ABSTRACT
Running field tests are utilised to assess the athlete’s fitness level with high specificity. However, in graded exercise tests the lack of speed control can result in an uneven pace, reducing reproducibility and accuracy. The purpose of this study was to develop feedback variants (FV) and investigate their effect on the athlete’s ability to keep the pace constant. Forty-eight participants completed four trials of a Conconi test (randomised) over four paces (7.5 − 10 km·h−1). A smartphone app provided athletes with four FVs: Classic, Sound (verbal), Vibration, and Sound & Vibration. We found a significant effect of FV on adherence (defined as time within 0.3 km·h−1 of target speed): F(3,141) = 41.45, p < .001, η2 = .268. Adherence in Classic (M± SD) 39.51 ± 13.51% was significantly lower (p < .001) than in Vibration (53.24 ± 14.98%), Sound (60.34 ± 11.21%) and Sound & Vibration (59.17 ± 15.17%). The novel FV provided better adherence compared to the conventional method, with Sound and Sound & Vibration showing the highest adherence. Generally, the novel FVs have the advantage of being easily implemented and decreasing the required time for setup, data collection and processing, therefore proving useful in conducting field performance tests.
KEYWORDS:
1. Introduction
Running in its different forms (jogging, sprinting, short or long distance running) is an ever-popular sport performed by numerous people (Schnohr et al., Citation2013). One of the reasons for its popularity might stem from the fact that it is a simple sport requiring little equipment. Moreover, several studies have shown that running is associated with physical and mental benefits (Lee et al., Citation2014; Schnohr et al., Citation2013). Consequently, running is picked up by a growing number of people with a significant proportion of them aiming to train methodologically in order to increase the physical and mental benefits (Etxegarai et al., Citation2018; Vickers & Vertosick, Citation2016).
To be efficient, training needs to be performed within the individual optimal intensity zones depending on the individual goals. One way to identify individual intensity zones is to implement various field performance tests in the training protocol. Field tests vary from covering as much distance as possible over a fixed period such as the Cooper test (Cooper, Citation1970) to graded exercise tests such as the Conconi test (Conconi et al., Citation1982) or the “Université de Montréal track test” (Uger & Boucher, Citation1980). In a graded exercise test athletes are required to run at a fixed pace for either a given duration or distance (Conconi et al., Citation1982; Uger & Boucher, Citation1980). The target pace is increased in a spatial (Conconi et al., Citation1982) or temporal manner (Uger & Boucher, Citation1980) until the athlete is no longer able to continue. Based on measured values such as heart rate, blood lactate or oxygen consumption, several different thresholds can be computed (Conconi et al., Citation1982; Uger & Boucher, Citation1980). These are later used by coaches for setting training volume and intensity of their athletes.
Any performance test needs to be objective, reliable, valid and reproducible in order to be useful. Reproducibility of a performance test can be ensured by requiring athletes to run at a predetermined speed and for that reason tests are often performed on a treadmill in a laboratory where the conditions can be easily controlled. However, laboratory tests are often criticised for not reflecting the competitive environment (Nigg et al., Citation1995; Sinclair et al., Citation2013). Therefore, numerous field tests such as the Cooper or Conconi test have been implemented so that the athlete can perform their usual physical activity. During these tests, the examiner has to control whether the athletes perform at the desired intensities and stop the test if athletes fail to comply.
During running tasks, the process or strategy behind the distribution of speed or energy is defined as pacing (Pryor et al., Citation2020). Pacing is not only essential for performance testing but also in running competitions. For flat running courses, the so-called “even pacing” is a strategy often applied by athletes in order to achieve the best results (Abbiss & Laursen, Citation2008; Hanley, Citation2015). While, traditionally, pace-related information was only provided to an athlete by a coach, it can now be given by a technical device through acoustic, optic or haptic feedback. These devices are commonly referred to as feedback systems (Baca, Citation2008). A simple type of feedback system can take the form of a sports watch. More sophisticated systems can also employ mobile technology such as smartphones or tablet computers. For example, Tate and Milner (Citation2017) used a tablet computer to visualise noise levels during treadmill running in order to help participants reduce impact forces. With the help of the so-called PerPot model a smartphone-based feedback system has been shown to help novice athletes to perform a 10 km trial by means of verbal messages (Dobiasch et al., Citation2018). Furthermore, smartphones have proven effective in assisting runners by keeping their cadence constant by employing verbal messages, vibrations, or auditory cues (e.g. beating-rhythm) (Aranki et al., Citation2018; Balvis et al., Citation2016). However, these systems are tailored for activities with constant pace rather than changing paces.
