ABSTRACT
Predictive models of species distribution can be beneficial for identifying the most important and influential environmental factors in species distribution and understanding the current and future habitat suitability of species. In this study, we used species distribution modeling to find out the potential distribution and habitat suitability of two spatially separated populations of Spalerosophis diadema and show the most important environmental factors that affect their habitat separation. The findings of SDM analyses demonstrated that the most important factors for the two populations are annual mean temperature, precipitation of coldest quarter, NDVI in May, and elevation, as these factors contributing about 80–85% of the environmental factors to the whole model. Two populations react similarly to these environmental predictors and there is no significant difference between the important, and influential environmental factors of their habitats. Although our results show some overlap of the potential distributions of populations among Mesopotamian plain and Afghanistan, there is no confirmed report or voucher specimen proving the overlap distribution in these regions. Our results support the hypothesis that the Zagros Mountains acted as a barrier to the east–west fragmentation of these two populations.
Introduction
Environmental factors are among the most important factors influencing the distribution pattern of terrestrial organisms, analyzing the ecological parameters affecting species distributions can help to understand the underlying ecological processes (Graham et al., Citation2004). The interaction of the different factors with species characteristics (centroid and tolerance in environmental space) highlighted the importance of the so-called “idiosyncrasy in species responses” to model efficiency. However, differences in prevalence may represent a better explanation (De Marco et al., Citation2018). Predictive modeling of species geographic distribution (also called ecological niche models, environmental niche models, habitat suitability, or bioclimate envelope models) based on environmental conditions of known occurrence sites is an important technique with applications in conservation and protected area planning, biogeography, phylogeny, ecology, evolution, epidemiology, wildlife management, and other related fields (Phillips et al., Citation2006).
Predictive potential distribution modeling is of increasing value in modern herpetological studies in many fundamental and applied aspects: biogeography, ecology (contribution to ecological niche theory), biodiversity conservation, monitoring and forecast of invasion of alien species as well as increasingly fragmented ranges of threatened and endangered species (Bernardes et al., Citation2013; Rödder & Lötters, Citation2010). Species distribution modeling tools are becoming increasingly popular in ecology and widely used in many ecological applications (Elith et al., Citation2006; Peterson, Citation2006). Species distribution models (SDMs) use environmental data for sites of occurrence (presence) of a species for describing ecological patterns and estimating the ecological niche or the potential distribution of species across space and time (Elith & Leathwick, Citation2009). Several methods of species distribution modeling have been developed since the 1980s. Among the different SDM algorithms, maximum entropy (Maxent; Phillips et al., Citation2006) is extensively used by scientists for species geographical distribution and environmental niche modeling (Elith & Leathwick, Citation2009). In recent comparisons, MaxEnt ranked as the best-performing model using presence-only data and showed better performance over more traditional algorithms used to analyze presence-absence data (Elith et al., Citation2006). Maxent has achieved a robust performance showing good accuracy with low sample sizes and an excellent predictive ability (Hernandez et al., Citation2006; Wisz et al., Citation2008). Practical testing of species distribution modeling or niche modeling is of increasing value in modern herpetological studies in many fundamental and applied aspects and can provide suitable information for many rare and poorly known taxa (Ananjeva et al., Citation2015).
During evolution, natural barriers have played an important role in speciation. Throughout the last several million years, all regions have been exposed to complex geomorphological and topographical changes, which resulted in the formation of substantial mountainous ranges a well-known barrier for small vertebrates (Hewitt, Citation2011; Tarkhnishvili, Citation2014). The Zagros Mountains are located on the western part of the Iranian Plateau and extend about 1200 km from the Anatolian Mountain belt in eastern turkey to the Strait of Hormoz in southeast Iran and connect to the subduction zone of Makran (Hatzfeld et al., Citation2010). The convergence between Eurasian and Arabian Plates made the Zagros Mountains uplift in the late Miocene or early Pliocene (Agard et al., Citation2005). It is claimed that these mountain ridges and the deep valleys are among the significant speciation centers in the Irano-Anatolian area (Ahmadzadeh et al., Citation2013; Goudarzi et al., Citation2019; Malekoutian et al., Citation2020). Based on some phylogenetic studies, the Zagros Mountains separate the central Iran from the Mesopotamian plain in the west and stand as a natural barrier between these parts and thus have essential contributions to the speciation of reptiles and amphibians (Fouad & Sissakian, Citation2011; Ghaedi et al., Citation2021).
Snakes are among the most successful reptiles, with more than 3600 species, which mainly exist in all continents except Antarctica (Greene, Citation1997). These limbless predators inhabit every continent except Antarctica and have successfully colonized terrestrial, freshwater, and marine habitats. Of the snake families, Colubridae is the most species and comprises of more than half of the world’s described snake species (>1,700 species, Vitt & Caldwell, Citation2013). Some snake species are complicated to detect due to their low densities, long periods of inactivity, and limited studies and information (Hosseinzadeh et al., Citation2017). Thus, their distribution ranges, and suitable habitats may be underestimated and less well known than the other reptiles, and these models can be used to fill these knowledge gaps to better know of these species (Bombi et al., Citation2009; Santos et al., Citation2006).
