Abstract
RATIONALE
Recurrent tuberculosis (TB) accounts for 5% of the Canadian TB burden. Recurrence can occur from relapse or reinfection. Identifying reinfection has implications for developing control policies.
OBJECTIVE
The objectives of this study were to quantify reinfection in recurrent TB using whole genome phylogenetic and single nucleotide polymorphism (SNP) and compare with epidemiological and clinical parameters from the Canadian prairies.
METHODS
DNA sequences of Mycobacterium tuberculosis isolates from recurrent TB cases along with epidemiological and clinical parameters were collected from Alberta and Saskatchewan. Inclusion criteria were two or more culture positive notifications age ≥17 years. SNP and phylogenetic tree scale differences (TIP) were used to determine the relapse and reinfection categories.
RESULTS
Of 7,627 notifications of TB disease, 533 were recurrent. 93 pairs (180 cases) were culture positive from which 26 (50 cases) were available for sequencing. 19 cases with SNP and TIP values ≤25 and ≤.001 were classified relapse. Seven SNP and TIP values >160 and >.001 were classified reinfection. Five of seven reinfections were Indigenous cases from high TB incidence areas. The non-sequenced and sequenced pairs differed only in age.
CONCLUSIONS
Recurrent culture positive TB is uncommon on the Canadian prairies and is more likely to be relapse. Reinfection is more likely in Indigenous persons living in high TB incidence communities. A limitation was the risk of selection bias since only 28% of eligible cases were sequenced. Since we did not know the non-sequenced reinfection risk, we could only conclude that the non-sequenced and sequenced categories were similar.
RÉSUMÉ
JUSTIFICATION:
La tuberculose récurrente représente 5 % du fardeau de la tuberculose au Canada. La récurrence peut résulter d'une rechute ou d’une réinfection. La détection de la réinfection a des implications pour l’élaboration de politiques de lutte contre la tuberculose.
OBJECTIF:
Quantifier les cas de réinfection dans la tuberculose récurrente à l’aide de la phylogénétique du génome entier et du polymorphisme d’un seul nucléotide (PSN), et la comparer aux paramètres épidémiologiques et cliniques des Prairies canadiennes.
MÉTHODES:
Des séquences d’ADN d’isolats de Mycobacterium tuberculosis provenant de cas de tuberculose récurrente, ainsi que des paramètres épidémiologiques et cliniques, ont été recueillis en Alberta et en Saskatchewan. Les critères d’inclusion étaient la notification de deux cultures positives ou plus, et un âge ≥17 ans. Les polymorphismes d’un seul nucléotide et les distances phylogénétiques ont été utilisés pour déterminer les catégories de rechute et de réinfection.
RÉSULTATS:
Sur 7 627 notifications de tuberculose, 533 cas étaient récurrents. 93 paires (180 cas) étaient positives à la culture, dont 26 (50 cas) étaient disponibles pour le séquençage. 19 cas avec des valeurs de polymorphisme d’un seul nucléotide et de ≤25 et ≤.001 ont été classés en rechute. Sept cas avec des valeurs de polymorphisme d’un seul nucléotide et de distance phylogénétique >160 et > 0,001 ont été classés comme une réinfection. Cinq des sept réinfections étaient des cas autochtones provenant de zones à forte incidence de tuberculose. Les paires non séquencées et séquencées ne différaient que par l'âge.
CONCLUSIONS:
La tuberculose récurrente à culture positive est peu fréquente dans les Prairies canadiennes et est plus susceptible d'être une rechute. La réinfection est plus probable chez les autochtones vivant dans des communautés à forte incidence de tuberculose. Le risque de biais de sélection constitue une limite puisque seulement 28 % des cas admissibles ont été séquencés. Comme nous ne connaissions pas le risque de réinfection en l'absence de séquençage, nous ne pouvions que conclure que les catégories non séquencées et séquencées étaient similaires.