The feedback provided by such a system can be classified by its modality into tactile, auditory or visual (Sigrist et al., Citation2013). Furthermore, the timing of feedback can be categorised as either terminal (after the action) or concurrent (during the action) (Lauber & Keller, Citation2014). Usually, sports watches provide concurrent feedback about the heart rate and speed of the athlete. Moreover, modern systems also offer online platforms where terminal feedback in the form of diagrams and computed metrics are available for further analysis (e.g. Strava, Strava Inc., USA or TrainingPeaks, Peaksware LLC, USA).
While for professional runners the usage of technical aids that provide external feedback is or has been forbidden for some competitive events (e.g. IAAF Regulations C2.1 Rule 144/6.4.4 (IAAF, Citationn.d.)), recreational runners are usually allowed to use them. Setting up a sports watch to achieve an “even pacing” during a race or training session can be a fairly simple task. Nevertheless, setting up other profiles can be cumbersome and, moreover, most systems do not offer support for adaptive profiles. Furthermore, setting up an incremental test can be quite a difficult and error-prone task. Most commercially available systems do not allow setting up individual stop criteria in case of an athlete no longer able to maintain the desired pace. Consequently, the adoption of sports watches or other systems for pacing is not yet pervasive enough.
The effects of mobile feedback systems have been studied with recreational and professional athletes. For example, a previous study by Strohrmann et al. (Citation2014) found that arm carriage can be improved while using vibration feedback similarly as if instructed by a human. Furthermore, Fortmann et al. found that auditory feedback might help experienced runners maintain a constant cadence during training (Fortmann et al., Citation2012), while Abernethy et al. examined and reported the influence of gait modes (preferred and non-preferred gait speeds) on cognition using an auditory probe reaction task (Abernethy et al., Citation2002).
Feedback systems are also used for injury prevention and recovery processes which is why several research applications aiming to modify the running pace of athletes have been developed (Agresta & Brown, Citation2015; Napier et al., Citation2015). For example, Van Gelder et al. showed that visual feedback might be able to help athletes reduce or control tibial acceleration during treadmill running (Van Gelder et al., Citation2018). Additionally, Sheerin et al. found that the use of tactile feedback was as effective as the visual counterpart when retraining runners to reduce tibial acceleration (Sheerin et al., Citation2020). Similarly, Wood and Kipp (Citation2014) showed a reduction in peak acceleration using “thresholds sounds” (i.e. a sound was played to participants when acceleration exceeded a threshold) in their study. Moreover, a study by Afzal et al. showed that tactile feedback using vibrations could help stroke patients to achieve gait symmetry (Afzal et al., Citation2019).
Previous studies have mostly investigated the effects of feedback at a constant pace. However, this does not reflect the varying intensities used in many testing protocols. Therefore, the main goal of this study is to examine the resulting adherence (defined as the time spent within 0.3 km·h−1 of target speed) of different feedback mechanisms (haptic, visual, and auditory) during different running pace levels. In order to investigate the effects of the feedback variants on adherence, we created a custom application for smartphones which makes the results easily applicable for everyday users as well as practitioners and athletes. We hypothesised that for recreational athletes, different feedback mechanisms will have different effects on pace adherence. Moreover, we expect that novel feedback variants (vibration, sound or sound and vibration) will lead to better results compared to classical acoustic signals.
2. Methods
2.1. Participants
Initially, 55 participants were recruited for the study; however, seven were excluded due to an insufficient number of completed stages in the trial runs. Therefore, a total of 48 recreational runners (Age: 26 ± 3.8 years, Mass: 72 ± 11.88 kg, Height: 177 ± 9.81 cm, 33 males, 15 females) participated in the study and completed all feedback runs. Participants reported to actively participate in 3 to 20 hours of various sports activities (running, swimming, weightlifting and cycling) per week. The study was approved by the ethics committee of the University of Vienna. Written informed consent was obtained from all participants prior to the experiment. Additionally, participants were asked to fill out a cardiovascular risk assessment questionnaire based on the ACSM guidelines (Thompson et al., Citation2010).
2.2. Protocol
The participants were equipped with a heart rate monitor (Garmin Soft Strap HRM, Switzerland) and a Foot Pod device (Garmin SDM4, Switzerland) to measure heart rate and speed/distance, respectively. Additionally, questions about the sporting background of the participants were asked such as whether they had performed running tests before and whether they train at a fixed speed (possible answers: “never”, “sometimes”, “often”). In order to reduce learning effects subjects were randomly assigned to a sequence of the feedback variants (Classic, Vibration, Sound, Sound & Vibration) based on their arrival on site.
