ABSTRACT
Sleep is often compromised in female athletes, and the monitoring of female athletes' sleep is an important preventative and educational tool. With self-reporting of sleep common practice for athletes as part of a daily wellness assessment, there is a need to understand whether sleep indices are being reported accurately, and thus whether self-report data are useful. This study aimed to compare the agreement between self-reported and actigraphy reported sleep duration in female football players, with the intention of informing best practice for athlete monitoring. Twenty-two female footballers (mean age 19.5 ± 1.3 years) provided a daily self-report across 7 days, whilst also wearing an actigraph across the same testing period. Agreement between the two measures was assessed using Bland-Altman limits of agreement, with acceptable limits of agreement defined as <30 minutes. Results showed evident disagreement between the two methods, with a mean bias of −0.54 (32 min, 95% CI −0.66 to −0.43) and a potential disagreement range of over 2 h (Lower 95% limits of agreement −1.49 to upper 95% limits of agreement 0.40). Coaches using self-reported sleep durations as a monitoring tool for female footballers should interpret the results with caution and be aware of the potential for inaccuracies in this measure. As such, where possible, coaches should consider other methods of sleep monitoring, rather than solely relying on a self-report, to ensure they are operating with optimal practice within situational constraints.
Introduction
Success in sport is underpinned by optimal preparation and recovery (Juliff et al. Citation2015). With sleep being highlighted by Halson (Citation2013) as the single most important recovery strategy available to an athlete, the accurate monitoring of sleep is crucial to optimise recovery status, and thus, performance. Short sleep has been shown to negatively affect sports-specific skills, coordination, mood, rating of perceived exertion and injury risk (Mah et al. Citation2019; Walsh et al. Citation2021; Costa et al. Citation2022); therefore, identifying athletes experiencing regular sleep debt is of paramount importance for both performance and athlete wellbeing, and thus, sleep must be monitored. Sleep is often compromised in athletes due to enforced training times, competition scheduling, travel, stress or physiological arousal in the evening (Nédélec et al. Citation2012; Halson Citation2014), with female athletes being at particular risk of sleep issues (Walsh et al. Citation2021). Athletes are particularly susceptible to short sleep duration, with a recent systematic review finding the mean sleep duration of over 1860 athletes, was 7.2 ± 1.1 h per night (Vlaihoyannis et al. Citation2021); a duration lower than recommendations from recent studies, which suggest athletes need 7.9 h (Morita and Sasai-Sakuma Citation2021) and 8.3 h (Sargent et al. Citation2021) in order to report satisfaction with their sleep. Sleep duration is a factor which contributes to the perception of ‘a good nights’ sleep’ (Ogeil et al. Citation2021).
With as many as 50–78% of elite athletes reporting sleep disturbance and 22–26% of athletes suffering highly disturbed sleep (Samuels Citation2008; Swinbourne et al. Citation2016; Gupta et al. Citation2017), the accurate reporting of athlete sleep data is paramount to ensure early intervention and prevention of performance degradation. Early work by Reilly and Piercy (Citation1994) demonstrated a significant reduction in maximal bench press and deadlift following sleep deprivation (3 h per night sleep allowance). Sleep deprivation in athletes has been shown to result in reductions in the following parameters: sprint times (Skein et al. Citation2011), countermovement jump performance (Skein et al. Citation2013), grip strength (Souissi et al. Citation2013), cognitive performance (Fullagar et al. Citation2015) and sports performance (Reyner and Horne Citation2013; Sinnerton and Reilly Citation2013). Findings from a systematic review of 69 publications on the effects of sleep loss on physical performance (Craven et al. Citation2022) suggested a negative impact of sleep loss on exercise performance, highlighted the need for robust monitoring of athletes’ sleep.
Across a sample of 207 male athletes and 215 female athletes, Kawasaki et al. (Citation2020) found females were more likely to have poor sleep, which could be attributed to lifestyle factors and hormonal changes throughout the menstrual cycle. Research around how sleep factors are affected by the MC are inconclusive – most likely due to the level of intra and inter-individual variation of the MC (Elliott-Sale et al. Citation2021).
