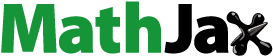
ABSTRACT
Soil is a substantive environmental medium that is subjected to various physiochemical challenges derived by natural, as well as human activities. The present review attempts to summarize the pollution status of soil from the catchments areas of Beas and Ravi Rivers in Punjab, India, as reported by different workers. Principal component analysis (PCA) showed that anthropogenic activities and lithogenic factors are the major sources of metals. The average values of heavy metals of Beas River for heavy metals, Cr, Cu, Co, and Cd, were lower than the values suggested by Awasthi, European Union, and Ewers. The average values of C (0.29%), P (0.05 mg/g), and N (0.13 mg/g) were found for the Beas River, whereas for Ravi River the average values recorded were C (0.32%), P (0.01 mg/g), and N (0.16 mg/g). The results of contamination factor (CF) indicate that soil of Beas River is less contaminated by the metals. The results of ecological risk index indicate that metals showed low ecological risk in the soils of Beas River.
Introduction
Soil is a complex and dynamic component of the ecosystem. It is being continuously polluted by the accumulation of various toxic substances, such as metals and various organic substances from the industrial or domestic areas (Li, Wu, Qian, & Zhou, Citation2016). These contaminates hampers the soil health which in turn modulates the efficiency of ecosystems (Burges, Alkorta, Epelde, & Garbisu, Citation2018). Soil plays significant role in exchange of energy and materials within the biotic and abiotic components. The proper management of soil requires good knowledge about soil characteristics that assists in sustaining or enhancing the level of soil quality and improves the degradation of soil, which is a worldwide problem (Thapa & Yila, Citation2012; Zhao, Mu, Wen, Wang, & Gao, Citation2013). The content of soil properties alters spatially because of integrated effect of physical, chemical and biological mechanisms working in soil in addition to the impact of human and natural activities (Goovaerts, Citation1998). A pollutant that enters the soil is likely to induce critical destruction to the plants, animals and most importantly humans through interconnected food chain (Micó, Recatalá, Peris, & Sánchez, Citation2006). Many industries deposit their unwanted waste materials in the soil which may generate ecological imbalance. Heavy metals are the main sources responsible for having adverse effect on the ecological quality of environment (Attia, Khadra, Nawwar, & Radwan, Citation2012; Bodin et al., Citation2013; Fernandes, Nayak, & Ilangovan, Citation2012; Jara-Marini, Soto-Jiménez, & Páez-Osuna, Citation2008). Heavy metals enter into the environment from various sources, such as natural weathering and human activities (Badr, El-Fiky, Mostafa, & Al-Mur, Citation2009; Madkour, Citation2013; Venkatramanan, Ramkumar, Anithamary, & Vasudevan, Citation2014). The soil enriched with heavy metals has significant negative impact on the plants and animals, as well as humans that eat these plants (Singh & Kumar, Citation2006; Tutic et al., Citation2015).
All the physiochemical and biochemical properties are related with the functioning of the soil (Trasar-Cepeda, Leirós, & Gil-Sotres, Citation2008). Accumulation and distribution of heavy metals are affected by many factors, i.e., structure and composition of sediment, size of grain and hydrodynamic conditions. Due to these factors, the content of heavy metals in sediment varies spatially and temporally (Liu et al., Citation2011). Sungur, Soylak, Yilmaz, Yilmaz, and Ozcan (Citation2015) studied the content of heavy metals in agricultural soil from Turkey. Spatial variability in soils may takes place naturally as result of complex interactions between topography, climate and geology. However, the spatial variability in soil properties which affected the soil susceptibility to erosion is highly associated with human activities mostly in the cultivated lands (Li, Qian, Howard, Wu, & Lyu, Citation2014; Wang & Shao, Citation2013). Thus, data on spatial variability and the interactions between soil characteristics is necessary for understanding the ecosystem processes and organizing sustainable soil management practices for specific land uses (Pérez-Rodríguez, Marques, & Bienes, Citation2007; Keesstra, Nunes, & Novara et al., Citation2018; Ziadat & Taimeh, Citation2013). Various multivariate techniques, such as principal component analysis (PCA), cluster analysis (CA), factor analysis, multiple linear regression analysis, and artificial neural networks have been used to assess the heavy metal origin in the soil (Kumar et al. Citation2016b; Masoodi, Majdzadeh Tabatabai, Noorzad, & Samadi, Citation2017; Patel et al., Citation2018; Suárez et al., Citation2016).
