ABSTRACT
Policy assessment is often limited to the evaluation of the physical effectiveness or economic efficiency. To analyse how policies intervene in the complex reality, qualitative and quantitative complex system modelling approaches from the field of Systems Thinking (STM), System Dynamics (SDM) or Agent-based modelling (ABM) can provide valuable insights. This article aims to illuminate the opportunities and limitations of these three exploratory modelling approaches for policy assessment. It addresses stakeholders in policy advices and decision makers designing policies and gives an overview of modelling approaches to evaluate the complex and social impact of policies including side-effects and non-linear system behaviour. After a short review of policy modelling in general, we compare STM, SDM and ABM regarding their methodology, modelling process and applicability in practical policy advice. We suggest that STM as a qualitative modelling approach is applicable to foster short-term policy design processes. In contrast, SDM and ABM as a time-resolved quantitative technique enable deeper system insights but requires more effort regarding model quantification. They are ideal to analyse how policies affect long-term societal problems like sustainability transformations. Finally, we propose first measures to establish such complex system modelling approaches, which today represent only a niche in policy evaluation.
Introduction
The large field of policy assessment aims to evaluate the impact of policies on solving existing problems in society. In political practice, this is often done by focusing on economic efficiency and physical effectiveness of a policy to solve a problem by different ex-ante analysis techniques ranging from expert evaluation based on experience and knowledge (Browne et al. Citation2019) over multi-criteria decision analysis (Greco, Ehrgott, and Figueira Citation2016; Huang and Keisler Citation2011) to tools from decision support, like operations research (Elsawah et al. Citation2023; Ritchey Citation2012). However, little attention is paid to how the policy might affect the future development of societies or other complex systems including non-linear behaviour, tipping points or unintended side effects (Edmonds and Meyer Citation2017). Because policies intend to change human decisions and behaviour e.g. towards implementing a technical or social innovation, this also includes the question of how a policy might be accepted by the affected actors and what other side-effects it might induce (Ghaffarzadegan, Lyneis, and Richardson Citation2011; Siegenfeld and Bar-Yam Citation2020). Therefore, when designing policies it is vital to consider social behaviour, which goes beyond rational behaviour (Lipman Citation1995). Policies can also be designed to transform societal norms and values as it is for example the case for climate change mitigation and adaptation. In this context, it is also essential to understand social dynamics. In addition, policies often not only aim to change or transform social but also technical, environmental or economic issues of existing problematic behaviour. Policies usually act in mixed complex systems, like social-technical, social-economic or social-economic-environmental systems (Balint et al. Citation2017; Barnes Citation2019; Cherp et al. Citation2018; Filatova et al. Citation2013; Lamperti et al. Citation2019; Schwarz et al. Citation2019). Different approaches to modelling complex systems are designed to consider the high complexity an implementation of a policy might cause, including its cross-sectoral and social impact. These modelling approaches mainly differ in whether they analyse a policy qualitatively, e.g. Systems Thinking modelling (STM) (Hovmand Citation2014a), or quantitatively, e.g. System Dynamics modelling (SDM) (Naugle, Langarudi, and Clancy Citation2024) or Agent-based Modelling (ABM) (Lamperti et al. Citation2020). STM and SDM focus on the macro perspective (aggregated) of understanding complex systems behaviour by identifying reinforcing and balancing feedback loops describing the non-linear dynamics (Sterman Citation2002). ABM is commonly used to describe the more detailed micro perspective by analysing emergent system behaviour through social interaction of (heterogeneous) individuals (Lippe et al. Citation2019) as demonstrated by various applications for innovation diffusion models (Scheller, Johanning, and Bruckner Citation2019; Valente and Rogers Citation1995; Zhang and Vorobeychik Citation2019). Which system modelling approach is most suitable to evaluate the efficacy of a policy in more detail depends on the specific question that the model should answer and the available resources for model development, i.e. available funding, expertise, time and data.
Our extensive preliminary literature review pointed out that a methodological comparison for the most common complex system policy modelling approaches, STM, SDM and ABM, was not performed before. While several studies compared both quantitative methods SDM and ABM (Filatova, Polhill, and van Ewijk Citation2016; Furtado, Fuentes, and Tessone Citation2019; Kelly et al. Citation2013; Khan and Abonyi Citation2022), only Coyle (2020) compared qualitative (STM) and quantitative (SDM) methods but without focus on policy modelling. However, since STM, SDM and ABM have very different access for describing how policies intervene in complex social systems, a direct comparison can help to select the most suitable method(s) for specific policy assessment tasks.
In our article, we attempt to fill this gap in the existing research literature on the potential use and benefit of STM, SDM and ABM for practical policy advice. With this, we want to change that those promising modelling techniques designed to consider complexity in policy assessment are nowadays only niches in policy advice (Schrieks et al. Citation2021). Therefore, we aim to compare the three modelling approaches, STM, SDM and ABM which are able to analyse the complex and especially social impact policies might induce. From all decision support modelling approaches we focus on these three approaches because their overall aim is to consider complexity and social aspects of implementing policies in societies. We focus on their differences and similarities of their suitability and applicability in practical policy advice. The addresses of our article are stakeholders in policy advice and decision makers designing policies and questioning what kind of approaches are available to evaluate the impact of policies in social realities including side-effects and non-linear system behaviour. This article serves as a narrative review comparing the three most promising complex system modelling approaches for policy assessment. An introducing overview of the field of policy modelling approaches and existing categories and classes is given in chapter 2 of this article. Based on this, the ongoing chapters focus on comparing the three most relevant modelling frameworks for ‘complex system policy modelling' (Lofdahl and Voshell Citation2018), STM, SDM and ABM, in more detail. For this purpose, we discuss the differences in the modelling methods in Chapter 3. The modelling process in Chapter 4 for complex system policy models shows how it depends on the choice of the modelling method. Chapter 5 synthesizes what the methodological differences discussed in Chapters 3 and 4 mean for various areas of application of STM, SDM, and ABM for practical policy advice. Finally Chapter 6 gives an overview of the existing obstacles for implementing such models in policy assessment and represent measures to overcome them.
Review of approaches for policy modelling
This chapter reviews various methodologies for policy modelling from different fields and theories able to evaluate policies from different perspectives. In general, policies can be assessed in two ways: as policy evaluation (ex-post assessment) or as policy appraisal (ex-ante assessment) (Adelle and Weiland Citation2012). Policy modelling usually is applied for ex-ante assessment which means the estimation of the effect of future policies on systems, which is the focus of the modelling approaches discussed here. It allows decision-makers to experiment in a virtual reality (Gilbert et al. Citation2018) and thus supports them in developing optimal policy designs before their implementation. Sun et al. (Citation2016) argue that the use of policy modelling ‘is partially driven by an increasing demand of stakeholders and decision-makers to provide support for understanding the potential implications of decisions in complex situations […]' (Sun et al. Citation2016).
As mentioned by (Ruiz Estrada and Yap Citation2013) policy modelling relies on different theories and approaches ranging from structural models, e.g. Decision Trees (Nilsson et al. Citation2008) or Concept Maps (Novak and Cañas Citation2006), over stochastic models, like Bayesian network (Parry et al. Citation2013), to dynamic simulations, like hydrodynamic simulations for flood risk assessment (Sauer and Ortlepp Citation2021) or SDM and ABM (De Ruig et al. Citation2023). In addition, such models are used in a broad spectrum of fields ranging from economics (e.g. computational general equilibrium (CGE) models (Niamir, Ivanova, and Filatova Citation2020)) to natural science (e.g. climate projections), engineering (e.g. hydrodynamic simulations (Sauer and Ortlepp Citation2021)) and social science (e.g. ABM and SDM (Brailsford et al. Citation2019)). The complexity of policy models varies significantly across different fields and approaches, as does the effort required to create valid models. Policy models can only represent the complex reality and the impact of policies on its behaviour to a limited degree.