Whilst the importance of sleep to recovery and performance seems clear (Fullagar et al. Citation2015), the best practice to monitor sleep within an athletic population is more controversial. There is currently no ‘standardised’ practice in sleep monitoring of athletes (Halson Citation2019) and the monitoring of choice is often largely determined by the practitioners’ interpretation of best practice. Polysomnography (PSG) is considered the gold standard method for monitoring sleep (Van De Water et al. Citation2011), a measure which detects sleep via brain-wave activity rather than motion. However, PSG is an expensive, lab-based measure, also potentially impractical and disruptive for sleep tracking purposes (Caia et al. Citation2018; Halson Citation2019). Therefore, a common method for the monitoring of athletes’ sleep is to use actigraphy devices. One of the primary benefits of actigraphy-based sleep monitoring is that data can be collected over multiple nights in the participants’ usual sleep environment, which has been demonstrated to provide more reliable estimates of sleep compared to one or two nights in a sleep laboratory (Blackwell et al. Citation2008; Rupp and Balkin Citation2011), as well as giving the data high ecological validity. Actigraphy has been compared against PSG in multiple populations (Sadeh et al. Citation1994; de Souza et al. Citation2003; Meltzer et al. Citation2012), including athletes (Sargent et al. Citation2016). Concordance between PSG and actigraphy appears good, with previous work yielding agreement in the range of 78–95% (Kushida et al. Citation2001).
Self-reporting can be defined as an individual’s’ own report of their perspectives, behaviours or beliefs (Stone et al. Citation1999) and self-reporting of sleep is commonly used within an athletic population. Both the use of both validated questionnaires (Buyssee et al. Citation1989) and simple questions, often utilising Likert scales (Sargent et al. Citation2021), are commonplace for the monitoring of athletes' sleep (Halson Citation2019). Recent research has employed the use of short questions (Caia et al. Citation2018; Sargent et al. Citation2021) to provide self-report measures, likely due to the lesser time commitment from the athlete, and the fact that many athletes within an elite setting are habitually answering these types of questions daily as a requirement for daily wellness monitoring. It must be established, however, whether these self-report measures are accurate and therefore providing meaningful data. In some circumstances, access to objective measures may be limited by funding or availability, therefore it must be established whether self-reported measures can provide accurate and reliable estimates of sleep factors. Self-reported measures are significantly less expensive to administer and are potentially less time-consuming in the process of data analysis, compared to other measures (Saw et al. Citation2016). In a systematic review of 56 studies comparing self-report and objective reporting of athlete recovery status, Saw et al. (Citation2016) summarised that subjective self-reported measures provided superior sensitivity and consistency compared to objective measures. They concluded self-reported measures, used on a regular basis, are useful to reflect changes in athlete wellbeing and that coaches should employ self-report measures with confidence. However, the use of self-report questionnaires may be affected by response bias, with data interpretation potentially affected by the lack of standardised data for athletes (Zhang et al. Citation2022). Despite these potential issues, self-report measures are widely used for monitoring athletes in high-performance sport (Taylor et al. Citation2012), with many daily wellness questionnaires incorporating questions regarding sleep factors (Brown et al. Citation2021).
Kölling et al. (Citation2016) compared actigraphy reporting and self-reporting of sleep duration for 72 physical education students (32 females); they demonstrated good agreement between the two measures for sleep duration (ICC = 0.90 to 0.92). Sub-analyses were not done to understand any potential differences in reporting between the sexes, demonstrating a gap in understanding of the reporting of female athlete sleep. There is a paucity of information on the validity and reliability of self-reported sleep measures compared to objective sleep measures in female athletes and this study would endeavour to be the first. With the intention to examine the usefulness of sleep questions within daily wellness questionnaires, this study aims to compare the agreement between self-reporting and actigraphy reporting of sleep duration in female football players, thus informing best practice for athlete monitoring. Based on comparable reports in a male athlete population, it was hypothesised that there would be good agreement between the two methods for both sleep durations.
Method
Participants
A convenience sample of 22 female football players volunteered to take part. All participants (mean age 19.5 ± 1.3 years) were part of the U21 squad at their football clubs and played regularly in the Women’s Super League (WSL) Academy League. Prior to the commencement of the study, all participants were informed of study requirements and gave informed consent. Participants were excluded if they reported a pre-existing sleep disorder, had a menstrual cycle outside the range of 21–35 days or did not give informed consent. Institutional ethical approval was issued (protocol number 2022–10035) in accordance with the Declaration of Helsinki 1964 (revised 2013). Prior to the testing period, participants were asked to wear an actigraphy device for 7 days to collect baseline sleep data; no outliers were identified during this familiarisation period. Throughout the whole data collection period, participants were advised to maintain their normal sleep routines, and slept in their usual, home-based environment. During the 7 days of data collection, participants competed their usual on-pitch training (mean 10.7 ± 1.5 h) and gym-based training (mean 3.0 ± 0.9 h). Sixteen players took part in one match, whilst six players did not take part in any matches during this period.