Before testing, the participants performed a preliminary run over 800 m. This calibration run, which also served as a warm-up, was a self-paced run and was used to obtain the calibration factor for the Foot Pod device. During this run, the speed was (M± SD) 10.59 ± 1.27 km·h−1, 95% confidence interval: 10.22 − 10.96 km·h−1 with a mean coefficient of variation of 19.38 ± 7.12%, 95% CI: 17.31 − 21.44%.
For the purpose of the study, an app was created using the PEGASOS framework (Dobiasch et al., Citation2016, Citation2019) that records data from the heart rate monitor (1 Hz) and Foot Pod synchronously (2 Hz). Speed was filtered using a five-second rolling average filter. This setting was based on the nature of the task (running with constant speed) and prior experiments. The app was installed on a Samsung A3 (2017 version) smartphone with a standard Android 8.0 operating system. By means of verbal explanation of the feedback variants and the testing procedure, participants were familiarised with the technical aspects of the app and the feedback signals. Additionally, prior to testing in order to get accustomed, we presented the participants with a sequence of the feedback signals. We standardised the position of the phone on the upper arm and secured it with a commercially available phone holster. During the familiarisation period participants were questioned about whether they could sense the vibrations of the phone. In case of a negative response, the phone was repositioned on the anterior part of the lower arm.
For the Classic variant (see below) participants were asked to start approximately 10 m before the first cone. For all other feedback variants, the trial started when the participant reached an initial target speed of 7 km·h−1. The participants then had to complete 200 m stages, while the speed increased by 0.5 km·h−1 with each stage. A trial was completed (irrelevant of where the athlete was) when one of the following criteria was met: either the athlete reached 90% of their self-reported maximal heart rate or the participant stopped running. Between two trials, a break of at least 20 min was implemented to allow participants to recover from the previous run.
Post-trial participants were asked to subjectively rank the feedback variants according to their personal preferences.
2.3. Feedback variants
In this study, four different feedback variants were examined a conventional variant (Classic) and three novel variants (Vibration, Sound and Sound & Vibration).
The Classic variant resembled the conventional method of the Conconi test. Cones were placed around the running track at a distance of 20 m between each other and correct positioning was ensured, as the track was equipped with markers for that specific purpose. The feedback consisted of an acoustic (beep) signal which was issued at predefined intervals. Participants were instructed to be at the next cone whenever a beep was sounded. At the beginning of the trial participants were instructed to start a few metres behind their first cone. An automatic countdown was applied to assist participants in their spatio-temporal adjustment before the first cone. Furthermore, participants were instructed to adjust their speed in order to reach the respective cone with their hip whenever a beep sound was signalled.
While the Classic variant had only one feedback signal, each of the novel feedback variants consisted of two different feedback signals: one for running slower and one for running faster than the target speed, while no feedback was given when participants were running at the target speed. A minimal interval of at least 5 s was ensured between any two consecutive feedback signals in order to avoid information overload.
The Vibration variant used two different vibration patterns for the feedback signals. The “too slow” signal consisted of 200 ms vibrations followed by a 150 ms break and the “too fast” signal consisted of 1000 ms vibrations followed by a 250 ms break. Each of these patterns was repeated three times whenever a participant did not run at a predefined pace.
The Sound variant used the Text-to-Speech software of the phone to provide “verbal” feedback. Since participants were expected to be fluent in German, commands were given in this language. The voice commands were “Zu langsam” (“too slow”) or “Zu schnell” (“too fast”) for signalling deviations from the target speed. Since Text-to-Speech software relies on pre-installed voices to convert text to speech, the phones were configured to use the pre-installed voice provided by Google (Google LLC, USA).
The Sound & Vibration variant combined the feedback signals of the two variants Sound and Vibration. Owing to that the phone vibrated with the same patterns as described above while simultaneously giving verbal feedback whenever a participant was outside the predefined pace.
Additionally, we also examined the participants’ preference of the feedback variant in order to identify possible individual preference effects on adherence.
2.4. Adherence
We defined adherence of a trial as the relative amount of time spent within ± 0.3 km·h−1 of the assigned target speed. Previous pilot trials revealed that 0.3 km·h−1 deviation from the target speed was sensitive enough to detect inaccuracies at a given running pace. Both feedback and calculation of adherence were based on the filtered (five-second rolling average) speed data sent from the Foot Pod device. For data cleaning purposes, the first and last – potentially incomplete – stage was removed from the analysis.
