ABSTRACT
This study assessed the quality of Global Navigation Satellite System (GNSS) signal during professional football match play in different stadia with the application of a novel Data Quality Control Criteria (DQCC).
DQCC was applied to GPS-files from match play, derived using 10 Hz GNSS devices for 27 professional soccer players across a season to assess external load measures accounting for poor positioning quality (%) and horizontal dilution of precision. Performances were categorised on playing position (Wide or Central) to assess proximity to stand cover on GNSS signal quality.
An average reduction in total distance (11.2%), high-speed running distance (6.4%), sprint distance (7.0%), accelerations (10.3%) and decelerations (10.0%) (all P < 0.01) was observed upon DQCC application. In worst cases, 90% of an external variable was affected by poor-quality signal. Signal quality was worse for wide-positioned players than centrally positioned (positioning quality 2.6% lower (P < 0.01)), resulting in larger reductions of external variables upon DQCC application. Large stands in football stadia affect the data quality of GNSS and is exacerbated for players positioned closer to stand cover. Viewing only data with acceptable Position Quality and HDOP meaningfully reduce measured external loads, which has implications for the application of match data.
1. Introduction
Physical loads of professional footballers are measured using internal (acute physiological response) and external load (work output) (Impellizzeri et al., Citation2005; J. Malone et al., Citation2015; Vanrenterghem et al., Citation2017). External load can be manipulated to periodise acute fatigue in an attempt to achieve adaptations that improve performance (Heishman et al., Citation2018) and minimise injury risk (Gabbett, Citation2016). For example, high speed running (HSR) and sprinting are fundamental and stressful actions in football (Abbott et al., Citation2018a; Tierney et al., Citation2016), placing high load on lower limb muscles (Van den Tillaar et al., Citation2017), with large rapid changes in prescribed loads associated with increased injury risk (S. Malone et al., Citation2018; Van den Tillaar et al., Citation2017). Conversely, high chronic exposure to these actions can offer players enhanced protection that reduces lower limb injury risk associated with maximal sprinting (S. Malone et al., Citation2018). Accelerations have a relatively high energetic cost (Osgnach et al., Citation2010) and influence a player’s ability to arrive to a ball first or move into a space earlier than an opponent (Carling et al., Citation2008). Decelerations are negative accelerations, which involve eccentric muscle actions and have larger implications for muscle damage and recovery than accelerations 48 hours after exercise (Hewit et al., Citation2011; De Hoyo et al., Citation2016). Measuring these movements in match-play to then replicate in training is therefore important.
Global Navigation Satellite Systems (GNSS) devices are commonly used to measure external load in matches. Such devices make use of both US Global Positioning System (GPS) and Russian GLONASS satellites. These data collection occur in stadia where large stands can cover areas on the football pitch and interfere with GNSS signals and satellite availability, known as the multipath effect (Misra & Enge, Citation2011; Schuessler & Axhausen, Citation2009). The number of connected satellites can affect the accuracy of positional data, and thus external load measures such as total distance- and velocity-based measurements (Gray et al., Citation2010; Witte & Wilson, Citation2004). With the availability of Russian Global Navigation Satellite System (GLONASS), there is now an increased constellation of satellites that the units can communicate with. The implications of stadia interference and an increased availability of satellites (GPS and GLONASS) on GNSS measurements in soccer have not yet been investigated (Cai et al., Citation2013).
The positional arrangement of satellites affects GNSS accuracy and is quantified as the horizontal dilution of precision (HDOP) (Witte & Wilson, Citation2004). A HDOP of 1 represents an ideal distribution of satellites, whereby one satellite is directly overhead and the remainder are spaced equally across the horizon. Conversely, having all satellites positioned in a small space directly above the receiver leads to a higher HDOP and therefore a poorer arrangement of satellites (Person, Citation2008). In football stadia where stands can cover large areas of the pitch, stationary GPS devices reported the largest horizontal positional error when closest to the stadium (Williams & Morgan, Citation2009). However, to the authors’ knowledge, no research exists on data quality derived from GNSS in stadia while measuring moving players. Suppliers of GNSS hardware and software now provide more information regarding “Positioning Quality” using proprietary calculations. These calculations factor in the number and strength of connected satellites (CatapultSports, Citation2017), offering further opportunities to explore the multipath effect in stadia on GNSS measurements quality.