Experimental design
A repeated measure design across 7 days was used to assess the agreement between self-reporting and actigraphy reporting of sleep duration and sleep quality parameters. Given the potential for seasonal adjustment of sleep patterns (Allebrandt et al. Citation2014), it should be noted that data was collected during the month of October.
Actigraphy sleep assessment
Baseline sleep data was collected for 7 days, which served an additional purpose of familiarisation. Following baseline data collection, participants were asked to wear an actigraphy device (ActiGraph GT9X, Florida, USA) for a duration of further seven nights. Devices were set to collect data in 1-min epochs, and participants were instructed to wear the devices on their non-dominant wrist, only removing them for matches throughout the testing period.
Subjective sleep assessment
Across the same monitoring period, participants were asked to give a self-repoted sleep duration on Microsoft Forms, first thing upon waking, as demonstrated by Caia et al. (Citation2018):
Please give an estimate of your sleep duration (how long you slept for (hh:mm))
Participants were sent a reminder to complete this via a group text message from coaches, as such, the completion rate was 100%. All participants were familiar with this question and were habitually completing this daily as a requirement from their clubs. At the end of the monitoring period, self-report for each night was compared with actigraph data of the same night for each participant.
Statistical Analyses
Descriptive statistics (mean ± SD) were calculated for daily for each method, with % CV used to present dispersion around the mean. Estimates of reliability and agreement between objective and subjective measures of sleep were computed. All of the above were calculated using the ‘SimplyAgree’ package (Caldwell Citation2022; https://doi.org/10.21105/joss.04148) in R (R core team, Citation2020). Limits of Agreement plots (plotting the mean against the difference of the measures) were used to estimate the range within which differences between the measurements lie; Bland and Altman (Citation2007) suggested this to be the most appropriate method for determining agreement between methods with multiple observations, thus maintaining within subject variation. Repeated assessments were taken into account in the estimation of limits of agreement by incorporating within-subject variation to reduce the risk of producing limits of agreement that are too narrow and therefore estimating higher agreement between measures than is true (Bland and Altman Citation2007). Repeats of objective and subjective sleep measurement were assumed to vary within participants across days (Bland and Altman Citation2007; Zou, Citation2013), with limits of agreement estimated based on methods accounting for such nested, ‘non-constant’ contexts using the ‘agree_nest’ function (Bland and Altman Citation2007; Caldwell Citation2022). Assumptions relating to the normal distribution of residuals, heteroscedasticity and proportional bias were checked and verified through visual inspection of the plots produced using the ‘check’ function within the ‘SimplyAgree’ package (Caldwell Citation2022). The agreement between the two methods was subsequently visually represented.
If measurements with the two methods are similar, the differences between them will be small, with an average near zero, they will be consistent over the range of measurement values, and the limits of agreement will be narrow (Abu-Arafeh et al. Citation2016). There is a paucity of research presenting either acceptable limits of agreement within this cohort, or minimal clinically important differences, however in line with available evidence in other populations (Werner et al. Citation2008; Meltzer et al. Citation2012), limits of agreement of <30 min were considered acceptable. Reporting of the Bland-Altman analysis has been aligned with considerations listed by Abu-Arafeh et al. (Citation2016).
Results
A total of 308 observations were collected from n = 22 participants (154 actigraph, 154 self-report) across a 7-day period. The mean sleep duration across the investigated days using actigraphy was 6.63 ± 0.87 h. Computed coefficient of variation (CV) indicated that duration of sleep as measured through actigraphy varied by 10.9% across the investigated period. The subjective assessment of sleep duration yielded a mean of 7.18 ± 0.84 h, with a CV of 9.64% across the studied duration, on average, for each participant ().
Table 1. Sleep durations (hours) recorded using actigraphy and self-report.
A limits of agreement plot were generated () and demonstrated that 95% of the actigraph scores were between 1.49 lower and 0.40 greater than self-report.
Figure 1. Limits of agreement plot comparing actigraph reported sleep duration and self-reported sleep duration. Red-dashed line and error bar represents the pre-defined acceptable range of agreement (−15 min to +15 min).