The genus Spalerosophis is a genus of Colubrinae, which occurs in arid and semiarid regions from northwestern Africa to northern India (Marx, Citation1959). During the last 150 years, the systematic concept of genus Spalerosophis was subject to modifications. Until now, six species of Spalerosophis have been recognized, consider S. diadema occurring from the western Sahel and Sudan to Central Asia and northwest India, S. arenarius and S. atriceps from north India and Pakistan, S. dolichospilus from the northwestern Africa, S. josephscorteccii from Galgalo Oasis in northwest Somalia and S. microlepis from Zagros Mountain in the west of Iran Schätti et al. (Citation2009). Spalerosophis diadema is an aglyphous, medium-sized, and the most widely distributed species of the genus Spalerosophis. It is one of the most doubtful species of this genus with at least two populations, S. d. schirasianus distributed from Iran to North India and S. d. cliffordii distributed from western Iran to Morocco in northwest Africa () (Yadollahvandmiandoab et al., Citation2018). The question about this species is whether its populations are in the phase of separation from each other and what is the difference is between the habitat selection of these populations.
Figure 1. Geographical distribution range of the S. d. schirasianus and S. d. cliffordii based on data taken from Marx (Citation1959), Baig and Masroor (Citation2008), www.GBIF.org website and our study.
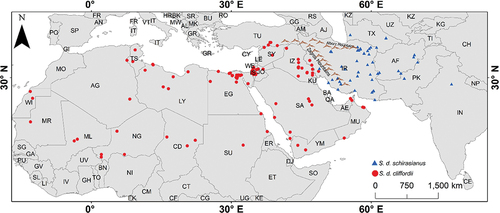
In this study, we investigate the potential geographic distribution for the populations of S. diadema using a near range-wide of sampling was investigated. We employed maximum entropy distribution (Maxent) modeling. Maxent was used to construct the potential distributions of this species under current environmental conditions. The aims of this study were: (1) to identify factors affecting the distribution of the two populations of S. diadema, (2) to identify suitable habitats of the two populations for S. diadema, and (3) to predict habitat overlap between S. d. cliffordii and S. d. schirasianus. Using SDM models allows us to help identify the main environmental factors affecting the distribution pattern of these snakes and to estimate possible events like uplifting the Zagros Mountains in western Iran, as a natural barrier at the approximate boundary of the split of the two studied populations that helped shape the current geographical distribution patterns.
Material and methods
Occurrence data collection
In order to run a presence-only habitat suitability model, two types of data, including species occurrences and environmental predictors, are required. From 2010 to 2020, a total of 164 occurrence records, including 59 localities’ data of S. d. schirasianus and 105 of S. d. cliffordii, obtained from literature records, Global Biodiversity Information Facility (GBIF; https://www.gbif.org/, accessed on 6 May 2020), and localities’ data on museum specimens (see Appendix I).
Environmental variables data
Environmental variables include climate (precipitation, temperature, and land surface temperature), topography, land cover LULC, and vegetation predictors (). Precipitation and temperature predictors were extracted from the WorldClim database as an initial set of 19 predictors at a spatial resolution of 30′′ (~1 km). Land Surface Temperature variables were acquired for 12 months in 2020 separately by Moderate Resolution Imaging Spectroradiometer (MODISFootnote1) – Terra at a spatial resolution of 30′′ (~1 km). Normalized Difference Vegetation Index (NDVIFootnote2) predictors were calculated for 12 months in 2020 separately using Landsat TM imagery at a spatial resolution of 30 m. Shuttle Radar Topography Mission (SRTMFootnote3) data with a spatial resolution of 90 m was used to generate the Elevation, Slope, and Roughness variables. Roughness was calculated as the standard deviation of the elevation of all raster cells at the 1 km spatial resolution. The land cover predictor of the region LULC was acquired from MODIS land cover type (Friedl & Sulla-Menashe, Citation2015) at a spatial resolution of 500 m. Finally, 1 km spatial resolution was used as the final spatial scale to model. Pearson correlation coefficient (r) was used to test the correlation between variables. Predictors with Pearson correlation coefficient values −0.7 > r > +0.7 were excluded from the modeling ().
Table 1. Environmental variables’ characteristics.
Table 2. Environmental variables and their relative contributions (the highest values are in bold).