Validity of GNSS device measures are often undertaken in open settings (i.e. no stadium signal blockage/noise) that do not replicate the difficult environments presented in most modern-day stadia. By using signal quality measures provided by the GNSS manufacturers (HDOP and Positional Quality), the authors will use manufacturers suggestions to create a novel Data Quality Control Criteria (DQCC) to explore the implications of “poor” GNSS signal. This will scrutinise each data point of the GNSS files to verify it meets the signal quality criteria. Any data points which do not meet the criteria will be excluded from the calculation of external load measures, thereby allowing a comparison of external loads with and without the application of the DQCC. Accordingly, the purpose of this study was to assess the quality of GNSS signal during professional football match-play in stadia by applying recommended signal quality thresholds in a Data Quality Control Criteria on commonly used measurements of external load. Practitioners can, therefore, understand the magnitude of effect recommended signal quality measures have to give external load variables and its implication for physical load monitoring and reporting.
2. Method
Twenty-seven elite soccer players (mean ± SD: age = 28 ± 4 years, body mass = 81.7 ± 6.1 kg) representing the same team in the English Football League Championship participated in this study. Participants comprised five central defenders (CD), seven wide defenders (FB), eight central midfielders (CM), three wide attackers (WA) and five central strikers (CF). These positions were grouped into Central (CD, CM & CF) and Wide (FB & WA) position groups. Institutional ethics approval for the study was provided by the Faculty of Medicine, Dentistry and Life Sciences Ethics Committee (1530/19/AS/SES).
External load data were collected during 50 competitive fixtures in 24 stadia throughout the 2017–18 season. GNSS data files were only used for players who completed the entire match. There was a total of 339 GNSS match data files for full-matches across the cohort (Central Players n = 215, Wide Players n = 124). Thirty GNSS match files were removed from the database due to human errors during data collection. Players’ movement data during match-play were recorded using portable 10 Hz GNSS devices (CatapultSports™ OptimEye S5, Melbourne, Australia) worn between the scapulae in specially designed vests underneath the playing jersey. The OptimEye S5 device has acceptable reliability and validity when measuring distance covered (Jackson et al., Citation2018) and peak speed via the doppler shift method (Roe et al., Citation2017). The device also meets average industry standards of validity for measuring low to high-speed distances in non-peer reviewed research (FIFA, Citation2019). All devices were turned on in an open area of the pitch 45 min before data collection began to allow acquisition of satellite signals and ensure a satellite lock. After each match, GNSS data were downloaded using proprietary software (CatapultSports™ Openfield software; v1.17) where the match was split into first half and second half based on start and end times. Half time data points were not included in any calculations. Instantaneous unfiltered 10 Hz sensor data were imported into customised software (R Studio, v1.1.423) for the calculation of: total distance, HSR distance (>5.5 m·s−1) and number of efforts, sprint distance (>7 m·s−1) and number of efforts, acceleration distance (>2 m·s−2) and number of efforts and deceleration distance (>-2 m·s−2) and number of efforts. The thresholds used to calculate these variables are commonly used to identify high-speed workloads in soccer (Abbott et al., Citation2018a; Bradley et al., Citation2009; J. Malone et al., Citation2015). The minimum effort duration was at least 0.5 s to prevent efforts being detected from small spikes in data points above the given threshold (Varley et al., Citation2017). The end of an effort was identified at 1 s after the final data point falling below the given threshold to prevent multiple efforts being detected within the same effort.
All external load variables were calculated over the whole match period, “With” and “Without” the application of a DQCC. Positioning quality (%) and Horizontal Dilution of Precision (HDOP) were used in the DQCC. Positional quality (%) is designed to present a single percentage score to identify the quality of positioning data, with reference to positional arrangement, satellites connected and their strength. Positional quality is calculated by taking the signal to noise ratio from each satellite, sorting these according to their numerical value and providing them with a weighting factor. The stronger satellites are given a higher weighting in the quality total (CatapultSports, Citation2017). The Data Quality Control Criteria required positioning quality to be equal to or above 70% as per manufacturer’s suggestions (CatapultSports, Citation2017) and HDOP to be less than 2.0 as recommended in previous literature (CatapultSports, Citation2017; Gray et al., Citation2010; Weston et al., Citation2015). When applied, if the DQCC rules were violated, the data point was not included in the calculation of any external load variables. All data points were included in the “Without” DQCC external load variables. The DQCC therefore provides the same external load variables with the exclusion of poor signal quality data points. Duration of data-points violating DQCC rules were summed and labelled as poor-quality signal duration.