2.5. Statistics
Calculations for this study were conducted with R version 4.0.3 (R Core Team, Citation2020). Effects were assessed using repeated-measures ANOVA. Sphericity was tested using Mauchly’s Test. The Greenhouse Geiser correction was used when sphericity could not be assumed. Effect sizes were calculated using the partial eta squared (η2). We used post-hoc analysis using Bonferroni correction in case of significant main effect.
3. Results
We found a significant main effect of feedback variant on adherence (F(3,141) = 41.45, p < .001, η2 = .268) (). A subsequent Bonferroni post hoc analysis revealed significant differences between the feedback variants (). The Classic variant (39.51 ± 13.51%) produced lowest adherence and differed significantly from Vibration (53.24 ± 14.98%): p < .001), Sound (60.34 ± 11.21%): p < .001) and Sound & Vibration (59.17 ± 15.17%): p < .001 (). Moreover, Vibration differed significantly from Sound (p = .010) and, additionally, a tendency for significant difference was observed between Vibration and Sound & Vibration (p = .051) (). However, no difference was found between Sound and Sound & Vibration (p > 0.999) ().
Figure 1. Boxplot of adherence achieved with the feedback variants during complete trials (n = 48). *** significant difference (p < .001). ** significant difference (p < .01)
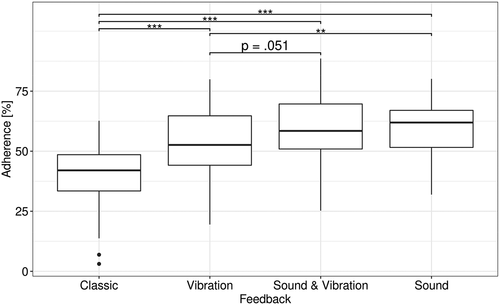
In order to have a consistent number of participants at each target speed, trials were only included up to the 10kmh−1 stage. We found a significant effect of target speed on the adherence in the four different feedback variants (F(5,235) = 9.04, p < .001, η2 = .098, F(5,235) = 4.65, p < .001, η2 = .054, F(5,235) = 9.80, p < .001, η2 = .119, F(5,235) = 5.61, p < .001, η2 = .065 for the Classic, Vibration, Sound and Sound & Vibration variants, respectively; ). To further examine changes in adherence over the course of the trials we compared the coefficient of variation (CV) of adherence in relation to target speed. This analysis provides more insight on the reasons for low adherence: high variability can induce low adherence. A significant difference in CV of adherence between target speeds was found (χ2(5) = 14.286, p = .014) using Friedman’s test ().
Figure 2. Changes in adherence with increasing speed for the different feedback variants. Each sub figure shows the mean adherence (y-axis) at a given target speed (x-axis) for a feedback variant (n = 48). Data was only included up to the 10kmh−1 stage in order to have an equal amount of values per target speed. *** significant difference (p < .001), ** significant difference (p < .01), * significant difference (p < .05)
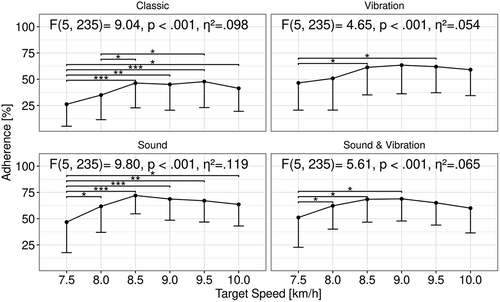
No effect of trial on adherence could be identified using a repeated-measures ANOVA (F(3,141) = 0.25, p = .865, η2 = .003). The mean adherence for the respective trials was (M± SD): 52.90 ± 16.33% in the first, 52.80 ± 16.41% in the second, 52.08 ± 18.09% in the third and 54.47 ± 13.27% in the last trial.
We found no effect of athletes’ participation in previous running tests on adherence (F(1,46) = 0.52, p = .476, η2 = .007) and, furthermore, the results showed no effect of whether participants reported training at fixed speeds (F(2,45) = 2.11, p = .134, η2 = .051).
A repeated measures ANOVA showed a significant effect of preference on adherence (F(2.49,117.16) = 3.31, p = .030, η2 = .038) (). However, the post-hoc analysis did not show significant differences in adherence between the variants when ordered according to the preference of the athletes (). Nevertheless, a tendency to a difference between the first (48.60 ± 16.70%) and second preference (57.37 ± 16.86%) could be observed (p = .057) (). Feedback variants ordered according to the preferences of each participant individually are presented in .