Zhang, Wei, Sun, Wadood, and Guo (Citation2018) studied soils of Zhuzhou city by employing multivariate statistical analysis and inferred that industrial and agricultural activities were responsible for contamination the soil ecosystem. The heavy metal content in soils mainly depends on parent material and various human activities (Lu et al., Citation2012). In India, various activities, such as fast industrialization, urbanization, agronomic practices, traffic activities, untreated waste water, etc. used for irrigation, were responsible for degrading the environmental quality that ultimately declines the soil quality (Kumar, Sharma, Bhardwaj, & Thukral, Citation2016a, Citationb, Citationc; Kumar, Sharma, Minakshi, & Thukral, Citation2018). These human activities in association with natural sources of the soil make complicated to assess the sources of heavy metals. Behera, Mathur, Shukla, Suresh, and Prakash (Citation2018) in their work on soils of west Godavari, Andhra Pradesh and observed that results of this work may be helpful in site specific management of the soil nutrients.
The multivariate techniques, such as CA and PCA will only provide the source apportionment of soil properties and heavy metals. These techniques will not give any idea about the pollution level of soil ecosystem. Further, in order to evaluate the degree of pollution in soil samples, the contamination factor (CF), and potential ecological risk index (RI) was applied to heavy metals. Various workers used CF and RI to assess the level of pollution in the soil and sediment samples (Ahmed et al., Citation2016; Duodu, Goonetilleke, & Ayoko, Citation2016; Kamani et al., Citation2018; Kumar et al., Citation2018; Moghtaderi, Mahmoudi, Shakeri, & Masihabadi, Citation2018; Tian, Huang, Xing, & Hu, Citation2017). RI is based upon CF and gives important information about the level of pollution and ecological risks posed by the metals in the soils.
Agriculture is the major resource for the people of Punjab. They applied extensive amount of pesticides, fertilizers, weedicides, etc. After application of these agrochemicals when they irrigated the land, the surface run from the soil will goes into the river and contaminated the soil quality (Nazzal, Howari, Jafri, Naeem, & Ghrefat, Citation2016; Tian et al., Citation2017). The National Highways around Beas and Ravi Rivers add pollution to the surrounding soils by traffic activities, such as tyre abrasions, vehicular emissions, etc. (Zhao, Ball, & Hazelton, Citation2018). The harmful impact of heavy metals in soils is represented not by measuring its content only but further determination about the contamination level and ecological risk evaluation is needed. Such reports are inadequate in Punjab and needs more surveillance.
The present review designed (i) to compile the soil data from catchment areas of Beas and Ravi Rivers, (ii) comparison of heavy metal data from Beas River with standards of Awasthi, European Union and Ewers, (iii) to find the possible sources of their origin by using CA and PCA, and (iv) computation of CF and ecological risk assessment for heavy metals of Beas River.
Study area and data collection
The Beas River originates from the Central Himalayas in the central Himachal Pradesh, India at latitude of 32º2ʹ N and longitude of 77°05ʹ E by flowing a distance of 470 km it merges with the river Sutlej in Harike Wetland. The Ravi River originates from the Kangra District of Himachal Pradesh, India at latitude of 30°35ʹ N and longitude of 71°49′ E. Both the Rivers are the tributaries of the Indus river system. The data of the soil properties for catchment areas of Beas and Ravi Rivers were analyzed from Moza (Citation2014), Kumar et al. (Kumar et al., Citation2016a, Citationb), and Moza and Mishra (Citation2007). There is no report of heavy metal analysis from catchment areas of river Ravi till date. The data of soil properties are given as supplementary material.
Measurement of contamination level and ecological risk assessment
The CF and potential ecological risk index (RI) was computed to find the level of contamination and ecological risk assessment of heavy metals in the soils. CF was computed by following the equation of Hakanson (Citation1980)
The ecological RI tells us about the sensitivity of various biological communities in the large contaminated areas (Hakanson 1980). RI takes into account the CF of metals, potential ecological risk factor (Er) and toxicological response factor (Tr) of metals taken from Duodu et al. (Citation2016). It was computed by the equation
where Eir is the potential ecological risk factor of each metal. Tri is the toxicological response factor of each metal (Duodu et al., Citation2016). CFi is the contamination factor of individual metal.
Statistical analysis
The results were statistically analyzed by employing Pearson’s correlation matrix, CA, and PCA. CA and PCA were used to find out the possible sources of soil parameters and heavy metals in the soils from the catchment areas of Beas and Ravi Rivers (Kumar et al., Citation2018).
