each surgical step or procedure.
While half of the papers in the 2013 review [Citation2], utilized monitors only, we observed that a minority of papers (11.4%) employed monitor displays. This method may facilitate collaboration and communication among the surgical team by providing a shared visualization during procedures. However, this approach necessitates diverting the surgeons’ attention away from the surgical field, causing focus shifts that may be detrimental to the surgical task [Citation39], specifically in cases where the surgeon is not navigating based on video feeds, such as in endoscopic or laparoscopic surgery.
It is worth noting in four publications [Citation10,Citation12,Citation25,Citation40], we identified perception location as the field of view. We use the term field of view (FOV) to characterize situations where images are not registered to the patient anatomy but rather are shown through smart glasses or an HMD (see ). In such cases, even if the surgeons shift their attention away from the surgical field, they can still view the images. This group accounted for (5.1%) of the total studies we have analyzed.
The remaining fraction of publications, accounting for (6.4%) in this study, opted for a more diversified approach by combining two or three different perception locations. For instance, Chengrun Li et al. [Citation41] used a combination of surgical field, FOV, and monitor as their perception location. This approach suggests a deliberate effort to integrate multiple visualization settings, allowing for greater flexibility in how visualized data is presented to the surgical team.
3.3.2. Display
Display refers to the specific hardware used to present the MR view to users, e.g. HMDs, monitors, microscopes, etc. (see ).
Figure 5. Examples of display types used for MR IGS. (A) Google Glass allows for the projection of simple images/text in the corner of the glasses [Citation25]. (B) The HoloLens HMD displays holograms of preoperative plans and anatomy in the surgical field of view in an abdominal cancer surgery case [Citation28]. (C) An iPad device used to show an AR view of the patient anatomy in neurosurgery [Citation42]. (D) The monitor of the neuronavigation system is used to show the AR view [Citation29]. (E) The surgical microscope’s HUD can be used to show the AR view in the oculars [Citation17].
![Figure 5. Examples of display types used for MR IGS. (A) Google Glass allows for the projection of simple images/text in the corner of the glasses [Citation25]. (B) The HoloLens HMD displays holograms of preoperative plans and anatomy in the surgical field of view in an abdominal cancer surgery case [Citation28]. (C) An iPad device used to show an AR view of the patient anatomy in neurosurgery [Citation42]. (D) The monitor of the neuronavigation system is used to show the AR view [Citation29]. (E) The surgical microscope’s HUD can be used to show the AR view in the oculars [Citation17].](/cms/asset/8a0cc695-1b0d-4f66-b1d2-7a1b6608e2a8/icsu_a_2355897_f0005_c.jpg)
The choice of display hardware significantly influences how users perceive and interact with the data, making it an important consideration. shows the frequency of each display type used to visualize data in the surveyed papers. As depicted in the figure, monitors, which account for 41.8% of the display technologies, emerge as the predominant display choice in IGS systems. This aligns with the findings of 2013 review, where monitors were the most popular choice of display with 47% of surveyed systems using them. External monitors include monitors that are part of an IGS system (e.g. [Citation14,Citation16–18,Citation26,Citation32,Citation36,Citation43]) or simply a monitor that streams videos from a microscope or camera (e.g. [Citation33,Citation35,Citation44–47]) or smartphones (e.g. [Citation9,Citation48–51]) and tablets [Citation52].
Head Mounted Displays (HMDs) comprised of 34.2% of the display technologies, making them the second most prevalent choice. Systems that incorporated HMDs provide surgeons with the advantage of accessing visualized data while maintaining their full concentration on the surgical field (e.g. ). It’s important to acknowledge that HMDs may not be suitable for microscopic, endoscopic, or exceptionally lengthy surgeries, as prolonged wearing of HMDs can lead to fatigue and discomfort.
In a small subset of papers (8.9%) microscopes were utilized as the display technology (e.g. ). The majority of these studies, totaling 71.5%, were focused on neurosurgery as the primary surgical domain. This has increased from the 2013 review where just over half (52%) of neurosurgery systems used the microscope for display. Furthermore, 1.3% utilized a combination of a microscope and monitor (e.g. [Citation53]). Using the microscope is an obvious choice in this domain due to the need for using a large magnification factor for surgery and accurate depth perception. Furthermore, using the microscope for MR requires no additional display device, has little to no additional cost, and can reduce disruption to the surgeon’s workflow.
Similar to the 2013 paper, there was a small portion of papers that used projectors. Out of the total 5.1% of the surveyed papers, 3.8% opted for projectors as the display technology for their IGS systems [Citation51,Citation54,Citation55] and 1.3% employed a combination of a monitor and projector [Citation51].
Despite the 2013 prediction that mobile devices, such as smartphones and tablets (e.g. ), would find their way into OR for testing, our review revealed a limited number of systems utilizing tablets (which we classify as monitor) as their display devices. Notably, Tang et al. [Citation52] and Guerriero et al. [Citation50] employed tablets for augmented guidance in their respective works. In addition to tablets, some research groups incorporated smartphones as mobile display devices [Citation48,Citation49,Citation56,Citation57].
3.3.3. Interaction tools
The DVV Taxonomy breaks down interaction tools into two types: virtual interaction tools, which describe how the user can manipulate the view and visualization of virtual objects, and hardware interaction tools (e.g. mouse, keyboard, pedal, tangible objects, etc.) that can be used to directly interact with the system. MR IGS systems typically allow surgeons to use gestures, voice, or other interaction methods to interact with the visualized data; surgeons can decide what to display or hide, adjust colors to provide better visualization, perform manual alignments, etc.
In line with the findings from 2013, where interaction methods and tools were infrequently described (mentioned in less than one-third of papers), our survey of current papers also reveals limited mention of interaction methods and tools. Moreover, apart from a few exceptions, the discussion on interaction tools primarily revolves around manual adjustments for image registration and visualization control. In the context of manual alignment of overlays to the surgical field, several papers allowed the surgeon to manually align or register the virtual elements with a patient’s actual anatomy to create the MR view [Citation42,Citation43,Citation49,Citation58]. For example, Schneider et al. described manual registration through two distinct interaction schemes: (1) a touch screen monitor and (2) a mouse [Citation59].
Some papers described allowing users to control the visualization of virtual models by adjusting parameters, such as transparency, color, and outlining. For instance, several studies highlighted the ability to interact with virtual objects by toggling the visibility of objects (e.g. [Citation15,Citation17,Citation18]), and modifying the colors to enhance contrast against the background, thereby enhancing the clarity of the guidance information during surgical procedures [Citation15].
Unlike the findings of the 2013 paper where the majority of systems that described interaction used keyboard or mouse as hardware due to the increasing use of tablets and HMDs, a number of the surveyed papers used natural user interfaces, e.g. voice and gestures. Gesture-based interactions, where hand movements are used to interact with virtual elements, were used to allow surgeons to precisely point to specific organs and manipulate 3D virtual models [Citation28]. This approach aims to enhance the surgeon’s ability to engage with the virtual elements during medical procedures and provides intuitive and sterile control over visualized data [Citation28]. Gestures can also be used to control the orientation of virtual objects or holograms (e.g. as in Ref. [Citation60]). In the work of Sherl et al. [Citation60,Citation61], the HoloLens based system enables the rotation of the holograms using either gestures to manipulate the objects directly or using menu buttons (that can be shown and hidden). Another interesting use of gestures for aligning images comes from the work of Saito et al. [Citation62]. In their work, several surgeons wearing HoloLens HMDs who are viewing the same hologram can move the hologram from their own perspective. For tablet devices or smartphones, gestures were also mentioned for zooming, rotating, changing transparency, turning on and off virtual elements, etc. [Citation42,Citation48,Citation50].
In the study by Buch et al. [Citation63] two approaches for interacting with a virtual model during surgery are presented: hand-gesture registration and controller registration. With hand-gesture registration, surgeons utilize the Microsoft HoloLens to intuitively adjust the model’s size, position, and orientation using hand gestures. The objective is to align virtual markers with real ones in the surgical field. In contrast, controller registration employed a customized HoloLens application in conjunction with a wireless Xbox gaming controller to enable translation, rotation, and magnification of the holographic model through button and joystick inputs. The authors found alignment was much more accurate with the controller-based registration (4.18 ± 2.83 mm) than with hand-gestures (20.2 ± 10.8 mm), and the registration accuracy improved over time, with the final patient achieving a registration error of 2.30 ± 0.58 mm.