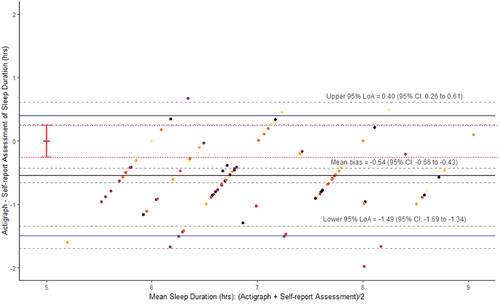
Analysis of the self-report vs actigraphy reported sleep duration demonstrated a mean bias of −0.54 h (95% CI − 0.66 to − 0.43) meaning there was a tendency for participants to overestimate their self-reported sleep duration by 32 min. With the limits of agreement ranging from +24 min to −90 min, true differences between objective and self-reported measurements for 95% of pairs of future sleep observations are expected to be as high as 2 h. Accordingly, our findings suggest self-reported measurements of sleep may lack justification as interchangeable to actigraphy-based tracking.
Discussion
This study aimed to assess measurement agreement between self-reported and actigraphy reported sleep duration for sleep tracking in female footballers, with the intention to determine whether a self-report of this factor could be considered valid and useful. Bland-Altman analysis demonstrated clear disagreement between the two methods, with a mean bias of −0.54 (32 min), and the width of the limits of agreement spanning almost more than 2 h. Therefore, the two measures did not meet earlier defined acceptable limits of agreement of <30 min. de Zambotti et al. (Citation2015) suggested even if data comply with defined acceptable limits, the determination of clinical usefulness should also take into account the dispersion of discrepancies (upper and lower limits of agreement) rather than solely the average of the discrepancies. Given the large agreement range within the present study, considering this factor gives greater confidence towards the measures in the current study presenting in evident disagreement, suggesting that self-reported sleep durations for female athletes may not be providing useful or meaningful data. Determining athletes’ sleep duration is a key component of athlete monitoring; an inaccurate answer could mean that adjustments to subsequent training routines could be unnecessary or illogical, whilst equivocally, in the absence of accurate data, necessary adjustments may be missed.
Previous research on non-athletic cohorts has demonstrated poor agreement between self-reported and actigraphy reported sleep duration (Billings Citation2022). Similarly, Jackowska et al. (Citation2016) reported weak association between self-reported and actigraphy reported sleep durations amongst women (non-athletes), mean age 26 ± 4.9. However, previous research comparing self-reported and actigraphy reported sleep parameters on athletic cohorts are sparse. Caia et al. (Citation2018) investigated this research question with male rugby players reporting a large, positive correlation (r = 0.85) between self-reported and actigraphy reported sleep duration. However, statistical issues limit the interpretation and comparison to the present study, as statistical correlation does not necessarily indicate good agreement between methods, simply the shared variance between measures (Giavarina Citation2015); additionally, the authors of the aforementioned study have used between-subjects correlations, despite examining repeated measures data. The present study is novel in its approach, addressing previous methodological errors in prior publications, by using the more appropriate Bland-Altman analysis for repeated measures, as well as providing novel insights into the usefulness of self-reported sleep duration for female athletes.
Results showed a tendency for players to overestimate their sleep duration with a mean bias of 32 min. One reason for such overestimation could perhaps be attributed to conscious bias, with athletes potentially keen to report more favourable responses (Saw et al. Citation2015; Halson Citation2019). Considering the mean bias and the large disagreement range within this female athlete cohort, relying on a self-reported sleep duration to provide meaningful monitoring of female athletes may be considered as problematic, potentially leading to a lack of appreciation for the athlete operating with repeated short sleep, or in a state of partial sleep deprivation. Physiologically, neural changes can be observed when operating in a state of partial sleep deprivation, with reductions in functional MRI signal in the dorsolateral prefrontal cortex and intraparietal sulcus evident whilst performing attentional tasks (Krause et al. Citation2017). Previous research has demonstrated the behavioural manifestations of such neural changes with athletes incurring reduced reaction time, focus, psychomotor performance, determination, and vigilance (Davenne Citation2009; Edwards and Waterhouse Citation2009; Underwood Citation2010) in response to lack of sleep. Early identification of sleep loss or mounting sleep debt is of paramount importance in the prevention of negative performance and health impacts, thus this study highlights the need for methods beyond solely self-reporting of sleep durations to be employed, where possible, for female footballers, to accurately determine sleep status, prevent potential performance degradation and in the proactive prevention of habitually poor sleep.