Results and discussion
Descriptive statistics of soil properties from catchment areas of Ravi and Beas rivers
shows the mean and ranges of physiochemical properties of soil from Beas and Ravi rivers. Among the textural characteristics, the sand content is found highest from the catchment areas of both Beas and Ravi rivers. The mean values of conductivity, C and N were found maximum from the catchment areas of Ravi River. The minimum values of C, P, and N were 0.13 (%), 0.013 (mg/g), and 0.001 (mg/g), whereas maximum values of C, P, and N were 0.69 (%), 0.20(mg/g), and 1.07 (mg/g) found for the Ravi River, respectively. The minimum values of C, P, and N were 0.14 (%), 0.006 (mg/g), and 0.11 (mg/g), whereas maximum values of C, P, and N were 0.52 (%), 0.07(mg/g), and 0.44 (mg/g) found for the Beas River, respectively. shows the mean and ranges of heavy metal contents from catchment areas of river Beas. The trend of metals found, i.e., Na> K> Mn> Ca> Ni> Mg> Zn> Cr> Cu> Co> Cd. In Beas river, maximum values of Zn, Mn, Ni, Cr, Cu, Co, and Cd were 0.04, 1.65, 0.80, 0.03, 0.03, 0.01, and 0.0007 (mg/g) found, respectively.
Table 1. Descriptive statistics of soil parameters from catchment areas of Beas and Ravi rivers.
Table 2. Descriptive statistics of heavy metals of soil from catchment areas of river Beas and their permissible limits.
Comparison of soil properties of Beas and Ravi rivers with national and International Studies
The average values of soil properties from catchment areas of Beas and Ravi rivers were compared with other studies done by researchers at national and international level. The silt (84.12%), clay (14.12%), pH (9.47), P (0.336 mg/g), and N (0.317%) contents from Coastal Wetlands, Laizhou Bay, Bohai Sea (Cao et al., Citation2015) were found higher than the average values of Beas and Ravi Rivers. The C (1.91%) and clay (19.2%) from Guadalquivir River marshes (Domínguez et al., Citation2016) were found higher than the Beas and Ravi rivers. The values reported by Awashthi (Citation2000), European Union (Citation2002), and Ewers (Citation1991) for Cr, Cu, Co, and Cd were found higher than the average values from Beas River. The results of Briki, Ji, Li, Ding, and Gao (Citation2015) in their work on agricultural soils of Hezhang, China for Cd (1.62 mg/kg), Co (38.95 mg/kg), Cr (157.50 mg/kg), Cu (79.75 mg/kg), and Ni (69.2 mg/kg) were found higher than the results of present study. Cai et al. (Citation2015) also found higher values for Cd (0.6 mg/kg), Co (16.7 mg/kg), Cr (78.8 mg/kg), and Ni (33.4 mg/kg) as compared to the results of present study. Similarly, results of heavy metals for present study were also found lesser than Romic and Romic (Citation2003) for Zagreb, Croatia, Micó et al. (Citation2006) for European Mediterranean, Spain, Ahmad & Goni (Citation2010) for Dhaka, Bangladesh, Facchinelli, Sacchi, and Mallen (Citation2001) for Piedmont, Italy, and Hani and Pazira (Citation2011) for Southern Tehran, Iran.
Pearson’s correlation analysis of soil properties
Pearson’s correlation was applied to different physiochemical properties of soil from Beas river bed (). Negative correlation existed between silt and sand. Correlation of P found with clay, conductivity and C. shows the Pearson’s correlation of heavy metals from Beas river bed. Positive correlation of Cu found with Fe, K, Mn, and Cr. Co showed positive correlation with Cu and Fe. Pearson’s correlation was applied to different physiochemical properties of soil from Ravi river bed (). Sand shows correlation with silt and clay. Correlation was also existed between pH and conductivity. Our results find support from Aminiyan et al. (Citation2018), in their work on soils of Rafsanjan, Iran and found that Cr showed positive correlation with Zn and Cu. The results of present study are in confirmation with Liang et al. (Citation2017) in their study on surface soils of China and observed correlation of Fe with Cr and Cu. They also observed correlation of Mn with Cu, which is in confirmation with our results.
Table 3a. Pearson’s correlation of physiochemical properties from the catchment areas of river Beas.
Table 3b. Pearson’s correlation among heavy metals from the catchment areas of river Beas.
Table 3c. Pearson’s correlation of physiochemical properties from the catchment areas of river Ravi.
Cluster analysis
CA was applied to different physiochemical properties of soil from Ravi river bed by using Ward’s method and Euclidean distance as a measure of similarity (Kumar et al., Citation2017a, Citation2017b) (). Shahpur and Madhopur are included in the same cluster and this may be due to the reason that values of silt, conductivity and N are almost similar in these sites. Kathlour formed a separate cluster. This may be due to the fact that high values of conductivity and N were found for Kathlour as compared to other sites. CA was also applied to physiochemical properties of soil from Beas river bed (). Talwara and Harike are included in the same cluster. Beas bridge, Mukerian, and Goindwal Sahib are also included in the same cluster. This may be due to that clay, C, P, and N values of these sites are almost similar to each other.