In the work of Guerrera et al. [Citation64], the authors assessed the efficacy of Hyper Accuracy 3D (HA3D) reconstruction during a VATS anterior right upper lobe segmentectomy (S3). HA3D reconstruction is a technique used in medical imaging and computer vision to create highly precise three-dimensional representations of anatomical structures or objects. The HA3D reconstruction facilitated real-time guidance, allowing a proctor surgeon to interact with a HA3D virtual model via a dedicated laptop, guiding the procedure.
Voice commands were also mentioned in a few papers. Surgeons used voice commands to toggle virtual structures, including anatomical elements, providing real-time control over their AR environment [Citation12,Citation58]. This hands-free approach may enable intuitive and easy interaction while preserving the operator’s sterility.
3.4. Surgical domain
Our goal was to identify trends in the use of MR IGS in specific surgical domains between the 2013 paper [Citation2] and now. presents the comparative results across these two literature reviews. Whereas illustrates the percentage of each type of surgery included in this review. In the 2013 paper, the majority of papers described MR systems for neurosurgery, consistent with it being one of the first applications for image-guided or computer-assisted surgery. As mentioned by Shuhaiber [Citation65] this can be attributed to the complex and sensitive nature of neurosurgery where there is a need to minimize damage to eloquent areas of the brain. Neurosurgery accounts for 20.3% (16 papers) of the reviewed papers however of those it should be mentioned that five papers [Citation15,Citation16,Citation18,Citation45,Citation66] focused on spine neurosurgery, while the remainder, 11 out of 16 papers, focused on brain tumor surgery.
Figure 7. Type of surgery: comparison between the 2013 review [Citation2] and the current review for the count of papers for each type of surgery.
![Figure 7. Type of surgery: comparison between the 2013 review [Citation2] and the current review for the count of papers for each type of surgery.](/cms/asset/fd1aad9a-855a-4d78-ac87-0176c9bc5e29/icsu_a_2355897_f0007_c.jpg)
Figure 8. Surgical domains: the chart displays surgical domains using generic categories, with ‘spine surgery’ falling under ‘neurosurgery’ as a superclass.
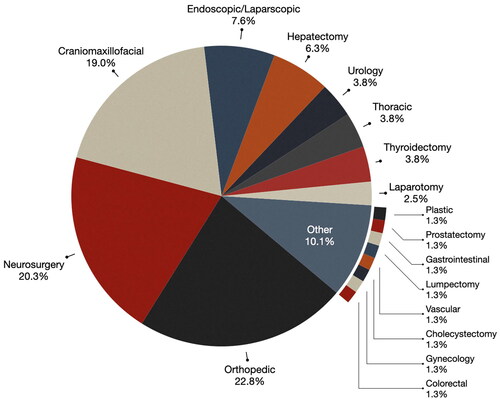
As can be seen from , orthopedic surgery has significantly increased in terms of testing in the OR, 5 papers published then and 18 now. This represents 22.8% of the reviewed publications, the most dominant domain in our review. IGS systems were used to improve the precision of implant placement and fracture reduction in orthopedic procedures. Notably, within this domain, 16 papers (88%) centered on spine surgery, while 2 papers [Citation30,Citation67] were in the domain of joint surgery.
Craniomaxillofacial surgery has also had an increase in MR IGS systems that have been brought into OR (from 12 papers to 15 papers). Craniomaxillofacial surgery emerged as the third most prevalent surgical domain, representing 19.0% of the reviewed publications. Within this domain, a notable subset of research (13.3%) was dedicated to the specific condition of craniosynostosis [Citation49], a congenital anomaly characterized by the premature fusion of cranial sutures that leads to abnormal skull shape and potential neurological complications.
Endoscopic and laparoscopic surgeries, where a telescopic rod lens with a video camera is used to guide surgical instruments to the patient anatomy through small incisions or keyholes, accounted for the fourth-highest proportion of studies, representing 7.6% of the total [Citation21,Citation22,Citation35,Citation36,Citation59,Citation68,Citation69]. In terms of count, however, this is a reduction from the 2013 review where 14 papers discussed MR systems for endoscopic surgery, whereas in our selected publications only 6 did. Endoscopic and laparoscopic surgery was used in the context of liver [Citation35,Citation59,Citation68], skull-based [Citation21,Citation22], transphenoidal [Citation36], and sinus surgery [Citation22].
Hepatectomy (liver) procedures [Citation12,Citation35,Citation52,Citation62,Citation70] represented 6.3% of papers published in this area and were consistent in terms of count from the 2013 review (6 papers then and 5 now).
In addition to the aforementioned surgical domains, MR in IGS found application in other surgical domains, urology [Citation25,Citation71], thoracic [Citation41,Citation54,Citation64], thyroidectomy [Citation40,Citation53,Citation72], and laparotomy [Citation10,Citation28], collectively constituting 13.9% of the reviewed publications. For example, in liver surgery, MR guidance was used to identify and navigate around critical anatomical structures, including blood vessels and bile ducts [Citation70]. In vascular surgery, MR was used to guide catheterization procedures and optimize stent placement for improved clinical outcomes [Citation14].
Approximately 10.1% of the studies encompassed examined MR use in other surgical specialties, including plastic surgery for breast [Citation54], colorectal surgery, gynecology [Citation37], lumpectomy for breast [Citation73], gastrointestinal surgery [Citation38], prostatectomy [Citation74], vascular surgery [Citation14], and cholecystectomy (i.e. gallbladder removal) [Citation75]. Within these surgical domains, MR was studied for such tasks as tumor localization, precise resection of lymph nodes, and the identification of critical anatomical structures.
Lastly, while cardiac surgeries accounted for 7% of IGS systems in the 2013 review [Citation2], we did not find any recent papers introducing MR IGS systems in this domain. This indicates a shift away from MR adoption in cardiac surgery, possibly due to the complexity of these procedures.
4. Validation and evaluation
In the following section, we describe the validation and evaluation of the MR IGS systems in the selected papers. We begin by giving an overview of the number of surgical cases per medical specialty and then for the most comprehensive clinical studies, we describe the qualitative and quantitative criteria that were used to evaluate the described systems.
4.1. Surgical cases
provides a summary of the number of studies and number of surgical cases, i.e. patients, investigated for each type of surgery. As can be seen from the table, orthopedic surgery emerged as the domain with the most extensive MR research, with a total of 18 clinical studies. These studies collectively encompassed 440 surgical cases, constituting 27.6% of the total cases analyzed in our review.
Table 4. The number of cases studied across various types of surgeries (other includes plastic, hepatectomy, gastrointestinal, gynecology, colorectal, lumpectomy).
Neurosurgery was the primary focus of 16 research studies which collectively examined 369 surgical cases, accounting for 23.2% of the total cases included in the analysis. The substantial number of studies reflects the significance of image-guided procedures in the context of neurosurgery.
Endoscopic and laparoscopic surgeries were the focus of six research studies, involving a cumulative total of 340 surgical cases, which accounted for 21.4% of the overall surgical cases analyzed. These studies were designed to explore the prospective applications of MR within minimally invasive procedures.
Thoracoscopy, a surgical technique for examining and treating the chest cavity, was the focus of three research studies. These investigations collectively examined 145 surgical cases, accounting for 9.1% of the total cases in the analysis. Maxillofacial surgeries, encompassing craniofacial and dental procedures, accounted for 15 distinct research studies with 77 surgical cases, representing 4.8% of the total cases considered in our analysis. Lastly, the application of MR in IGS in additional surgical domains, such as vascular surgery, liver surgery, thyroidectomy, urology, and prostatectomy, were explored in a limited number of studies. These domains represented a smaller proportion of cases, ranging from 1.1 to 3.6% of the total.
4.2. Large clinical studies
In this section, we focus on the papers that tested an MR system in 15 or more surgical cases. In , we reference each of the papers, their clinical context, the number of surgical cases that were performed, and the evaluation criteria that were used.
Table 5. Included studies: evaluated or validated components (≥15 patients).
4.2.1. Orthopedic surgery
Butler et al. [Citation23] conducted a prospective study involving 164 varying minimally invasive spine surgery cases that involved the placement of 606 pedicle screws. They assessed the time for percutaneous screw placement in surgical procedures and found an average time of 3 min and 54 s per screw and that the learning curve did not significantly impact surgical times. The study also found a low rate of intra-operative screw replacements (0.49%) and the absence of postoperative complications, such as radiculopathy or neurologic deficit.
Aoyama et al. [Citation76] conducted a study involving 49 patients to evaluate the use of AR in spine surgery. Their research focused on the accuracy of identifying the level of the spinous process during surgery, both with and without the use of an AR HMD. The results of the study showed that without an HMD, the misidentification rate of the level was significantly higher (26.5%) then when AR was used to overlay holographic guidance information onto the patient (14.3%). The study also showed that in cases where misidentification occurred without an HMD, the addition of an HMD assessment helped accurately reconfirm the level in nearly half of the cases (46.2%). Furthermore, the misidentification rate tended to be higher in the thoracolumbar spine, but the use of an HMD significantly reduced the range of misidentification to within one vertebral body.