Introduction
Tuberculosis disease, caused by M. tuberculosis (TB), resulted in 6.4 million cases and 1.6 million deaths globally in 2021.Citation1 Canada reported 1,796 new and recurrentCitation2 cases (definitions in Appendix A1 of the Online Supplementary Material) in 2017,Citation3 the last year for which statistics were available; 1,290 (71.8%) cases originated outside Canada and 95 (5.3%) were recurrent. Furthermore, 148 of 1,459 TB isolates were resistant to one or more first line drugs.Citation4 Recurrent disease contributes to ongoing transmission of infection and is more likely to be drug resistant.Citation5 Drug resistance further contributes to transmission of infection, increased duration and cost of treatment. Both are significant factors that affect the successful control of TB disease.Citation4–7
Recurrent disease can be classified as relapse or reinfection (Appendix A1 of the Online Supplementary Material).Citation2,Citation8–10 However, differentiating relapse from reinfection is a challenge without genotyping or genomic analysis.Citation8,Citation9 Factors associated with relapse include incomplete treatment, drug resistance, and lineage.Citation7,Citation8,Citation11 Factors associated with reinfection include resident in area of high incidence TB, (Appendix A1 of the Online Supplementary Material) living in crowded conditions, and contact with infectious TB (Appendix A1 of the Online Supplementary Material).Citation8,Citation11 An added complexity for identifying reinfection is mixed infection which may be uncharacterized due to limited samplingCitation9 and occurs in similar frequency and similar conditions as reinfectionCitation9,Citation11,Citation12 (Appendix A1 of the Online Supplementary Material) The proportion of mixed infections was reported to account for up to 20% of pulmonary TB (PTB) and up to 51% of extrapulmonary TB (EPTB).Citation9 Differentiating mixed infection from reinfection is important. It is also important to differentiate relapse from reinfection in developing effective control strategies, especially the resource intensive search for the source of the reinfection.Citation7,Citation8
Whole-genome sequencing (WGS) has been used to investigate drug resistant mutations, transmission, genomic evolution, social-networks, and distinguishing reinfection from relapse.Citation11–18 WGS has a higher resolution than genotyping methods for M. tuberculosis, such as restriction fragment length polymorphism (RFLP), mycobacterial interspersed repetitive unit–variable number tandem repeat (MIRU-VNTR) and spacer oligonucleotide genotyping (spoligotyping).Citation11,Citation12,Citation15 The proportion of reinfection in recurrent cases of TB varied from 5% in HIV negative cases in India,Citation15 to 9% in Beijing,Citation19 to 25% in Hunan province China,Citation20 to 27% in MalawiCitation8 and 75% in HIV positive cases in India.Citation15 Reinfection is important since it implies new transmission events, particularly when the source case is drug resistant.Citation5,Citation6
The Canadian Tuberculosis Standards (CTBS)Citation21 state that repeated exposure is rare in low incidence areas such that active TB usually results from a first infection. However, it has been shown that reinfection occurs in high-incidence, high-transmission areas. Our objective was to identify the contribution of relapse and reinfection TB disease in high and low incidence settings in the Canadian prairies and compare WGS analysis with epidemiological and clinical data.
Materials and methods
Study participants and epidemiology
This was a retrospective study of recurrent TB. Inclusion criteria for WGS were two or more notifications of culture positive TB, age 17 years and older, and diagnosed and treated in Alberta and Saskatchewan. Not all isolates were sequenced. Isolates that were not found or could not be regrown were not sequenced. The majority had one recurrence while some had two recurrences: the first notification was episode 1, the second was episode 2 and the third was episode 3. Epidemiological and clinical data – diagnosis date, age, sex, population group, country of origin, domicile area, site of TB, contacts that included travel to endemic countries, community TB incidence, treatment completion, sensitivity tests and episode interval were obtained from the TB program for each province.
Reinfection risk score
Relapse factors comprised incomplete treatment and drug resistance, both for the first episode. Reinfection factors comprised identified contact(s) and living in a high-incidence community at the time of notification (>30/100,000), both for the second episode. A reinfection score was developed for each case based on the risk factors for reinfection: that is, +1 for a high-incidence community, +1 for a contact, −1 for incomplete treatment, −1 for drug resistance and 0 for HIV coinfection, diabetes, silicosis, smoking, air pollution and unavailable data.Citation11 Zero was scored since these comorbidities were associated with both relapse and reinfection.Citation11
Mycobacterial culture and DNA extraction
The details are described in Appendix A2 of the Online Supplementary Material.
Whole genome sequencing
Genome Quebec prepared the libraries using Illumina Truseq Prep Kit and performed on an Ultra II Illumina NovaSeq 6000 S4 sequencer (300 cycles) that generated 2 × 150 bp paired end reads. The defined coverage depth was ≥100x. The required minimum total reads were 1.5 M.