Principal component analysis
PCA was applied to different soil properties from catchment areas of Beas and Ravi rivers. It gives information about the sources of metals in the catchment areas of Beas and Ravi rivers. First four components of PCA explained 86.50% of the total variance in case of metals from Beas River (). PC1 had maximum loadings on Fe, Zn, Cr, Cu, and Co and accounted for 31.74% of the total variance. This PC indicates the lithogenic factors and parent rock materials are the major sources of these metals. Ma and Gui (Citation2017) while working on Bengbu, Eastern China and Li et al. (Citation2015) in their studies on Yangtze River Delta reported loadings of Cu and Zn on PC1 and reported that human activities are responsible for these metals. PC2 explained 20.56% of the total variance and had maximum loadings on Mg, Ni, and Cd. Cd contribution to this PC mainly due to agriculture activities, i.e., pesticides, fertilizers, etc. (Xia, Meng, & Yin et al., Citation2011; Zhang & Shan, Citation2008). Ca and Mn had maximum loadings on PC3 and this PC accounted for 17.62% of the total variance. PC4 had maximum loadings on K and it explained 16.58% of the total variance. First three components of PCA explained 69.90% of the total variance of soil parameters from catchment areas of Beas River (). PC1 had maximum loadings on sand and silt, and accounted for 27.53% of the total variance. This PC represents the textural properties of soil. PC2 explained 23.98% of the total variance and had maximum loadings on clay, C and P. PC2 represent organic load and fertility of soil. Conductivity, N and pH contribute to PC4 and explained 18.39% of the total variance.
Table 4a. Principal component analysis of metals from catchment areas of river Beas.
Table 4b. Principal component analysis and loadings of soil parameters from catchment areas of river Beas.
First three principal components explained 75.58% of the total variance in case of soil properties from Ravi River (). Sand, silt, clay, and N have maximum loadings on PC1 and accounted for 32.91% of the total variance. PC1 indicates the textural characteristics of soil. The contribution of N to this PC is attributed to fertility of the soil (Kumar et al. 2016). pH and conductivity contributes to PC2 and accounted for 23.94% of the total variance. PC3 explained 18.73% of the total variance and had maximum loadings on C and P. PC3 indicates the organic load and fertility of the soil.
Table 4c. Principal component analysis and loadings of soil parameters from catchment areas of river Ravi.
Indexing approach
CF and ecological risk assessment (RI) were computed for metals of Beas River to find the level of contamination and ecological risk assessment of each metal in the Beas River (). From the results of CF, it was observed that the CF of each metal is below 1, which indicates low contamination of metals in the study area. The results of Er values for each metal showed that Cd followed by Ni showed maximum ecological risk in the Beas River. The Er values of each metal were found below than 40, which indicate low ecological risk in the Beas River. Kamani et al. (Citation2017) while working on street dusts of Iran reported high Er values for Cd. Nouri and Haddioui (Citation2016) while working on ammar iron mine in Morocco also reported that CF of Cd, Cr, Cu, and Zn is less than one. The results of RI in present study also supported by Zhang, Wu, Zhang, and Cao (Citation2017) while working on southern Loess Plateau, China. They also reported low potential ecological risk of metals in their study. Mamut, Eziz, Mohammad, Anayit, and Rixit (Citation2017) in their studies on Xinjiang, Northwest China also reported that Cd is the main contaminant that contributes to RI. Similarly, Ma and Gui (Citation2017) in their studies on Bengbu, Anhui province reported that Cd is the main contaminant that contributes to potential ecological risk of soil in Bengbu. Wang et al. (Citation2017) while working on urban soils from industrial city, Suzhou reported low ecological risk of metals. Bhatti et al. (Citation2017, Citation2018) while working on agricultural soils of Punjab reported that Cd is the major contaminant that provides potential ecological risk to the soils of Punjab which are in confirmation with the results of present study.
Conclusions
The present review attempts to create a database of the physiochemical properties of the soils from the catchment areas of Beas and Ravi Rivers. Soil ecosystem receives various contaminates including Cd, Cu, Zn, Ni, Cu, Fe, etc. from natural and anthropogenic activities, such as release of sewage effluents and vehicular emissions, and their average values were found below the permissible limits of soil for Awasthi, European Union, and Ewers. The mean values of sand, conductivity, C and N were found maximum from the catchment areas of Ravi River, whereas silt, clay, pH and P contents were found maximum from the catchment areas of Beas River. PCA indicates that lithogenic and anthropogenic sources are responsible for origin of metals. CF and RI indicate that soils of Beas River were less polluted by the metals.
