Abstract
In Holsteins, emerging evidence suggests that long non-coding RNAs (lncRNAs) are actively involved in the regulation of mammary gland development. However, the lncRNA expression pattern in the early growth stage of Holstein mammary gland remains little known. In this study, we comprehensively analyzed 11 mammary gland tissue RNA-Seq datasets derived from Holstein heifer calves (ca. 8 weeks old) retrieved from the Sequence Read Archive database. We identified a total of 588 lncRNAs in the intergenic region. By comparing the sequences with NONCODEv5 database, 241 novel lncRNAs were detected in the mammary gland. Prediction of cis-regulated target gene revealed that 364 out of 588 lncRNAs potentially targeted 417 protein-coding genes. Furthermore, Gene Ontology enrichment determined that potential target genes were mainly involved in the terms associated with energy metabolism and cell differentiation, such as cellular respiration, respiratory electron transport chain, electron transport chain, and regulation of cell differentiation, which were closely related to mammary gland development. This study provides potential candidate lncRNAs in regulating mammary gland development in the early growth stage of Holsteins, as well as a better general understanding of Holstein mammary gland development.
Introduction
Holsteins are one of the most popular cow breeds in the world mainly because of their high milk yield. Their mammary glands are an important organ for Holsteins as they affect both the yield and quality of the produced milk intended for human consumption. Therefore, improving the development of the mammary gland has long become an interest in the dairy industry. Mammary gland development is a continuous process in the entire life of Holsteins starting from the embryonic, pre-weaning, pubertal, and until adult development (Macias & Hinck Citation2012; Vailati-Riboni et al. Citation2018). In particular, a well development of the mammary gland in pre-weaning may contribute to greater future production (Soberon et al. Citation2012). The association mechanisms and underlying genetic factor for the regulation of mammary gland development in the early growth stage have recently garnered increased attention.
The vast majority of genome transcripts have been found to be non-coding RNAs (ncRNAs) in eukaryotes (Stein Citation2004). NcRNAs are functional RNAs that do not encode proteins but play important roles in various cellular processes (Li et al. Citation2014; Chen & Yang Citation2015; He et al. Citation2018). Specifically, long non-coding RNAs (lncRNAs) are a class of ncRNAs that have more than 200 nucleotides and lack functional open reading frames (ORFs) (Derrien et al. Citation2012; Rinn & Chang Citation2012; Batista & Chang Citation2013). They can be classified into four types based on their location in the genome, namely antisense lncRNAs, intronic lncRNAs, sense lncRNAs, and intergenic lncRNAs (lincRNAs).
Interestingly, large-scale genome sequencing has revealed that lncRNAs are positively involved in mammary gland metabolism and development. For example, 28,562 lncRNAs have been found to be expressed in the bovine mammary gland using RNA-Seq, of which 174 were differentially expressed during heat stress, suggesting that lncRNAs were involved in the physiological adaptation of mammary glands (Li et al. Citation2018). Ibeagha-Awem et al. also found 4955 lncRNAs expressed in the mammary gland of treated cows with a dietary response to 5% linseed oil or 5% safflower oil, demonstrating that lncRNAs expression pattern in the mammary gland could be modulated by nutritional signal (Ibeagha-Awemu et al. Citation2018). Yang et al., on the other hand, identified 3746 differentially expressed lncRNAs from the dry and lactation mammary glands of Holstein cows, suggesting that the lncRNAs were important regulators for lactation cycle (Yang et al. Citation2018). Meanwhile, Tong et al. reported 36 lincRNA in the Holstein mammary gland, which were related to 172 milk quantitative trait loci (QTLs) with one lincRNA found within clinical mastitis QTL region (Tong et al. Citation2017).
However, the lncRNA expression patterns in the early growth stage of Holstein mammary gland remain largely unknown. To investigate the lncRNA profiles and identify lncRNAs that were involved in mammary gland development in the early growth stage of Holstein, we comprehensively analyzed 11 RNA-Seq datasets that were generated from mammary gland parenchyma of Holsteins heifer calves (ca. 8 weeks old) and identified candidate lncRNAs including novel ones in the mammary gland using bioinformatics analysis. Gene Ontology (GO) enrichment revealed these lncRNAs might modulate mammary gland development via cis-regulating. Our findings provide a list of potential lncRNAs involved in regulating mammary gland development in the early growth stage of Holsteins, thus may contribute to improving yield and quality of the milk production, as well as expand our understanding of Holstein mammary gland.