Species distribution modeling
The habitat suitability of the two populations was performed by the MaxEnt model independently. MaxEnt employs the principle of maximum entropy, which means that the model finds the probability distribution nearest to a uniform distribution, with the condition that the predicted value for each environmental predictor must conform to its actual data (Phillips, Citation2008; Phillips et al., Citation2006). MaxEnt predicts the habitat suitability and produces response curves for all environmental predictors (Elith et al., Citation2011). MaxEnt was selected to develop predictive models for two reasons: (1) MaxEnt has performed robustly for small sample sizes and has outperformed other methods in such cases; (2) owing to their lower sensitivity to over-prediction compared to standard GLM models (Elith et al., Citation2011; Shcheglovitova & Anderson, Citation2013). The empirical average for predictors is calculated using values at presence points. Previous studies have shown the reliability of MaxEnt’s default settings for different species under various environmental conditions and sampling bias (Elith et al., Citation2011; Hosseini et al., Citation2019; Phillips & Dudík, Citation2008); as a result, the model was run on default settings (regularization multiplier β = 1; auto features; convergence threshold = 0.0000, background points = 200) and Presence points were randomly divided into training and test datasets (80% and 20%, respectively). Environmental predictor’s contribution for the best performing was calculated using the Jackknife analysis. The Jackknife analysis was repeated to select variables such that any variable contribution to the model with relative contributions less than 2%.
Model evaluation
The model was evaluated using TSSFootnote4 (Allouche et al., Citation2006), AUCFootnote5 (Fielding & Bell, Citation1997), and Boyce index (Boyce et al., Citation2002) that was calculated in the modEvA R package. MaxEnt provides a continuous map of habitat suitability. A threshold value for generating a classified map (predicted presence or absence) was selected by both maximizing training sensitivity and specificity (Liu et al., Citation2011). Next, grid cells with a probability value smaller than the threshold were defined as 0, marking unsuitable areas. All cells with probability value more remarkable than the threshold value were given 1 to indicate a suitable habitat for the species. Finally, classified maps of habitat suitability were used to evaluate the overlap between the two population’s suitable habitats in Idrisi TerrSet.
Results
Species distribution models
TSS, AUC and Boyce index values showed that MaxEnt for Spalerosophis diadema cliffordii (AUC: 0.95 ± 0.008, TSS: 0.85 ± 0.009, Boyce index: 90 ± 0.921), and Spalerosophis diadema schirasianus (AUC: 0.97 ± 0.066, TSS: 0.82 ± 0.011, Boyce index: 91 ± 0.003) perform significantly better than random selection.
Results of Jackknife tests revealed reveal that the importance of environmental predictors is similar for the two populations (). Annual mean Temperature, Precipitation of coldest quarter, NDVI in May, and Elevation are the most critical environmental variables in modeling habitat suitability for the two populations.
Response curves are revealed to show that the two populations react similarly to environmental variables (). The results demonstrated that increased annual mean temperature caused an increase in habitat suitability for the two populations, while precipitation of the coldest quarter and elevation had the opposite effect on the two populations. Increased precipitation in the coldest quarter and elevation caused a decrease in habitat suitability for the two populations. The response curve for NDVI in May showed a positive/negative relationship with the habitat suitability for the two populations. In the beginning, increased NDVI caused an increase in habitat suitability until 0.2–0.4 and then gradually declined with increasing values of NDVI in May.
Figure 2. Relationships between environmental variables and the probability of presence for S. d. cliffordii (left) and S. d. schirasianus (right) (red curves show the mean response and blue margins are ± 1SD).
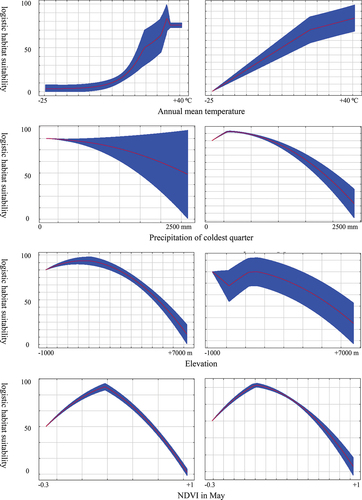
The habitat suitability maps of the MaxEnt for each population are illustrated in . Our results show that a small area of the region is suitable for the population. Suitable habitats of S. d. schirasianus cover a large area of Iran. Suitable habitats of S. d. cliffordii are scattered in more countries than S. d. schirasianus (). The results revealed that a small area of suitable habitats for two populations overlapped of around 10% ().
Discussion
Over the past decades, several attempts have been made to evaluate the taxonomy of the genus Spalerosophis based on morphological evidence (Baig & Masroor, Citation2008; Marx, Citation1959; Schatti et al., Citation2010; Schätti et al., Citation2009). Finally, Schätti et al. (Citation2009) published a key of the genus Spalerosophis according to traditional morphology and discussed the history of classification of this genus. He showed that western and eastern populations of S. diadema are very similar considering morphological characteristics, except for the number of subcaudal scales, which is following previous studies (Baig & Masroor, Citation2008; Schatti et al., Citation2010). So, he divided these two populations of S. diadema just based on the number of subcaudal scales: Saharo-Arabian population as S. d. cliffordii with less than 80 subcaudal scales and the Iranian population as S. d. schirasianus with more than 80 subcaudal scales.