3. Statistical analysis
Data were initially checked for normality using the Shapiro-Wilk test (P > 0.05), with further checks for heteroscedastic errors (data with unequal variability across a set of second predictor variables) performed using Pearson’s product-moment correlation (r-value) between the measurement and its error. Heteroscedasticity was present for total distance only (r = 0.6). positioning quality, number of connected satellites, HDOP and poor-quality signal duration were analysed using box plot and whisker, with outliers defined as data points lower or higher than 1.5 times the interquartile range.
Separate repeated measures analysis of variance (ANOVA) were used to assess interactions between external variables and position group (external load x position group). Where an F value revealed a main effect, post hoc analysis using paired samples t-tests was used to calculate differences (biases) between means of variables for With and Without the DQCC. To further examine the influence of DQCC, the typical error (TE) (square root of the standard deviation of differences with and without DQCC) and coefficient of variation (CV = typical error/grand mean * 100) of external load metrics with and without DQCC applied was determined. The CV and typical error were also used to quantify the variation in positioning quality between each match. The magnitude of TE and CV were quantified in accordance with recommendations by Atkinson and Nevill, using suitable “analytical” goals (Atkinson & Nevill, Citation1998).
4. Results
illustrates GNSS signal quality and strength variables for all matches, with the interquartile range, median, range and outliers. Mean positioning quality was 77 ± 6%, with low value outliers ranging from 64% to 52%. Mean HDOP was 0.8 ± 0.1 for all matches. The mean number of connected satellites across all matches was 11.4 ± 0.7, with outliers as low as 8.1. Mean poor-quality signal duration across the matches was 11.6 ± 13.2 min, with high outliers ranging from 38.0 to 86.5 min. For mean positioning quality, values were lower for wide players compared to central players (Central = 78.2% ± 5%, Wide = 75.7% ± 5.2%, p < 0.01). For HDOP and poor-quality signal duration, values were higher for wide players compared to central players (HDOP; Central = 0.80 ± 0.11, Wide = 0.84 ± 0.11, p < 0.01. Poor quality signal duration; Central = 8.4% ± 11.1%, Wide = 16.5% ± 13.9%, p < 0.01).
Figure 1. Average Positioning Quality, Poor Quality Signal Duration, Horizontal Dilution of Precision and Number of Connected Satellites for All players, Centre Positions (Central Defender, Central Midfielder, Central Attacker) and Wide Positions (Wide Defender, Wide Midfielder, Wide Attacker)
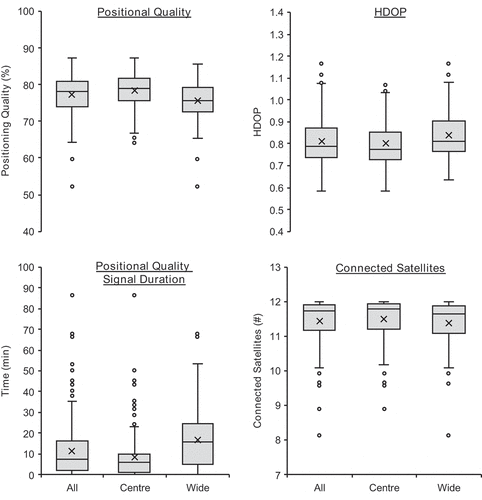
ANOVA revealed an interaction of position group and DQCC application for total distance (F = 35.1, p < 0.01), HSR (F = 25.1, p < 0.01), sprint distance (F = 19.7, p < 0.01), acceleration distance (F = 29.4, p < 0.01), deceleration distance (F = 32.8, p < 0.01), HSR efforts (F = 31.2, p < 0.01), sprint efforts (F = 15.4, p < 0.01), acceleration efforts (F = 29.3, p < 0.01), and deceleration efforts (F = 43.7, p < 0.01). Across all reported external variables, excluding maximum velocity, a larger reduction and poorer level of agreement was reported for wide players when compared with central players when DQCC was applied. show external variables with and without the application of DQCC, along with the coefficient of variation (CV) and typical error (TE).