Edström et al. [Citation33] conducted a comparative study involving a total of 44 cases, where 15 deformity cases were treated using AR surgical navigation (ARSN), while 29 cases were treated using a conventional free-hand (FH) approach. All surgeries were performed by the same orthopedic spine surgeon. The study evaluated several key outcomes, with a primary focus on implant density, including pedicle screw, hook, and combined implant density. The study also considered several secondary outcomes, including procedure time, deformity correction effectiveness, length of hospital stay, and blood loss. The authors found that both ARSN and FH groups had overall high-density construct (>80% total implant density) but that the ARSN group had a significantly higher pedicle screw density (86.3 vs. 74.7%, p < 0.05). The procedure time and deformity correction were not significantly different between groups.
In another study of the ARSN system [Citation32], researchers used a hybrid OR to facilitate real-time adjustments by comparing actual screw placement with pre-planned trajectories using AR overlays. The authors found that the ARSN system minimized radiation exposure by reducing the need for continuous fluoroscopy. Although initial surgical times increased due to additional imaging and planning—making up ∼8% of the total surgery time—these steps significantly enhanced overall surgical efficiency, thereby reducing the risk of complications and the need for revision surgeries. Further, with workflow optimizations, the researchers were able to significantly reduce the median total OR time from 84 to 46 min per level.
The ARSN study was also evaluated in the work of Elmi-Terander et al. [Citation34], where it was compared to the FH technique in patients with scoliosis, kyphosis, and other conditions. Each group comprised 20 patients, who were well-matched based on clinical diagnosis and the proportion of screws placed in the thoracic vs. lumbosacral spine. The ARSN group demonstrated a significantly higher share of clinically accurate screws (93.9 vs. 89.6%, p < 0.05) and twice as many screws placed without a cortical breach (63.4 vs. 30.6%, p < 0.0001) compared to the FH group.
In another pedicle screw placement study conducted by Bhatt et al. [Citation24], the effectiveness of HMD AR was tested in 32 patients with a total of 222 screws. Intraoperative 3D imaging was employed to assess screw accuracy, utilizing the Gertzbein-Robbins (G-R) grading scale. Only four screws (1.8%) were deemed misplaced and required revision, while the remaining 218 screws (98.2%) were placed accurately. More specifically, out of the 208 AR-placed screws with 3D imaging confirmation, 97.1% were considered clinically accurate, with 91.8% classified as Grade A and 5.3% as Grade B. Furthermore, there were no intraoperative adverse events or complications, and the AR technology was not abandoned in any case. Additionally, the study reported no early postoperative surgical complications or need for revision surgeries during the 2-week follow-up period.
In a study by Guo et al. [Citation55], which retrospectively reviewed 21 patients with scapular fractures, the authors compared two groups: Group I (nine patients) who underwent conventional fixation and Group II (12 patients) who had pre-operative virtual simulation and intra-operative navigation-assisted fixation using an AR-scapular system. In Group II, the pre-operative virtual simulation took an average of 44.42 min, with 16.08 min required for plate contouring. Group II showed significantly shorter operation times and reduced blood loss (28.75 min and 81.94 ml less, respectively; p < 0.05) compared to Group I. The number of plates used was slightly lower in Group II, but not statistically significant and the functional outcomes at follow-up were not statistically significant between the two groups. No complications were observed for any patients. These findings suggest that AR in scapular fracture surgery can lead to shorter surgery times, reduced blood loss, and similar functional outcomes compared to conventional techniques.
In a study by Burström et al. [Citation31] that involved four cadavers and 20 patients who underwent spinal surgery with pedicle screw placement, AR technology was used to identify the bone entry point. The study included a total of 366 pedicle devices. The overall mean technical accuracy for pedicle screw placement was 1.65 ± 1.24 mm at the bone entry point. Notably, the technical accuracy for the cadaveric study (n = 113) was 0.94 ± 0.59 mm, while the clinical study (n = 253) reported slightly lower accuracy at 1.97 ± 1.33 mm. An analysis of 253 pedicle screws across 35 CBCTs found no statistically significant differences between relative CBCT position and technical accuracy. Furthermore, a sub-analysis of all CBCTs specifically involving the thoracolumbar junction, where breathing might theoretically affect accuracy, revealed no difference in accuracy between lumbar or thoracic levels (p = 0.22).
Lastly, in a prospective case–control study conducted by Hu et al. [Citation79], a total of 18 patients were investigated, with nine patients in the AR computer-assisted spine surgery (ARCASS) group having 11 levels of lesions, and the control group consisting of nine patients with 10 levels of lesions. The ARCASS group had significantly reduced fluoroscopy usage (6 vs. 18, p < 0.001) and shorter operative times (78 vs. 205 s, p < 0.001) during the process of entry point identification and local anesthesia. Moreover, the ARCASS group achieved superior accuracy, with a significantly higher proportion of ‘good’ entry points compared to the control group in both lateral (81.8 vs. 30.0%, p = 0.028) and anteroposterior views (72.7 vs. 20.0%, p = 0.020).
These studies combined show that AR technology offers significant benefits compared to freehand techniques in the context of pedicle screw placement. AR improves the efficiency of pedicle screw placement in minimally invasive spine surgery, reducing the average time per screw and resulting in lower complication rates. It has high accuracy, irrespective of the spinal level, and has been shown to outperform traditional free-hand surgery in terms of implant density. Moreover, AR-guided procedures were found to be safe, with minimal screw misplacements and no complications. The results of the scapular fracture surgery showed that AR-assisted techniques have the potential to lead to shorter operation times and reduced blood loss while maintaining similar functional outcomes compared to conventional methods. These findings suggest that AR has the potential to enhance precision and efficiency in a range of orthopedic surgical procedures.
4.2.2. Neurosurgery
Liu et al. [Citation9] conducted a study with pre-operative planning using slicer and AR guidance using a smartphone in 77 brain glioma cases. They assessed various surgical indicators between the experimental group and control group, including total resection rates, hospital stay after surgery, surgery time, and patient’s quality of life (QOL) using the Karnofsky performance scale (KPS). The results of their study showed that the number of cases with total resection, hospital stay after surgery, and surgery time were significantly better in the smartphone group compared to the control group. They also found that after treatment, the KPS score was significantly higher in the experimental group (75.66 ± 4.01 vs. 65.3 ± 5.23). The results suggest that preoperative planning in 3D Slicer combined with intraoperative smartphone guidance can enhance the precision of brain glioma surgery, facilitating total resection while safeguarding patients’ neural function.
In another brain tumor study conducted by Kim et al. [Citation19], the potential benefits of using an AR system were investigated in 34 cases. The study was a single-arm study without a control group and did not include statistical analysis or specific outcome measures. However, the results suggested that the integration of an AR system is beneficial to neurosurgeons, particularly those who may have less experience, and that AR visualization can improve workflows by providing intuitive guidance information.
The work by Incekara et al. presents a tumor study that included 25 patients. Here, the authors compared the localization of the tumor using the HoloLens with the standard neuronavigation system. They found that in 40% of cases, the tumors were localized as with the neuronavigation system and that on average the deviation in localization of the HoloLens to the actual tumor location was 0.4 cm. The average time for preoperative planning using the Hololens (5 min 20 s) was found to be significantly longer than using the standard neuronavigation system (4 min 25 s); however, the authors reported improved ergonomics and focus when using the HoloLens.
Goto et al. [Citation44] studied the efficacy of an AR navigation system in a cohort of 15 consecutive patients with sellar and parasellar tumors. The AR navigation system demonstrated significant utility in enhancing the immediate three-dimensional understanding of the lesions and surrounding structures. Using an original five-point scale, two neurosurgeons and three senior residents who participated in the endoscopic skull base surgeries assessed the AR navigation system as highly effective in 5 out of the 15 cases and with a score of 4 or higher in 13 cases. The average score across all cases was 4.7 (95% confidence interval: 4.58–4.82), signifying that the AR navigation system was either as useful as or even more beneficial than conventional navigation, with only two cases presenting slight challenges in depth perception.