Materials and methods
Ethics statement
No ethical approval is required for this study.
Data collection and reads quality check
In this study, 11 single-end RNA-Seq datasets (30–101 bp) generated by sequencing using an Illumina HiSeq 2500 platform were retrieved from the Sequence Read Archive (SRA) database (Table S1). As described in the original study by Loor and colleagues (Vailati-Riboni et al. Citation2018), those samples were subsets of Gene Expression Omnibus database GSE102435 and collected from mammary parenchyma of Holstein heifer calves (ca. 8 weeks old). The cattle (Bos taurus) reference genome (ARS-UCD1.2) and genome annotation file (GTF format file, release 96) were downloaded from the Ensembl website (http://asia.ensembl.org/). Known splice sites in the cattle genome were extracted from the genome annotation file using HISAT2 program (Kim et al. Citation2015). The quality of these retrieved reads in FASTQ format was evaluated using Fastqc program (Babraham Institute, http://www.bioinformatics.babraham.ac.uk/projects/fastqc/). QC check results yielded from Fastqc showed that all reads were of high quality and did not contain adapters (Table S2). Those retrieved reads were then used for other downstream analyses.
Transcript reconstruction and lncRNA identification
An inhouse lncRNA analysis pipeline was designed to identify lncRNAs in the mammary gland (). All clean reads for each of the samples were mapped back onto the cattle reference genome using read aligner HISAT2 (2.1.0) (Kim et al. Citation2015) with parameters “–known-splicesite-infile” and “–dta”. The transcripts were reconstructed for each sample by Stringtie program (v1.3.4d) (Pertea et al. Citation2016) with the parameters “-f 0.1“, “-a 10“, “-j 1“, and “-c 2.5“. Then, the 11 samples were merged using Stringtie with “-merge” parameter to generate a global, unified set of transcripts across multiple samples. The fragment per kilobase of transcript per million mapped reads (FPKM) were estimated for the merged transcripts using the Stringtie program (Pertea et al. Citation2016) with the parameters “-e” and “-B” (Pertea et al. Citation2016). To obtain new transcripts, merged transcripts were compared with the reference genome annotation file using Gffcompare software (v0.10.6, available online http://ccb.jhu.edu/software/stringtie/gffcompare.shtml), and only the unknown intergenic transcripts (marked with class code “u”) were subjected to further analyses (Trapnell et al. Citation2012). For the unknown intergenic transcripts, stringent conditions were performed to identify true lncRNAs by removing the transcripts that (1) had FPKM <0.5, (2) single exons, and (3) length size shorter than 200 bp. Then, (4) for the remaining transcripts, five softwares of CPC2 (Kong et al. Citation2007), CNCI (Sun et al. Citation2013), CPAT-H (model trained by human lncRNA) (Wang et al. Citation2013), CPAT_M (model trained by mouse lncRNA) (Wang et al. Citation2013), and Pfam (Finn et al. Citation2014) were employed to discriminate non-coding transcripts from the coding ones by calculating for coding ability. Further, (5) we selected the intersection of the non-coding transcripts yield from those five softwares (CPC2, CNCI, CPAT-H, CPAT_M, and Pfam) as the lncRNA set. Lastly, BLASTN (v2.8.1) was used to compare those predicted lncRNAs with NONCODEv5 database (Fang et al. Citation2018), and the standard for sequence similarity was defined with e-value of <0.000001. In addition, the sequence similarity of the identified lncRNAs was detected using software CD-HIT (Fu et al. Citation2012), and sequence identity threshold was set with default parameter.
Potential target prediction and functional enrichment
Most studies suggest that lncRNA expression could act on neighboring protein-coding RNAs by cis-regulating way (Ren et al. Citation2016; Wang et al. Citation2016). In this study, the coding genes located at the lncRNA within the distance of 10 kb were considered potential cis-regulated target genes, which were extracted using BEDtools windowBed program (Quinlan Citation2014). Cis-regulating networks that constructed based on lncRNA–target pairs were screened out using Cytoscape software (Shannon et al. Citation2003). The prediction of lncRNA functions was based on functional annotation of the potential cis-regulated target genes. GO enrichment for the potential target genes was performed using the David database (Huang et al. Citation2009).