Table 1. Distance-based external variables with and without Data Quality Control Critera (DQCC)
Table 2. Effort count external variables with and without Data Quality Control Critera (DQCC)
Table 3. Maximum Velocity with and without Data Quality Control Critera (DQCC)
5. Discussion
This study assessed the quality of the GNSS signal during professional football matches over an entire season, with and without the application of a DQCC. Despite mean measures of positioning quality and HDOP during match-play satisfying previously adopted criteria of acceptability (i.e. positioning quality ≧ 70%, HDOP ≦ 2) (CatapultSports, Citation2017; Gray et al., Citation2010), there were many individual performances that did not demonstrate acceptable signal quality. The application of DQCC resulted in changes to all measured GNSS variables, whilst wide-positioned players presented poorer signal quality than centrally positioned players and exhibited a poorer level of agreement with the application of DQCC.
While HDOP and the number of connected satellites are accepted GNSS signal quality measures used when assessing training load (Scott et al., Citation2016); these measures are often reported as means over an entire dataset (Abbott et al., Citation2018b), or not at all during data collection. In the present study, mean connected satellites were lower than reported in previous studies using the same model GNSS units while assessing training and match data (Abbott et al., Citation2018b) (current study = 11.4 ± 0.7 cf. previous studies = 14.7 ± 1.8). Abbott and colleagues’ study contained data from training and match play for an under 23s team (Abbott et al., Citation2018b). GNSS signal during data collection was less likely to be influenced by the stadia as under-23s teams do not play all matches in stadia. However, the mean HDOP reported in the current study was consistent with that of other studies during both match and training activity (Abbott et al., Citation2018b) and linear sprints of short duration (Varley et al., Citation2012). Taken together, these data highlight that despite being connected to fewer satellites our GNSS units provided, on average, a strong positional arrangement in stadia.
For the first time, this study evaluates the positioning quality proprietary measure provided by Catapult Sports, calculated by assessing and sorting signal to noise ratio from each satellite and providing them with a weighting factor (CatapultSports, Citation2017). In our study, a mean value of 77 ± 6% for positioning quality during match-play across the season is above the minimum value of 70% suggested by the manufacturer (CatapultSports, Citation2017)
This study also reported time spent with poor quality signal duration (positioning quality ≦ 70% and/or HDOP ≧ 2.0) was 11.6 ± 13.2 min (11.8% ± 13.5% minutes of a match). This implies that, on average, ~11 min of match-play was subject to poor GNSS signal and, consequently, questionable external load data. Moreover, some matches contained poor-quality signal duration of 66 to 87 minutes (), indicating the external load data for most of the match were compromised by poor signal.
The current study applied a Data Quality Control Criteria by providing external-load variables summed over the match excluding periods of poor-signal quality (positioning quality ≦ 70% and/or HDOP ≧ 2.0). We report that, on average, a player’s total distance during a match was reduced by 1158 m (8948 m in worst-case scenario) with the application of DQCC; there was also a 981 m (CV = 10%) variation in distance covered when comparing data with and without DQCC applied. The level of agreement between data collected with and without DQCC is poor when considered against an appropriate analytical goal. For example, practitioners often wish to determine the positional requirements associated with team sports. It has been reported that the difference in mean total distance during match play across a season between central defenders (9669 m) and central midfielders (10,395 m) is 726 m (−7%, p = 0.001) (Tierney et al., Citation2016). The current study suggests when we applied the manufacturer’s suggested DQCC, the mean reduction in total distance (~1158 m) is larger than the mean positional difference practitioners might wish to quantify (~726 m). As such, the difference in distance covered with DCQQ applied can be considered sufficiently large to substantially affect the interpretation of data. In the worst case, the current research identified an individual player presenting an 88% reduction of total distance with DQCC applied, questioning the integrity of most of the match data.
When DQCC was applied a mean reduction in HSR of 34 m (CV = 9%) was observed, with a reduction of 473 m (equating to 5.3 m·min−1; 94% reduction) in worst-case scenarios. These changes are meaningful when considered against differences between training and match-play intensities. For example, observed differences between HSR intensity in match-play (approx. 9.0 m·min−1) and moderate-sided games (~5 m·min−1) is approximately 4 m·min−1 (Abbott et al., Citation2018a). We suggest after DQCC application, the intensity of training drills such as moderate-sided games might be misrepresented as replicating match-play intensity that under-prepares players. Similarly, acceleration and deceleration distances were both reduced after DQCC was applied (26 and 16 m, respectively; CV = 11% and 16%, respectively), with worst case scenarios of 89% reductions.