Supplemental Material
Download MS Word (70.3 KB)Disclosure statement
No potential conflict of interest was reported by the authors.
Supplementary material
Supplemental data can be accessed here.
References
- Ahmad, J. U., & Goni, M. A. (2010). Heavy metal contamination in water, soil, and vegetables of the industrial areas in Dhaka, Bangladesh. Environmental Monitoring and Assessment, 166(1–4), 347–357.
- Ahmed, F., Fakhruddin, A. N. M., Imam, M. T., Khan, N., Khan, T. A., Rahman, M. M., & Abdullah, A. T. M. (2016). Spatial distribution and source identification of heavy metal pollution in roadside surface soil: A study of Dhaka Aricha highway, Bangladesh. Ecological Processes, 5(1), 2.
- Aminiyan, M. M., Baalousha, M., Mousavi, R., Aminiyan, F. M., Hosseini, H., & Heydariyan, A. (2018). The ecological risk, source identification, and pollution assessment of heavy metals in road dust: A case study in Rafsanjan, SE Iran. Environmental Science and Pollution Research, 25(14), 13382–13395.
- Attia, O. E. A., Khadra, A. M. A., Nawwar, A. H., & Radwan, G. E. (2012). Impacts of human activities on the sedimentological and geochemical characteristics of Mabahiss Bay, North Hurghada, Red Sea, Egypt. Arabian Journal of Geosciences, 5(3), 481–499.
- Awashthi, S. K. (Ed.). (2000). Prevention of food adulteration Act No. 37 of 1954. Central and state rules as amended for 1999. New Delhi: Ashoka Law House.
- Badr, N. B., El-Fiky, A. A., Mostafa, A. R., & Al-Mur, B. A. (2009). Metal pollution records in core sediments of some Red Sea coastal areas, Kingdom of Saudi Arabia. Environmental Monitoring and Assessment, 155(1), 509–526.
- Behera, S. K., Mathur, R. K., Shukla, A. K., Suresh, K., & Prakash, C. (2018). Spatial variability of soil properties and delineation of soil management zones of oil palm plantations grown in a hot and humid tropical region of southern India. Catena, 165, 251–259.
- Bhatti, S. S., Bhat, S. A., Kumar, V., Kaur, M., Minakshi, S., & Nagpal, A. K. (2017). Ecological risk assessment of metals in roadside agricultural soils: A modified approach. Human and Ecological Risk Assessment: an International Journal, 24(1), 186–201.
- Bhatti, S. S., Kumar, V., Kumar, A., Gouzos, J., Kirby, J., Singh, J., & Nagpal, A. K. (2018). Potential ecological risks of metal (loid) s in riverine floodplain soils. Ecotoxicology and Environmental Safety, 164, 722–731.
- Bodin, N., Gom-Kâ, R., Kâ, S., Thiaw, O. T., De Morais, L. T., Le Loc’h, F., & Chiffoleau, J. F. (2013). Assessment of trace metal contamination in mangrove ecosystems from Senegal, West Africa. Chemosphere, 90(2), 150–157.
- Briki, M., Ji, H., Li, C., Ding, H., & Gao, Y. (2015). Characterization, distribution, and risk assessment of heavy metals in agricultural soil and products around mining and smelting areas of Hezhang, China. Environmental Monitoring and Assessment, 187(12), 767.
- Burges, A., Alkorta, I., Epelde, L., & Garbisu, C. (2018). From phytoremediation of soil contaminants to phytomanagement of ecosystem services in metal contaminated sites. International Journal of Phytoremediation, 20, 384–397.
- Cai, L., Xu, Z., Bao, P., He, M., Dou, L., Chen, L., & Zhu, Y. G. (2015). Multivariate and geostatistical analyses of the spatial distribution and source of arsenic and heavy metals in the agricultural soils in Shunde, Southeast China. Journal of Geochemical Exploration, 148, 189–195.
- Cao, L., Song, J., Li, X., Yuan, H., Li, N., Duan, L., & Wang, Q. (2015). Geochemical characteristics of soil C, N, P, and their stoichiometrical significance in the coastal wetlands of Laizhou Bay, Bohai Sea. CLEAN–Soil, Air, Water, 43(2), 260–270.