Validation of lncRNAs by RT-PCR
The RT-PCR primers in the validation of lncRNAs were designed by online software Primer-BLAST (https://www.ncbi.nlm.nih.gov/tools/primer-blast/) (Table S3). Total RNA was extracted from bovine mammary epithelial cells (MAC-T) by Trizol reagent (Invitrogen, Hong Kong, China) and was converted into cDNA using PrimeScripts RT Reagent Kit containing gDNA Eraser (TAKARA, Dalian, China). PCR was performed using 2× Taq PCR Master Mix (TIANGEN, Beijing, China) and amplification reaction program used 94°C for 10 s, followed by 35 cycles of 94°C for 30 s, annealing temperature for 30 s and 72°C for 30 s, and final extension for 10 min at 72°C. PCR product was analyzed using 1.5% agarose gel.
Results
In this study, a total of 292,855,791 reads were retained from those originally retrieved from the SRA datasets after a quality check. About 96.84% of reads were successfully aligned back to the reference genome for each sample. An average of 35,975 transcripts (34,124–39,211) were reconstructed from all 11 samples (Table S1). After comparing with the reference annotation using Gffcompare program, a total of 1151 unknown intergenic transcripts were obtained. Most of the unknown intergenic transcripts contained 2–4 exons and were shorter than 1000 bp. Comparing with other chromosomes, chromosome 21 contained more unknown intergenic transcripts and was observed to be slightly different in unknown intergenic transcript length distribution and exon content ().
LncRNA-sets contained 767, 759, 714, 739, and 723 lncRNA transcripts that were predicted by CPC2, CNCI, CPAT-H, CPAT-M, and Pfam, respectively. The intersection of these lncRNA-sets yielded 588 lncRNA transcripts ( and Table S4). Those lncRNAs were further classified into lincRNA, which were located on the intergenic region of the cattle genome. The distribution of 588 lncRNAs on each chromosome is shown in . The ORF length size of most lncRNA transcripts was less than 100 (). The length size of the identified lncRNAs ranged from 203 to 12,421 bp, most of which ranged from 300 bp to 900 bp (). In addition, the distance between identified autosomal lncRNAs ranged from 114 bp to 56 M bp, showing a large span in distance. Sequence similarity analysis showed that the 558 lncRNAs were classified into 557 clusters and were very different in sequence structure. After comparing lncRNA sequences with NONCODEv5 database using BLASTN (v2.8.1), 347 out of the 588 lncRNAs were aligned to the database significantly (e-value <0.000001) (Table S5), and 241 novel lncRNAs were detected.
Figure 3. Identification and characterization of lncRNAs in the mammary gland of Holstein heifer calves. (a) A Venn diagram showing the lncRNA transcripts generated by CPC2, CPAT-H, CPAT-M, CNCI, and Pfam. The lncRNA identified by all five softwares was used for downstream analyses. (b) Distribution of lncRNAs on each chromosome. (c) ORF length size distribution of lncRNA transcripts. (d) Length distribution of lncRNA transcripts
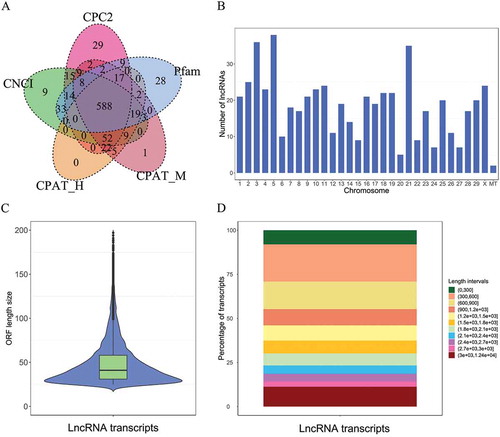
To obtain potential cis-regulated targets of identified lncRNAs, we explored the cis-regulated targets based on the different thresholds of ±100 kb, ±50 kb, and ±10kb from protein-coding gene to lncRNA. shows that approximately 2–6, 1–4, and 1–2 potential genes were targeted by each of the lncRNAs in the three respective different threshold conditions. shows that approximately 1–2, 1, and 1 lncRNAs were targeting each of the protein-coding genes in the three respective different threshold conditions. To increase the accuracy in the prediction of cis-regulated target gene, the ±10 kb threshold was selected as the filtering condition. With this threshold, for 364 out of 588 lncRNAs, we obtained 417 potential protein-coding RNAs, and two major lncRNA–target networks are shown in . In addition, for the remaining 224 lncRNAs, we have not obtained their cis-regulated target on their ±10 kb window.