Bopp et al. [Citation26] report on a single surgeon series of 165 transsphenoidal surgeries, with 81 patients undergoing surgery without AR and 84 patients with AR. iCT-based registration was used and found to significantly improve the accuracy of AR (measured by target registration error, TRE) to 0.76 ± 0.33 mm, compared to the landmark-based approach with a TRE of 1.85 ± 1.02 mm. In terms of surgical duration, the time was not significantly different across the two groups; however, the time required for the patient registration increased by ∼12 min (32.33 ± 13.35 vs. 44.13 ± 13.67 min). This was deemed reasonable, given that AR was felt to enhanced surgical orientation, increased surgeon comfort, and improved patient safety. These benefits were observed not only in patients with prior transsphenoidal surgery but also in cases involving anatomical variations.
Carl et al. [Citation16] conducted a study involving 42 neuro-spinal procedures, including 12 intra- and eight extradural tumors, seven other intradural lesions, 11 degenerative cases, two infections, and two deformities. In these procedures, the Zeiss Brain Lab HUD AR system was used. The authors looked at registration error, surgical workflow, AR visualization, and radiation. The results of their studies showed that AR could be easily integrated into a normal surgical workflow procedure and that the visualization of anatomical structures overlaid on the surgical field allowed for intuitive guidance. They also reported a low mean registration error (≈1 mm) and a reduced radiation dose for iCT procedures of about 70%.
In another study by Carl et al. [Citation14], involving 20 patients and 22 aneurysms, the authors used an ICG-AR (Indocyanine Green Angiography AR) with the Kinevo900 operating microscope. ICG-AR improved surgical manipulation and blood flow interpretation during ICG angiography. Surgeons could view ICG-AR information directly through the microscope oculars in white light, eliminating the need for separate screens. AR visualization enhanced the understanding of 3D vessel anatomy for aneurysm clipping. In addition to reporting TRE (0.71 ± 0.21 mm) and the intraoperative low-dose CT effective dose (42.7 µSv), the authors reported the number of times adjustments were made to compensate for brain shift and to create a more accurate AR view. Linear and rotational adjustments (in 2D) were done in 18 of 20 patients and applied 28 times (translation, n = 28; range = 1–8.5 mm; rotational adjustment, n = 3; range = 2.9–14.4°). Although this generated a more accurate AR overly it could not compensate for 3D deformations in depth. Lastly, the authors also reported that surgical complications occurred in two cases (10%) which is a similar rate when compared to traditional ICG video angiography. In one case, ICG-AR revealed an incomplete aneurysm occlusion, leading to the placement of an additional clip. In another case, ICG-AR identified insufficient flow in a vessel branch, resulting in clip repositioning.
Rychen et al. [Citation20] also studied AR guidance for neurovascular procedures in a study of 18 consecutive revascularization procedures in a cohort of 15 patients. The procedures encompassed various pathologies, including Moyamoya disease/syndrome, intracranial vascular occlusion, extracranial internal carotid occlusion, and middle cerebral artery (MCA) aneurysm. AR technology proved to be a valuable tool throughout the surgery, significantly enhancing safety and efficacy during specific surgical steps, including the microsurgical dissection of the donor vessel (STA), tailoring the craniotomy above the recipient vessel (M4 branch of the MCA), craniotomy adjustments to spare the middle meningeal artery (MMA), and modifications to spare the precentral artery (PMC).
As can be seen from the described studies, MR technologies have shown promise in improving surgical precision, reducing registration errors, and enhancing patient outcomes across a range of neurosurgical procedures. The studies further emphasize the potential for MR to augment surgical workflows, improve visualization, and contribute to better surgical results.
4.2.3. Endoscopic/laparoscopic
Zeiger et al. [Citation22] conducted a study involving 134 cases with various pathological conditions, including anterior skull base tumors, inflammatory sinus disease, and cerebrospinal fluid leaks. Preoperative imaging data were used to generate 3D models of patient anatomy that were used in surgical planning and allowed surgeons to anticipate critical structures during endoscopic procedures using Brainlab’s system. The authors reported that surgeons found the technology helpful in guiding bony dissection and identifying important anatomical landmarks, such as the internal carotid arteries and optic nerves.
Linxweiler et al. [Citation69] conducted a comprehensive study involving 100 patients with chronic sinus disease, comparing conventional navigation software with AR navigation. Their results demonstrated no significant difference in operating time between the two systems, indicating the feasibility of AR integration without compromising efficiency. The study also highlighted the importance of surgeon experience, as operations by senior physicians were notably shorter. All surgeons, however, reported high accuracy levels with AR-based guidance.
Carl et al. [Citation36] conducted a consecutive single-surgeon series involving 288 transphenoidal procedures. In this extensive series, 47 patients (16.3%) underwent microscope-based AR to visualize the target and risk structures during the surgical interventions. The accuracy of the AR depended on navigation precision and microscope calibration. Patients with fiducial-based registration had a target registration error of 2.33 ± 1.30 mm. Automatic iCT-based registration, which is user-independent, significantly improved AR accuracy (TRE = 0.83 ± 0.44 mm, p < 0.001). Furthermore, the use of low-dose iCT protocols reduced the effective dose from iCT registration scanning, and no vascular injuries or worsened neurological deficits were observed in the series. AR greatly enhanced orientation during reoperations and in patients with anatomical variations, contributed to increased surgeon comfort and better surgical workflows. The authors further mentioned that AR visualizations improved 3D perception compared to the standard display of dashed lines via the HUD of the operating microscope.
In a study led by Schneider et al. [Citation59], an AR IGS system, SmartLiver, was used in Laparoscopic Liver Resection (LLR). In this study involving 18 patients, seven underwent LLR, and 11 underwent conventional staging laparoscopy. The primary goal of the study was to achieve successful registration as determined by the operating surgeon, and secondary objectives included assessing the system’s usability through a surgeon questionnaire and comparing the accuracy of manual vs. semi-automatic registration. In 16 out of 18 patients, the primary endpoint was successfully met after overcoming an initial issue related to distinguishing the liver surface from surrounding structures, thanks to the implementation of a deep learning algorithm. The mean registration accuracy was 10.9 ± 4.2 mm for manual registration and 13.9 ± 4.4 mm for semi-automatic registration, with positive feedback from surgeons regarding the system’s handling and improved intraoperative orientation. However, there were suggestions for simplifying the setup process and enhancing integration with laparoscopic ultrasound. The study did not assess the impact of SmartLiver on perioperative outcomes as it is still in development.
The results of these studies demonstrated the feasibility of integrating MR into laparoscopic and endoscopic surgery for various procedures. Initial results show no significant impact on operation time and underscore the importance of surgeon experience and the potential improvement in accuracy that can achieved with MR systems.
4.2.4. Cholecystectomy
Diana et al. [Citation75] performed a study with 58 patients undergoing cholecystectomy for gallbladder lithiasis. In their study, planning was performed on VR models and AR was used. Furthermore, indocyanine green was injected intravenously before and after Calot triangle dissection. The authors found that the use of 3D VR planning successfully identified 12 anatomical variants in eight patients, with only seven of these variants being accurately reported by the radiologists, indicating a statistically significant difference (p = 0.037). Moreover, VR allowed for the detection of a hazardous variant, which prompted the use of a ‘fundus first’ approach. The visualization of the cystic-common bile duct junction was achieved before Calot triangle dissection in 100% of cases with VR, in 98.15% with NIR-C, and in 96.15% with IOC. Additionally, the duration for obtaining relevant images was significantly reduced with NIR-C compared to VR (p = 0.008) and IOC (p < 10−7). Lastly, the quality of the images was assessed using a 5-point Likert scale, revealing that NIR-C scored comparatively lower when compared to AR (p = 0.018) and IOC (p < 0.0001). These findings demonstrate the use of multimodal imaging with MR for precise cholecystectomy, highlighting the potential of these visualization techniques to compliment each other and mitigate the risk of biliary injuries.
4.2.5. Thoracic surgery
Li et al. [Citation41] investigated the application of AR in thoracoscopic segmentectomy or subsegmentectomy for early lung cancers, in a study involving 142 patients. The study compared using 3D lung models either displayed on screen (n = 87) or printed out and displayed using AR (n = 55) during surgery in OR. The surgical outcome data, both before and after propensity score matching, demonstrated that the utilization of 3D printing with AR resulted in a reduced duration of surgery (p = 0.001 and 0.001, respectively), decreased intraoperative blood loss (=0.024 and 0.006, respectively), and a shorter hospital stay (p = 0.001 and 0.001, respectively) compared to the on-screen group. However, there were no significant differences in complications and the success rate of the procedure (p = 0.846 and >0.999, respectively) and (p = 0.567 and >0.999, respectively). Furthermore, surgeons assigned higher scores to the tangible group compared to the on-screen group (p = 0.001 vs. 0.001, respectively). This research highlights the feasibility and potential benefits of AR with 3D printing in improving surgical visualization and precision.