- Domínguez, M. T., Alegre, J. M., Madejón, P., Madejón, E., Burgos, P., Cabrera, F., & Murillo, J. M. (2016). River banks and channels as hotspots of soil pollution after large-scale remediation of a river basin. Geoderma, 261, 133–140.
- Duodu, G. O., Goonetilleke, A., & Ayoko, G. A. (2016). Comparison of pollution indices for the assessment of heavy metal in Brisbane River sediment. Environmental Pollution, 219, 1077–1091.
- European Union (2002) heavy metals in wastes, European commission on environmet. Retreived from <http://ec.europa.eu/environment/waste/studies/pdf/heavymetalsreport.pdf. .
- Ewers, U. (1991). Standards, guidelines and legislative regulations concerning metals and their compounds. In E. Merian (Ed.), Metals and their compounds in environment: Occurrence, analysis and biological relevance (pp. 458–468). Weinheim: VCH.
- Facchinelli, A., Sacchi, E., & Mallen, L. (2001). Multivariate statistical and GIS-based approach to identify heavy metal sources in soils. Environmental Pollution, 114(3), 313–324.
- Fernandes, L., Nayak, G. N., & Ilangovan, D. (2012). Geochemical assessment of metal concentrations in mangrove sediments along Mumbai coast, India. World Academy of Science, Engineering and Technology, 61, 258–263.
- Goovaerts, P. (1998). Geostatistical tools for characterizing the spatial variability of microbiological and physico-chemical soil properties. Biology and Fertility of Soils, 27(4), 315–334.
- Hakanson, L. (1980). An Ecological Risk Index for Aquatic Pollution Control. Water Research, 14(8), 975-1001. doi:10.1016/0043-1354(80)90143-8
- Hani, A., & Pazira, E. (2011). Heavy metals assessment and identification of their sources in agricultural soils of Southern Tehran, Iran. Environmental Monitoring and Assessment, 176(1–4), 677–691.
- Jara-Marini, M. E., Soto-Jiménez, M. F., & Páez-Osuna, F. (2008). Bulk and bioavailable heavy metals (Cd, Cu, Pb, and Zn) in surface sediments from Mazatlán Harbor (SE Gulf of California). Bulletin of Environmental Contamination and Toxicology, 80(2), 150–153.
- Kamani, H., Mirzaei, N., Ghaderpoori, M., Bazrafshan, E., Rezaei, S., & Mahvi, A. H. (2017). Concentration and ecological risk of heavy metal in street dusts of Eslamshahr, Iran. Human and Ecological Risk Assessment: an International Journal, 1–10. doi:10.1080/10807039.2017.1403282
- Kamani, H., Mirzaei, N., Ghaderpoori, M., Bazrafshan, E., Rezaei, S., & Mahvi, A. H. (2018). Concentration and ecological risk of heavy metal in street dusts of Eslamshahr, Iran. Human and Ecological Risk Assessment: an International Journal, 24, 1–10.
- Keesstra, S., Nunes, J., Novara, A.…,Cerdà, A. (2018). The superior effect of nature based solutions in land management for enhancing ecosystem services. The Science of the Total Environment, 610, 997–1009.
- Kumar, V., Sharma, A., Bhardwaj, R., & Thukral, A. K. (2016a). Monitoring and characterization of soils from River Bed of Beas, India, using Multivariate and remote sensing techniques. British Journal of Applied Science and Technology, 12(2), 1–12.
- Kumar, V., Sharma, A., Chawla, A., Bhardwaj, R., & Thukral, A. K. (2016c). Water quality assessment of river Beas, India, using multivariate and remote sensing techniques. Environmental Monitoring and Assessment, 188(3), 137.
- Kumar, V., Sharma, A., Dhunna, G., Chawla, A., Bhardwaj, R., & Thukral, A. K. (2017a). A tabulated review on distribution of heavy metals in various plants. Environmental Science and Pollution Research, 24, 2210–2260.
- Kumar, V., Sharma, A., Kaur, R., Thukral, A. K., Bhardwaj, R., & Ahmad, P. (2017b). Differential distribution of amino acids in plants. Amino Acids, 1–49. doi:10.1007/s00726-017-2401-x
- Kumar, V., Sharma, A., Minakshi, B., . R., & Thukral, A. K. (2018). Temporal distribution, source apportionment, and pollution assessment of metals in the sediments of Beas river, India. Human and Ecological Risk Assessment: an International Journal, 1–20.
- Kumar, V., Sharma, A., & Thukrala, A. K. (2016b). Assessment of soil enzyme activities based on soil samples from the Beas river bed, India using multivariate techniques. Malaysian Journal of Soil Science, 20, 135–145.