Our results also show mean reductions of 0.15 m·s−1 in maximum velocity when DQCC is applied, which is larger than the measurement error of this GNSS unit (0.07 m·s−1) (Roe et al., Citation2017). The magnitude of the DQCC application is meaningful when considering positional differences in maximum velocity of 0.14 m·s−1 between central defenders and central midfielders, and 0.08 m·s−1 between wide defenders and forwards (Massard et al., Citation2018). In worst-case scenarios, up to 64% reductions were observed after DQCC application to maximum velocity readings of up to 23 m·s−1. The questionable validity of maximal velocity measured during match-play in stadia is an important observation given players’ maximum (or peak) velocity is often identified within match-play rather than training (Massard et al., Citation2018) and its important role in injury prevention and player preparation (S. Malone et al., Citation2018; Van den Tillaar et al., Citation2017).
Players in wide positions had a poorer GNSS signal (HDOP: Central = 0.80 ± 0.11, Wide = 0.84 ± 0.11, p < 0.01). Positioning Quality; Central = 78.2% ± 5%, Wide = 75.7% ± 5.2%, p < 0.01) and greater poor quality signal duration (Central = 8.4% ± 11.1%, Wide = 16.5% ± 13.9%, p < 0.01). when compared to central position players, suggesting that data for players who occupy wide positions on the pitch are likely to be more problematic for practical application. A likely explanation for the observed difference in signal quality is that units worn by wide-positioned players were subject to more cover from large stands surrounding the stadia, resulting in more noise and blockage to GNSS signal (Schuessler & Axhausen, Citation2009; Scott et al., Citation2016). Proximity to the stands, theoretically, affects the positional arrangement of the satellites, influencing the quality of the HDOP (Scott et al., Citation2016). Displacement of static units through positional errors of GNSS units have been found to be largest in those units closest to the stands (Williams & Morgan, Citation2009). Our findings reaffirm the influence of stadia on GNSS signal quality (i.e. canyon effect) and for the first time confirm this phenomenon on moving devices. Moreover, this study is the first to show that the data quality associated with playing in stadia is likely to affect “wide” players to a greater extent than central positioned players.
A direction for research could be to compare the external loads from GNSS devices to a criterion measure of movement, such as a 3-D motion capture system, to further assess the implications of the DQCC. This would enable an assessment of accuracy for the novel DQCC and should be the focus of future work. Also, despite the manufacturer suggesting a 70% threshold for acceptable positioning quality, future research will be required to investigate the validity of the threshold. Comparing the signal quality of the manufacturer’s units to other manufacturers’ units across a season of match-play would provide better information on the tolerance of challenging stadium environments.
We conclude that practitioners should be mindful of the magnitude of effect stadia can have on GNSS signal quality. Phenomena such as the multipath effect can affect positional arrangement of connected satellites, and therefore the validity of resulting GNSS-derived variables. GPS manufacturers should be encouraged to provide sufficient signal-quality measures to allow these assessments.
6. Practical applications
GNSS manufacturers should provide signal-quality measures for each data point to allow the user to scrutinise the GNSS signal quality to the level of the suppliers in this study.
The use of poor-quality signal duration can contextualise how the quality of signal might influence the measurement of important GNSS external load metrics.
Practitioners should take extra care when analysing external load data from match-play for players in wide positions as poor-quality GNSS signal could affect interpretations of the players’ outputs.
Acknowledgments
No financial assistance was provided in this project.
Disclosure statement
No potential conflict of interest was reported by the author(s).