There are two species of Spalerosophis in Iran: Spalerosophis diadema (distributed from North Africa to central India) and S. microlepis (Endemic species of Iran). S. diadema is a thermophilous species often found in arid regions (open plains and deserts) and near agricultural farms (Amr & Disi, Citation2011). Based on Moadab et al. (Citation2018), the Zagros Mountains are not a suitable habitat for S. diadema. However, they can be found in both the inner and outer edges of the mountains, indicating the role of slope in the distribution of this species. Also, in this research, the most important variable for S. diadema is the average temperature of the coldest quarter.
In another study that was done on Spalerosophis microlepis in the Zagros Mountains, the results showed that precipitation in the coldest quarter and annual precipitation have the highest amount of percent contribution in modeling (Hosseinzadeh et al., Citation2017). Our findings of SDM analyses results demonstrated that the two populations react similarly to environmental variables, and among the 13 environmental variables that were used in this study, the most critical factors for the two populations were annual mean temperature, precipitation of coldest quarter, NDVI in May, and elevation, as these factors contributed about 80–85% of the environmental factors to the entire model. Although our distribution models show some overlap of the potential distributions of species (around 10%) among Mesopotamian plain and Afghanistan, there is no confirmed report or voucher specimen proving the overlap distribution in these regions.
Our results also revealed that S. d. cliffordii and S. d. schirasianus are mainly found in areas with low vegetation cover. The species is dependent on areas with sparse vegetation because of refuge and food. This result agrees with previous studies which have supported the relationship between the habitat suitability of the species and low vegetation cover (Almasieh et al., Citation2019; Pour et al., Citation2016). Also, Almasieh et al. (Citation2019) showed that the probability of the species’ occurrence increased in agricultural lands that had sparse vegetation.
As studies have shown, while the distribution of a species seems to be influenced chiefly by climatic conditions, landscape features, ecological communities, predator–prey interactions, and anthropogenic pressures, the large-scale distribution of the species will depend on the existence of favorable climatic conditions plus the dispersal ability (Austin & Van Nicl, Citation2011; Sahlean et al., Citation2014). Although our distribution models show western Afghanistan as a potentially suitable habitat for S. d. cliffordii and also some parts of Iraq and Syria as suitable habitats of S. d. schirasianus, it seems that these populations are isolated from each other by physical barriers and could not colonize these areas.
The Zagros orogeny, as a barrier to distribution and gene flow between the western and eastern populations, has also been reported in some taxonomic groups of small vertebrates (Asadi Aghbolaghi et al., Citation2019; Rezazadeh et al., Citation2020). Such a natural barrier is effective in interrupting the distribution and communication of reptile populations and species (Ahmadzadeh et al., Citation2017; Forcina et al., Citation2019; Ghaedi et al., Citation2021; Parsa et al., Citation2009). Findings of the present study provided evidence that Zagros Mountain probably acted as a real natural barrier and prevented the distribution of Spalerosophis populations between the western and eastern parts of the mountains because of vicariance and geological events that eventually led to the separation of S. d. schirasianus and S. d. cliffordii in the central Iranian Plateau and Mesopotamian plain to western Africa, respectively. Further phylogenetic studies are necessary to investigate the phylogenetic relationships, genetic diversity, and divergence time for these populations to prove the role of the Zagros Mountains in this separation.
Using MaxEnt in ecological studies has become common practice (Fourcade et al., Citation2018). Nevertheless, MaxEnt models have shortcomings, such presence-only models underperform in predicting species’ distributions compared to presence-absence models since absence data can help indicate unsuitable habitats for species, a process known as “fixing the floor”. Thus, the importance of absence data is in eliminating low suitability areas that could have been categorized as suitable habitats if presence-only data had been used (Lahoz‐Monfort et al., Citation2014); and MaxEnt models can take advantage of museum and atlas data, which provide presence only information. However, there are biases in the data provided by museums and atlases towards accessible areas or areas where species are expected to occur (Pearce & Boyce, Citation2006). The data we have used as input face the same challenges, rather than being collected with strategies such as random or systematic sampling, data in museums and atlases are gathered without sampling and any particular purpose in mind (Elith et al., Citation2006). Biases in sampling intensity can therefore arise (Guillera‐Arroita et al., Citation2015). Unlike the presence-absence models, presence-only models such as MaxEnt are strongly affected by such sampling biases.
Conclusion
The distribution of the species is influenced by various factors such as climatic factors, topography, fishing relations, etc. At the same time, the existence of geographical barriers can also be one of the influential factors in the dispersion of animal species. The Zagros Mountain range as an obstacle has prevented the communication of the populations of the spalerosophis. It has finally led to the separation of the S. d. schirasianus and the S. d. cliffordii.