In this investigation, menstrual cycle was monitored for cycle length within inclusion criteria. However, a potential limitation of this study was the fact that the menstrual cycle phase was not controlled for during the testing period, and many participants may have been at different phases of their cycles. Menstrual cycle phases may affect sleep variables (Baker and Driver Citation2007) and thus potentially, both the subjective interpretation of sleep factors and the actigraph report. Elliott-Sale et al. (Citation2021) states when a study is not examining direct effects on the menstrual cycle on an outcome, a description of the population is solely relevant. However, not controlling for cycle phase may also be viewed as a strength of the study, as the results are representative of females at varying points of their cycle thus indicative of a wider representation of the data.
During the process of data collection, it is feasible that participants felt a pressure to perform, which could have affected the self-reported data. Additionally, Kuosmanen et al. (Citation2022) noted the potential for sleep monitoring and wearing sleep tracking devices to provide an additional stress, which may have skewed results. The use of collecting baseline data in this instance, not only served as establishing sleep norms for each participant but also could have had the additional benefit of familiarisation and mitigation of the aforementioned factors. Although the testing period in this study was comparable with previous research, which ranged from 3 days (Kölling et al. Citation2016) to 10 days (Caia et al. Citation2018), further prevention of these issues could be done via a longer testing period.
Practical applications and limitations
Coaches using a self-report of sleep duration as part of daily athlete monitoring should be interpreting the results with caution. With issues in the accuracy of self-reported sleep durations in this cohort, coaches should be aware of the potential misinformation they may be receiving regarding athletes’ sleep status, the subsequent risk of implementing potentially misinformed programme adjustments based on this data, or missing key information which could affect their athletes' health and performance. As such, coaches should consider other methods of sleep monitoring, rather than solely relying on a self-report, to ensure they are operating with optimal practice within situational constraints. Although many studies have compared actigraphy with concurrent polysomnography reporting high correlations, this study did not include internal validation of the actigraphs used, which could be a potential limitation with regard to the validity of the data. Although this study chose not to control for menstrual cycle phase, further research may be directed towards the comparison of self-reported and actigraphy reported sleep data during different phases of the menstrual cycle, with a view to offering a more detailed analysis of the accuracy of self-reporting at various points throughout the monthly cycle. Finally, the sample size used may not have been adequate (Lu et al. Citation2016), yet was limited due to players’ availability. As such, the study would benefit from being repeated with a more appropriate sample size.
Author contributions
Study conception – Julie Gooderick, Neil Maxwell, Mark Hayes, Will Abbott
Data collection – Julie Gooderick, Toby Wood
Data analysis – Julie Gooderick, Mark Hayes, Will Abbott, Toby Wood
Write up – Julie Gooderick, Mark Hayes, Neil Maxwell
Disclosure statement
No potential conflict of interest was reported by the author(s).
Data availability statement
The data that support the findings of this study are available from the corresponding author, JG, upon reasonable request.
Additional information
Funding
References
- Abu-Arafeh A, Jordan H, Drummond G. 2016. Reporting of method comparison studies: a review of advice, an assessment of current practice, and specific suggestions for future reports. BJA: Br J Anaesth. 117(5):569–575.
- Allebrandt KV, Teder-Laving M, Kantermann T, Peters A, Campbell H, Rudan I, Wilson JF, Metspalu A, Roenneberg T. 2014. Chronotype and sleep duration: the influence of season of assessment. Chronobiol Int. 31(5):731–740. doi: 10.3109/07420528.2014.901347.
- Baker FC, Driver HS. 2007. Circadian rhythms, sleep, and the menstrual cycle. Sleep Med. 8(6):613–622. doi: 10.1016/j.sleep.2006.09.011.
- Billings JM. 2022. Firefighter sleep: a pilot study of the agreement between actigraphy and self-reported sleep measures. J Clin Sleep Med. 18(1):109–117. doi: 10.5664/jcsm.9566.
- Blackwell T, Redline S, Ancoli-Israel S, Schneider JL, Surovec S, Johnson NL, Cauley JA, Stone KL, Study of Osteoporotic Fractures Research Group. 2008. Comparison of sleep parameters from actigraphy and polysomnography in older women: the SOF study. Sleep. 31(2):283–291. doi: 10.1093/sleep/31.2.283.
- Bland JM, Altman DG. 2007. Agreement between methods of measurement with multiple observations per individual. J Biopharm Stat. 17(4):571–582. doi: 10.1080/10543400701329422.