4.2.6. Urology
Borgmann et al. [Citation25] conducted a study involving seven urological surgeons, consisting of three board-certified urologists and four urology residents. In the study, AR technology was used in 10 different types of surgeries and a total of 31 urological procedures. The feasibility of AR-assisted surgery was assessed using technical metadata, which included the number of photographs taken, the number of videos recorded, and the total video time recorded during the procedures. Furthermore, structured interviews were conducted with the participating urologists to gather insights into their use of surgical guidance. Complications were also recorded and graded according to the Clavien–Dindo classification. No specific complications were observed during the surgical procedures. However, during the postoperative course, a total of 6 complications occurred among the series of 31 urological surgeries of varying complexity, ranging from simple procedures, such as vasectomy to complex ones like cystectomy. Grade I complications included two urinary tract infections (after TURB and prostate adenomectomy) and one superficial wound infection (after prostate adenomectomy). Grade II complications comprised two cases of ileus (after open cystectomy) and one case of anastomotic leak (after laparoscopic radical prostatectomy). Importantly, all surgeons reported that they did not experience relevant distraction while using AR surgical guidance during surgery. This research underscores the feasibility of implementing AR in urological surgery across a range of procedures.
4.2.7. Prostatectomy
In a study by Porpiglia et al. [Citation74], 40 patients underwent prostate cancer procedures. Twenty patients underwent 3D Elastic AR Robotic Assisted Radical Prostatectomy (3D AR-RARP), whereas the control group had the traditional 2D Cognitive procedure (2D Cognitive RARP). In both approaches, 3D AR and 2D Cognitive, capsular involvement (CI) was accurately identified in preoperative imaging, and microscopic assessment confirmed cancer presence in suspicious areas identified by both methods. The 3D AR group showed more accurate CI identification (100%) than the 2D Cognitive group (47.0%). Furthermore, there was a statistically significant advantage for the 3D AR Group in detecting cancerous lesions during the nerve-sparing (NS) phase. Despite the need for manual image segmentation and alignment, which the authors mentioned as a shortcoming of the system, the authors concluded that the introduction of elastic 3D virtual models effectively simulates prostate deformation during surgery, leading to accurate lesion localization, potentially reducing the positive surgical margin rate, and simultaneously optimizing functional outcomes.
4.2.8. Craniomaxillofacial
The study by Zhu et al. [Citation78] introduced an AR system to visualize Inferior Alveolar Nerves (IANs) in 20 patients undergoing craniomaxillofacial surgery. The research focused on assessing the system’s accuracy and registration accuracy across the 20 cases was found to be 0.96 ± 0.51 mm. The authors further concluded that maxillofacial surgery using AR offers the benefits of user-friendly operation and precision, ultimately enhancing the overall surgical performance.
5. Challenges and limitations
The application of MR IGS systems has shown great potential, however, there continue to be some challenges and limitations that were mentioned in the surveyed papers.
First, the integration of MR IGS systems introduces a learning curve for surgeons, which, while not necessarily affecting surgical times, emphasizes the importance of training and familiarity. Multiple studies have shown that early cases and those performed by experienced surgeons have comparable surgical times, indicating a minimal impact on surgical efficiency [Citation23]. In cranial neurosurgery, experienced teams reported no learning curve due to prior experience with microscope-based AR support [Citation15]. However, the significance of the learning curve in spine surgery, particularly for resident education, remains to be determined [Citation16]. Notably, the low adoption of manual or robotic computer navigation in spine surgeries is often attributed to steep adoption learning curves [Citation80].
In terms of display, while HMDs are a promising tool for MR-assisted surgery, the HoloLens and similar devices have notable limitations. The low resolution of these devices is sometimes detrimental to the quality and fidelity of the 3D images they render, potentially impeding the precision required in surgical settings. Moreover, the restricted 30° horizontal field of view can hinder the user’s overall experience by limiting their spatial awareness [Citation11]. As well as the limited field of view, the camera performance, limitations in magnification, and light source issues can also negatively impact the ability to capture deep surgical fields accurately [Citation47,Citation61]. Furthermore, it is important to note that the rechargeable battery, which typically supports 2–3 h of active use, poses an additional limitation, especially during longer surgical procedures [Citation61]. Lastly, the prolonged usage of glasses may lead to discomfort and even dizziness, raising concerns about their practicality during extended surgical procedures [Citation24,Citation40]. Finally, as demonstrated by Condino et al. [Citation81] in a laboratory study, current optical see-through HMDs suffer from technological limitations that reduce their suitability for surgical applications. Specifically, their incorrect focus cues at short range can negatively impact localization accuracy, which is paramount in surgical applications.
In the work of Cecceraglia et al. [Citation58] the authors mentioned several specific challenges based on their experiences using the HoloLens. These included that when the surgeon is operating with an assistant the virtual image or hologram can appear at a different spatial position in each of their views (even after manual registration by the surgeon), furthermore OR lights can affect the quality of holograms and the overlap of the hologram on the surgical field can obstruct the view of the surgical site and anatomical structures. This last point was also mentioned in the work of Bopp et al. [Citation26] who wrote that the large amount of AR information visualized within the microscopic view of the system they tested can conceal the clear view of the surgical field. Tang et al. [Citation52] who used an iPad for AR also mentioned that the AR view can cover portions of the surgical field which may not be desired. Furthermore, the line of sight of the intraoperative camera in their studies was sometimes obstructed by the surgeon’s hands or surgical instruments, which consequently reduced the accuracy of the overlaid 3D images.
Although not specific to MR systems, challenges related to soft tissue deformation, organ motion, and the absence of fixed bony landmarks were mentioned as complicating the accuracy of MR IGS systems [Citation56,Citation59]. Furthermore, numerous papers mentioned issues in terms of workflow, either pre-operatively, including the time to create virtual models and set-up systems (e.g. [Citation44,Citation56,Citation71]) as well as introspectively the time needed for registration. For example, Sasaki et al. [Citation82] mention that the time needed to register the 3D virtual image to the surgical field (which they do manually) is complicated and causes an interruption in the surgical process whenever it has to be done. In some cases, also the need for postoperative registration and retrospective registration arises due to intraoperative difficulties [Citation59]. Indeed, registration accuracy was mentioned by numerous groups as a limitation of their systems (e.g. [Citation11,Citation28]).
Despite the increase in the number of clinical studies, the study design for accessing MR IGS systems for surgical procedures has had some limitations, including the use of retrospective not prospective studies, small sample sizes, and the absence of control groups [Citation17,Citation33,Citation34,Citation55,Citation56]. Measuring the specific benefits of MR and addressing potential bias in subjective evaluations during randomized trials are also challenging aspects. These limitations highlight the need for more robust and standardized clinical study designs to assess MR IGS systems’ impact on surgical outcomes.
In terms of cost, the initial investment necessary for acquiring and maintaining MR systems can also limit their accessibility, especially for healthcare institutions with budget constraints [Citation46,Citation61,Citation72,Citation83,Citation84]. Furthermore, the involvement of a technology team member to operate and maintain these can increase operational costs and may lead to difficulties in finding qualified staff to manage the technology effectively. Despite, some efforts toward low cost systems, these too can come with limitations. For example, Yodrabum et al. [Citation51] mentioned some disadvantages of their low-cost AR approaches including distortion of the image, and the need for an assistant to hold the mobile device still and fixed at an appropriate distance and angle to capture the surgical field. This led to the surgeon’s movement being limited and needing to move between the patient and the device to take advantage of the augmented view.
6. Discussion
In this section, we present key highlights and observations drawn from the reviewed literature concerning the applications of MR in image-guided procedures.
6.1. Data
In terms of raw imaging data, as expected, most systems rely on CT or MR imaging data. Surprisingly, despite the increased use of ultrasound in the clinical domain, we found no systems that integrated ultrasound with MR. However, with the recent increase in research combining MR technologies with ultrasound, such as aiding in ultrasound acquisition (e.g. [Citation85]), facilitating ultrasound learning and interpretation [Citation86], and re-registering intraoperative images to account for factors like brain shift [Citation87]), we anticipate that ultrasound will increasingly be used with MR in IGS systems.
When it comes to visualized data, almost all systems leverage 3D visual representations of the patient’s anatomy to assist surgeons. Surprisingly, few papers discussed visualizing prior knowledge data, such as atlases, and there were limited novel uses or representations in terms of data. Despite, the limited use of prior knowledge and derived data in the survey papers, we posit that in the future more sensors will be integrated into ORs and more AI models will be used resulting in new types of preoperative and intraoperative measurements. Thus, we anticipate the visualization of uncertainties inherent in AI models or guidance information, as well as the quantification of intraoperative distances and residual tumor volumes in AR views.