To better classify existing approaches in the landscape of policy modelling, we conducted an extensive review which turned out to be challenging for two reasons. First, a variety of disciplines from strategic foresight (Dreyer and Stang Citation2013), policy analysis (Browne 2019), policy impact assessment (Acs et al. Citation2019; Adelle and Weiland Citation2012; Podhora et al. Citation2013), complexity science (Mischen and Jackson Citation2008), operations research (Scheller and Bruckner Citation2019) decision-support systems (Ritchey Citation2012), innovation diffusion (Scheller, Johanning, and Bruckner Citation2019), transformation or transition studies (Köhler et al. Citation2018) to strategic management and behaviour modelling (Darnton Citation2008; Fuentes, Tessone, and Furtado Citation2019; Furtado, Fuentes, and Tessone Citation2019; Ruiz Estrada and Yap Citation2013) provide different perspectives on modelling policy efficacy. Second, due to the wide range of approaches to policy modelling (e.g. in the natural sciences, engineering and social sciences, and economics), there exists no standard classification of modelling methods, no unified terminology for the different modelling approaches and their characteristics, and no unified approach to test the suitability of different modelling methods for different tasks of policy assessment (Adelle and Weiland Citation2012; Badham Citation2014; Ritchey Citation2012; Voinov et al. Citation2018).
Reviewing the field of policy modelling, models can be assigned to different existing categories or classes:
Qualitative and quantitative models (Coyle Citation2000): Qualitative models offer a descriptive representation of the impact of a policy on the system, without the requirement of quantifying the model variables. The focus is on the model structure and process (Hovmand Citation2014a). Quantitative models can describe the time-dependent dynamic behaviour of a system and the impact of policies through simulations or stochastic analyses. Quantitative approaches permit direct comparisons between different policy instruments, policy design options, and application contexts, either through direct comparison or by contrasting them with reference cases. The major challenge of this modelling approach is to quantify variables, particularly when dealing with social data (Sterman Citation2002). Qualitative modelling approaches can be beneficial for evaluating policy efficacy because they require few resources to build a valid model as quantification of system variables is not necessary. For policy evaluation, this is advantageous because of the short time frame from policy development to implementation.
Predictive modelling and exploratory models (Bankes Citation1993): Predictive models are based on the assumption that models can be a surrogate for the actual system. They focus on accurate predictions and model validity and require resolvable uncertainty and knowledge about system characteristics and initial conditions as well as a complete parameter set (for example: reduction of flooded areas by implementing mitigation measures modelled by hydrodynamic simulations). In contrast, exploratory models admit that a reliable image of the target system cannot be achieved. They ask how the world would behave if the model assumptions (e.g. scenario) would be correct and use inductive and abductive reasoning to gather information that informs the decisions of stakeholders. Their modus operandi is to explore the implications of assumptions, implications and hypotheses on the development of a system. Exploratory models are not able to precisely evaluate how a policy changes a system. This is caused by the considered complexity and limited accuracy in available data, e.g. considering social data (example: analysing diffusion dynamics of a social innovation (e.g. how flood mitigation measures are implemented by homeowners) by ST, SD or ABM). Exploratory Models are used in the fields of ‘Coupled human and natural systems (CHANS)' which consider various complexities such as heterogeneity, nonlinearity, feedback, and emergence (focussing decision models by ABM) (An Citation2012). In this context, ‘Exploratory Modelling and Analysis’ (EMA) is an additional field that uses computational experiments to analyse complex and uncertain issues (Kwakkel and Pruyt Citation2013). The wide field of "Social-ecological Systems’ (SES) also belongs to the field of exploratory modelling because of their social component (Schlüter et al. Citation2017)
Micro and macro perspective models (Filatova, Polhill, and van Ewijk Citation2016; Furtado, Fuentes, and Tessone Citation2019; Haynes and Alemna Citation2022): Micro perspective modelling, like ABM (including microsimulation) considers modelling elements of systems in detail (for example: how flood mitigation measures are implemented caused by the interaction dynamics of heterogeneous homeowners). On the contrary, macro perspective modelling, like CGE, STM and SDM describes the system-wide variables and their interaction (for example: what ecosystem change can be evoked by reducing the flooded area due to flood mitigation measures). Whether macro or micro perspective modelling is more suitable to evaluate a policy depends on the specific question the model is intended to analyse.
Differential equation-based models (DEBM) and discrete event simulation (DES): DEBM are a form of equation-based models where differential equations are solved empirically as e.g. for System Dynamics models (SDM) (Sterman Citation2002). In contrast, in DES the dynamics come from time-steps where the state of variables can change and thus change the dynamics of the system. ABM is a form of DES, focussing on individual entities in the system and their interactions instead of modelling process flows in the traditional DES (Siebers et al. Citation2010). Also, combinations of these modelling approaches like hybrid simulation in the operational research field exist, which are defined by combining DES/ABM and SD (Brailsford et al. Citation2019).
Concept/theory-driven and data-driven models: Data-driven models are computational models that establish relationships between the input and output of a system based on historical data. They are commonly used in big data, artificial intelligence, and machine learning (Rendall, Chiang, and Reis Citation2019). As their semantics are derived from data, data-driven or empirical models do not explicitly care about the semantics of the system under consideration. In contrast to this, concept- or theory-driven approaches are based on understanding the system and its behaviour.
Given this variety of classifying models, it may be difficult to determine which modelling technique is the most suitable for evaluating policy efficacy. In addition, models can also be a mixture of the discussed opposing classifications, e.g. concept-driven and data-driven modelling.
In policy advice, predictive modelling approaches are commonly used to evaluate the physical effect a measure or policy might cause. Exploratory modelling approaches analysing the impact of policies on a system level (e.g. economic, technological, and techno-ecological systems) are comparatively rarely used in policy advice (Voinov et al. Citation2018). However, such policy evaluation including complex interdependencies, non-linear dynamics and unintended side effects policies can evoke, is essential if policies act in complex systems. In addition, policies are developed to affect the decisions of specific actors in a society (e.g. land owners, companies, municipalities) to e.g. adopt the intended measure or to change their behaviour. From this perspective, policy assessment should also consider the social aspect of a system. Accordingly, analysing the efficacy of policies requires modelling techniques that can handle complex social systems (e.g. socio-economic, socio-technical, socio-techno-ecological systems). gives an overview of the most frequently used modelling approaches that can analyse the impact of policies in systems in an exploratory manner from different perspectives – qualitative to quantitative and macro to micro perspective. In addition to data-driven models, SDM and ABM are found to be the most widely used quantitative modelling approaches, applying macro as well as micro perspectives (Furtado, Fuentes, and Tessone Citation2019; Pejic Bach et al. Citation2019). These approaches provide deep insight into non-linear responses of the system, including tipping points and unintended side effects (Fuentes, Tessone, and Furtado Citation2019). From a qualitative angle of assessing how policies intervene in complex social systems, STM is seen as a promising approach (Haynes and Alemna Citation2022). In the next sections we focus on the three exploratory modelling approaches STM, SDM and ABM as promising candidates to widen the policy assessment to the impact it might have in complex reality and social behaviour.
Figure 1. Mapping exploratory modelling approaches to assess policies in systems in dependence if the analysed system is represented in a qualitative (top) or a quantitative (bottom) manner as well as if it focusses more on the macro perspective (causal relationships; left) or on the micro perspective (individual interactions; right). Modelling techniques overlapping the sectors can be applied for both, e.g. data-driven models ranging from aggregated to detailed analysis.