Figure 4. Adherence of the different feedback variants ordered by preference and the corresponding rankings by the athletes. (a) shows differences between the feedback variants preferred by the athletes. Pref. 1–4 describes the order of feedback variant preferred by the athletes. (b) bars indicating the frequency of a feedback ranked in the respective preference (1–4) by the athletes
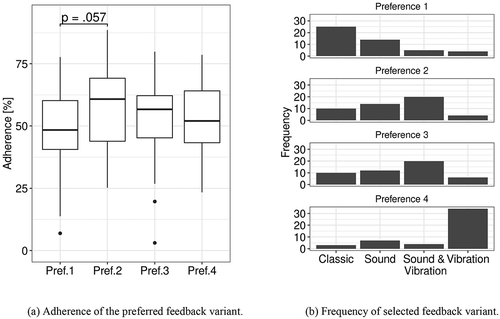
4. Discussion
The main result of this study was that a significant effect of feedback variants on mean adherence has been found. The three novel variants showed significantly higher adherence than the classic feedback variant.
A possible explanation for the low adherence of the classical variant could be the frequency of feedback. While the novel variants provide continuous feedback, the conventional variant provides feedback only whenever an athlete is at a specific cone. For example, at 10 km·h−1 the maximal amount of feedback over 200 m could be 10 while with the novel variants it can reach ~14 or even ~19 signals at the lower target speeds (7.5 km·h−1). This type of feedback is decoupled from the athlete’s pace as feedback is given at fixed time intervals and, as a consequence, athletes will receive feedback only in relation to a cone and not in relation to their actual pace.
Another explanation could be the way the feedback is used by the participants. While the novel variants do not allow athletes the choice of pacing since they provide a constant pace control, athletes using the conventional variant can control their own pace throughout the short duration interval. Each interval is 20 m long, and the participant is left with a choice on how to pace it. Owing to that some participants admitted (personal communication) having applied a strategy optimise cone timing in order to be at the next cone at the right time rather than running at a constant speed. As a consequence, the participants could have run faster or slower at some points during an interval or might even have had to wait at the cone. This could have resulted in a lower adherence to the classic variant.
The second important result of this study was the significant differences in adherence between different target speed levels. A common result for all variants was the increased adherence over the first three stages (observable in ). It appears that after the 8.5 km·h−1 target speed a plateau was reached and no further increase in adherence could be observed. One explanation for the decreased adherence during the first three stages might be the preferred running speed of the athletes. Most athletes reported (personal communication) that they were not usually running at speeds as low as the target speeds of the first three stages. This can be supported by the fact that the calibration speed of the participants, which could be seen as the preferred speed, exceeded 10 km·h−1. Consequently, a higher proportion of their attention could be placed on their running technique (Abernethy et al., Citation2002). We additionally hypothesised that due to the higher attention required when running at non-preferred speeds, participants were less accurate. To test this hypothesis, we additionally compared the coefficient of variation (CV) of adherence of the different feedback variants and target speeds. We expected that at target speeds lower than the preferred speeds the participants would adjust their pace more than they would at higher speeds, thus resulting in a higher variation in adherence. The results show significant differences in CVs between the target speeds (). This is also apparent in the combined CV, where at the first two target speeds the coefficient of variation shows the highest values and then reaches a plateau (8.5 km·h−1), indicating that there is probably a threshold or preferred speed where the speed variation is minimised. Therefore, we can conclude that the participants tend to run with less variation at their preferred pace rather than the non-preferred pace.
Next, to notice is that the pure vibration feedback had lower adherence on average than the feedback variants with sound (Sound, Sound & Vibration). The reason for this difference could lie in difficulties with sensing the vibrations or sensing differences between the vibration patterns. Some participants reported difficulties sensing the vibrations or distinguishing between the patterns during running. This can also be supported by the findings of the participants’ preference where the vibration variant was the least preferred among the four (). However, it seems that the ability to sense vibrations depends on the task and individual since other studies reported no difficulties (Strohrmann et al., Citation2014). One factor that could contribute to the lower sensitivity might have been the clothing of the athletes as some participants wore a jacket during the entire testing session. Additionally, some participants further reported problems sensing differences between the two patterns, which could be related to the low pulse intensity produced by the phones. However, as the aim of this study was to find a simple way of providing feedback, and having additional devices such as an external vibration motor as previously described (Van Gelder et al., Citation2018) would have been beyond the scope of this study. Since other studies showed good results when using tactile feedback provided by an external apparatus (Afzal et al., Citation2019; Sheerin et al., Citation2020), future research should investigate the difference between phone vibrations and vibrations provided by dedicated external motor devices on pace adherence.