6.2. Visualization processing
Our review highlights that most research papers still tend to favor conventional visualization techniques, primarily relying on surface-rendering. This approach is often complemented with color-coding and transparency to facilitate the distinction of anatomical objects. However, we have also noticed a growing awareness among researchers regarding the importance of thoughtful data visualization for enhancing the effectiveness of surgical guidance. Of note are instances where AR seamlessly integrates with visualization processing, as seen in cases involving BrainLab’s Microscope Navigation and Leica ARveo. These instances provide a promising glimpse into the future of research in this domain. Although volume rendering remains underutilized, its potential benefits, like tumor localization and surgical planning, are evident in a study conducted by Wierzbicki et al. [Citation38]. Continuous exploration and development in visualization processing are important to maximize the potential of MR in surgery.
6.3. View
In terms of displays, the increasing popularity of HMDs in recent years can largely be attributed to advancements in ergonomics and ease of interaction. These devices offer significant advantages as they can be comfortably adjusted in front of both eyes, providing an immersive experience. Additionally, modern HMD models are lighter than their predecessors, addressing concerns related to physical fatigue among users.
Tablets and smartphones were not often used in the surveyed papers, however, we believe the promising results of those that were tested in the OR [Citation48–50,Citation52,Citation56,Citation57] will encourage more groups to look at these low cost solutions. Léger et al. [Citation42], for example, provide an open source mobile AR interactive neuronavigation system (MARIN) that performs real-time AR on a mobile device in the context of image-guided neurosurgery and may pave the way for others to bring similar systems for testing in the OR.
Despite, the increasing popularity of HMDs, it is important to note that the landscape of displays in the surgical domain remains diverse. Various display devices are adapted to meet the unique requirements of different surgical domains. When choosing specific devices for a specific surgical procedure, it becomes evident that there is no one-size-fits-all solution for IGS. To make the appropriate choice for the display, careful consideration of factors, such as the surgical field, specific tasks, surgeon workflows, and the OR environment is essential.
In terms of interaction devices, the landscape is slowly shifting away from traditional interaction tools like keyboards and mice and increasingly adopting more advanced and diverse methods, including touch screens, hand gestures, and voice commands that are more commonly used with hardware, such as tablets and HMDs.
6.4. Surgical domain
We found that although many trends were similar between the 2013 state-of-the-art and our analysis, there were some new findings. For example, although the frequency of systems used for specific surgeries was similar in 2013 in terms of the most popular surgical domains, several new domains where MR was tested (e.g. thoracic surgery, urology, etc.) emerged, underscoring the expanding utilization of MR across a spectrum of surgical domains. Furthermore, increases in papers and specifically clinical studies testing MR indicate a growing adoption of MR technologies in clinical practice. Large studies in neurosurgery and spine surgery can partially be attributed to two FDA approved commercial systems for MR technology, BrainLab’s Microscope Navigation with Leica ARveo or Zeiss Pentero and Augmedics xvision system.
6.5. General
One significant aspect that has gained attention over the years is the cost of implementing MR systems in clinical settings. Researchers and practitioners are increasingly exploring low-cost solutions to make these technologies more accessible and affordable. This shift in focus toward cost-effective solutions demonstrates the growing awareness and interest in democratizing the use of these technologies for a wider range of surgical applications and settings. The affordability of hardware, coupled with cloud-based solutions and intelligent image processing software, is likely to drive the expansion of MR applications in a broad spectrum of surgical domains [Citation54].
In terms of democratizing high quality clinical care, one aspect that was mentioned by Balci et al. [Citation68], was that emerging MR technologies will empower less experienced surgical teams to narrow the disparity with their more experienced counterparts, enhancing planning precision and procedure safety, ultimately reducing the likelihood of complications arising from challenging anatomical variations and allowing the team to operate within specified error margins.
Despite the progress made, several challenges persist in the field of MR for image-guided procedures. These challenges include the need for improved registration accuracy, real-time data visualization, robust tracking, and seamless integration of these technologies into surgical workflows. Addressing these challenges will be an important step to ensure the safe and effective utilization of these technologies in daily clinical practice.
7. Conclusion
The last decade has witnessed significant advancements in the application of MR for image-guided procedures. The increased use of these technologies in the pre-operative phase, the growing adoption in the OR, the development of more ergonomic HMDs, and focus on cost-effective solutions demonstrate the maturation of this field. However, challenges remain, and further research is needed to address these challenges and fully unlock the potential of MR in enhancing surgical interventions. The future holds exciting possibilities for the continued integration of these technologies into clinical practice, ultimately leading to improved surgical outcomes and the advancement of surgical techniques.
Disclosure statement
No potential conflict of interest was reported by the author(s).
Additional information
Funding
References
- Milgram P, Takemura H, Utsumi A, et al. Augmented reality: a class of displays on the reality-virtuality continuum. In: Telemanipulator and telepresence technologies, vol. 2351. SPIE; 1995. p. 1–25.
- Kersten-Oertel M, Jannin P, Collins DL. The state of the art of visualization in mixed reality image guided surgery. Comput Med Imaging Graph. 2013;37(2):98–112. doi: 10.1016/j.compmedimag.2013.01.009.
- Kersten-Oertel M, Jannin P, Collins DL. DVV: a taxonomy for mixed reality visualization in image guided surgery. IEEE Trans Vis Comput Graph. 2011;18(2):332–352. doi: 10.1109/TVCG.2011.50.
- Móga K, Hölgyesi Á, Zrubka Z, et al. Augmented or mixed reality enhanced head-mounted display navigation for in vivo spine surgery: a systematic review of clinical outcomes. J Clin Med. 2023;12(11):3788. doi: 10.3390/jcm12113788.
- Han J, Kang HJ, Kim M, et al. Mapping the intellectual structure of research on surgery with mixed reality: bibliometric network analysis (2000–2019). J Biomed Inform. 2020;109:103516. doi: 10.1016/j.jbi.2020.103516.
- Gorpas D, Phipps J, Bec J, et al. Autofluorescence lifetime augmented reality as a means for real-time robotic surgery guidance in human patients. 2019.
- Alfonso-Garcia A, Bec J, Sridharan Weaver S, et al. Real-time augmented reality for delineation of surgical margins during neurosurgery using autofluorescence lifetime contrast. J Biophotonics. 2020;13(1):e201900108. doi: 10.1002/jbio.201900108.
- Cabrilo I, Bijlenga P, Schaller K. Augmented reality in the surgery of cerebral aneurysms: a technical report. Oper Neurosurg. 2014;10 Suppl 2(2):252–261. doi: 10.1227/NEU.0000000000000328.
- Liu J, Li X, Leng X, et al. Effect of 3D slicer preoperative planning and intraoperative guidance with mobile phone virtual reality technology on brain glioma surgery. Contrast Media Mol Imaging. 2022;2022. doi: 10.1155/2022/9627663.
- Simone M, Barile G, Luca RD, et al. Experimental setup employed in the operating room based on virtual and mixed reality: analysis of pros and cons in open abdomen surgery. J Healthc Eng. 2020;2020:8851964. doi: 10.1155/2020/8851964.
- Lu L, Wang H, Liu P, et al. Applications of mixed reality technology in orthopedics surgery: a pilot study. Front Bioeng Biotechnol. 2022;10:740507. doi: 10.3389/fbioe.2022.740507.
- Sauer IMM, Queisner M, Tang P, et al. Mixed reality in visceral surgery: development of a suitable workflow and evaluation of intraoperative use-cases. 2017.
- Bernard F, Haemmerli J, Zegarek G, et al. Augmented reality–assisted roadmaps during periventricular brain surgery. Neurosurg Focus. 2021;51(2):E4. doi: 10.3171/2021.5.FOCUS21220.
- Carl B, Bopp M, Benescu A, et al. Indocyanine green angiography visualized by augmented reality in aneurysm surgery. World Neurosurg. 2020;142:e307–e315. doi: 10.1016/j.wneu.2020.06.219.
- Carl B, Bopp M, Saß B, et al. Implementation of augmented reality support in spine surgery. Eur Spine J. 2019;28(7):1697–1711. doi: 10.1007/s00586-019-05969-4.
- Carl B, Bopp M, Saß B, et al. Spine surgery supported by augmented reality. Spine J. 2020;23:27–33.
- Carl B, Bopp M, Saß B, et al. Microscope-based augmented reality in degenerative spine surgery: initial experience. World Neurosurg. 2019;128:e541–e551. doi: 10.1016/j.wneu.2019.04.192.