- Brown GA, Veith S, Sampson JA, Whalan M, Fullagar HH. 2021. Adolescent football players’ sleep, wellness and school physical activity over different phases of the year. Int J Sports Sci Coach. 16(3):701–709. doi: 10.1177/1747954120973628.
- Buysse DJ, Reynolds CF III, Monk TH, Berman SR, Kupfer DJ. 1989. The pittsburgh sleep quality index: a new instrument for psychiatric practice and research. Psychiatry Res. 28(2):193–213.
- Caia J, Thornton HR, Kelly VG, Scott TJ, Halson SL, Cupples B, Driller MW. 2018. Does self-perceived sleep reflect sleep estimated via activity monitors in professional rugby league athletes? J Sports Sci. 36(13):1492–1496. doi: 10.1080/02640414.2017.1398885.
- Caldwell AR. 2022. SimplyAgree: an R package and jamovi module for simplifying agreement and reliability analyses. J Open Source Softw. 7(71):4148. doi: 10.21105/joss.04148.
- Costa C, Teodoro M, Mento C, Giambò F, Vitello C, Italia S, Fenga C. 2022. Work performance, mood and sleep alterations in home office workers during the COVID-19 pandemic. Int J Envir Res Pub Health. 19(4):1990. doi: 10.3390/ijerph19041990.
- Craven J, McCartney D, Desbrow B, Sabapathy S, Bellinger P, Roberts L, Irwin C. 2022. Effects of acute sleep loss on physical performance: a systematic and meta-analytical review. Sport Med. 52(11):2669–2690. doi: 10.1007/s40279-022-01706-y.
- Davenne D. 2009. Sleep of athletes – problems and possible solutions. Biol Rhythm Res. 40(1):45–52. doi: 10.1080/09291010802067023.
- de Souza L, Benedito-Silva AA, Pires MLN, Poyares D, Tufik S, Calil HM. 2003. Further validation of actigraphy for sleep studies. Sleep. 26(1):81–85. doi: 10.1093/sleep/26.1.81.
- de Zambotti M, Claudatos S, Inkelis S, Colrain IM, Baker FC. 2015. Evaluation of a consumer fitness-tracking device to assess sleep in adults. Chronobiol Int. 32(7):1024–1028. doi: 10.3109/07420528.2015.1054395.
- Edwards BJ, Waterhouse J. 2009. Effects of one night of partial sleep deprivation upon diurnal rhythms of accuracy and consistency in throwing darts. Chronobiol Int. 26(4):756–768. doi: 10.1080/07420520902929037.
- Elliott-Sale KJ, Minahan CL, de Jonge XA, Ackerman KE, Sipilä S, Constantini NW, Lebrun CM, Hackney AC. 2021. Methodological considerations for studies in sport and exercise science with women as participants: a working guide for standards of practice for research on women. Sport Med. 51(5):843–861. doi: 10.1007/s40279-021-01435-8.
- Fullagar HH, Skorski S, Duffield R, Hammes D, Coutts AJ, Meyer T. 2015. Sleep and athletic performance: the effects of sleep loss on exercise performance, and physiological and cognitive responses to exercise. Sport Med. 45(2):161–186. doi: 10.1007/s40279-014-0260-0.
- Giavarina D. 2015. Understanding bland altman analysis. Biochem Med. 25(2):141–151. doi: 10.11613/BM.2015.015.
- Gupta L, Morgan K, Gilchrist S. 2017. Does elite sport degrade sleep quality? A systematic review. Sport Med. 47(7):1317–1333. doi: 10.1007/s40279-016-0650-6.
- Halson SL. 2013. Recovery techniques for athletes. Sports Sci Exch. 26(120):1–6.
- Halson SL. 2014. Sleep in elite athletes and nutritional interventions to enhance sleep. Sport Med. 44(1):13–23. doi: 10.1007/s40279-014-0147-0.
- Halson SL. 2019. Sleep monitoring in athletes: motivation, methods, miscalculations and why it matters. Sport Med. 49(10):1487–1497. doi: 10.1007/s40279-019-01119-4.
- Jackowska M, Ronaldson A, Brown J, Steptoe A. 2016. Biological and psychological correlates of self-reported and objective sleep measures. J Psychosom Res. 84:52–55. doi: 10.1016/j.jpsychores.2016.03.017.