Bioinformatics data analysis
Coverage was calculated with fastq-info v2.0 with H37Rv (accession no: NC 000962.3) as a reference genomeCitation22 based on the Lander Waterman equation.Citation23 For each sample, raw read-pairs were subjected to quality control using Fastqc (v0.11.9).Citation24 The Illumina universal adapters were present in one sample and removed using Trimmomatic (with ILLUMINACLIP option).Citation25 WGS raw reads and trimmed reads were aligned to the reference genome M. tuberculosis H37Rv;Citation26 using the read mapping function (bowtie v 2-2.4.2)Citation27 implemented in Gen2Epi.Citation28 De novo assemblies were generated using SPAdes (v 3.15.1)Citation29 implemented in Gen2EpiCitation28 (with parameters –cov-cutoff auto –careful –kmer 21,33,55,71). The quality of the assembled contigs was checked with QUAST (v 5.0.2)Citation30 using M. tuberculosis H37Rv as a referenceCitation26 (Genbank accession number [annotation number]: NC_000962.3) and its respective annotation. The mean genome fraction covered by contigs was 98.3%. Annotation of the de novo assembled contigs was performed using ProkkaCitation31 (with parameter “-compliant” using a minimum contig length of ≥200 bp for gene prediction and product name assignment). Lineage classification and drug resistance prediction was performed using Mykrobe version 0.10.0.Citation32
Single nucleotide polymorphism and phylogenetic analysis
Variant calling and pairwise comparison of SNPs between samples were determined by uploading the cleaned WGS reads to the MTBseq pipeline version 1.0.3Citation33 using Mycobacterium tuberculosis strains H37Rv (Genbank accession number: NC_000962.3) as reference.Citation26 Variants were filtered automatically based on repetitive regions (including the polymorphic PPE/PE regionsCitation34 and regions encoding for phage proteins), genes associated with resistance and SNPs within a window of 12 bp within the same subsets.Citation33 For variance prediction, MTBseq pipeline used the default parameters: minimum coverage 4 forward reads, 4 reverse reads, and a minimum Phred score ≥20. At the default setting, MTBseq reliably detected variants if they were present in ≥75% allele frequency in the bacterial population.Citation33,Citation35 The minimum coverage to call a variant was 8x. The consensus fastq file for the filtered variants was used for phylogenetic analysis. The phylogenetic tree was generated using RAxML version 8 using the GTRGAMMA model with 200 bootstrap replications.Citation36 The output tree was visualized using iTOL.Citation37
Threshold determination to identify relapse and reinfection
A numeric table was constructed summing the branch length of each case and episode to calculate the value of the final horizontal position on the phylogenetic tree scale, (ie, tree tip [TIP]), . The TIP difference between episodes was determined as a positive value, Online Supplementary Table S1. This value was compared to the SNP results for each pair. Two methods for evolutionary distance were used to delineate similar and unique samples: SNPs based on MTBseq pipeline, and the phylogenetic tree scale based on maximum likelihood RAxML software. They are two methods for identifying relatedness using the same data with different algorithms.Citation38,Citation39
Figure 1. Phylogenetic tree scale.
Unrooted tree scale that measures genetic distance between 50 genomes comprised of 22 pairs and two triplets. Genomes are listed by CaseNo., episode and lineage. The horizontal axis shows the scale representing the number of single nucleotide polymorphism (SNP)s divided by the sequence length; 0.100 is equivalent to 10%. CaseNo. 1, 9, 11, 14, 20, 24 (all episode 2) and 11 (episode 3) had SNPs >160 whose final tree scale positions were different from Episode 1 and 2. They were all lineage 4. The horizontal final position can also be obtained from Online Supplementary Table S1.
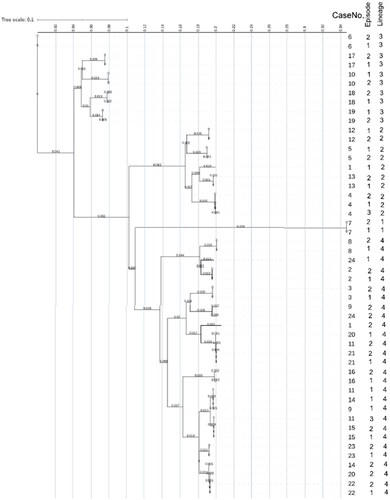
Statistical analysis and ethics
Statistical analysis was performed with SPSS v28.0.1.0. Fisher exact test was conducted using a two-sided alpha level 0.05. Ethics approval was granted by University of Saskatchewan REB Bio-782 and by the University of Alberta REB Pro00088456.
Results
Epidemiology and clinical parameters
Alberta and Saskatchewan reported 7,627 new and recurrent cases from 1989 to 2018. A total of 533 were recurrent, of which 93 pairs (180 cases) were culture positive. The remaining 353 cases were not culture confirmed, . Twenty-six pairs (50 cases) were sequenced, comprised of 13 foreign-born, 12 Indigenous, and one non-Indigenous cases. Indigenous and non-Indigenous cases were all lineage 4 compared to foreign born cases that were lineage 1 to 4, . Sixty-seven pairs (130 cases) that were not sequenced were comprised of one foreign-born, 65 Indigenous, and one non-Indigenous cases (Online Supplementary Table S2). The non-sequenced pairs were younger, mostly Indigenous who lived in remote, high TB rate communities, with longer recurrence intervals. Online Supplementary Table S3 compares the same parameters for Indigenous non-sequenced with sequenced pairs. The not sequenced cases were younger. The remaining nine parameters of interest were not significantly different.
Figure 2. Distribution of reported tuberculosis (TB) cases 1989–2018.
Shows the distribution for all reported TB cases 1989 to 2018 by step to the number of sequenced cases. A total of 67 culture positive recurrent pairs were not sequenced because they could not be found or could not be reconstituted, 26 pairs were sequenced and 67 pairs were not sequenced.