Figure 4. Prediction of potential cis-regulated target genes of lncRNAs. (a) Distribution of potential cis-regulated target gene number for each of the lncRNAs in different threshold conditions. (b) Distribution of the number of lncRNAs that targeting each of the coding genes in different threshold conditions. (c) and (d) Two major cis-regulating networks. The color of orange represents protein-coding genes, and the color of green represents lncRNAs
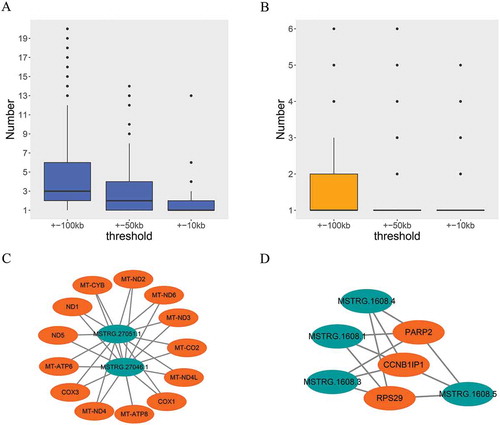
GO enrichment analysis further showed that the 417 potential cis-regulated targets were significantly enriched in 157 GO terms (p < 0.05), of which cellular respiration (GO:0045333), respiratory electron transport chain (GO:0022904), and electron transport chain (GO:0022900) were the top three listed GO terms in the biological process (BP) category; respiratory chain (GO:0070469), respiratory chain complex (GO:0098803), and mitochondrial respiratory chain (GO:0005746) were the top three listed GO terms in the cellular component (CC) category; and NADH dehydrogenase (ubiquinone) activity (GO:0008137), NADH dehydrogenase (quinone) activity (GO:0050136), and NADH dehydrogenase activity (GO:0003954) were the top three listed GO terms in the molecular function (MF) category (). In addition, cell development (GO:0048468), mesenchymal cell development (GO:0014031), and regulation of cell differentiation (GO:0060284) were also significantly enriched (Table S6).
Figure 5. GO enrichment analysis for potential cis-regulated target genes of lncRNAs. Histogram shows the top ten significantly enriched terms in BP, CC, and MF (p < 0.05)
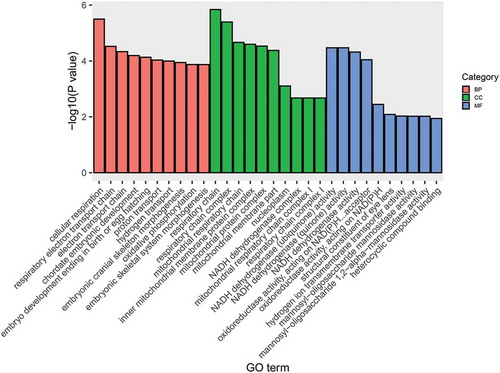
To confirm whether the lncRNAs identified by our identification pipeline were indeed expressed, we randomly selected 7 lncRNAs from overall 588 identified lncRNAs and examined them by RT-PCR. The RT-PCR result showed that the RT-PCR products of all seven lncRNAs (MSTRG.1772.2, MSTRG.8240.1, MSTRG.3052.1, MSTRG.229.1, MSTRG.8927.2, MSTRG.1214.1, and MSTRG.65.1) were amplified successfully and showed the expected size (). Therefore, the lncRNAs we identified in this study were reliable.