References
- Abbott, W., Brickley, G., & Smeeton, N. J. (2018a). Positional differences in GPS outputs and perceived exertion during soccer training games and competition. The Journal of Strength & Conditioning Research,32(11), 3222-3231. https://doi.org/https://doi.org/10.1519/jsc.0000000000002387
- Abbott, W., Brickley, G., & Smeeton, N. J. (2018b). Physical demands of playing position within English Premier League academy soccer [Article]. Journal of Human Sport and Exercise, 13(2), 285–295. https://doi.org/https://doi.org/10.14198/jhse.2018.132.04
- Atkinson, G., & Nevill, A. M. (1998). Statistical methods for assessing measurement error (reliability) in variables relevant to sports medicine. Sports Medicine, 26(4), 217–238. https://doi.org/https://doi.org/10.2165/00007256-199826040-00002
- Bradley, P. S., Sheldon, W., Wooster, B., Olsen, P., Boanas, P., & Krustrup, P. (2009). High-intensity running in English FA Premier League soccer matches. Journal of Sports Sciences, 27(2), 159–168. https://doi.org/https://doi.org/10.1080/02640410802512775
- Cai, C. S., Liu, Z. Z., & Luo, X. M. (2013). Single-frequency Ionosphere-free Precise Point Positioning Using Combined GPS and GLONASS Observations [Article]. Journal of Navigation, 66(3), 417–434. https://doi.org/https://doi.org/10.1017/S0373463313000039
- Carling, C., Bloomfield, J., Nelsen, L., & Reilly, T. (2008). The Role of Motion Analysis in Elite Soccer Contemporary Performance Measurement Techniques and Work Rate Data [Review]. Sports Medicine, 38(10), 839–862. https://doi.org/https://doi.org/10.2165/00007256-200838100-00004
- CatapultSports. (2017). OpenField 1.17 released to streamline workflows and enhance sports science leadership | Catapult Sports. CatapultSports. https://www.catapultsports.com/blog/openfield-1-17-released
- de Hoyo, M., Cohen, D. D., Sanudo, B., Carrasco, L., Alvarez-Mesa, A., del Ojo, J. J., Dominguez-Cobo, S., Manas, V., & Otero-Esquina, C. (2016). Influence of football match time–motion parameters on recovery time course of muscle damage and jump ability. Journal of Sports Sciences, 34(14), 1363–1370. https://doi.org/https://doi.org/10.1080/02640414.2016.1150603
- FIFA. (2019). EPTS Performance Test Report Catapult S5. https://football-technology.fifa.com/media/172169/catapultgps-s5-epts-report-nov2018.pdf
- Gabbett, T. J. (2016). The training—injury prevention paradox: Should athletes be training smarter and harder? British Journal of Sports Medicine, 50(5), 273. https://doi.org/https://doi.org/10.1136/bjsports-2015-095788
- Gray, A. J., Jenkins, D., Andrews, M. H., Taaffe, D. R., & Glover, M. L. (2010). Validity and reliability of GPS for measuring distance travelled in field-based team sports [Article]. Journal of Sports Sciences, 28(12), 1319–1325. Article Pii 927093190. https://doi.org/https://doi.org/10.1080/02640414.2010.504783
- Heishman, A. D., Curtis, M. A., Saliba, E., Hornett, R. J., Malin, S. K., & Weltman, A. L. (2018). Noninvasive Assessment of Internal and External Player Load: Implications for Optimizing Athletic Performance. Journal of Strength and Conditioning Research, 32(5), 1280–1287. https://doi.org/https://doi.org/10.1519/JSC.0000000000002413
- Hewit, J., Cronin, J., Button, C., & Hume, P. (2011). Understanding Deceleration in Sport [Article]. Strength and Conditioning Journal, 33(1), 47–52. https://doi.org/https://doi.org/10.1519/SSC.0b013e3181fbd62c
- Impellizzeri, F. M., Rampinini, E., & Marcora, S. M. (2005). Physiological assessment of aerobic training in soccer [Article; Proceedings Paper]. Journal of Sports Sciences, 23(6), 583–592. https://doi.org/https://doi.org/10.1080/02640410400021278
- Jackson, B. M., Polglaze, T., Dawson, B., King, T., & Peeling, P. (2018). Comparing global positioning system and global navigation satellite system measures of team-sport movements. International Journal of Sports Physiology and Performance, 13(8), 1005–1010. https://doi.org/https://doi.org/10.1123/ijspp.2017-0529
- Malone, J., Di Michele, R., Morgans, R., Burgess, D., Morton, J. P., & Drust, B. (2015). Seasonal training-load quantification in elite English premier league soccer players. International Journal of Sports Physiology and Performance, 10(4), 489–497. https://doi.org/https://doi.org/10.1123/ijspp.2014-0352
- Malone, S., Owen, A., Mendes, B., Hughes, B., Collins, K., & Gabbett, T. J. (2018). High-speed running and sprinting as an injury risk factor in soccer: Can well-developed physical qualities reduce the risk? [Article]. Journal of Science and Medicine in Sport, 21(3), 257–262. https://doi.org/https://doi.org/10.1016/j.jsams.2017.05.016
- Massard, T., Eggers, T., & Lovell, R. (2018). Peak speed determination in football: Is sprint testing necessary? Science and Medicine in Football, 2(2), 123–126. https://doi.org/https://doi.org/10.1080/24733938.2017.1398409
- Misra, P., & Enge, P. (2011). Global Positioning System: Signals, Measurements, and Performance. Ganga-Jamuna Press.