- Carl B, Bopp M, Saß B, et al. Augmented reality in intradural spinal tumor surgery. Acta Neurochir. 2019;161(10):2181–2193. doi: 10.1007/s00701-019-04005-0.
- Kim HM, Lee MH, Lee TK. Application of augmented reality using neuro-navigation system for individualized brain tumor surgery. J Korean Skull Base Soc. 2022;17(2): 68–74.
- Rychen J, Goldberg J, Raabe A, et al. Augmented reality in superficial temporal artery to middle cerebral artery bypass surgery: technical note. Oper Surg. 2019;18(4):444–450. doi: 10.1093/ons/opz176.
- Schwam ZG, Kaul VF, Bu DD, et al. The utility of augmented reality in lateral skull base surgery: a preliminary report. Am J Otolaryngol. 2021;42(4):102942. doi: 10.1016/j.amjoto.2021.102942.
- Zeiger J, Costa A, Bederson J, et al. Use of mixed reality visualization in endoscopic endonasal skull base surgery. Oper Surg. 2019;19(1):43–52. doi: 10.1093/ons/opz355.
- Butler AJ, Colman MW, Lynch J, et al. Augmented reality in minimally invasive spine surgery: early efficiency and complications of percutaneous pedicle screw instrumentation. Spine J. 2023;23(1):27–33. doi: 10.1016/j.spinee.2022.09.008.
- Bhatt FR, Orosz LD, Tewari A, et al. Augmented reality-assisted spine surgery: an early experience demonstrating safety and accuracy with 218 screws. Global Spine J. 2023;13(7):2047–2052. doi: 10.1177/21925682211069321.
- Borgmann H, Rodríguez Socarrás M, Salem J, et al. Feasibility and safety of augmented reality-assisted urological surgery using smartglass. World J Urol. 2017;35(6):967–972. doi: 10.1007/s00345-016-1956-6.
- Bopp MH, Saß B, Pojskić M, et al. Use of neuronavigation and augmented reality in transsphenoidal pituitary adenoma surgery. J Clin Med. 2022;11(19):5590. doi: 10.3390/jcm11195590.
- Collins T, Pizarro D, Gasparini S, et al. Augmented reality guided laparoscopic surgery of the uterus. IEEE Trans Med Imaging. 2020;40(1):371–380. doi: 10.1109/TMI.2020.3027442.
- Ivanov VM, Krivtsov AM, Strelkov SV, et al. Practical application of augmented/mixed reality technologies in surgery of abdominal cancer patients. J Imaging. 2022;8(7):183. doi: 10.3390/jimaging8070183.
- Kersten-Oertel M, Gerard I, Drouin S, et al. Augmented reality in neurovascular surgery: feasibility and first uses in the operating room. Int J Comput Assist Radiol Surg. 2015;10(11):1823–1836. doi: 10.1007/s11548-015-1163-8.
- Pratt P, Ives M, Lawton G, et al. Through the Hololens™ looking glass: augmented reality for extremity reconstruction surgery using 3D vascular models with perforating vessels. Eur Radiol Exp. 2018;2(1):2. doi: 10.1186/s41747-017-0033-2.
- Burström GM, Nachabe RP, Homan RB, et al. Frameless patient tracking with adhesive optical skin markers for augmented reality surgical navigation in spine surgery. Spine. 2020;45(22):1598–1604. doi: 10.1097/BRS.0000000000003628.
- Edström E, Burström G, Nachabe R, et al. A novel augmented-reality-based surgical navigation system for spine surgery in a hybrid operating room: design, workflow, and clinical applications. Oper Neurosurg. 2020;18(5):496–502. doi: 10.1093/ons/opz236.
- Edström E, Burström G, Persson O, et al. Does augmented reality navigation increase pedicle screw density compared to free-hand technique in deformity surgery? Single surgeon case series of 44 patients. Spine. 2020;45(17):E1085–E1090. doi: 10.1097/BRS.0000000000003518.
- Elmi-Terander A, Burström G, Nachabé R, et al. Augmented reality navigation with intraoperative 3D imaging vs fluoroscopy-assisted free-hand surgery for spine fixation surgery: a matched-control study comparing accuracy. Sci Rep. 2020;10(1):707. doi: 10.1038/s41598-020-57693-5.
- Golse N, Petit A, Lewin M, et al. Augmented reality during open liver surgery using a markerless non-rigid registration system. J Gastrointest Surg. 2021;25(3):662–671. doi: 10.1007/s11605-020-04519-4.
- Carl B, Bopp M, Voellger B, et al. Augmented reality in transsphenoidal surgery. World Neurosurg. 2019;125:e873–e883.
- Bourdel N, Chauvet P, Calvet L, et al. Use of augmented reality in gynecologic surgery to visualize adenomyomas. J Minim Invasive Gynecol. 2019;26(6):1177–1180. doi: 10.1016/j.jmig.2019.04.003.
- Wierzbicki R, Pawłowicz M, Job J, et al. 3D mixed-reality visualization of medical imaging data as a supporting tool for innovative, minimally invasive surgery for gastrointestinal tumors and systemic treatment as a new path in personalized treatment of advanced cancer diseases. J Cancer Res Clin Oncol. 2022;148(1):237–243. doi: 10.1007/s00432-021-03680-w.
- Léger É, Drouin S, Collins DL, et al. Quantifying attention shifts in augmented reality image-guided neurosurgery. Healthc Technol Lett. 2017;4(5):188–192. doi: 10.1049/htl.2017.0062.
- Lim AK, Ryu J, Yoon HM, et al. Ergonomic effects of medical augmented reality glasses in video-assisted surgery. Surg Endosc. 2022;36:988–998.
- Li C, Zheng B, Yu Q, et al. Augmented reality and 3-dimensional printing technologies for guiding complex thoracoscopic surgery. Ann Thorac Surg. 2021;112(5):1624–1631. doi: 10.1016/j.athoracsur.2020.10.037.
- Léger É, Reyes J, Drouin S, et al. Marin: an open-source mobile augmented reality interactive neuronavigation system. Int J Comput Assist Radiol Surg. 2020;15(6):1013–1021. doi: 10.1007/s11548-020-02155-6.
- Hussain R, Guigou C, Lalande A, et al. Vision-based augmented reality system for middle ear surgery: evaluation in operating room environment. Otol Neurotol. 2022;43(3):385–394. doi: 10.1097/MAO.0000000000003441.
- Goto Y, Kawaguchi A, Inoue Y, et al. Efficacy of a novel augmented reality navigation system using 3D computer graphic modeling in endoscopic transsphenoidal surgery for sellar and parasellar tumors. Cancers. 2023;15(7):2148. doi: 10.3390/cancers15072148.
- Mozaffari K, Foster C, Rosner M. Practical use of augmented reality modeling to guide revision spine surgery: an illustrative case of hardware failure and overriding spondyloptosis. Oper Neurosurg. 2022;23(3):212–216. doi: 10.1227/ons.0000000000000307.
- Phan TN, Prakash KJ, Elliott RJS, et al. Virtual reality–based 3-dimensional localization of stereotactic EEG (sEEG) depth electrodes and related brain anatomy in pediatric epilepsy surgery. Childs Nerv Sys. 2022;38:537–546.
- Satoh M, Nakajima T, Yamaguchi T, et al. Evaluation of augmented-reality based navigation for brain tumor surgery. J Clin Neurosci. 2021;94:305–314. doi: 10.1016/j.jocn.2021.10.033.
- Alshomer F, Alazzam A, Alturki A, et al. Smartphone-assisted augmented reality in craniofacial surgery. Plast Reconstr Surg Glob Open. 2021;9(8):e3743.
- García-Mato D, Moreta-Martínez R, García-Sevilla M, et al. Augmented reality visualization for craniosynostosis surgery. Comput Methods Biomech Biomed Eng Imaging Vis. 2021;9(4):392–399. doi: 10.1080/21681163.2020.1834876.
- Guerriero L, Quero G, Diana M, et al. Virtual reality exploration and planning for precision colorectal surgery. Dis Colon Rectum. 2018;61(6):719–723. doi: 10.1097/DCR.0000000000001077.
- Yodrabum N, Rudeejaroonrung K, Chaikangwan I, et al. Precision of low-cost augmented reality in prefabricated cutting guide for fibular free flap surgery. J Craniofac Surg. 2022;33(3):916–919. doi: 10.1097/SCS.0000000000008074.
- Tang R, Ma L, Xiang C, et al. Augmented reality navigation in open surgery for hilar cholangiocarcinoma resection with hemihepatectomy using video-based in situ three-dimensional anatomical modeling: a case report. Medicine. 2017;96(37):e8083. doi: 10.1097/MD.0000000000008083.