Discussion
Transcripts including protein-coding RNAs and ncRNAs have been widely discovered or further studied by RNA-Seq (Ibeagha-Awemu et al. Citation2018; Li et al. Citation2018; Yun et al. Citation2018). The ability of discovering new transcripts is a major advantage of RNA-Seq technology because of the global and systematic summary of whole transcripts for biological samples (Chen et al. Citation2017). In this study, 1151 unknown intergenic transcripts were assembled by reconstruction from a transcriptome. Credible lncRNAs, not including false-positive ones, were obtained by calculating for the protein-coding ability of the unknown intergenic transcripts using different models from five different softwares including CPC2, CNCI, CPAT-H, CPAT-M, and Pfam. The intersection of non-coding results generated from these five softwares was considered as the lncRNAs. Our data showed that most ORFs of Holstein lncRNAs had a length size less than 100, consistent with previous studies that have reported short length size of ORFs for lncRNAs in some species of rabbit and mouse (Ruizorera et al. Citation2014; Wang et al. Citation2018). The length size of the most lncRNAs ranged from 300 bp to 900 bp, corroborating those reported by Tong et al. in adult Holstein (Tong et al. Citation2017).
In the past, lncRNAs were considered as a class of by-products of genomic transcription and did not possess any biological function (Doolittle Citation2013). However, in recent years, more and more studies reported that lncRNAs were widely involved in biological processes such as DNA methylation, histone modification, and chromatin remodeling (Rinn & Chang Citation2012) and regulated the expression of target genes at the transcriptional, post-transcriptional, and epigenetic levels (Chen & Carmichael Citation2010; Wierzbicki Citation2012; Bai et al. Citation2015). In this study, the results of GO enrichment showed that the lncRNAs were associated with energy metabolism and cell differentiation. Energy metabolism is the process of generating energy (ATP) from diet and closely related to organ development. In Holsteins, energy metabolism mainly depends on the composition of the diet of an individual. The development of the mammary gland in Holstein heifers was reported to be affected by different dietary metabolizable proteins, metabolizable energy ratios (Albino et al. Citation2015), and enhanced by high plane of nutrition (Vailati-Riboni et al. Citation2018). The enriched GO terms of cellular respiration, respiratory electron transport chain, and electron transport chain in the present study indicate that lncRNAs might regulate energy metabolism in the early growth stages of Holstein mammary gland. Cell proliferation and differentiation are also required for organ development, and recent studies have shown that lncRNAs such as H19 (Li et al. Citation2019), Neat1 (Standaert et al. Citation2014), and XIST (Ma et al. Citation2019) were positively involved in growth and proliferation of mammary epithelial cells. Functional prediction of lncRNAs in the present study showed that some biological processes such as cell development, mesenchymal cell development, and regulation of cell differentiation were significantly enriched, suggesting that lncRNAs might modulate mammary gland development by regulating cell differentiation in the early growth stage of Holstein. However, the exact functions of these lncRNAs further need more focused investigation to verify our observations.
Conclusion
In conclusion, lncRNAs in Holstein mammary gland were analyzed using bioinformatics analysis. A list of credible lncRNAs including novel ones was obtained via bioinformatics analysis and RT-PCR validation. Prediction of potential cis-regulated target gene revealed that lncRNAs might regulate mammary gland development by regulating energy metabolism and cell differentiation. Since mammary gland development was closely related to the milk production, lncRNAs identified in this study might also be the molecular markers underlying the trait of milk production. This study provides valuable insights and could be the basis for further investigations to determine the functions of lncRNAs in the mammary gland development in Holstein. It also generally contributes to a better understanding of Holstein mammary gland development.
supplementary_tables_pdf.zip
Download Zip (35.9 MB)Disclosure statement
No potential conflict of interest was reported by the authors.
Supplemental data
Supplemental data for this article can be accessed here.
Additional information
Funding
References
- Albino RL, Marcondes MI, Akers RM, Detmann E, Carvalho BC, Silva TE. 2015. Mammary gland development of dairy heifers fed diets containing increasing levels of metabolisable protein: Metabolisable energy. The Journal of Dairy Research 82:113–120. DOI: 10.1017/S0022029914000697.
- Bai Y, Dai X, Harrison AP, Chen M. 2015. RNA regulatory networks in animals and plants: A long noncoding RNA perspective. Briefings in Functional Genomics 14:91–101. DOI: 10.1093/bfgp/elu017.
- Batista PJ, Chang HY. 2013. Long noncoding RNAs: Cellular address codes in development and disease. Cell 152:1298–1307. DOI: 10.1016/j.cell.2013.02.012.
- Chen G, Shi T, Shi L. 2017. Characterizing and annotating the genome using RNA-seq data. Science China Life Sciences 60:116–125. DOI: 10.1007/s11427-015-0349-4.