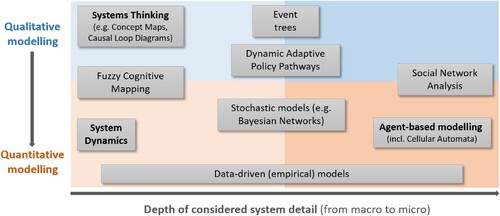
Complex system policy modelling approaches to assess the impact of policies
In the previous section, we have shown that evaluating and assessing policy implications of complex models of systems (integrating technical, social, economic and ecological factors) requires modelling approaches that address their complex and social nature. Due to their complexity (non-linearity, feedback loops, socio-ecological influences etc.), predictive models fall short in key phenomenological aspects. In the following, we will thus limit ourselves to suitable exploratory modelling approaches for describing complex system behaviour. For this, we will focus on data availability, modelling resources and theoretical knowledge of the phenomenon under investigation. As discussed in Section 2, STM, SDM and ABM are approaches that respect the complex nature of mixed systems. Accordingly, we discuss these approaches in more detail in this section.
Systems thinking modelling
Systems Thinking modelling is a toolbox of qualitative aggregated (macro-level) modelling approaches aiming at gaining knowledge about the structure of complex systems where the policy intervenes. For qualitative system behaviour analysis, Causal Loop Diagrams (CLD) are an important modelling approach of the STM toolbox focusing on feedback loops and delays to describe system behaviour from system structure. This focus on system structure can also be seen in the modelling process. STM processes often involve the knowledge of different stakeholders to describe the system on a conceptual basis, identifying relevant entities with a significant influence on the system as well as their relations in a qualitative manner. The direction in which different system entities or variables influence each other is indicated only through the polarity (positive or negative correlation). Additionally, CLDs visualize feedback loops and indicate whether these loops are balancing (i.e. dampening/centring the system behaviour) or reinforcing (leading to run-away effects). Important for descriptive system behaviour from CLDs is their consideration of delays between system entities. STM has the advantage that stakeholders can be involved easily and time-efficiently for policy advice. CLDs are a suitable tool for modelling overall system behaviour in a matter of hours or days. For policy evaluation, this is advantageous because of the short time frame from policy development to implementation. However, due to their qualitative nature, they are not suited for executing computations and thus simulations. Accordingly, for application in policy advice, STM is appropriate to gain a comprehensive overview of where in a system the policy intervenes, how it changes the system behaviour by feedback loop changes and what side effects it might induce. Overall, STM can be summarized as a qualitative, concept-driven exploratory approach focusing on the macro perspective as summarized in .
Table 1. Assignment of the three Complex system policy modelling approaches to various modelling categories.
System Dynamics modelling
System Dynamics modelling is a quantitative aggregated (macro-level) modelling method to investigate the dynamic (time-resolved) non-linear system behaviour emerging from feedback loops as an extension of Causal Loop Diagrams. It can be used as an extension (quantification) of STM methods by quantifying (parameterizing) the system variables derived in the STM generation process as well as explicitly expressing the equations describing the system influences, capturing the complex time-resolved dynamics of a system. This allows for direct comparisons between different policy instruments, policy design options, and application contexts, either through direct comparison or by contrasting them with reference cases. SDM focuses on considering the system observation variables, stocks and flows from a phenomenological respectively macro perspective. These models are suitable where aggregate effects dominate the system behaviour. They use differential equations to describe, analyse and predict the macro-behaviour of complex systems (Sterman Citation2002). In contrast to STM which primarily considers system structure, SDM aims at system parameterization after the system structure is laid out. SDM can also be used to analyse innovation diffusion from the perspective of feedback loops and system structure (Uriona and Grobbelaar Citation2019). The advantage of SDM is its visual structure, which is easier to communicate and understand than the code of ABM described in the following.
As with other macro-perspective models, SDM suffers from several shortcomings. By aggregating individual entities in system variables, it assumes a homogeneous population, or at least homogeneous groups that can either interact with every other member of the population or can’t interact with one another at all. Due to their aggregate nature, they are furthermore not suited to model geospatial system aspects (in high resolution) and can’t study the decision process of individual actors. For policy advice, the strength of SDM lies in demonstrating how the dynamics of a system can be changed by policies in an unexpected manner because of policies influencing feedback loops and thus complex non-linear temporal system behaviour. Overall, SDMs can be summarized as quantitative exploratory equation-based macro-perspective models that are developed in a concept-driven fashion. This is summarized in .
Agent-based modelling
Agent-based modelling is a quantitative detailed (micro-level) modelling method to analyse the complex dynamic system behaviour emerging from individual interactions and decisions of agents. As such it allows direct comparisons between different policies, policy design options, and application contexts, either through direct comparison or by contrasting them with reference cases.
In contrast to STM and SDM, ABM views system observation variables as emergent properties of many interlocking interactions of multiple heterogeneous micro-entities (agents). To model these agents, ABM needs many more parameters and system variables to record the states of the individual agents. Due to the multitude of variables, verification and validation of these models is much more complicated than in the case of aggregated models. If populations are split into different agent groups (such as social milieus), this size reduction could furthermore result in higher required significance levels, preventing more subtle effects from being measured.
ABM has significance when the observed patterns go beyond the aggregation of individual attributes and when they exhibit self-organization and the emergence of social order (Furtado, Fuentes, and Tessone Citation2019). Behaviour in ABM can thus be seen to be generated by the interaction of system elements. As Kiesling (Citation2011) observes, ABMs can reproduce and explain complex non-linear diffusion patterns observed in the real world resulting from simple local micro-level interactions (Kiesling Citation2011). From the point of innovation diffusion theory, implementing a policy to influence the decision of an individual is transferable to analysing how policies as innovations diffuse between actors or in a society and are classically connected using ABM as a micro-level modelling approach (Palm and Lantz Citation2020). Overall, ABM can be seen as an exploratory quantitative, micro-perspective, concept-driven modelling approach for policy assessment using discrete event simulation. This is summarized in .
Comparison of complex system modelling approaches
While all approaches discussed in this section are exploratory and concept-driven, they differ significantly in their level of aggregation and quantitative nature. STM, SDM and ABM are suited for different problems and modelling goals and each approach comes with its own set of opportunities and limitations as discussed above. It is important to emphasise that in both quantitative modelling approaches, ABM and SDM, the focus is on exploration to gain deeper insight into the system rather than on predicting future system dynamics (Heath, Hill, and Ciarallo Citation2009). The reason for this is that a sharp prediction of the future is in general not possible due to high uncertainties in how the variables of a system will evolve in the future (Bankes Citation1993). Thus, like all modelling approaches exploring possible futures, ABM and SDM use scenarios to analyse the impact of policies for different possible futures (Murray-Rust et al. Citation2013).
For policy advice, ABM and SDM are both suitable to demonstrate how policies can alter temporal system behaviour under defined boundary conditions, identify potential side effects of policies, and determine their ability to achieve significant behavioural changes in the system towards the desired state. ABM as well as SDM ‘aim to improve the system understanding rather than prediction or forecasting’ (Kelly et al. Citation2013). In contrast to the SDM and ABM simulations, qualitative STM does not require time-consuming parametrisation and validation of the models. It is a tool to conceptually understand how policies might affect the system behaviour descriptively without any quantification. A comparison of all three simulation approaches, SDM, ABM and CLD/STM for their application in policy assessment is given in .
Table 2. Main characteristics of Causal Loop Diagrams as qualitative and System Dynamics as well as Agent-based modelling as quantitative modelling approach including the way how policies can be implemented. Table based on (Kelly et al. Citation2013; Khan and Abonyi Citation2022; Martin and Schlüter Citation2015).
Here we have highlighted the methodological differences/variations between the three selected approaches. These variations also affect the modelling process. In this modelling process, the selection of the most appropriate modelling method is an important step, depending on the goal, dispensable resources, availability of data, modellers expertise and trade-offs modellers are willing to make. The modelling process is outlined in the following section.