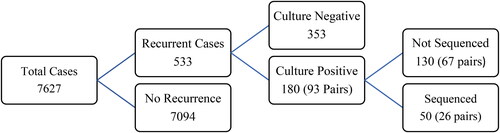
SNP and phylogenetic analysis
The samples where sequence reads percent aligned to H37Rv of two samples was <55% were removed from further analysis. The remaining 50 samples percentage aligned to H37Rv, which was ≥90% (Online Supplementary Table S4). The coverage depth ranged from 34 to 62 M reads. The depth per sample was ≥100x (Online Supplementary Table S4). Total number of reads was 2,225 M. For the 26 pairs (50 genomes) analyzed, SNPs ranged from 0 to 1,116 () and the TIP differences ranged from 0.000 to 0.007 (). shows that SNPs ≤ 25 and TIPs ≤ .001 were concordant when identifying relapse and SNPs > 160 and TIPs > .001 were concordant identifying reinfection. Based on the SNP and phylogenetic tree analysis, 19 recurrent episodes were classified relapses and seven were classified reinfections, a 26.9% prevalence. Relapse cases comprised lineages 1, 2, 3 and 4 while reinfection episodes comprised only lineage 4 ().
Table 1. Paired SNP matrix for 26 recurrent TB cases.
Table 2. Paired phylogenetic TIP difference matrix for 26 recurrent TB cases.
Table 3. Determining relapse and reinfection combining SNPs and TIPs.
Clinical reinfection risk score
The reinfection risk score, SNP and TIP results are listed in . The best fit threshold for reinfection was defined as a risk score >1 and for relapse ≤1. A sensitivity analysis comparing the reinfection score to the SNP standard showed that the reinfection score specificity was 0.842 and the negative predictive value was 0.882. Six of seven reinfections occurred in Indigenous persons, of which five were resident in high TB incidence communities. Reinfection cases were associated with Indigenous population, lineage 4, contact with TB, living in high TB incidence community and reinfection risk score >1 (). Multivariate logistic regression analysis was not significant.
Table 4. Reinfection risk score.
Table 5. Epidemiological and clinical comparison of relapse and reinfection cases.
Phenotypic resistance and genotypic mutations
Phenotypic drug resistance was reported in six, and drug sensitive in 44 of 50 isolates (Online Supplementary Table S5). Three episode 2 isolates, two rifampicin and one isoniazid acquired drug resistance. Genotypic resistant mutations were observed in four and zero mutations in 46 of 50 genomes. Case 8 showed phenotypic INH resistance and Case 19 phenotypic streptomycin resistance. Both were genetically not predicted to be resistant to these agents.
Discussion
The main finding was the 19 cases classified as relapse and 7 cases classified as reinfection. The reinfection cases were likely to be Indigenous and resident in remote high TB incidence communities. The significant reinfection risk factors for this culture positive recurrent TB cohort were Indigenous ethnicity, lineage 4, contact with TB, living in high TB incidence community and reinfection risk score >1. Although the reinfection burden was low, false positive reinfections have program implications resulting from new infection follow-up policy.
Our data delineated cases of relapse and reinfection. We used two methods, SNP and phylogenetic tree TIP differences, to determine relapse and reinfection. There were discordant results between SNP and TIP thresholds. By removing the discordant thresholds, relapse and reinfection were identified to the exclusion of all other cases. Because there were no SNPs between 25 and 161, a SNP threshold could not be defined in this population since the intermediate values were indeterminate.Citation40 More studies that include intermediate SNP values between 25 and 161 need to be done in this population to define the threshold.