- Juliff LE, Halson SL, Peiffer JJ. 2015. Understanding sleep disturbance in athletes prior to important competitions. J Sci Med Sport. 18(1):13–18. doi: 10.1016/j.jsams.2014.02.007.
- Kawasaki Y, Kasai T, Koikawa N, Hanazato N, Suda S, Murata A, Ozaki R, Nagai S, Matsumura Y, Kaneko H, et al. 2020. Sex differences in factors associated with poor subjective sleep quality in athletes. J Sport Med Phys Fit. 60(1):140–151. doi: 10.23736/S0022-4707.19.09875-X.
- Kölling S, Endler S, Ferrauti A, Meyer T, Kellmann M. 2016. Comparing subjective with objective sleep parameters via multisensory actigraphy in German physical education students. Behav Sleep Med. 14(4):389–405. doi: 10.1080/15402002.2015.1017096.
- Krause AJ, Simon EB, Mander BA, Greer SM, Saletin JM, Goldstein-Piekarski AN, Walker MP. 2017. The sleep-deprived human brain. Nat Rev Neurosci. 18(7):404–418. doi: 10.1038/nrn.2017.55.
- Kuosmanen E, Visuri A, Kheirinejad S, van Berkel N, Koskimäki H, Ferreira D, Hosio S. 2022. How does sleep tracking influence your life? Experiences from a longitudinal field study with a wearable ring. Proc ACM Hum Comput Interact. 6(MHCI):1–19. doi: 10.1145/3546720.
- Kushida CA, Chang A, Gadkary C, Guilleminault C, Carrillo O, Dement WC. 2001. Comparison of actigraphic, polysomnographic, and subjective assessment of sleep parameters in sleep-disordered patients. Sleep Med. 2(5):389–396. doi: 10.1016/S1389-9457(00)00098-8.
- Lu MJ, Zhong WH, Liu YX, Miao HZ, Li YC, Ji MH. 2016. Sample size for assessing agreement between two methods of measurement by Bland− altman method. Int J Biostat. 12(2). doi: 10.1515/ijb-2015-0039.
- Mah CD, Sparks AJ, Samaan MA, Souza RB, Luke A. 2019. Sleep restriction impairs maximal jump performance and joint coordination in elite athletes. J Sports Sci. 37(17):1981–1988. doi: 10.1080/02640414.2019.1612504.
- Meltzer L, Walsh C, Traylor J, Westin A. 2012. Direct comparison of two new actigraphs and polysomnography in children and adolescents. Sleep. 35:159–166. doi: 10.5665/sleep.1608.
- Morita Y, Sasai-Sakuma T. 2021. Optimal nocturnal sleep duration to maintain health-related quality of life in male collegiate athletes: a cross-sectional study. Res Square. 1–16.
- Nédélec M, McCall A, Carling C, Legall F, Berthoin S, Dupont G. 2012. Recovery in soccer. Sport Med. 42(12):997–1015. doi: 10.2165/11635270-000000000-00000.
- Ogeil RP, Nguyen MT, Savic M, Lubman DI. 2021. Assembling a ‘good’and ‘bad’night’s sleep: a multifactorial proposition. Lifestyle Med. 2(4):e48. doi: 10.1002/lim2.48.
- R Core Team. 2020. R: a language for statistical computing. Vienna, Austria: R Foundation for Statistical Computing.
- Reilly T, Piercy M. 1994. The effect of partial sleep deprivation on weight-lifting performance. Ergonomics. 37(1):107–115. doi: 10.1080/00140139408963628.
- Reyner LA, Horne JA. 2013. Sleep restriction and serving accuracy in performance tennis players, and effects of caffeine. Physiol Behav. 120:93–96. doi: 10.1016/j.physbeh.2013.07.002.
- Rupp TL, Balkin TJ. 2011. Comparison of motionlogger watch and actiwatch actigraphs to polysomnography for sleep/wake estimation in healthy young adults. Behav Res Methods. 43(4):1152–1160. doi: 10.3758/s13428-011-0098-4.
- Sadeh A, Sharkey M, Carskadon MA. 1994. Activity-based sleep-wake identification: an empirical test of methodological issues. Sleep. 17(3):201–207. doi: 10.1093/sleep/17.3.201.
- Samuels C. 2008. Sleep, recovery, and performance: the new frontier in high-performance athletics. Neurol Clin. 26(1):169–180. doi: 10.1016/j.ncl.2007.11.012.