Comparing the process of developing complex system models for policy assessment
To understand the different applicability of the STM, SDM and ABM approaches for policy advice, it is important to know what the modelling process itself looks like and where they differ. Our literature review on modelling approaches in policy advice has revealed that there is no standardized process for the development of policy models (Schünemann, Sidorova, and Herold Citation2023b). However, generalised steps for model development can be applied to most policy modelling processes. Based on the contribution of Voinov (2018), we developed the process scheme in that illustrates the different phases of a typical complex system modelling process as a diagram. The scheme is a key to understand the main steps in creating a valid policy model. Each step of model development requires a different amount of effort, resulting in models that vary in complexity. Our scheme is based on extensive literature research and our own experience. The following sections provide a detailed explanation of each process stage.
Figure 2. Generalized process steps for developing qualitative and quantitative complex system models for the application of policy evaluation.
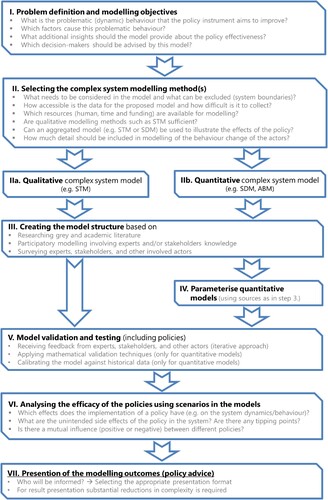
I. Problem definition and modelling objectives
During the initial phase, it is important to precisely define the problem that is intended to be analysed by the developed model. The clients and the modelling team should collaborate closely in this respect. The objective is to identify the specific problematic behaviour that the policies should address. Misunderstandings may arise between the client and the modelling team during this iterative process. To ensure clarity, it is crucial to define the system boundaries of the model and the influences to be mapped. In addition, it is important to discuss the insights provided by the model for assessing policy effectiveness which are not possible to achieve with other low resource policy assessment methods.
II. Selecting the complex system modelling method(s)
Based on the problem definition the client and modelling team need to decide on the most appropriate complex system modelling approach to assess the policy impact on the affected systems. Selecting the most appropriate method is not a trivial task and depends on the available resources, the question of what policy impact needs to be modelled and other issues (see ). Especially what kind of impact of the policy should be analysed by the model is crucial for the choice of the most suitable modelling approach. Is an aggregated qualitative model like STM sufficient for this evaluation describing the system behaviour response to a policy by the system structure qualitatively? Or is it necessary to analyse the non-linear system dynamics in a quantitative time-resolved manner speaking for SDM. Or is there a need to understand how individual decisions and interactions influence the policy efficacy which speaks in favour of ABM. It is also possible to choose a combination of different modelling methods. The modelling team must have sufficient expertise to assess the potentials and limitations of various approaches.
In we suggest a decision tree for identifying the most suitable modelling method. The decision can be made from either the perspective of the research objective or the availability of resources. A pragmatic approach to resource availability leads to a quick response: many resources allow for all types of modelling, limited resources restrict the feasibility of SDM and STM, and few resources reduce the choice to only STM. The selection of modelling type is more multi-faceted when considering it from a research perspective. It is important to clarify whether the modelling should focus on system interrelationships or social actor behaviour. The former can be described by aggregated models, leading to STM or SDM depending on whether qualitative or quantitative data input and model output is needed. For social actor behaviour, a distinction must be made between detailed (micro), requiring ABM, or aggregated (macro) modelling (SDM, STM). It is up to the discretion of the clients and the expertise of the modelling team to make the appropriate choice for each modelling project.
III. Creating the model structure
The model structure is developed by the modelling team for the selected modelling method. For both, SDM and STM, system variables needs to be identified and connected to uncover the system structure. The main challenge is to map the complex system structure in a way that is detailed enough to capture the major processes but simple enough that it can be handled (system boundary definition, level of aggregation). An important issue at this point is that the value system of the modelling team and client should be avoided as much as possible to be integrated into the model to avoid bias. The modelling team can gather the relevant variables and system parameters from various sources like research of grey and scientific literature or integration of expert or stakeholder knowledge in the model by surveying them or involving them in the model development, e.g. applying Group Model Building (Hovmand Citation2014b).
For ABM, one of the fundamental choices is whether the model should be built on existing technical (e.g. RePast (see https://repast.github.io/)) or conceptual (e.g. IRPact (Johanning et al. Citation2020)) modelling frameworks or should be developed from the ground up. If a conceptual framework is chosen, certain model entities and model mechanisms are already suggested. Researchers should identify what model entities exist, how they can be described, and what actions they can perform. Agents are bound in time, space and their environment. Finally, for all entities, relevant variables, states, state transition rules (process models) and further processes for governing the simulation overall need to be identified.
IV. Parameterize quantitative models
The different modelling approaches require different types of information and data. Qualitative approaches like STM demand only descriptive data to capture system structure and relationships. Quantitative models like ABM and SDM, on the other hand, need to be parameterized with quantitative data. This includes defined weighting factors and equations for SDM as well as decision rules with quantified threshold values for ABM. Acquiring data for quantitative models can be resource-intensive, especially if the data is not available at the quality or resolution required. Retrieving individual data for ABM is in general more time-consuming than tabulating data that reflects macro behaviour for SDM (Kunc Citation2017).
V. Model validation and testing
Model structure and behaviour must be exhaustively tested and validated. Validation is essential to ensure that models have the requisite reliability to be used for policy advice. The model testing can be multi-tiered with first excluding policies in a first stage and subsequently analysing the impact of policies. The validation effort for quantitative models (SDM, ABM) is usually much larger than for qualitative models (STM). For validation, the different techniques in can be employed:
Receiving feedback from experts, stakeholders, and other actors (iterative approach)
Applying mathematical validation techniques (only for quantitative models)
Calibrating the model against historical data (only for quantitative models)
VI. Analysing the efficacy of policies using scenarios
To assess the impact of the policies in the explorative model, scenarios are commonly used. The considered scenarios need to be developed with the client and the stakeholders involved. Especially for quantitative models, parameters can be varied to capture uncertain variables in the model and its effect on the policy impact. The objective is to gain new insights into the effects of the analysed policies and to improve their design. To achieve this goal, the questions depicted in can be used.
VII. Presentation of the modelling outcomes (policy advice)
Even the best model is useless if its outcomes cannot be communicated in an intelligible manner. The proper presentation format for the model outcomes therefore is a crucial step in the modelling process. When disseminating the results, it is important to consider the target audience first. The level of detail in communicating the results should be adjusted based on the audience's knowledge and time. In this context, the reduction of the model's complexity is essential to highlight the major model outcomes that can support the policy design process. Various tools for knowledge transfer can be used for this purpose, such as Rich Pictures, scenarios or policy briefs (Voinov et al. Citation2018) Serious Gaming (Bär, Johnsen, and Gölz Citation2023) and Digital Twins (Schrotter and Hürzeler Citation2020) are helpful approaches to knowledge transfer as well. Our research indicates that this step has not been sufficiently addressed in the scientific literature.