In a review of published reports that used SNPs to identify TB genomes, the lower SNP thresholds below which cases were considered relapse, varied from <2 to <50 and an upper threshold from >12 to >1,300, above which cases were considered reinfection.Citation40 Both categories were within the range of previous reports.Citation40 The epidemiological context in which the SNP thresholds were applied to delineate different genomes, differed from study to study. They were different populations with different objectives, with variable HIV infection prevalence, different definitions of recurrent TB (episodes intervals between 17 wk to greater than one year, ie, they included recurrent intervals consistent with treatment failure).Citation8,Citation12,Citation20,Citation41,Citation42 The results were not comparable between studies and showed a wide range of SNPs that could reasonably be applied.Citation40 Strict SNP thresholds to infer transmission may not be universally valid.Citation43 SNP thresholds are also thought to be influenced by mutation rates that ranged from 0 to 17 SNPs/genome/year with means of 0.3 to 5.37.Citation19,Citation41,Citation44,Citation45 One review concluded that SNPs varied by lineageCitation46 and could not be reliably calculated for intervals less than 15 years.Citation46 These differences were most likely the result of different assumptions and methods.Citation46 In another review, the molecular clock data did not show a steady mutation rate/year and was thought to be dependent on patient characteristics (age, country of origin, disease site, drug resistance, treatment outcome), treatment regimen, treatment compliance and strain type or lineage.Citation44
SNPs may also arise from mixed TB infection, the prevalence of which was reported to be as high as 20% in PTB casesCitation9,Citation47 and 51% in EPTB cases.Citation9 Mixed infections occurred in the same settings in which reinfections occurred, mostly high-incidence communities.Citation12 Moreover, they were demonstrated in postmortem TB cases dated as early as eighteenth century Hungary, during the peak TB wave in Europe.Citation47 In our study, the clinical assessment for Case 1 was mixed infection at the time of the second episode. Episode 1 showed INH and streptomycin resistance while episode 2 showed fully sensitive organisms, with no history of contact or travel to an endemic area. The left upper lobe was destroyed during the initial episode. TB organisms have a propensity to recur in damaged tissue,Citation11 with a four-fold increased risk following residual lung cavitation,Citation48 suggesting that persistence of the organism is enhanced. Mixed infection connotes infection with more than one strain. Recurrence of TB with a strain that was initially present after completed treatment is the definition of relapse.Citation2 Genomic assessment of Case 11 also raised the possibility of mixed infection. Episodes 1 and 2 and 2 and 3 differed by 603 and 607 SNPs, respectively. Episode 1 and 3 differed by 44 SNPs and a TIP difference of 0.001, that is, they were similar genomes as defined for this cohort. Case 11 was an Indigenous resident in a high TB incidence community, a setting in which mixed infections have been reported.Citation12 It was possible that the episode 2 genome was part of the initial infection. Since mixed infection analysis was not included in the protocol, both cases could not be verified as mixed infection.
The reinfection risk score was based on a history of TB contact, resident in a high TB incidence community, treatment completion and drug sensitivity. Compared to the SNP standard, the reinfection risk score ≤1 had a specificity of 0.84. It meant that the reinfection risk score correctly classified recurrent TB as relapse 84% of the time. The negative predictive value was 0.88. It meant that the reinfection risk score <1 correctly identified a relapse case 88% of the time. Due to the small number of cases and possible selection bias, these results may not be representative of all recurrent cases and cannot be recommended as a proxy for sequencing.
An incidental finding was the discordance between phenotypic resistance and genotypic analysis for INH and streptomycin. Discordance with INH and other drugs has been previously reported.Citation49–52 Other reports noted an association between drug resistance and lineage 2.Citation53,Citation54 Our study noted this association as well. Notably, we observed three cases of acquired drug resistance. This suggested that drug adherence played a role in phenotypic drug resistance.Citation55
There were limitations in this study. The main limitation was that 67 of 93 culture positive pairs were not sequenced. This represented a risk of selection bias. Since only two non-Indigenous pairs were not sequenced, the risk arose from the 65 Indigenous pairs that were not sequenced (Online Supplementary Table S4). In the ten parameters of interest that are listed in Online Supplementary Table S4, nine were not significantly different from the sequenced pairs. The difference was the non-sequenced cases were younger. shows that age was not associated with reinfection. The reinfection risk of the 65 not sequenced pairs was not available.
Another limitation was mixed infection that we did not identify given that it was not part of the protocol. Since mixed infections occur in the same settings as reinfections,Citation12 it was possible that some cases that were classified as reinfection were mixed infections. Assessment of two cases indicate that this might have been possible. This emphasizes the challenge in attempting to infer disease transmission. Unidentified mixed infection might have overestimated reinfection. A third limitation was the omission of clinical data such as sputum quality or delay in processingCitation9 and the size of the mycobacterial population (extent of disease).Citation40,Citation48
In summary, recurrent culture positive TB is uncommon on the Canadian prairies and more likely to be relapse than reinfection. Reinfection is more likely to occur in Indigenous persons living in remote high TB incidence communities. WGS might have overestimated the reinfection rate since the protocol did not include mixed infection. A limitation was the risk of selection bias since only 28% of eligible cases were sequenced.
Author contributions
R. Singh, J.R. Dillon and M.K. Sharma were responsible for the study design, analysis, interpretation, drafting, and critical review of the manuscript. F. Jamieson, R. Long and M. Richard-Greenblatt were responsible for acquisition data, analysis, interpretation, and critical review of the manuscript. I. Khan and G.J. Tyrrell were responsible for acquisition data, interpretation and critical review of the manuscript. R. McDonald, J. Minion and S. Shokoples were responsible for acquisition data, data analysis and critical review of the manuscript. E. Rea was responsible for data analysis, interpretation and critical review of the manuscript. V. Hoeppner was responsible for concept, study design, acquisition data, data analysis, interpretation, drafting and critical review of the manuscript. W. Wobeser was responsible for concept, study design, data acquisition, data analysis, interpretation and critical review manuscript.
All authors approved the final draft and agreed to accountability for all aspects.