- Chen LL, Carmichael GG. 2010. Decoding the function of nuclear long non-coding RNAs. Current Opinion in Cell Biology 22:357–364. DOI: 10.1016/j.ceb.2010.03.003.
- Chen LL, Yang L. 2015. Regulation of circRNA biogenesis. RNA Biology 12:381–388. DOI: 10.1080/15476286.2015.1020271.
- Derrien T, et al. 2012. The GENCODE v7 catalog of human long noncoding RNAs: Analysis of their gene structure, evolution, and expression. Genome Research 22:1775–1789. DOI: 10.1101/gr.132159.111.
- Doolittle WF. 2013. Is junk DNA bunk? A critique of ENCODE. Proceedings of the National Academy of Sciences 110:5294–5300. DOI: 10.1073/pnas.1221376110.
- Fang S, et al. 2018. NONCODEV5: A comprehensive annotation database for long non-coding RNAs. Nucleic Acids Research 46:D308–D314. DOI: 10.1093/nar/gkx1107.
- Finn RD, et al. 2014. Pfam: The protein families database. Nucleic Acids Research 42:222–230. DOI: 10.1093/nar/gkt1223.
- Fu L, Niu B, Zhu Z, Wu S, Li W. 2012. CD-HIT: Accelerated for clustering the next-generation sequencing data. Bioinformatics 28:3150–3152. DOI: 10.1093/bioinformatics/bts565.
- He H, et al. 2018. miR-148a-3p promotes rabbit preadipocyte differentiation by targeting PTEN. In Vitro Cellular & Developmental Biology - Animal 54:1–9. DOI: 10.1007/s11626-018-0232-z.
- Huang DW, Sherman BT, Lempicki RA. 2009. Systematic and integrative analysis of large gene lists using DAVID bioinformatics resources. Nature Protocols 4(1):44–57. DOI: 10.1038/nprot.2008.211.
- Ibeagha-Awemu EM, Li R, Dudemaine PL, Do DN, Bissonnette N. 2018. Transcriptome analysis of long non-coding RNA in the bovine mammary gland following dietary supplementation with linseed oil and safflower oil. International Journal of Molecular Sciences 19:E3610. DOI: 10.3390/ijms19113610.
- Kim D, Langmead B, Salzberg SL. 2015. HISAT: A fast spliced aligner with low memory requirements. Nature Methods 12:357–360. DOI: 10.1038/nmeth.3317.
- Kong L, Zhang Y, Ye ZQ, Liu XQ, Zhao SQ, Wei L, Gao G. 2007. CPC: Assess the protein-coding potential of transcripts using sequence features and support vector machine. Nucleic Acids Research 35:W345–349. DOI: 10.1093/nar/gkm391.
- Li H, Yu B, Li J, Su L, Yan M, Zhu Z, Liu B. 2014. Overexpression of lncRNA H19 enhances carcinogenesis and metastasis of gastric cancer. Oncotarget 5:2318–2329. DOI: 10.18632/oncotarget.1913.
- Li Q, Qiao J, Zhang Z, Shang X, Chu Z, Fu Y, Chu M. 2018. Identification and analysis of differentially expressed long non-coding RNAs of Chinese Holstein cattle responses to heat stress. Animal Biotechnology 31:9–16. DOI: 10.1080/10495398.2018.1521337.
- Li X, Wang H, Zhang Y, Zhang J, Qi S, Zhang Y, Gao MQ. 2019. Overexpression of lncRNA H19 changes basic characteristics and affects immune response of bovine mammary epithelial cells. PeerJ 7:e6715. DOI: 10.7717/peerj.6715.
- Ma M, Pei Y, Wang X, Feng J, Zhang Y, Gao MQ. 2019. LncRNA XIST mediates bovine mammary epithelial cell inflammatory response via NF-kappaB/NLRP3 inflammasome pathway. Cell Proliferation 52:e12525. DOI: 10.1111/cpr.12525.
- Macias H, Hinck L. 2012. Mammary gland development. Wiley Interdisciplinary Reviews Developmental Biology 1:533–557. DOI: 10.1002/wdev.35.
- Pertea M, Kim D, Pertea GM, Leek JT, Salzberg SL. 2016. Transcript-level expression analysis of RNA-seq experiments with HISAT, StringTie and Ballgown. Nature Protocols 11:1650–1667. DOI: 10.1038/nprot.2016.095.