Applicability of complex system policy models for policy advice
The previous chapter is essential to understand the different applicabilities of complex system policy modelling approaches STM, SDM and ABM for policy advice. All of them can analyse the social impact of policies and take into account the complex reality in which policies intervene. The discussed approaches for modelling complex systems have the potential to support the design of effective policies by considering the impact of policies on the multiple interrelations of systems as well as on the social behaviour (e.g. decision-making) of actors affected by the policies. This existing complexity is in general very difficult to grasp without considering system models as the prevailing linear cause-and-effect relationships are usually too simplified to depict the impact of policies in the real world (Badham Citation2010). In addition, such models are suitable for including how policies affect the behaviour of actors in different ways, depending on the modelling approach (STM & SDM for macro-level focussing on feedback loops, ABM for micro-level focussing on more (heterogeneous) individual decisions). In doing so, the models can help to test if policies show the intended effect on the behavioural change of the targeted actors or induce unintended side-effects and co-benefits. Behaviour changes can also be analysed without those modelling approaches by empirical social research methods. However, considering the impact of behaviour changes in system models allows a deep understanding of how a change in actor behaviour through a policy can change the macroscopic system behaviour in future. Especially ABM is well suited to gain deeper insights into how individual, heterogeneous behaviour changes of actors by policies affect the emergent system dynamics. STM and SDM techniques can include the knowledge of different experts for highly interdisciplinary or cross-sectoral problems (such as climate change protection or adaptation) in participatory modelling approaches like Group Model Building (Hovmand Citation2014a). This enables a more comprehensive description of the interdependencies in complex systems where policies are intended to intervene by integrating the inter- and transdisciplinary system knowledge (mental models). In addition, the mental model of the participants in joint modelling can be significantly expanded, linear thinking patterns can be overcome (Scott Citation2018). Such participatory modelling processes can also serve to reconcile strongly divergent opinions on the solution of complex problems through policies and thus help build consensus (Hovmand Citation2014a).
In sum, complex system models can supplement conventional methods of policy assessment, e.g. through purely expert-knowledge-based (qualitative) assessment or using predictive models, to consider social impacts, side-effects, non-linear dynamics or emergent system changes by policies. This is particularly true for policies acting in very complex, cross-sectoral problems such as climate change, sustainable urban planning or transformation processes hard to capture with other approaches. For the application of quantitative modelling approaches for advising political decision-makers the high expenditure of resources and especially required time for the model creation is a major obstacle, especially if the available data for quantification is insufficient. summarizes the discussed differences between qualitative and quantitative modelling approaches for their application in policy advice.
Table 3. Comparison of qualitative and quantitative modelling approaches to assess policies in social complex systems focusing on their practical application in policy advice.
Obstacles for implementing such models in policy assessment and how to overcome them
Various limitations and challenges exist to implement such exploratory system models in policy advice. Although societal, economic or ecological problems are globally connected and increasing in complexity (Filatova, Polhill, and van Ewijk Citation2016), modelling approaches to analyse the policy efficacy in social and complex systems are rarely used to support policy design (Schünemann, Sidorova, and Herold Citation2023b). This has several reasons as visualized in a simplified manner in the conflict situation of decision maker and modeller in . The required resources for developing models (human resources including expertise, financing, and required data) represent a significant limitation to applying them in practical policy advice. As already mentioned, these are significantly larger for quantitative system modelling approaches, especially dynamic simulations such as ABM or SDM, than for qualitative ones like STM. This also affects the duration of model development, which depends on the modelling method and the inquired depth of analysis of the impact of the policy and can therefore range from hours (STM) to years (SDM and ABM). Especially when introducing policies in response to urgent problems, building a valid simulation model would take too long.
Figure 4. Illustration of the conflict situation between decision maker or political advisor and modeller to explain the challenges to implement complex system modelling in practical policy advice (illustrated by Nicolaas Boengarts, IOER Media).
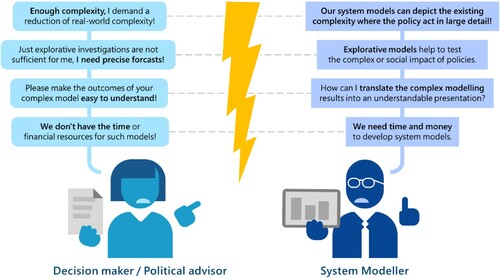
In addition, exploratory system modelling cannot provide precise forecasts of how policies affect future dynamics. The future development of complex systems is too uncertain for such a forecast and therefore scenarios are used for in-depth analysis of what effects policies might show under certain boundary conditions in a system.
Another limitation is given by modelling the social dynamics induced by policies in such models. Even if these modelling approaches can be used to depict the change in human decisions and behaviour caused by policies, this effect is difficult to implement in models due to the bounded rational decision-making patterns and the individual heterogeneities in decision-making (Schrieks et al. Citation2021). Decision rules in ABM are difficult to quantify despite the greatest care, especially when one considers that they may change in future – depending on external circumstances. When mapping behaviour in quantitative system models, there may be ethical challenges in obtaining the required data, such as compliance with data protection (Süsser et al. Citation2021) or abuse of the models for manipulation of actors (Bertoni et al. Citation2022).
Another critical issue is that the models are often seen as rational assessments of policies but because they depend on the modellers' and experts' expertise, norms and values complex system models cannot be seen as purely objective. In this context, models can also be misused to legitimise prefabricated decisions (Turnpenny et al. Citation2008). This problem can be addressed through a high degree of transparency, accessibility and the involvement of a large variety of experts, actors and stakeholders (knowledge integration) (Bertoni et al. Citation2022). Whether models are finally used for policy advice depends to a significant part on the question of how the model outcome is presented to the decision makers or the policy advisors and if they trust the model results (Hovmand Citation2014a; Scott Citation2018).
To overcome that the use of complex system policy models are nowadays only niches in policy assessment we suggest the following measures based on the conflict situation between modeller and decision maker in :
Enhancing the knowledge in policy advice of the presence and opportunities and applications of the modelling techniques
Explaining decision makers and stakeholders in policy advice that decisions and policies in social (societies) and mixed systems can have significant unintended side effects that such methods can capture ex-ante (before implementation) – simplified (linear) cause–effect relationships do not reflect such dynamics in a correct manner
Raising the number of best practice examples to illustrate where such modelling approaches can generate an added value
Demonstrating that analysing the complex and social impact of policies in such models can significantly enhance policy design
Making an integration of system modellers in policy advice processes to a standard such as the evaluation of economic and physical efficacy of new policies are standards nowadays
The expertise of system modelers should be controlled, perhaps by a certification system
To implement the outcome of the complex system policy model into the decisions how a policy is designed, it is very crucial that the system modelers are aware to use suitable approaches for knowledge transfer and knowledge integration
These are first recommendations based on the experience of the authors from literature research and personal exchange with actors in policy advice in Germany within the project ‘Machbarkeitsstudie: Modellierung von Anpassungsmaßnahmen: Akteure, Entscheidungen und Wirksamkeit' (FKZ 3721 48 104 0) funded by the German Federal Environmental Agency. A deeper analysis of leverages is needed to analyse how complex system policy modelling can become established in policy advice. This is already started by creating a STM in the working group ‘System modelling for Policy Advice' by the ‘German Society for System Dynamics'.