Supplemental Material
Download MS Word (31.5 KB)Supplemental Material
Download MS Word (53.4 KB)Acknowledgments
The authors thank the following for their contribution to grant application: Donna Goodridge PhD, Dept. Med, University of Saskatchewan; Ida Lemaigre, Indigenous Community Representative; Kathleen McMullin MEd, Dept. Comm Health and Epi, University of Saskatchewan; Michael Patterson MD, Chief Public Health Officer Nunavut; Lisa Puchalski Ritchie MD PhD, Dept. Clin Epidemiol and Health Care Research, University of Toronto; Keith Travers MSc, Dept. Health and Soc Services, Govt. Nunavut. The authors also wish to thank Jennifer Guthrie PhD, Dept. Microbiol and Immunol, University Western Ontario, for critical review of the manuscript and Josh Lawson PhD, Canadian Centre for Health and Safety in Agriculture, University of Saskatchewan for critical review of the data and interpretation. We are pleased to acknowledge the support of this manuscript by the director of VIDO-InterVac as journal series no. 1043.
Disclosure statement
No potential conflict of interest was reported by the author(s).
Additional information
Funding
References
- World Health Organization. Global Tuberculosis Report. 2021. http://Users/vhh783/Downloads/9789240061729-eng.pdf.
- Glossary of terms. Can J Respir Crit Care Sleep Med. 2022. 6:sup1, 242–247. doi:10.1080/24754332.2022.2045118.
- LaFreniere M, Hussain H, He N, McGuire M. Tuberculosis in Canada: 2017. Can Commun Dis Rep. 2019;45(2-3):67–74. doi:10.14745/ccdr.v45i23a04.
- LaFreniere M, Dam D, Strudwick L, McDermott S. Tuberculosis drug resistance in Canada: 2018. Can Commun Dis Rep. 2020;46(1):9–15. doi:10.14745/ccdr.v46i01a02.
- Johnston J, Cooper R, Menzies D. Chapter 5: Treatment of tuberculosis disease. Can J Respir Crit Care Sleep Med. 2022;6(S1):66–76. doi:10.1080/24745332.2022.2036504.
- Gygli SM, Borrell S, Trauner A, Gagneux S. Antimicrobial resistance in Mycobacterium tuberculosis: mechanistic and evolutionary perspectives. FEMS Microbiol Rev. 2017;41(3):354–373. doi:10.1093/femsre/fux011.
- Chaisson RE, Churchyard GJ. Recurrent tuberculosis – relapse, reinfection, and HIV. J Infect Dis. 2010;201(5):653–655. doi:10.1086/650531.
- Guerra-Assunção JA, Houben RM, Crampin AC, et al. Recurrence due to relapse or reinfection with Mycobacterium tuberculosis: a whole-genome sequencing approach in a large, population-based cohort with a high HIV infection prevalence and active follow-up. J Infect Dis. 2015;211(7):1154–1163. doi:10.1093/infdis/jiu574.
- McIvor A, Koornhof H, Kana BD. Relapse, re-infection and mixed infections in tuberculosis disease. Pathog Dis. 2017;75(3):20. doi:10.1093/femspd/ftx020.
- Jasmer RM, Bozeman L, Schwartzman K, et al. Recurrent tuberculosis in the United States and Canada. Am J Respir Crit Care Sleep Med. 2004;170(12):1360–1366.
- Naidoo K, Dookie N. 2018. Insights into recurrent tuberculosis: relapse versus reinfection and related risk factors. In Tuberculosis 2018 Sep 26. IntechOpen. doi:10.5772/intechopen.73601.
- Bryant JM, Harris SR, Parkhill J, et al. Whole-genome sequencing to establish relapse or re-infection with Mycobacterium tuberculosis: a retrospective observational study. Lancet Respir Med. 2013;1(10):786–792. doi:10.1016/S2213-2600(13)79231-5.
- Advani J, Verma R, Chatterjee O, et al. Whole genome sequencing of Mycobacterium tuberculosis clinical isolates from India reveals genetic heterogeneity and region-specific variations that might affect drug susceptibility. Front Microbiol. 2019;10:309. doi:10.3389/fmicb.2019.00309.
- Gautam SS, Mac Aogáin M, Cooley LA, et al. Molecular epidemiology of tuberculosis in Tasmania and genomic characterisation of its first known multi-drug resistant case. PLoS One. 2018;13(2):e0192351. doi:10.1371/journal.pone.0192351.
- Shanmugam S, Bachmann NL, Martinex E, et al. Whole genome sequencing base differentiation between re-infection and relapse in Indian patients with tuberculosis recurrence, with and without HIV co-infection. Int J Infect Dis. 2021;13(S1):s43–s47. Accessed June 15, 2023
- Gardy JL, Johnston JC, Sui SJ, et al. Whole-genome sequencing, and social-network analysis of a tuberculosis outbreak. N Engl J Med. 2011;364(8):730–739. doi:10.1056/NEJMoa1003176.