- Osgnach, C., Poser, S., Bernardini, R., Rinaldo, R., & Di Prampero, P. E. (2010). Energy Cost and Metabolic Power in Elite Soccer: A New Match Analysis Approach [Article]. Medicine and Science in Sports and Exercise, 42(1), 170–178. https://doi.org/https://doi.org/10.1249/MSS.0b013e3181ae5cfd
- Person, J. (2008). Writing Your Own GPS Applications: Part 2. Code Project. https://www.codeproject.com/Articles/9115/Writing-Your-Own-GPS-Applications-Part-2
- Roe, G., Darrall-Jones, J., Black, C., Shaw, W., Till, K., & Jones, B. (2017). Validity of 10-HZ GPS and Timing Gates for Assessing Maximum Velocity in Professional Rugby Union Players. International Journal of Sports Physiology and Performance, 12(6), 836–839. https://doi.org/https://doi.org/10.1123/ijspp.2016-0256
- Schuessler, N., & Axhausen, K. (2009). Processing Raw Data from Global Positioning Systems Without Additional Information. Transportation Research Record: Journal of the Transportation Research Board, 2105(1), 28–36. https://doi.org/https://doi.org/10.3141/2105-04
- Scott, M. T. U., Scott, T. J., & Kelly, V. G. (2016). The Validity and Reliability of Global Positioning Systems in Team Sport: A Brief Review [Review]. Journal of Strength and Conditioning Research, 30(5), 1470–1490. https://doi.org/https://doi.org/10.1519/JSC.0000000000001221
- Tierney, P. J., Young, A., Clarke, N. D., & Duncan, M. J. (2016). Match play demands of 11 versus 11 professional football using Global Positioning System tracking: Variations across common playing formations. Human Movement Science, 49, 1–8. https://journals.lww.com/nsca-jscr/FullText/2018/12000/Quantification_of_a_Professional_Football_Team_s.26.aspx
- van den Tillaar, R., Solheim, J. A. B., & Bencke, J. (2017). COMPARISON OF HAMSTRING MUSCLE ACTIVATION DURING HIGH-SPEED RUNNING AND VARIOUS HAMSTRING STRENGTHENING EXERCISES [Article]. International Journal of Sports Physical Therapy, 12(5), 718–727. https://doi.org/https://doi.org/10.26603/ijspt20170718
- Vanrenterghem, J., Nedergaard, N. J., Robinson, M. A., & Drust, B. (2017). Training Load Monitoring in Team Sports: A Novel Framework Separating Physiological and Biomechanical Load-Adaptation Pathways [Article]. Sports Medicine, 47(11), 2135–2142. https://doi.org/https://doi.org/10.1007/s40279-017-0714-2
- Varley, M. C., Fairweather, I. H., & Aughey, R. J. (2012). Validity and reliability of GPS for measuring instantaneous velocity during acceleration, deceleration, and constant motion [Article]. Journal of Sports Sciences, 30(2), 121–127. https://doi.org/https://doi.org/10.1080/02640414.2011.627941
- Varley, M. C., Jaspers, A., Helsen, W. F., & Malone, J. J. (2017). Methodological Considerations When Quantifying High-Intensity Efforts in Team Sport Using Global Positioning System Technology. International Journal of Sports Physiology and Performance, 12(8), 1059–1068. https://doi.org/https://doi.org/10.1123/ijspp.2016-0534
- Weston, M., Siegler, J., Bahnert, A., McBrien, J., & Lovell, R. (2015). The application of differential ratings of perceived exertion to Australian Football League matches. Journal of Science and Medicine in Sport, 18(6), 704–708. https://doi.org/https://doi.org/10.1016/j.jsams.2014.09.001
- Williams, M., & Morgan, S. (2009). Horizontal positioning error derived from stationary GPS units: A function of time and proximity to building infrastructure. International Journal of Performance Analysis in Sport, 9(2), 275–280. https://doi.org/https://doi.org/10.1080/24748668.2009.11868483
- Witte, T. H., & Wilson, A. M. (2004). Accuracy of non-differential GPS for the determination of speed over ground [Article]. Journal of Biomechanics, 37(12), 1891–1898. https://doi.org/https://doi.org/10.1016/j.jbiomech.2004.02.031