Conclusion
In this article, we focussed on comparing the three complex system policy modelling approaches STM, SDM and ABM for assessing the complex and social impact of policies. After an overview of methods and categories in policy modelling, we compared the characteristics, modelling process and applicability of each modelling technique. By doing so, the focus layon the different applications of qualitative to quantitative and micro perspective (detailed) vs. macro perspective (aggregated) system modelling approaches for policy assessment. Our comparisons resulted in very different applications of qualitative (based on STM) and quantitative (like SDM or ABM) modelling approaches which is mainly due to the disparities in required resources. Conceptual STM can be used to evaluate the impact of a policy in a complex social system in a qualitative, descriptive way. It focuses on identifying the system structure (especially feedback loops in CLD) to explain the non-linear system behaviour and how policies might affect this by changes in the system structure. Its qualitative nature has the advantage that it can be created in a short period as well as the disadvantage that time-dependent future developments cannot be resolved in such a qualitative manner. In contrast, quantitative system modelling approaches like SDM or ABM are well suited for analysing the non-linear temporal dynamics a policy might induce in a systems future and thus give a deeper understanding of how a policy might intervene in complex social systems. However, this advantage is associated with much higher resources (time, expertise and data) required to create the model, especially for parametrisation, quantification and validation of the model. Especially providing the data to quantify social variables in a model might require the use of high effort empirical social research methods (e.g. social data analysis, expert interviews, questionnaires, data collection, …). For policies designed to address urgent problems, the lack of time does not allow to develop valid quantitative ABM or SDM. For this purpose, qualitative STM can be used to consider the systemic and social effectiveness of a policy in addition to other policy assessment techniques. Because of the manageable expertise for STM creation, this work can also be performed in-house in institutions of political decision-makers, e.g. ministries. We also recommend that such STM should be done by participatory modelling approaches (like Group Model Building) to implement the different inter- and transdisciplinary system knowledge of experts and stakeholders as well as to expand their mental model and system understanding. Quantitative models, especially simulation models based on SDM or ABM, should be generated by experienced modelling experts and therefore be commissioned externally by research institutions or consulting companies. Because of their resource-intensive model generation, the timespan for developing a valid simulation model is too large to assess urgently needed policies. Thus, the focus of these modelling approaches is analysing policies designed to solve long-term societal problems like transformation processes in energy markets or global warming mitigation. We also found that exploratory modelling approaches like STM, SDM and ABM are rarely used in policy advice which has several reasons discussed in the manuscript. To foster the application of such methods in policy practice a change in policy assessment is needed. Instead of focusing on economic and physical effectiveness, the social efficacy of policies (e.g. adopted or not) as well as their non-linear effect in complex reality should be considered as well, especially for policies designed to transform systems in the long term. It is important to note that the focus of the article was on the comparison of the modelling approaches for their application in policy advice without reviewing examples of policy models of the different approaches. Such a comparison of complex system policy modelling examples in science and political practice is strongly recommended for future research as it can support the explanation of the use of the different modelling approaches for policy assessment.
Competing interests
The authors report there are no competing interests to declare.
Disclosure statement
No potential conflict of interest was reported by the author(s).
Additional information
Funding
References
- Acs, S., N. Ostlaender, G. Listorti, J. Hradec, M. Hardy, P. Smits, and L. Hordijk. 2019. Modelling for EU Policy Support: Impact Assessments, EUR 29832 EN. Luxembourg: Publications Office of the European Union. https://doi.org/10.2760/748720
- Adelle, C., and S. Weiland. 2012. “Policy Assessment: The State of the Art.” Impact Assessment and Project Appraisal 30 (1): 25–33. https://doi.org/10.1080/14615517.2012.663256.
- An, L. 2012. “Modeling Human Decisions in Coupled Human and Natural Systems: Review of Agent-Based Models.” Ecological Modelling 229: 25–36. https://doi.org/10.1016/j.ecolmodel.2011.07.010.
- Badham, J. 2010. A Compendium of Modelling Techniques. (Integration Insights; No. 12), (Integration Insights). Canberra: The Australian National University, College of Medicine, Biology & Environment.
- Badham, J. 2014. “Bridging Complexity Science and the Social Determinants of Health.” Journal on Policy and Complex Systems 1: 60–87. https://doi.org/10.18278/jpcs.1.2.5.
- Balint, T., F. Lamperti, A. Mandel, M. Napoletano, A. Roventini, and A. Sapio. 2017. “Complexity and the Economics of Climate Change: A Survey and a Look Forward.” Ecological Economics 138: 252–265. https://doi.org/10.1016/j.ecolecon.2017.03.032.
- Bankes, S. 1993. “Exploratory Modeling for Policy Analysis.” Operations Research 41 (3): 435–449.
- Bär, C., L. Johnsen, and S. Gölz. 2023. Potenzial von Serious Games als Instrument zur Beförderung von Nachhaltigkeit. Eine Betrachtung aus Sicht des Umweltbundesamtes (Zwischenbericht No. 80/2023), Texte. Umweltbundesamt, Dessau-Roßlau.
- Barnes, J. 2019. “The Local Embedding of Low Carbon Technologies and the Agency of User-Side Intermediaries.” Journal of Cleaner Production 209: 769–781. https://doi.org/10.1016/j.jclepro.2018.10.258.
- Bertoni, E., M. Fontana, L. Gabrielli, S. Signorelli, and M. Vespe. 2022. Mapping the Demand Side of Computational Social Science for Policy. EUR 31017 EN. Luxembourg: Publications Office of the European Union.
- Brailsford, S. C., T. Eldabi, M. Kunc, N. Mustafee, and A. F. Osorio. 2019. “Hybrid Simulation Modelling in Operational Research: A State-of-the-Art Review.” European Journal of Operational Research 278 (3): 721–737. https://doi.org/10.1016/j.ejor.2018.10.025.
- Browne, J., B. Coffey, K. Cook, S. Meiklejohn, and C. Palermo. 2019. “A Guide to Policy Analysis as a Research Method.” Health Promotion International 34 (5): 1032–1044. https://doi.org/10.1093/heapro/day052.
- Checa, D., and A. Bustillo. 2020. “A Review of Immersive Virtual Reality Serious Games to Enhance Learning and Training.” Multimedia Tools and Applications 79 (9-10): 5501–5527. https://doi.org/10.1007/s11042-019-08348-9.
- Cherp, A., V. Vinichenko, J. Jewell, E. Brutschin, and B. Sovacool. 2018. “Integrating Techno-Economic, Socio-Technical and Political Perspectives on National Energy Transitions: A Meta-Theoretical Framework.” Energy Research & Social Science 37: 175–190. https://doi.org/10.1016/j.erss.2017.09.015.
- Coyle, G. 2000. “Qualitative and Quantitative Modelling in System Dynamics: Some Research Questions.” System Dynamics Review 16 (3): 225–244. https://doi.org/10.1002/1099-1727(200023)16:3<225::AID-SDR195>3.0.CO;2-D.
- Darnton, A. 2008. Reference Report: An Overview of Behaviour Change Models and Their Uses, GSR Behaviour Change Knowledge Review. Centre for Sustainable Development. London, UK: University of Westminster.
- De Ruig, L. T., T. Haer, H. De Moel, P. Orton, W. J. W. Botzen, and J. C. J. H. Aerts. 2023. “An Agent-Based Model for Evaluating Reforms of the National Flood Insurance Program: A Benchmarked Model Applied to Jamaica Bay, NYC.” Risk Analysis 43: 405–422. https://doi.org/10.1111/risa.13905.
- Dreyer, I., and G. Stang. 2013. “Foresight in Governments–Practices and Trends Around the World.” Yearbook of European Security 1368: 7–32.
- Edmonds, B., and R. Meyer2017. Simulating Social Complexity: A Handbook, Understanding Complex Systems. Cham: Springer. https://doi.org/10.1007/978-3-319-66948-9
- Elsawah, S., E. Bakhanova, R. P. Hämäläinen, and A. Voinov. 2023. “A Competency Framework for Participatory Modeling.” Group Decision and Negotiation 32 (3): 569–601. https://doi.org/10.1007/s10726-023-09818-0.
- Filatova, T., J. G. Polhill, and S. van Ewijk. 2016. “Regime Shifts in Coupled Socio-Environmental Systems: Review of Modelling Challenges and Approaches.” Environmental Modelling & Software 75: 333–347. https://doi.org/10.1016/j.envsoft.2015.04.003.
- Filatova, T., P. H. Verburg, D. C. Parker, and C. A. Stannard. 2013. “Spatial Agent-Based Models for Socio-Ecological Systems: Challenges and Prospects.” Environmental Modelling & Software 45: 1–7. https://doi.org/10.1016/j.envsoft.2013.03.017.
- Fuentes, M. A., C. J. Tessone, and B. A. Furtado. 2019. “Public Policy Modeling and Applications.” Complexity, 1–4. https://doi.org/10.1155/2019/4128703.