Acknowledgments
We wish to thank Prof. Eskandar Rastegar-Pouyani from Hakim Sabzevari University, Iran, Prof. Annamaria Nistri from Natural history museum of Florence, Italy, Prof. Andreas Schmitz from Natural History Museum of Geneva, Switzerland, Prof. Nicolas Vidal from National Museum of Natural History, Paris, France, Prof. Wolfgang Böhme and Dr. Morris Flecks from Zoological Research Museum Alexander Koenig, Bonn, Germany for their cooperation on access to specimens and scientific supports. Also, we thank CNPq for the research scholarship.
Disclosure statement
No potential conflict of interest was reported by the author(s).
Notes
1 Moderate Resolution Imaging Spectroradiometer.
2 Normalized Difference Vegetation Index.
3 Shuttle Radar Topography Mission.
4 True Skill Statistic.
5 Area Under the Curve.
References
- Agard, P., Omrani, J., Jolivet, L., & Mouthereau, F. (2005). Convergence history across Zagros (Iran): Constraints from collisional and earlier deformation. International Journal of Earth Sciences, 94(3), 401–419. https://doi.org/10.1007/s00531-005-0481-4
- Ahmadzadeh, F., Carretero, M. A., Rödder, D., Harris, D. J., Freitas, S. N., Perera, A., & Böhme, W. (2013). Inferring the effects of past climate fluctuations on the distribution pattern of Iranolacerta (Reptilia, Lacertidae): Evidence from mitochondrial DNA and species distribution models. Zoologischer Anzeiger-A Journal of Comparative Zoology, 252(2), 141–148. https://doi.org/10.1016/j.jcz.2012.05.002
- Ahmadzadeh, F., Lymberakis, P., Pirouz, R. S., & Kapli, P. (2017). The evolutionary history of two lizards (Squamata: Lacertidae) is linked to the geological development of Iran. Zoologischer Anzeiger, 270, 49–56. https://doi.org/10.1016/j.jcz.2017.09.003
- Allouche, O., Tsoar, A., & Kadmon, R. (2006). Assessing the accuracy of species distribution models: Prevalence, kappa and the true skill statistic (TSS). Journal of Applied Ecology, 43(6), 1223–1232. https://doi.org/10.1111/j.1365-2664.2006.01214.x
- Almasieh, K., Mirghazanfari, S. M., & Mahmoodi, S. (2019). Biodiversity hotspots for modeled habitat patches and corridors of species richness and threatened species of reptiles in central Iran. European Journal of Wildlife Research, 65(6), 1–13. https://doi.org/10.1007/s10344-019-1335-x
- Amr, Z., & Disi, A. H. M. A. D. (2011). Systematics, distribution and ecology of the snakes of Jordan. Vertebrate Zoology, 61(2), 179–266. https://doi.org/10.3897/vz.61.e31150
- Ananjeva, N. B., Golynsky, E. E., Lin, S. M., Orlov, N. L., & Tseng, H. Y. (2015). Modeling habitat suitability to predict the potential distribution of the kelung cat snake Boiga kraepelini Steineger, 1902. Russian Journal of Herpetology, 22, 197–205. https://doi.org/10.30906/1026-2296-2015-22-3-197-205
- Asadi Aghbolaghi, M., Ahmadzadeh, F., Kiabi, B., & Keyghobadi, N. (2019). The permanent inhabitant of the oak trees: Phylogeography and genetic structure of the Persian squirrel (Sciurus anomalus). Biological Journal of the Linnean Society, 127(2), 197–212. https://doi.org/10.1093/biolinnean/blz032
- Austin, M. P., & Van Nicl, K. P. (2011). Improving species distribution models for climate change studies: Variable selection and scale. Journal of Biogeography, 38(1), 1–8. https://doi.org/10.1111/j.1365-2699.2010.02416.x
- Baig, J. K., & Masroor, R. (2008). The snakes of the genus Spalerosophis JAN, 1865 in Indo-Pakistan and Iran. Herpetozoa, 20(3/4), 109–115.
- Bernardes, M., Rödder, D., Nguyen, T. T., Pham, C. T., Nguyen, T. Q., & Ziegler, T. (2013). Habitat characterization and potential distribution of Tylototriton vietnamensis in northern Vietnam. Journal of Natural History, 47(17–18), 1161–1175. https://doi.org/10.1080/00222933.2012.743611
- Bombi, P., Luiselli, L., Capula, M., & Salvi, D. (2009). Predicting elusiveness: Potential distribution model of the Southern smooth snake, Coronella girondica, in Italy. Acta Herpetologica, 4(1), 7–13. https://doi.org/10.13128/Acta_Herpetol-2950
- Boyce, M. S., Vernier, P. R., Nielsen, S. E., & Schmiegelow, F. K. (2002). Evaluating resource selection functions. Ecological Modelling, 157(2–3), 281–300.