- Lee D, Yu HW, Kim S, et al. Vision-based tracking system for augmented reality to localize recurrent laryngeal nerve during robotic thyroid surgery. Sci Rep. 2020;10(1):8437. doi: 10.1038/s41598-020-65439-6.
- Arensmeyer J, Bedetti B, Schnorr P, et al. Novel application of real-time editable 3D-reconstructed imaging in a mixed-reality holographic overlay using a video pass-through head-mounted display – a pathway to future navigation in thoracic surgery? 2022.
- Guo Q, Li X, Tang Y, et al. Augmented reality and three-dimensional plate library-assisted posterior minimally invasive surgery for scapula fracture. Int Orthop. 2022;46(4):875–882. doi: 10.1007/s00264-022-05303-6.
- Han W, Yang X, Wu S, et al. A new method for cranial vault reconstruction: augmented reality in synostotic plagiocephaly surgery. J Craniomaxillofac Surg. 2019;47(8):1280–1284. doi: 10.1016/j.jcms.2019.04.008.
- Liu J, Li X, Leng X, et al. Effect of 3D slicer preoperative planning and intraoperative guidance with mobile phone virtual reality technology on brain glioma surgery. Contrast Media Mol Imaging. 2022;2022:9627663. doi: 10.1155/2022/9627663.
- Ceccariglia F, Cercenelli L, Badiali G, et al. Application of augmented reality to maxillary resections: a three-dimensional approach to maxillofacial oncologic surgery. J Pers Med. 2022;12(12):2047. doi: 10.3390/jpm12122047.
- Schneider CS, Thompson SJS, Totz JNR, et al. Comparison of manual and semi-automatic registration in augmented reality image-guided liver surgery: a clinical feasibility study. Surg Endosc. 2020;34(10):4702–4711. doi: 10.1007/s00464-020-07807-x.
- Scherl C, Stratemeier J, Karle C, et al. Augmented reality with Hololens in parotid surgery: how to assess and to improve accuracy. Eur Arch Otorhinolaryngol. 2021;278(7):2473–2483. doi: 10.1007/s00405-020-06351-7.
- Scherl C, Stratemeier J, Rotter N, et al. Augmented reality with Hololens® in parotid tumor surgery: a prospective feasibility study. 2021.
- Saito Y, Sugimoto M, Imura S, et al. Intraoperative 3D hologram support with mixed reality techniques in liver surgery. Ann Surg. 2020;271(1):e4–e7. doi: 10.1097/SLA.0000000000003552.
- Buch VP, Mensah-Brown KG, Germi JW, et al. Development of an intraoperative pipeline for holographic mixed reality visualization during spinal fusion surgery. Surg Innov. 2021;28(4):427–437. doi: 10.1177/1553350620984339.
- Guerrera F, Nicosia S, Costardi L, et al. Proctor-guided virtual reality–enhanced three-dimensional video-assisted thoracic surgery: an excellent tutoring model for lung segmentectomy. Tumori J. 2021;107(6):NP1–NP4. doi: 10.1177/0300891620972173.
- Shuhaiber J. Augmented reality in surgery. Arch Surg. 2004;139(2):170–174. doi: 10.1001/archsurg.139.2.170.
- Umebayashi D, Yamamoto Y, Nakajima Y, et al. Augmented reality visualization-guided microscopic spine surgery: transvertebral anterior cervical foraminotomy and posterior foraminotomy. J Am Acad Orthop Surg Glob Res Rev. 2018;2(4):e008. doi: 10.5435/JAAOSGlobal-D-17-00008.
- Mishvelov AE, Ibragimov AK, Amaliev IT, et al. Computer-assisted surgery: virtual- and augmented-reality displays for navigation during planning and performing surgery on large joints. Pharmacophore. 2021;12(2):32–38. doi: 10.51847/50jmUfdufI.
- Balci D, Kirimker EO, Raptis DA, et al. Uses of a dedicated 3D reconstruction software with augmented and mixed reality in planning and performing advanced liver surgery and living donor liver transplantation (with videos). Hepatob Pancreat Dis Int. 2022;21:455–461.
- Linxweiler M, Pillong L, Kopanja D, et al. Augmented reality-enhanced navigation in endoscopic sinus surgery: a prospective, randomized, controlled clinical trial. Laryngosc Investig Otol. 2020;5(4):621–629. doi: 10.1002/lio2.436.
- Medzhidova SA, Koshenov NA, Nahusheva LR, et al. Application of augmented reality technology in abdominal surgery in patients with liver diseases. Int Trans J Eng Manag Appl Sci Technol. 2022;13:1–9.
- De Backer P, Van Praet C, Simoens J, et al. Improving augmented reality through deep learning: real-time instrument delineation in robotic renal surgery. Eur Urol. 2023;84(1):86–91. doi: 10.1016/j.eururo.2023.02.024.
- Dongheon L, Hyoun-Joong K, Donguk K, et al. Preliminary study on application of augmented reality visualization in robotic thyroid surgery. ASTR. 2018;95:297–302.
- Gouveia PF, Costa J, Morgado P, et al. Breast cancer surgery with augmented reality. Breast. 2021;56:14–17. doi: 10.1016/j.breast.2021.01.004.
- Porpiglia F, Checcucci E, Amparore D, et al. Augmented-reality robot-assisted radical prostatectomy using hyper-accuracy three-dimensional reconstruction (HA 3DTM) technology: a radiological and pathological study. BJU Int. 2019;123(5):834–845.
- Diana M, Soler LP, Agnus VP, et al. Prospective evaluation of precision multimodal gallbladder surgery navigation: virtual reality, near-infrared fluorescence, and x-ray-based intraoperative cholangiography. Ann Surg. 2017;266(5):890–897. doi: 10.1097/SLA.0000000000002400.
- Aoyama R, Anazawa U, Hotta H, et al. Augmented reality device for preoperative marking of spine surgery can improve the accuracy of level identification. Spine Surg Relat Res. 2022;6(3):303–309. doi: 10.22603/ssrr.2021-0168.
- Incekara F, Smits M, Dirven C, et al. Clinical feasibility of a wearable mixed-reality device in neurosurgery. World Neurosurg. 2018;118:e422–e427. doi: 10.1016/j.wneu.2018.06.208.
- Zhu M, Liu F, Chai G, et al. A novel augmented reality system for displaying inferior alveolar nerve bundles in maxillofacial surgery. Sci Rep. 2017;7(1):42365. doi: 10.1038/srep42365.
- Hu MH, Chiang CC, Wang ML, et al. Clinical feasibility of the augmented reality computer-assisted spine surgery system for percutaneous vertebroplasty. Eur Spine J. 2020;29(7):1590–1596. doi: 10.1007/s00586-020-06417-4.
- Molina CAM, Sciubba DMM, Greenberg JK, et al. Clinical accuracy, technical precision, and workflow of the first in human use of an augmented-reality head-mounted display stereotactic navigation system for spine surgery. 2021.
- Condino S, Carbone M, Piazza R, et al. Perceptual limits of optical see-through visors for augmented reality guidance of manual tasks. IEEE Trans Biomed Eng. 2019;67:411–419.
- Sasaki T, Dehari H, Ogi K, et al. Application of a mixed reality device to oral surgery. Adv Oral Maxillofac Surg. 2022;8:100331. doi: 10.1016/j.adoms.2022.100331.
- Gregory TM, Gregory J, Sledge J, et al. Surgery guided by mixed reality: presentation of a proof of concept. Acta Orthop. 2018;89(5):480–483. doi: 10.1080/17453674.2018.1506974.
- Lysenko A, Razumova A, Andrey Y, et al. The use of augmented reality navigation technology in combination with endoscopic surgery for the treatment of an odontogenic cyst of the upper jaw: a technical report. Imaging Sci Dent. 2022;52(2):225–230. doi: 10.5624/isd.20210256.
- Nguyen T, Plishker W, Matisoff A, et al. Holous: augmented reality visualization of live ultrasound images using Hololens for ultrasound-guided procedures. Int J Comput Assist Radiol Surg. 2022;17(2):385–391. doi: 10.1007/s11548-021-02526-7.
- Farshad-Amacker NA, Kubik-Huch RA, Kolling C, et al. Learning how to perform ultrasound-guided interventions with and without augmented reality visualization: a randomized study. Eur Radiol. 2023;33(4):2927–2934. doi: 10.1007/s00330-022-09220-5.
- Gerard IJ, Kersten-Oertel M, Drouin S, et al. Combining intraoperative ultrasound brain shift correction and augmented reality visualizations: a pilot study of eight cases. J Med Imaging. 2018;5(2):021210. doi: 10.1117/1.JMI.5.2.021210.