- Furtado, B. A., M. A. Fuentes, and C. J. Tessone. 2019. “Policy Modeling and Applications: State-of-the-Art and Perspectives.” Complexity, 1–11. https://doi.org/10.1155/2019/5041681.
- Ghaffarzadegan, N., J. Lyneis, and G. P. Richardson. 2011. “How Small System Dynamics Models Can Help the Public Policy Process.” System Dynamics Review 27 (1): 22–44. https://doi.org/10.1002/sdr.442.
- Gilbert, N., P. Ahrweiler, P. Barbrook-Johnson, K. P. Narasimhan, and H. Wilkinson. 2018. “Computational Modelling of Public Policy: Reflections on Practice.” Journal of Artificial Societies and Social Simulation 21: 14. https://doi.org/10.18564/jasss.3669.
- Greco, S., M. Ehrgott, and J. R. Figueira. 2016. Multiple Criteria Decision Analysis: State of the Art Surveys, International Series in Operations Research & Management Science. New York, NY: Springer New York. https://doi.org/10.1007/978-1-4939-3094-4
- Haynes, P., and D. Alemna. 2022. “A Systematic Literature Review of the Impact of Complexity Theory on Applied Economics.” Economies 10: 192–342. https://doi.org/10.3390/economies10080192.
- Heath, B., R. Hill, and F. Ciarallo. 2009. “A Survey of Agent-Based Modeling Practices (January 1998 to July 2008).” Journal of Artificial Societies and Social Simulation 12.
- Hovmand, P. S. 2014. “Group Model Building and Community-Based System Dynamics Process.” In Community Based System Dynamics, edited by P. S. Hovmand, 17–30. New York, NY: Springer New York. https://doi.org/10.1007/978-1-4614-8763-0_2
- Huang, I., and J. Keisler. 2011. “Multi-Criteria Decision Analysis in Environmental Sciences: Ten Years of Applications and Trends.” Science of The Total Environment 409 (19): 3578–3594. https://doi.org/10.1016/j.scitotenv.2011.06.022.
- Johanning, S., F. Scheller, D. Abitz, C. Wehner, and T. Bruckner. 2020. “A Modular Multi-Agent Framework for Innovation Diffusion in Changing Business Environments: Conceptualization, Formalization and Implementation.” Complex Adapt Syst Model 8.
- Kelly, R. A., A. J. Jakeman, O. Barreteau, M. E. Borsuk, S. ElSawah, S. H. Hamilton, H. J. Henriksen, et al. 2013. “Selecting Among Five Common Modelling Approaches for Integrated Environmental Assessment and Management.” Environmental Modelling & Software 47: 159–181. https://doi.org/10.1016/j.envsoft.2013.05.005.
- Khan, A. A., and J. Abonyi. 2022. “Simulation of Sustainable Manufacturing Solutions: Tools for Enabling Circular Economy.” Sustainability (Switzerland), 14. https://doi.org/10.3390/su14159796.
- Kiesling, E. 2011. Planning the Market Introduction of new Products (Dissertation). Universität Wien. Fakultät für Wirtschaftswissenschaften.
- Köhler, J., F. Haan, G. Holtz, K. Kubeczko, E. A. Moallemi, G. Papachristos, and E. Chappin. 2018. “Modelling Sustainability Transitions: An Assessment of Approaches and Challenges.” Journal of Artificial Societies and Social Simulation 21: 8. https://doi.org/10.18564/jasss.3629.
- Kunc, M. 2017. “System Dynamics: A Soft and Hard Approach to Modelling.” Presented at the 2017 Winter Simulation Conference (WSC), IEEE, Las Vegas, NV, 597–606. https://doi.org/10.1109/WSC.2017.8247818.
- Kwakkel, J. H., and E. Pruyt. 2013. “Exploratory Modeling and Analysis, an Approach for Model-Based Foresight Under Deep Uncertainty.” Technological Forecasting and Social Change 80 (3): 419–431. https://doi.org/10.1016/j.techfore.2012.10.005.
- Lamperti, F., G. Dosi, M. Napoletano, A. Roventini, and A. Sapio. 2020. “Climate Change and Green Transitions in an Agent-Based Integrated Assessment Model.” Technological Forecasting and Social Change 153: 119806. https://doi.org/10.1016/j.techfore.2019.119806.
- Lamperti, F., A. Mandel, M. Napoletano, A. Sapio, A. Roventini, T. Balint, and I. Khorenzhenko. 2019. “Towards Agent-Based Integrated Assessment Models: Examples, Challenges, and Future Developments.” Regional Environmental Change 19 (3): 747–762. https://doi.org/10.1007/s10113-018-1287-9.
- Lipman, B. L. 1995. “Information Processing and Bounded Rationality: A Survey.” The Canadian Journal of Economics 28 (1): 42. https://doi.org/10.2307/136022.
- Lippe, M., M. Bithell, N. Gotts, D. Natalini, P. Barbrook-Johnson, C. Giupponi, M. Hallier, et al. 2019. “Using Agent-Based Modelling to Simulate Social-Ecological Systems Across Scales.” Geoinformatica 23 (2): 269–298. https://doi.org/10.1007/s10707-018-00337-8.
- Lofdahl, C., and M. Voshell. 2018. “Challenges in Making Policy Decision-Support Systems Operational.” In Advances in Cross-Cultural Decision Making, Advances in Intelligent Systems and Computing, edited by M. Hoffman, 99–107. Cham: Springer International Publishing. https://doi.org/10.1007/978-3-319-60747-4_10
- Martin, R., and M. Schlüter. 2015. “Combining System Dynamics and Agent-Based Modeling to Analyze Social-Ecological Interactions—An Example from Modeling Restoration of a Shallow Lake.” Frontiers in Environmental Science 3. https://doi.org/10.3389/fenvs.2015.00066.
- Mischen, P. A., and S. K. Jackson. 2008. “Connecting the Dots: Applying Complexity Theoriy, Knowledge Management and Social Network Analysis to Policy Implementation.” Public Administration Quarterly 32: 314–338.
- Murray-Rust, D., V. Rieser, D. T. Robinson, V. Miličič, and M. Rounsevell. 2013. “Agent-Based Modelling of Land Use Dynamics and Residential Quality of Life for Future Scenarios.” Environmental Modelling & Software 46: 75–89. https://doi.org/10.1016/j.envsoft.2013.02.011.
- Naugle, A., S. Langarudi, and T. Clancy. 2024. “What is (Quantitative) System Dynamics Modeling? Defining Characteristics and the Opportunities They Create.” System Dynamics Review, sdr.1762. https://doi.org/10.1002/sdr.1762.
- Niamir, L., O. Ivanova, and T. Filatova. 2020. “Economy-Wide Impacts of Behavioral Climate Change Mitigation: Linking Agent-Based and Computable General Equilibrium Models.” Environmental Modelling & Software 134: 1–24. https://doi.org/10.1016/j.envsoft.2020.104839.
- Nilsson, M., A. Jordan, J. Turnpenny, J. Hertin, B. Nykvist, and D. Russel. 2008. “The Use and Non-Use of Policy Appraisal Tools in Public Policy Making: An Analysis of Three European Countries and the European Union.” Policy Sciences 41 (4): 335–355.
- Novak, J., and A. Cañas. 2006. The Theory Underlying Concept Maps and How to Construct Them (No. Technical Report IHMC CmapTools 2006-01 Rev 01-2008). Pensacola, FL: Florida Institute for Human and Machine Cognition (IHMC).
- Palm, A., and B. Lantz. 2020. “Information Dissemination and Residential Solar PV Adoption Rates: The Effect of an Information Campaign in Sweden.” Energy Policy 142: 111540. https://doi.org/10.1016/j.enpol.2020.111540.