- De Marco, P., Nóbrega, C. C., & Bosso, L. (2018). Evaluating collinearity effects on species distribution models: An approach based on virtual species simulation. PloS One, 13(9), e0202403. https://doi.org/10.1371/journal.pone.0202403
- Ebrahimi Pour, F., Rastegar-Pouyani, E., & Ghorbani, B. (2016). A preliminary study of the Reptile’s fauna in northwestern Yazd province, Iran. Russian Journal of Herpetology, 23(4), 243–248.
- Elith, J., Graham, C. H., Anderson, R. P., Dudı´k, M., Ferrier, S., Guisan, A., Hijmans, R. J., Huettmann, F., Leathwick, J. R., Lehmann, A., Li, J., Lohmann, L. G., Loiselle, B. A., Manion, G., Moritz, C., Nakamura, M., Nakazawa, Y., Overton, J. M. … Zimmermann, N. E. (2006). Novel methods improve prediction of species’ distributions from occurrence data. Ecography, 29(2), 129–151. https://doi.org/10.1111/j.2006.0906-7590.04596.x
- Elith, J., Graham, C. P., Anderson, R., Dudík, M., Ferrier, S., Guisan, A. … Zimmermann, N. (2006). Novel methods improve prediction of species’ distributions from occurrence data. Ecography, 29(2), 129–151. https://doi.org/10.1111/j.2006.0906-7590.04596.x
- Elith, J., & Leathwick, J. R. (2009). Species distribution models: Ecological explanation and prediction across space and time. Annual Review of Ecology, Evolution, and Systematics, 40(1), 677–697. https://doi.org/10.1146/annurev.ecolsys.110308.120159
- Elith, J., Phillips, S. J., Hastie, T., Dudík, M., Chee, Y. E., & Yates, C. J. (2011). A statistical explanation of MaxEnt for ecologists. Diversity and Distributions, 17(1), 43–57. https://doi.org/10.1111/j.1472-4642.2010.00725.x
- Fathinia, B., Rastegar-Pouyani, N., Darvishnia, H., & Rajabizadeh, M. (2010). The snake fauna of Ilam Province, southwestern Iran. Iranian Journal of Animal Biosystematics, 6(1), 9–23. https://doi.org/10.22067/IJAB.V6I1.9153
- Fielding, A. H., & Bell, J. F. (1997). A review of methods for the assessment of prediction errors in conservation presence/absence models. Environmental Conservation, 24(1), 38–49. https://doi.org/10.1017/S0376892997000088
- Forcina, G., Guerrini, M., Panayides, P., Hadjigerou, P., Khan, A. A., & Barbanera, F. (2019). Molecular taxonomy and intra-Palaearctic boundary: New insights from the biogeography of the black francolin (Francolinus francolinus) by means of microsatellite DNA. Systematics and Biodiversity, 17(8), 759–772. https://doi.org/10.1080/14772000.2019.1691673
- Fouad, S. F., & Sissakian, V. K. (2011). Tectonic and structural evolution of the Mesopotamia plain. Iraqi Bulletin of Geology and Mining, 4(4), 33–46.
- Fourcade, Y., Besnard, A. G., & Secondi, J. (2018). Paintings predict the distribution of species, or the challenge of selecting environmental predictors and evaluation statistics. Global Ecology and Biogeography, 27(2), 245–256. https://doi.org/10.1111/geb.12684
- Franzen, M. (1999). A record of Spalerosophis diadema (Reptilia: Colubridae) from Adıyaman province, Turkey. Zoology in the Middle East, 19(1), 33–36. https://doi.org/10.1080/09397140.1999.10637793
- Friedl, M., & Sulla-Menashe, D. (2015). MCD12Q1 MODIS/Terra+Aqua land cover type yearly L3 global 500m SIN grid V006 [data set]. NASA EOSDIS Land Processes DAAC.
- Gasperetti, J. (1988). Snakes of Arabia. Fauna of Saudi Arabia, 9(9), 169–450.
- GBIF. (2020). Global Biodiversity Information Facility. Available from https://www.gbif.org/ [Accessed 8 April 2020].
- Ghaedi, Z., Badri, S., Saberi-Pirooz, R., Vaissi, S., Javidkar, M., & Ahmadzadeh, F. (2021). The Zagros Mountains acting as a natural barrier to gene flow in the middle East: More evidence from the evolutionary history of spiny-tailed lizards (Uromasticinae: Saara). Zoological Journal of the Linnean Society, 192(4), 1123–1136. https://doi.org/10.1093/zoolinnean/zlaa113
- Göçmen, B., Franzen, M., Yildiz, M. Z., Akman, B., & Yalçinkaya, D. (2009). New locality records of eremial snake species in southeastern Turkey (Ophidia: Colubridae, Elapidae, Typhlopidae, Leptotyphlopidae). Salamandra, 45(2), 110–114.