- Sargent C, Lastella M, Halson SL, Roach GD. 2016. The validity of activity monitors for measuring sleep in elite athletes. J Sci Med Sport. 19(10):848–853. doi: 10.1016/j.jsams.2015.12.007.
- Sargent C, Lastella M, Halson SL, Roach GD. 2021. How much sleep does an elite athlete need? Int J Sport Physiol. 16(12):1746–1757. doi: 10.1123/ijspp.2020-0896.
- Saw AE, Main LC, Gastin PB. 2015. Monitoring athletes through self-report: factors influencing implementation. J Sports Sci Med. 14(1):137.
- Saw AE, Main LC, Gastin PB. 2016. Monitoring the athlete training response: subjective self-reported measures trump commonly used objective measures: a systematic review. Br J Sports Med. 50(5):281–291. doi: 10.1136/bjsports-2015-094758.
- Sinnerton SA, Reilly T. 2013. Effects of sleep loss and time of day in swimmers. In: Biomechanics and medicine in swimming V1. London, UK: Taylor & Francis; p. 347–351.
- Skein M, Duffield R, Edge J, Short MJ, Muendel T. 2011. Intermittent-sprint performance and muscle glycogen after 30 h of sleep deprivation. Med Sci Sport Exerc. 43(7):1301–1311. doi: 10.1249/MSS.0b013e31820abc5a.
- Skein M, Duffield R, Minett GM, Snape A, Murphy A. 2013. The effect of overnight sleep deprivation after competitive rugby league matches on postmatch physiological and perceptual recovery. Int J Sport Physiol. 8(5):556–564. doi: 10.1123/ijspp.8.5.556.
- Souissi N, Chtourou H, Aloui A, Hammouda O, Dogui M, Chaouachi A, Chamari K. 2013. Effects of time-of-day and partial sleep deprivation on short-term maximal performances of judo competitors. J Strength Cond Res. 27(9):2473–2480. doi: 10.1519/JSC.0b013e31827f4792.
- Stone AA, Bachrach CA, Jobe JB, Kurtzman HS, Cain VS, eds. 1999. The science of self-report: implications for research and practice. London, UK: Psychology Press.
- Swinbourne R, Gill N, Vaile J, Smart D. 2016. Prevalence of poor sleep quality, sleepiness and obstructive sleep apnoea risk factors in athletes. Eur J Sport Sci. 16(7):850–858. doi: 10.1080/17461391.2015.1120781.
- Taylor K, Chapman D, Cronin J, Newton M, Gill N. 2012. Fatigue monitoring in high performance sport: a survey of current trends. J Aust Strength Cond. 20(1):12–23.
- Underwood J. 2010. Sleep now clearly a predictor of performance. Coaches Plan. 17:31–34.
- Van De Water AT, Holmes A, Hurley DA. 2011. Objective measurements of sleep for non‐laboratory settings as alternatives to polysomnography–a systematic review. J Sleep Res. 20(1pt2):183–200. doi: 10.1111/j.1365-2869.2009.00814.x.
- Vlahoyiannis A, Aphamis G, Bogdanis GC, Sakkas GK, Andreou E, Giannaki CD. 2021. Deconstructing athletes’ sleep: a systematic review of the influence of age, sex, athletic expertise, sport type, and season on sleep characteristics. J Sport Health Sci. 10(4):387–402. doi: 10.1016/j.jshs.2020.03.006.
- Walsh NP, Halson SL, Sargent C, Roach GD, Nédélec M, Gupta L, Leeder J, Fullagar HH, Coutts AJ, Edwards BJ, et al. 2021. Sleep and the athlete: narrative review and 2021 expert consensus recommendations. Br J Sports Med. 55(7):356–368. doi:10.1136/bjsports-2020-102025.
- Werner H, Molinari L, Guyer C, Jenni OG. 2008. Agreement rates between actigraphy, diary, and questionnaire for children’s sleep patterns. Arch Pediatr Adolesc Med. 162(4):350–358. doi: 10.1001/archpedi.162.4.350.
- Zhang B, Bender A, Tan X, Wang X, Le S, Cheng S. 2022. Assessment of sleep disturbances with the Athlete sleep screening questionnaire in Chinese athletes. Sports Med Health Sci. 4(2):133–139. doi: 10.1016/j.smhs.2022.02.001.
- Zou GY. 2013. Confidence interval estimation for the bland–altman limits of agreement with multiple observations per individual. Stat Methods Med Res. 22(6):630–642.