Another important finding of the present study is that the results did not yield significant differences between the trials. Firstly, this may suggest that the recovery time between the trials was sufficient for participants and thus the adherence was not decreasing due to fatigue. Secondly, this indicates that the trial order of the participants did not affect the outcome of the study. Additionally, it can be assumed that none of the feedback variants provoked a significant learning effect for the participants and, therefore, we can conclude that the results are not skewed by participants who might have prior experience with running tests. Furthermore, it can be assumed that the pacing behaviour is not altered by a single session.
A further finding from this study shows an effect of subjective feedback preference on adherence was found. This can be observed in where the first preference of participants was the Classic variant, which provided the lowest overall adherence (). Additionally, the Vibration variant was the least preferred among the variants () although it showed higher adherence than the classic one but less than the other novel types. These results could indicate that the problems associated with sensing the vibration (see discussion above) could play an important role in the test procedure and hence influence the results. Since the Classic variant showed the least adherence and the Vibration variant was the least preferred, we could suggest that the other novel types (Sound, Sound & Vibration) should be chosen for incremental performance speed tests.
5. Limitations
One limitation of this study is the lack of a true speed measurement (e.g. timing gates) since it was only assessed using a Foot Pod device. It can be argued that the Foot Pod can only accurately measure the speed for speeds close to the calibration speed, while for other values (lower than the target speed) the device would over- or underestimate the measured speed. However, since the average calibration speed of the participants was 10.59 ± 1.27 km·h−1 (95% confidence interval: 10.22 − 10.96 km·h−1), which was higher than the maximal target speed (10 km·h−1), any possible systematic error (under- or overestimation) in speed measurement would not affect the main outcome of this study, which the higher feedback frequency of the novel variant required the participants to keep the scheduled pace and hence increase the adherence.
The validity and reliability of the speed values calculated by the used Foot Pod device may constitute a limitation of this study. Whereas Mahendran et al. (Citation2016), Willy et al. (Citation2016), and Han et al. (Citation2020) report a high reliability or accuracy of the steps count, there is a lack of respective studies on speed values. However, since the same approach was implemented for all participants and feedback variants, a systematic error should probably not change the main outcome of this study, that is, the novel feedback variants showed a better adherence than the classical method.
Another limitation could be the nature of the task used for the evaluation of the feedback variants. Due to the stepwise increase in target speeds the results might not apply to other tasks such as variable pacing on uneven tracks. However, the protocol was chosen in order to reflect typical field testing scenarios. Nevertheless, future work should investigate whether the findings of this study apply to variable pacing as well.
Finally, the authors would like to point out that the testing protocol was based on the Conconi test just for simplicity. This study neither proves nor refutes the validity of the original protocol. The presented feedback variants could also be applied to any other protocol where running at a fixed pace is required.
6. Conclusion
The results of this study suggest that mobile feedback systems might be a valuable tool for athletes and coaching staff to conduct (fitness) tests due to their increased adherence. Moreover, as a result of their ease of use they might decrease the time required for setting up the testing site and can also reduce the effort required for data collection and further processing.
Disclosure statement
No potential conflict of interest was reported by the author(s).
Additional information
Funding
References
- Abbiss, C. R., & Laursen, P. B. (2008). Describing and understanding pacing strategies during athletic competition. Sports Medicine, 38(3), 239–252. https://doi.org/https://doi.org/10.2165/00007256-200838030-00004
- Abernethy, B., Hanna, A., & Plooy, A. (2002). The attentional demands of preferred and non-preferred gait patterns. Gait & Posture, 15(3), 256–265. https://doi.org/https://doi.org/10.1016/S0966-6362(01)00195-3
- Afzal, M. R., Lee, H., Eizad, A., Lee, C. H., Oh, M. K., & Yoon, J. (2019). Effects of vibrotactile biofeedback coding schemes on gait symmetry training of individuals with stroke. IEEE Transactions on Neural Systems and Rehabilitation Engineering : A Publication of the IEEE Engineering in Medicine and Biology Society, 27(8), 1617–1625. https://doi.org/https://doi.org/10.1109/TNSRE.2019.2924682
- Agresta, C., & Brown, A. (2015). Gait retraining for injured and healthy runners using augmented feedback: A systematic literature review. Journal of Orthopaedic and Sports Physical Therapy, 45(8), 576–584. https://doi.org/https://doi.org/10.2519/jospt.2015.5823
- Aranki, D., Peh, G., Kurillo, G., & Bajcsy, R. (2018). The feasibility and usability of running coach: a remote coaching system for long-distance runners. Sensors, 18(2), 175. https://doi.org/https://doi.org/10.3390/s18010175
- Baca, A. (2008). Feedback systems. In P. Dabnichki & A. Baca (Eds.), Computers in Sport (pp. 43–67). WIT Press. https://doi.org/https://doi.org/10.2495/978-1-84564-064-4/02
- Balvis, L., Boratto, L., Mulas, F., Spano, L. D., Carta, S., & Fenu, G. (2016). Keep the beat: Audio guidance for runner training. In C. Bogdan, J. Gulliksen, S. Sauer, P. Forbrig, M. Winckler, C. Johnson, P. Palanque, R. Bernhaupt, & F. Kis (Eds.), Human-centered and error-resilient systems development (Vol. 9856, pp. 246–257). Springer International Publishing. https://doi.org/https://doi.org/10.1007/978-3-319-44902-9_16
- Conconi, F., Ferrari, M., Ziglio, P. G., Droghetti, P., & Luciano, C. (1982). Determination of the anaerobic threshold by a noninvasive field test in runners. Journal of Applied Physiology, 52(4), 869–873. https://doi.org/https://doi.org/10.1152/jappl.1982.52.4.869
- Cooper, K. H. (1970). The new aerobics. Bantam Books Inc.