- Goudarzi, F., Hemami, M. R., Rancilhac, L., Malekian, M., Fakheran, S., Elmer, K. R., & Steinfartz, S. (2019). Geographic separation and genetic differentiation of populations are not coupled with niche differentiation in threatened Kaiser’s spotted newt (Neurergus kaiseri). Scientific Reports, 9(1), 1–12. https://doi.org/10.1038/s41598-019-41886-8
- Graham, C. H., Ferrier, S., Huettman, F., Moritz, C., & Peterson, A. T. (2004). New developments in museum-based informatics and applications in biodiversity analysis. Trends in Ecology & Evolution, 19(9), 497–503. https://doi.org/10.1016/j.tree.2004.07.006
- Greene, H. W. (1997). Snakes: The evolution of mystery in nature. Univ of California Press. https://doi.org/10.1525/9780520935433
- Guillera‐Arroita, G., Lahoz‐Monfort, J. J., Elith, J., Gordon, A., Kujala, H., Lentini, P. E., McCarthy, M. A., Tingley, R., & Wintle, B. A. (2015). Is my species distribution model fit for purpose? Matching data and models to applications. Global Ecology and Biogeography, 24(3), 276–292. https://doi.org/10.1111/geb.12268
- Hatzfeld, D., Authemayou, C., van der Beek, P., Bellier, O., Lavé, J., Oveisi, B., Tatar, M., Tavakoli, F., Walpersdorf, A., & Yamini-Fard, F. (2010). The kinematics of the Zagros Mountains (Iran) (Vol. 330). Geological Society.
- Hernandez, P. A., Graham, C. H., Master, L. L., & Albert, D. L. (2006). The effect of sample size and species characteristics on performance of different species distribution modeling methods. Ecography, 29(5), 773–785. https://doi.org/10.1111/j.0906-7590.2006.04700.x
- Hewitt, G. M. (2011). Quaternary phylogeography: the roots of hybrid zones. Genetica, 139(5), 617–638.
- Hosseini, M., Farashi, A., Khani, A., & Farhadinia, M. S. (2019). Landscape connectivity for mammalian megafauna along the Iran-Turkmenistan-Afghanistan borderland. Journal for Nature Conservation, 52, 125735. https://doi.org/10.1016/j.jnc.2019.125735
- Hosseinzadeh, M. S., Ghezellou, P., & Kazemi, S. M. (2017). Predicting the potential distribution of the endemic snake Spalerosophis microlepis (Serpentes: Colubridae), in the Zagros Mountains, western Iran. Salamandra, 53(2), 294–298.
- Lahoz‐Monfort, J. J., Guillera‐Arroita, G., & Wintle, B. A. (2014). Imperfect detection impacts the performance of species distribution models. Global Ecology and Biogeography, 23(4), 504–515. https://doi.org/10.1111/geb.12138
- Liu, C., White, M., & Newell, G. (2011). Measuring and comparing the accuracy of species distribution models with presence–absence data. Ecography, 34(2), 232–243. https://doi.org/10.1111/j.1600-0587.2010.06354.x
- Malekoutian, M., Sharifi, M., & Vaissi, S. (2020). Mitochondrial DNA sequence analysis reveals multiple Pleistocene glacial refugia for the Yellow‐spotted mountain newt, Neurergus derjugini (Caudata: Salamandridae) in the mid‐Zagros range in Iran and Iraq. Ecology and Evolution, 10(5), 2661–2676. https://doi.org/10.1002/ece3.6098
- Marx, H. (1959). Review of the Colubrid snake genes Spalerosophis. Fieldiana Zoology, 39(30), 347–361.
- Moadab, M., Zargan, J., Rastegar-Pouyani, E., & Hajinourmohammadi, A. (2018). Modeling the potential distribution of Spalerosophis diadema (Schlegel, 1837) (Serpents: Colubridae) in Iran. Herpetology Notes, 11(11), 805–808.
- Parsa, H., Oraie, H., Khosravani, A., & RASTEGAR, P. N. (2009). Systematics and distribution of the Iranian Plateau leaf-toed geckos of the genus Asaccus (Sauria: Gekkonidae). Iranian Journal of Animal Biosystematics, 52, 43–55. https://doi.org/10.22067/IJAB.V5I2.3338
- Pearce, J. L., & Boyce, M. S. (2006). Modelling distribution and abundance with presence‐only data. Journal of Applied Ecology, 43(3), 405–412. https://doi.org/10.1111/j.1365-2664.2005.01112.x
- Peterson, A. T. (2006). Uses and requirements of ecological niche models and related distributional models. Biodiversity Informatics, 3(0). https://doi.org/10.17161/bi.v3i0.29
- Phillips, S. J. (2008). Transferability, sample selection bias and background data in presence-only modelling: A response to Peterson et al. (2007). Ecography, 31(2), 272–278. https://doi.org/10.1111/j.0906-7590.2008.5378.x
- Phillips, S. J., Anderson, R. P., & Schapire, R. E. (2006). Maximum entropy modeling of species geographic distributions. Ecological Modelling, 190(3–4), 231–259. https://doi.org/10.1016/j.ecolmodel.2005.03.026
- Phillips, S. J., & Dudík, M. (2008). Modeling of species distributions with Maxent: New extensions and a comprehensive evaluation. Ecography, 31(2), 161–175. https://doi.org/10.1111/j.0906-7590.2008.5203.x
- Pour, F. E., Rastegar-Pouyani, E., & Ghorbani, B. (2016). A preliminary study of the Reptile’s fauna in northwestern Yazd province, Iran. Russian Journal of Herpetology, 23(4), 243–248.