- Homolka S, Projahn M, Feuerriegel S, et al. High resolution discrimination of clinical Mycobacterium tuberculosis complex strains based on single nucleotide polymorphisms. PLoS One. 2012;7(7):e39855. doi:10.1371/journal.pone.0039855.
- Parvaresh L, Crighton T, Martinez E, Bustamante A, Chen S, Sintchenko V. Recurrence of tuberculosis in a low incidence setting: a retrospective cross-sectional study augmented by whole genome sequencing. BMC Infect Dis. 2018;18(1):265. doi:10.1186/s12879-018-3164-z.
- Du J, Li Q, Liu M, et al. Distinguishing relapse from Reinfection with whole-genome sequencing in recurrent pulmonary tuberculosis: a retrospective cohort study in Beijing. Front Microbiol. 2021;12:754352. doi:10.3389/fmicb.2021.754352.
- He W, Tan Y, Song Z, et al. Endogenous relapse and exogenous reinfection in recurrent pulmonary tuberculosis: a retrospective study revealed by whole genome sequencing. Front Microbiol. 2023;14:1115295. doi:10.3389/fmicb.2023.1115295.
- Long R, Divangahi M, Schwartzman K. Chapter 2: Transmission and pathogenesis of tuberculosis. Can J Respir Crit Care Sleep Med. 2022;6(S1):22–32. doi:10.1080/24745332.2022.2035540.
- Kiu R. Compute estimated sequencing depth/coverage of genomes. https://github.com/raymondkiu/fastq-info. v2.0, 2020.
- Port E, Sun F, Martin D, Waterman MS. Genomic mapping by fingerprinting random clones: a mathematical analysis. Genomics. 1988;26(1):84–100. doi:10.1016/0888-7543(88)90007-9.
- Fastqc v0.11.9. https://www.bioinformatics.babraham.ac.uk/projects/fastqc/. Accessed June 14, 2023.
- Bolger AM, Lohse M, Usadel B. Trimmomatic: a flexible trimmer for Illumina sequence data. Bioinformatics. 2014;30(15):2114–2120. doi:10.1093/bioinformatics/btu170.
- Cole ST. Learning from the genome sequence of Mycobacterium tuberculosis H37Rv. FEBS Lett. 1999;452(1-2):7–10. doi:10.1016/s0014-5793(99)00536-0.
- Langmead B. Aligning short sequencing reads with Bowtie. Current protocols. Bioinformatics. 2010;32(1):11–17.
- Singh R, Dillon JR, Demczuk W, Kusalik A. Gen2Epi: an automated whole-genome sequencing pipeline for linking full genomes to antimicrobial susceptibility and molecular epidemiological data in Neisseria gonorrhoeae. BMC Genomics. 2019;20(1):165. doi:10.1186/s12864-019-5542-3.
- Bankevich A, Nurk S, Antipov D, et al. SPAdes: a new genome assembly algorithm and its applications to single-cell sequencing. J Comput Biol. 2012;19(5):455–477.
- Gurevich A, Saveliev V, Vyahhi N, Tesler G. QUAST: quality assessment tool for genome assemblies. Bioinformatics. 2013;29(8):1072–1075. doi:10.1093/bioinformatics/btt086.
- Seemann T. Prokka: rapid prokaryotic genome annotation. Bioinformatics. 2014;30(14):2068–2069. doi:10.1093/bioinformatics/btu153.
- Hunt M, Bradley P, Lapierre SG, et al. Antibiotic resistance prediction for Mycobacterium tuberculosis from genome sequence data with Mykrobe. Wellcome Open Res. 2019;4:191. doi:10.12688/wellcomeopenres.15603.1.
- Kohl TA, Utpatel C, Schleusener V, et al. MTBseq: a comprehensive pipeline for whole genome sequence analysis of Mycobacterium tuberculosis complex isolates. PeerJ. 2018;6:e5895. doi:10.7717/peerj.5895.
- Phelan JE, Coll F, Bergval I, et al. Recombination in ppe/pe genes contributes to genetic variation in Mycobacterium tuberculosis lineages. BMC Genomics. 2016;17(1):151. doi:10.1186/s12864-016-2467-y.
- Jajou R, Kohl TA, Walker T, et al. Towards standardisation: comparison of five whole genome sequencing (WGS) analysis pipelines for detection of epidemiologically linked tuberculosis cases. Euro Surveill. 2019;24(50):pii=1900130. doi:10.2807/1560-7917.ES.2019.24.50.1900130.
- Stamatakis A. RAxML version 8: a tool for phylogenetic analysis and post-analysis of large phylogenies. Bioinformatics. 2014;30(9):1312–1313. doi:10.1093/bioinformatics/btu033.
- Letunic I, Bork P. Interactive Tree Of Life (iTOL) v5: an online tool for phylogenetic tree display and annotation. Nucleic Acids Res. 2021;49(W1):W293–W296. doi:10.1093/nar/gkab301.