- Li, J., Pu, L., Liao, Q., Zhu, M., Dai, X., Xu, Y., & Jin, Y. (2015). How anthropogenic activities affect soil heavy metal concentration on a broad scale: A geochemistry survey in Yangtze River Delta, Eastern China. Environmental Earth Sciences, 73(4), 1823–1835.
- Li, P., Qian, H., Howard, K. W. F., Wu, J., & Lyu, X. (2014). Anthropogenic pollution and variability of manganese in alluvial sediments of the Yellow River, Ningxia, northwest China. Environmental Monitoring and Assessment, 186(3), 1385–1398.
- Li, P., Wu, J., Qian, H., & Zhou, W. (2016). Distribution, enrichment and sources of trace metals in the topsoil in the vicinity of a steel wire plant along the Silk Road economic belt, northwest China. Environment Earth Sciences, 75(10), 909.
- Liang, J., Feng, C., Zeng, G., Gao, X., Zhong, M., Li, X., … Fang, Y. (2017). Spatial distribution and source identification of heavy metals in surface soils in a typical coal mine city, Lianyuan, China. Environmental Pollution, 225, 681–690.
- Liu, S., Shi, X., Liu, Y., Zhu, Z., Yang, G., Zhu, A., & Gao, J. (2011). Concentration distribution and assessment of heavy metals in sediments of mud area from inner continental shelf of the East China Sea. Environmental Earth Sciences, 64(2), 567–579.
- Lu, A., Wang, J., Qin, X., Wang, K., Han, P., & Zhang, S. (2012). Multivariate and geostatistical analyses of the spatial distribution and origin of heavy metals in the agricultural soils in Shunyi, Beijing, China. Science of the Total Environment, 425, 66–74.
- Ma, L., & Gui, H. (2017). Anthropogenic impacts on heavy metal concentrations in surface soils from the typical polluted area of Bengbu, Anhui province, Eastern China. Human and Ecological Risk Assessment: an International Journal, 23(7), 1763–1774.
- Madkour, H. A. (2013). Impacts of human activities and natural inputs on heavy metal contents of many coral reef environments along the Egyptian Red Sea coast. Arabian Journal of Geosciences, 6(6), 1739–1752.
- Mamut, A., Eziz, M., Mohammad, A., Anayit, M., & Rixit, A. (2017). Contamination and risk assessment of heavy metals in farmland soils of Baghrash County, Xinjiang, Northwest China. Sciences in Cold and Arid Regions, 9(5), 467–475.
- Masoodi, A., Majdzadeh Tabatabai, M. R., Noorzad, A., & Samadi, A. (2017). Effects of soil physico-chemical properties on stream bank erosion induced by seepage in northeastern Iran. Hydrological Sciences Journal, 62(16), 2597–2613.
- Micó, C., Recatalá, L., Peris, M., & Sánchez, J. (2006). Assessing heavy metal sources in agricultural soils of an European Mediterranean area by multivariate analysis. Chemosphere, 65(5), 863–872.
- Moghtaderi, T., Mahmoudi, S., Shakeri, A., & Masihabadi, M. H. (2018). Heavy metals contamination and human health risk assessment in soils of an industrial area, Bandar Abbas–South Central Iran. Human and Ecological Risk Assessment: an International Journal, 1–16. doi:10.1080/10807039.2017.1405723
- Moza, U. (2014). River Ravi ecology and fishery. New Delhi: Indian Council of Agricultural Research.
- Moza, U., & Mishra, D. N. (2007). River Beas ecology and fishery. India: Central Inland Fisheries Research Institute (Indian Council of Agricultural Research) West Bengal.
- Nazzal, Y., Howari, F. M., Jafri, M. K., Naeem, M., & Ghrefat, H. (2016). Risk assessment through evaluation of potentially toxic metals in the surface soils of the Qassim area. Central Saudi Arabia Ital Journal Geosci, 135(2), 210–216.
- Nouri, M., & Haddioui, A. E. M. (2016). Assessment of metals contamination and ecological risk in Ait Ammar abandoned iron mine soil, Morocco. Ekológia (Bratislava), 35(1), 32–49.
- Patel, P., Raju, N. J., Reddy, B. S. R., Suresh, U., Sankar, D. B., & Reddy, T. V. K. (2018). Heavy metal contamination in river water and sediments of the Swarnamukhi River Basin, India: Risk assessment and environmental implications. Environmental Geochemistry and Health, 40(2), 609–623.