- Rezazadeh, E., Aliabadian, M., Darvish, J., & Ahmadzadeh, F. (2020). Diversification and evolutionary history of brush-tailed mice, Calomyscidae (Rodentia), in southwestern Asia. Organisms Diversity & Evolution, 20(1), 155–170. https://doi.org/10.1007/s13127-019-00426-y
- Rhadi, F. A., Mohammed, R. G., Rastegar-Pouyani, N., Rastegar-Pouyani, E., & Yousefkhani, S. S. (2017). On the snake fauna of central and Southern Iraq and some Zoogeographic Remarks. Russian Journal of Herpetology, 24(4), 251–266. https://doi.org/10.30906/1026-2296-2019-24-4-251-266
- Rödder, D., & Lötters, S. (2010). Explanative power of variables used in species distribution modelling: An issue of general model transferability or niche shift in the invasive Greenhouse frog (Eleutherodactylus planirostris). Naturwissenschaften, 97(9), 781–796. https://doi.org/10.1007/s00114-010-0694-7
- Sahlean, T. C., Gherghel, I., Papeş, M., Strugariu, A., Zamfirescu, Ş. R., & Bohrer, G. (2014). Refining climate change projections for organisms with low dispersal abilities: A case study of the Caspian whip snake. PLoS One, 9(3), e91994. https://doi.org/10.1371/journal.pone.0091994
- Santos, X., Brito, J. C., Sillero, N., Pleguezuelos, J. M., Llorente, G. A., Fahd, S., & Parellada, X. (2006). Inferring habitat-suitability areas with ecological modelling techniques and GIS: A contribution to assess the conservation status of Vipera latastei. Biological Conservation, 130(3), 416–425. https://doi.org/10.1016/j.biocon.2006.01.003
- Schatti, B., Ineich, I., & Kucharzewski, C. (2010). Nominal taxa of Spalerosophis diadema (Schlegel, 1837) from Iraq to Pakistan – two centuries of confusion (Reptilia: Squamata: Colubrinae). Revue Suisse de Zoologie, 117, 637–664. https://doi.org/10.5962/bhl.part.145579
- Schätti, B., Tillack, F., & Helfenberger, N. (2009). A contribution to Spalerosophis microlepis Jan 1865, with a short review of the genus and a key to the species (Squamata: Serpentes: Colubridae). Herpetozoa, 22(3/4), 115–135.
- Shcheglovitova, M., & Anderson, R. P. (2013). Estimating optimal complexity for ecological niche models: A jackknife approach for species with small sample sizes. Ecological Modelling, 269, 9–17. https://doi.org/10.1016/j.ecolmodel.2013.08.011
- Tarkhnishvili, D. (2014). Historical biogeography of the Caucasus (No. A). NOVA Science Publishers.
- Vitt, L. J., & Caldwell, J. P. (2013). Herpetology: An introductory biology of amphibians and reptiles. Elsevier. https://doi.org/10.1016/B978-0-12-386919-7.00002-2
- Wisz, M. S., Hijmans, R. J., Li, J., Peterson, A. T., Graham, C. H., & Guisan, A. NCEAS Predicting Species Distributions Working Group. (2008). Effects of sample size on the performance of species distribution models. Diversity & Distributions, 14(5), 763–773. https://doi.org/10.1111/j.1472-4642.2008.00482.x
- Yadollahvandmiandoab, R., Mesquita, D. O., & Kami, H. G. (2018). A preliminary study on the biology of the Diadem snake (Spalerosophis diadema), from Iran (Reptilia: Colubridae). Herpetology Notes, 11, 481–487.
- Yousefkhani, S. S. H., Yousefi, M., Khani, A., & Pouyani, E. R. (2014). Snake fauna of Shirahmad wildlife refuge and Parvand protected area, Khorasan Razavi province, Iran. Hepetology Notes, 7, 75–82.
- Zareian, H., Esmaeili, H. R., Gholamhosseini, A., Teimory, A., Zohrabi, H., & Kami, H. G. (2009). A preliminary study on the herpetofauna of Gorm mountain no- hunting zone, Jahrom, Fars province. Taxonomy and Biosystematics, 1(1), 1–8.