- Parry, H. R., C. J. Topping, M. C. Kennedy, N. D. Boatman, and A. W. A. Murray. 2013. “A Bayesian Sensitivity Analysis Applied to an Agent-Based Model of Bird Population Response to Landscape Change.” Environmental Modelling & Software 45: 104–115. https://doi.org/10.1016/j.envsoft.2012.08.006.
- Pejic Bach, M., E. Tustanovski, A. W. H. Ip, K.-L. Yung, and V. Roblek. 2019. “System Dynamics Models for the Simulation of Sustainable Urban Development.” Kybernetes 49: 460–504. https://doi.org/10.1108/K-04-2018-0210.
- Podhora, A., K. Helming, L. Adenäuer, T. Heckelei, P. Kautto, P. Reidsma, K. Rennings, J. Turnpenny, and J. Jansen. 2013. “The Policy-Relevancy of Impact Assessment Tools: Evaluating Nine Years of European Research Funding.” Environmental Science & Policy 31: 85–95. https://doi.org/10.1016/j.envsci.2013.03.002.
- Rendall, R., L. H. Chiang, and M. S. Reis. 2019. “Data-Driven Methods for Batch Data Analysis – A Critical Overview and Mapping on the Complexity Scale.” Computers & Chemical Engineering 124: 1–13. https://doi.org/10.1016/j.compchemeng.2019.01.014.
- Ritchey, T. 2012. “Outline for a Morphology of Modelling Methods: Contribution to a General Theory of Modelling.” Acta Morphologica Generalis 1.
- Ruiz Estrada, M., and S.-F. Yap. 2013. “The Origins and Evolution of Policy Modeling.” Journal of Policy Modeling 35), https://doi.org/10.1016/j.jpolmod.2011.12.003.
- Sauer, A., and R. Ortlepp. 2021. “Parameter Uncertainties in Flood Hazard Analysis of Heavy Rain Events.” ASCE-ASME Journal of Risk and Uncertainty in Engineering Systems, Part A: Civil Engineering 7 (2): 04021016. https://doi.org/10.1061/AJRUA6.0001125.
- Scheller, F., and T. Bruckner. 2019. “Entity-Oriented Multi-Level Energy System Optimization Modeling.” In Operations Research Proceedings 2018, Operations Research Proceedings, edited by B. Fortz, and M. Labbé, 191–197. [Place of publication not identified]: Springer Nature. https://doi.org/10.1007/978-3-030-18500-8‗25
- Scheller, F., S. Johanning, and T. Bruckner. 2019. “Review of Designing Empirically Grounded Agent-Based Models of Innovation Diffusion.” Beiträge des Instituts für Infrastruktur und Ressourcenmanagement.
- Schlüter, M., A. Baeza, G. Dressler, K. Frank, J. Groeneveld, W. Jager, M. A. Janssen, et al. 2017. “A Framework for Mapping and Comparing Behavioural Theories in Models of Social-Ecological Systems.” Ecological Economics 131: 21–35. https://doi.org/10.1016/j.ecolecon.2016.08.008.
- Schrieks, T., W. J. W. Botzen, M. Wens, T. Haer, and J. C. J. H. Aerts. 2021. “Integrating Behavioral Theories in Agent-Based Models for Agricultural Drought Risk Assessments.” Frontiers in Water 3: Art. 686329, 1–19. https://doi.org/10.3389/frwa.2021.686329.
- Schrotter, G., and C. Hürzeler. 2020. “The Digital Twin of the City of Zurich for Urban Planning.” PFG – Journal of Photogrammetry, Remote Sensing and Geoinformation Science 88 (1): 99–112. https://doi.org/10.1007/s41064-020-00092-2.
- Schünemann, C., H. Herold, and A. Sidorova. 2023a. Qualitative und quantitative Modellierungen der Wirksamkeit von Politikinstrumenten. Möglichkeiten und Grenzen der Übertragbarkeit auf das Feld der Anpassung an den Klimawandel. (Teilbericht No. 41/2023), Climate Change. Umweltbundesamt, Dessau-Roßlau.
- Schünemann, C., A. Sidorova, and H. Herold. 2023b. Qualitative and Quantitative Modelling of the Efficacy of Policy Instruments (No. 42/2023), Climate Change. Umweltbundesamt, Dessau-Roßlau.
- Schwarz, N., G. Dressler, K. Frank, W. Jager, M. Janssen, B. Müller, M. Schlüter, N. Wijermans, and J. Groeneveld. 2019. “Formalising Theories of Human Decision-Making for Agent-Based Modelling of Social-Ecological Systems: Practical Lessons Learned and Ways Forward.” Socio-Environmental Systems Modelling 2: 16340. https://doi.org/10.18174/sesmo.2020a16340.
- Scott, R. 2018. Group Model Building. Using Systems Dynamics to Achieve Enduring Agreement, SpringerBriefs in Operations Research. Singapore: Springer.
- Siebers, P. O., C. M. Macal, J. Garnett, D. Buxton, and M. Pidd. 2010. “Discrete-event Simulation is Dead, Long Live Agent-Based Simulation!.” Journal of Simulation 4 (3): 204–210. https://doi.org/10.1057/jos.2010.14.
- Siegenfeld, A. F., and Y. Bar-Yam. 2020. “An Introduction to Complex Systems Science and Its Applications.” Complexity, 1. https://doi.org/10.1155/2020/6105872.
- Sterman, J. 2002. “System Dynamics: Systems Thinking and Modeling for a Complex World.” Massachussetts Institute of Technology. Engineering Systems Division (ESD) Working Paper Series ESD-WP-2003-01.13-ESD Internal Symposium.
- Sun, Z., I. Lorscheid, J. D. Millington, S. Lauf, N. R. Magliocca, J. Groeneveld, S. Balbi, et al. 2016. “Simple or Complicated Agent-Based Models? A Complicated Issue.” Environmental Modelling & Software 86: 56–67. https://doi.org/10.1016/j.envsoft.2016.09.006.
- Süsser, D., A. Ceglarz, H. Gaschnig, V. Stavrakas, A. Flamos, G. Giannakidis, and J. Lilliestam. 2021. “Model-Based Policymaking or Policy-Based Modelling? How Energy Models and Energy Policy Interact.” Energy Research & Social Science 75: 101984. https://doi.org/10.1016/j.erss.2021.101984.
- Turnpenny, J., M. Nilsson, D. Russel, A. Jordan, J. Hertin, and B. Nykvist. 2008. “Why is Integrating Policy Assessment so Hard? A Comparative Analysis of the Institutional Capacities and Constraints.” Journal of Environmental Planning and Management 51 (6): 759–775. https://doi.org/10.1080/09640560802423541.
- Uriona, M., and S. S. Grobbelaar. 2019. “Innovation System Policy Analysis Through System Dynamics Modelling: A Systematic Review.” Science and Public Policy 46 (1): 28–44. https://doi.org/10.1093/scipol/scy034.
- Valente, T. W., and E. M. Rogers. 1995. “The Origins and Development of the Diffusion of Innovations Paradigm as an Example of Scientific Growth.” Science Communication 16 (3): 242–273. https://doi.org/10.1177/1075547095016003002.
- Voinov, A., K. Jenni, S. Gray, N. Kolagani, P. D. Glynn, P. Bommel, C. Prell, et al. 2018. “Tools and Methods in Participatory Modeling: Selecting the Right Tool for the Job.” Environmental Modelling & Software 109: 232–255. https://doi.org/10.1016/j.envsoft.2018.08.028.
- Zhang, H., and Y. Vorobeychik. 2019. “Empirically Grounded Agent-Based Models of Innovation Diffusion: A Critical Review.” Artificial Intelligence Review 52 (1): 707–741. https://doi.org/10.1007/s10462-017-9577-z.