- Denzin NK. Triangulation 2.0. J Mixed Methods Res. 2012;6(2):80–88.
- Fusch P, Fusch GE, Ness LR. Denzin’s paradigm shift: revisiting triangulation in qualitative research. JOSC. 2018;10(1):19–32. doi:10.5590/JOSC.2018.10.1.02.
- Stimson J, Gardy J, Mathema B, Crudu V, Cohen T, Colijn C. Beyond the SNP threshold: identifying outbreak clusters using inferred transmissions. Mol Biol Evol. 2019;36(3):587–603. doi:10.1093/molbev/msy242.
- Korhonen V, Smit PW, Haanperä M, et al. Whole genome analysis of Mycobacterium tuberculosis isolates from recurrent episodes of tuberculosis, Finland, 1995–2013. Euro Soc Clin Microbiol Inf Dis. 2016;22:540–554.
- Bryant JM, Schürch AC, van Deutekom H, et al. Inferring patient to patient transmission of Mycobacterium tuberculosis from whole genome sequencing data. BMC Infect Dis. 2013;13(1):110. doi:10.1186/1471-2334-13-110.http://www.biomedcentral.com/1471-2334/13/110.
- Pérez-Lago L, Comas I, Navarro Y, et al. Whole genome sequencing analysis of intrapatient microevolution in Mycobacterium tuberculosis: potential impact on the inference of tuberculosis transmission. J Infect Dis. 2014;209(1):98–108. doi:10.1093/infdis/jit439.
- Walker TM, Ip CL, Harrell RH, et al. Whole-genome sequencing to delineate Mycobacterium tuberculosis outbreaks: a retrospective observational study. Lancet Infect Dis. 2013;13(2):137–146. doi:10.1016/S1473-3099(12)70277-3.
- Menardo F, Duchêne S, Brites D, Gagneux S. The molecular clock of Mycobacterium tuberculosis. PLoS Pathog. 2019;15(9):e1008067. doi:10.1371/journal.ppat.1008067.
- Warren RM, Victor TC, Streicher EM, et al. Patients with active tuberculosis often have different strains in the same sputum. Am J Respir Crit Care Sleep Med. 2004;169:61014.
- Kay GL, Sergeant MJ, Zhou Z, et al. Eighteenth-century genomes show that mixed infections were common at the time of peak tuberculosis in Europe. Nature Comm. 2015;6:6717. doi:10.1038/ncomms7717.
- Panjabi R, Comstock GW, Golub JE. Recurrent tuberculosis and its risk factors: adequately treated patients are still at high risk. Int J Tuberc Lung Dis. 2007;11(8):828–837.
- Enkirch T, Werngren J, Groenheit R, et al. Systematic Review of Whole-Genome Sequencing Data To Predict Phenotypic drug resistance and susceptibility in Swedish Mycobacterium tuberculosis Isolates, 2016 to 2018. Antimicrob Agents and Chemother. 2020;64(5):e02550-19.
- Wollenberg K, Harris M, Gabrielian A, et al. A retrospective genomic analysis of drug resistant strains of M. tuberculosis in a high burden setting, with an emphasis on comparative diagnostics and reactivation and reinfection status. BMC Infect Dis. 2020;20(1):17. doi:10.1186/s12879-019-4739-z.
- Tamilzhalagan S, Shanmugam S, Selvaraj A, et al. Whole-Genome Sequencing to Identify Missed Rifampicin and Isoniazid Resistance Among Tuberculosis Isolates—Chennai, India, 2013–2016. Front Microbiol. 2021;12:720436. doi:10.3389/fmicb.2021.720436.
- Ahmad S, Mokaddas E, Al-Mutairi N, Eldeen HS, Mohammadi S. Discordance across phenotypic and molecular methods for drug susceptibility testing of drug-resistant mycobacterium tuberculosis isolates in a low TB incidence country. PLoS One. 2016;11(4):e0153563.doi:10.1371/journal.pone.0153563.
- Dixit A, Kagal A, Ektefaie Y, et al. Modern lineages of Mycobacterium tuberculosis were recently introduced in western India and demonstrate increased transmissibility. Open Forum Infect Dis. 2021;8(Suppl 1):783–784. doi:10.1101/2022.01.04.22268645.
- Phyu AN, Aung ST, Palittapongarnpim P, et al. Distribution of Mycobacterium tuberculosis lineages and drug resistance in upper Myanmar. Trop Med Infect Dis. 2022;7(12):448. doi:10.3390/tropicalmed7120448.
- Brode SK, Dwilow R, Kunimoto D, Menzies D, Khan FA. Chapter 8: Drug resistant tuberculosis. Can J Resp Crit Care Sleep Med. 2022;6(Sl):109–128. doi:10.1080/24745332.2022.2039499.