- Pérez-Rodríguez, R., Marques, M. J., & Bienes, R. (2007). Spatial variability of the soil erodibility parameters and their relation with the soil map at subgroup level. Science Of The Total Environment, 378(1–2), 166-173. doi:10.1016/j.scitotenv.2007.01.044
- Romic, M., & Romic, D. (2003). Heavy metals distribution in agricultural topsoils in urban area. Environmental Geology, 43(7), 795–805.
- Singh, S., & Kumar, M. (2006). Heavy metal load of soil, water and vegetables in peri-urban Delhi. Environmental Monitoring and Assessment, 120(1), 79–91.
- Suárez, P. A., Vega, M., Pardo, R., Orfeo, O., Cuesta, J. L. G., & Ronco, A. (2016). Hydrochemical and sedimentological dynamics in a subtropical plain river: Assessment by multivariate statistical analysis. Environmental Earth Sciences, 75(12), 1004.
- Sungur, A., Soylak, M., Yilmaz, E., Yilmaz, S., & Ozcan, H. (2015). Characterization of heavy metal fractions in agricultural soils by sequential extraction procedure: The relationship between soil properties and heavy metal fractions. Soil and Sediment Contamination: an International Journal, 24(1), 1–15.
- Thapa, G. B., & Yila, O. M. (2012). Farmers’ land management practices and status of agricultural land in the Jos Plateau, Nigeria. Land Degradation & Development, 23(3), 263–277.
- Tian, K., Huang, B., Xing, Z., & Hu, W. (2017). Geochemical baseline establishment and ecological risk evaluation of heavy metals in greenhouse soils from Dongtai, China. Ecological Indicators, 72, 510–520.
- Trasar-Cepeda, C., Leirós, M. C., & Gil-Sotres, F. (2008). Hydrolytic enzyme activities in agricultural and forest soils. Some implications for their use as indicators of soil quality. Soil Biology and Biochemistry, 40(9), 2146–2155.
- Tutic, A., Novakovic, S., Lutovac, M., Biocanin, R., Ketin, S., & Omerovic, N. (2015). The heavy metals in agrosystems and impact on health and quality of life. Open Access Macedonian Journal of Medical Sciences, 3(2), 345–355.
- Venkatramanan, S., Ramkumar, T., Anithamary, I., & Vasudevan, S. (2014). Heavy metal distribution in surface sediments of the Tirumalairajan river estuary and the surrounding coastal area, east coast of India. Arabian Journal of Geosciences, 7(1), 123–130.
- Wang, G., Liu, H. Q., Gong, Y., Wei, Y., Miao, A. J., Yang, L. Y., & Zhong, H. (2017). Risk assessment of metals in urban soils from a typical industrial city, Suzhou, Eastern China. International Journal of Environmental Research and Public Health, 14(9), 1025.
- Wang, Y. Q., & Shao, M. A. (2013). Spatial variability of soil physical properties in a region of the Loess Plateau of PR China subject to wind and water erosion. Land Degradation and Development, 24(3), 296–304.
- Xia, P., Meng, X. W., Yin, P., Cao, Z., & Wang, X. (2011). Eighty-year sedimentary record of heavy metal inputs in the intertidal sediments from the Nanliu River estuary, Beibu Gulf of South China Sea. Environmental Pollution, 159, 92–99.
- Zhang, H., & Shan, B. Q. (2008). Historical records of heavy metal accumulation in sediments and the relationship with agricultural intensification in the Yangtze-Huaihe region, China. The Science of the Total Environment, 399, 113–120.
- Zhang, X., Wei, S., Sun, Q., Wadood, S. A., & Guo, B. (2018). Source identification and spatial distribution of arsenic and heavy metals in agricultural soil around Hunan industrial estate by positive matrix factorization model, principle components analysis and geo statistical analysis. Ecotoxicology and Environmental Safety, 159, 354–362.
- Zhang, Y., Wu, F., Zhang, X., & Cao, N. (2017). Pollution characteristics and ecological risk assessment of heavy metals in three land-use types on the southern Loess Plateau, China. Environmental Monitoring and Assessment, 189(9), 470.
- Zhao, G., Mu, X., Wen, Z., Wang, F., & Gao, P. (2013). Soil erosion, conservation, and eco‐environment changes in the Loess Plateau of China. Land Degradation & Development, 24(5), 499–510.
- Zhao, Z., Ball, J., & Hazelton, P. (2018). Application of statistical inference for analysis of heavy metal variability in roadside soil. Water, Air, and Soil Pollution, 229(1), 23.
- Ziadat, F. M., & Taimeh, A. Y. (2013). Effect of rainfall intensity, slope, land use and antecedent soil moisture on soil erosion in an arid environment. Land Degradation and Development, 24(6), 582–590.