- Quinlan AR. 2014. BEDTools: The Swiss-army tool for genome feature analysis. Current Protocols in Bioinformatics 47:1–34. DOI: 10.1002/0471250953.bi1112s47.
- Ren H, et al. 2016. Genome-wide analysis of long non-coding RNAs at early stage of skin pigmentation in goats (Capra hircus). BMC Genomics 17:67. DOI: 10.1186/s12864-016-2365-3.
- Rinn JL, Chang HY. 2012. Genome regulation by long noncoding RNAs. Annual Review of Biochemistry 81:145–166. DOI: 10.1146/annurev-biochem-051410-092902.
- Ruizorera J, Messeguer X, Subirana JA, Alba MM. 2014. Long non-coding RNAs as a source of new peptides. eLife 3:e03523. DOI: 10.7554/eLife.03523.
- Shannon P, et al. 2003. Cytoscape: A software environment for integrated models of biomolecular interaction networks. Genome Research 13:2498–2504. DOI: 10.1101/gr.1239303.
- Soberon F, Raffrenato E, Everett RW, Amburgh MEV. 2012. Preweaning milk replacer intake and effects on long-term productivity of dairy calves. Journal of Dairy Science 95:783–793. DOI: 10.3168/jds.2011-4391.
- Standaert L, et al. 2014. The long noncoding RNA Neat1 is required for mammary gland development and lactation. RNA 20:1844–1849. DOI: 10.1261/rna.047332.114.
- Stein LD. 2004. Human genome: End of the beginning. Nature 431:915–916. DOI: 10.1038/431915a.
- Sun L, et al. 2013. Utilizing sequence intrinsic composition to classify protein-coding and long non-coding transcripts. Nucleic Acids Research 41:e166. DOI: 10.1093/nar/gkt646.
- Tong C, Chen Q, Zhao L, Ma J, Ibeagha-Awemu EM, Zhao X. 2017. Identification and characterization of long intergenic noncoding RNAs in bovine mammary glands. BMC Genomics 18:468. DOI: 10.1186/s12864-017-3858-4.
- Trapnell C, et al. 2012. Differential gene and transcript expression analysis of RNA-seq experiments with TopHat and Cufflinks. Nature Protocols 7:562–578. DOI: 10.1038/nprot.2012.016.
- Vailati-Riboni M, Bucktrout RE, Zhan S, Geiger A, McCann JC, Akers RM, Loor JJ. 2018. Higher plane of nutrition pre-weaning enhances Holstein calf mammary gland development through alterations in the parenchyma and fat pad transcriptome. BMC Genomics 19:900. DOI: 10.1186/s12864-018-5303-8.
- Wang GZ, et al. 2018. Genome-wide identification and characterization of long non-coding RNAs during postnatal development of rabbit adipose tissue. Lipids in Health and Disease 17:271. DOI: 10.1186/s12944-018-0915-1.
- Wang L, Park HJ, Dasari S, Wang S, Kocher JP, Li W. 2013. CPAT: Coding-Potential Assessment Tool using an alignment-free logistic regression model. Nucleic Acids Research 41:e74. DOI: 10.1093/nar/gkt006.
- Wang Y, et al. 2016. Analyses of long non-coding RNA and mRNA profiling using RNA sequencing during the pre-implantation phases in pig endometrium. Scientific Reports 6:20238. DOI: 10.1038/srep20238.
- Wierzbicki AT. 2012. The role of long non-coding RNA in transcriptional gene silencing. Current Opinion in Plant Biology 15:517–522. DOI: 10.1016/j.pbi.2012.08.008.
- Yang B, Jiao B, Ge W, Zhang X, Wang S, Zhao H, Wang X. 2018. Transcriptome sequencing to detect the potential role of long non-coding RNAs in bovine mammary gland during the dry and lactation period. BMC Genomics 19:605. DOI: 10.1186/s12864-018-4974-5.
- Yun J, Jin H, Cao Y, Zhang L, Zhao Y, Jin X, Yu Y. 2018. RNA-seq analysis reveals a positive role of HTR2A in adipogenesis in Yan Yellow Cattle. International Journal of Molecular Sciences 19:1760. DOI: 10.3390/ijms19061760.