- Dobiasch, M., Endler, S., & Baca, A. (2018). Development and evaluation of a feedback System for endurance running (PerPot-live). In H. Dancs, M. Hughes, A. Penichet-Tomás, J. Gaillard, & A. Baca (Eds.), Recent researches in sport science, 41-48.
- Dobiasch, M., & Baca, A. (2016). Pegasos – Ein Generator für Feedbacksysteme. In dvs (Ed.), Sportinformatik 2016. 11. Symposium der dvs Sportinformatik, 14.-16. September 2016 (p. 18). Magdeburg, Germany.
- Dobiasch, M., Stöckl, M., & Baca, A. (2019). Direct mobile coaching & a software framework for the creation of mobile feedback systems. Book of abstracts. 12th International Symposium on Computer Science in Sport. IACSS 2019, Moscow, Russia, 8–10 July 2019, 109–110.
- Etxegarai, U., Insunza, A., Larruskain, J., Santos-Concejero, J., Gil, S. M., Portillo, E., & Irazusta, J. (2018). Prediction of performance by heart rate-derived parameters in recreational runners. Journal of Sports Sciences, 36(18), 2129–2137. https://doi.org/https://doi.org/10.1080/02640414.2018.1442185
- Fortmann, J., Buscher, M., Mittelsdorf, M., Trienen, S., Pielot, M., & Boll, S. (2012). PaceGuard: Improving running cadence by real-time auditory feedback. Mobile HCI ’12, companion proceedings of the 14th international conference on human-computer interaction with mobile devices and services, San Francsico, CA, USA, September 21- 24,2012, December 2017, 5–10. New York, United States: Association for Computing Machinery. https://doi.org/https://doi.org/10.1145/2371664.2371668
- Han, H., Kim, H., Sun, W., Malaska, M., & Miller, B. (2020). Validation of wearable activity monitors for real-time cadence. Journal of Sports Sciences, 38(4), 383–389. https://doi.org/https://doi.org/10.1080/02640414.2019.1702281
- Hanley, B. (2015). Pacing profiles and pack running at the IAAF world half marathon championships. Journal of Sports Sciences, 33(11), 1189–1195. https://doi.org/https://doi.org/10.1080/02640414.2014.988742
- IAAF. (n.d.). IAAF Rules. IAAF Rules. Retrieved January 26, 2021, from https://www.worldathletics.org/about-iaaf/documents/book-of-rules
- Lauber, B., & Keller, M. (2014). Improving motor performance: Selected aspects of augmented feedback in exercise and health. European Journal of Sport Science, 14(1), 36–43. https://doi.org/https://doi.org/10.1080/17461391.2012.725104
- Lee, D.-C., Pate, R. R., Lavie, C. J., Sui, X., Church, T. S., & Blair, S. N. (2014). Leisure-time running reduces all-cause and cardiovascular mortality risk. Journal of the American College of Cardiology, 64(5), 472–481. https://doi.org/https://doi.org/10.1016/j.jacc.2014.04.058
- Mahendran, N., Kuys, S. S., Downie, E., Ng, P., & Brauer, S. G. (2016). Are accelerometers and GPS devices valid, reliable and feasible tools for measurement of community ambulation after stroke? Brain Impairment, 17(2), 151–161. https://doi.org/https://doi.org/10.1017/BrImp.2016.13
- Napier, C., Cochrane, C. K., Taunton, J. E., & Hunt, M. A. (2015). Gait modifications to change lower extremity gait biomechanics in runners: A systematic review. British Journal of Sports Medicine, 49(21), 1382–1388. https://doi.org/https://doi.org/10.1136/bjsports-2014-094393
- Nigg, B. M., De Boer, R. W., & Fisher, V. (1995). A kinematic comparison of overground and treadmill running. Medicine and Science in Sports and Exercise, 27(1), 98–105. https://doi.org/https://doi.org/10.1249/00005768-199501000-00018
- Pryor, J. L., Johnson, E. C., Yoder, H. A., & Looney, D. P. (2020). Keeping pace: A practitioner-focused review of pacing strategies in running. Strength and Conditioning Journal, 42(1), 67–75. https://doi.org/https://doi.org/10.1519/SSC.0000000000000505
- R Core Team. (2020). R: A language and environment for statistical computing. [Manual]. https://www.R-project.org/
- Schnohr, P., Marott, J. L., Lange, P., & Jensen, G. B. (2013). Longevity in male and female joggers: The Copenhagen city heart study. American Journal of Epidemiology, 177(7), 683–689. https://doi.org/https://doi.org/10.1093/aje/kws301
- Sheerin, K. R., Reid, D., Taylor, D., & Besier, T. F. (2020). The effectiveness of real-time haptic feedback gait retraining for reducing resultant tibial acceleration with runners. Physical Therapy in Sport, March, 43, 173–180. https://doi.org/https://doi.org/10.1016/j.ptsp.2020.03.001
- Sigrist, R., Rauter, G., Riener, R., & Wolf, P. (2013). Augmented visual, auditory, haptic, and multimodal feedback in motor learning: A review. Psychonomic Bulletin & Review, 20(1), 21–53. https://doi.org/https://doi.org/10.3758/s13423-012-0333-8
- Sinclair, J., Richards, J., Taylor, P. J., Edmundson, C. J., Brooks, D., & Hobbs, S. J. (2013). Three-dimensional kinematic comparison of treadmill and overground running. Sports Biomechanics, 12(3), 272–282. https://doi.org/https://doi.org/10.1080/14763141.2012.759614
- Strohrmann, C., Seiter, J., & Tröster, G. (2014). Feedback provision on running technique with a smartphone. Journal of Ubiquitous Systems & Pervasive Networks Volume, 5(1), 25–31. https://doi.org/https://doi.org/10.5383/JUSPN.05.01.004
- Tate, J. J., & Milner, C. E. (2017). Sound-intensity feedback during running reduces loading rates and impact peak. Journal of Orthopaedic and Sports Physical Therapy, 47(8), 565–569. https://doi.org/https://doi.org/10.2519/jospt.2017.7275
- Thompson, W. R., Gordon, N. F., & Pescatello, L. S. (2010). ACSM’s guidelines for exercise testing and prescription. Lippincott Williams & Wilkins.
- Uger, L., & Boucher, R. (1980). An indirect continuous running multistage field test: The universite de Montreal track test. Can. J. Appl. Sport. Sci, 1980 Jun,5(2), 77–84. https://pubmed.ncbi.nlm.nih.gov/7389053/
- van Gelder, L. M. A., Barnes, A., Wheat, J. S., & Heller, B. W. (2018). Characterizing the learning effect in response to biofeedback aimed at reducing tibial acceleration during running. Proceedings of ISEA 2018, 2(6):200. https://doi.org/https://doi.org/10.3390/proceedings2060200
- Vickers, A. J., & Vertosick, E. A. (2016). An empirical study of race times in recreational endurance runners. BMC Sports Science, Medicine and Rehabilitation, 8(1), 1. https://doi.org/https://doi.org/10.1186/s13102-016-0052-y
- Willy, R. W., Buchenic, L., Rogacki, K., Ackerman, J., Schmidt, A., & Willson, J. D. (2016). In-field gait retraining and mobile monitoring to address running biomechanics associated with tibial stress fracture: In-field gait retraining and monitoring. Scandinavian Journal of Medicine & Science in Sports, 26(2), 197–205. https://doi.org/https://doi.org/10.1111/sms.12413
- Wood, C. M., & Kipp, K. (2014). Use of audio biofeedback to reduce tibial impact accelerations during running. Journal of Biomechanics, 47(7), 1739–1741. https://doi.org/https://doi.org/10.1016/j.jbiomech.2014.03.008