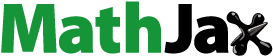
Our thanks to all the discussants for their enlightening comments, their excellent discussions of the broader context of BIC-type procedures and their many exciting possibilities for further investigation. We respond to each discussant below, generally omitting commenting on those aspects of the discussions that we agree with and to which we have nothing to add.
Response to Jiahua Chen and Zeny Feng
Chen and Feng reiterate the dangers of blindly applying BIC for model selection problems that do not conform to the regularity conditions that it was originally designed for. They propose a competing approximation of the Bayes Factor, denoted by aBIC, that retains extra terms in the Laplace approximation, terms which become negligible when the sample size n is very large. They show that this approximation gives a reasonable answer to Example 1.2 in the main paper. We agree that this strategy works in specific situations to deal with the effective sample size issue and has recently been exploited by Bollen, Ray, Zavisca, and Harden (Citation2012) and Bollen, Harden, Ray, and Zavisca (Citation2014) to develop model selection tools for selecting structural equation models.
While using as the effective sample size works in some examples, it is not a general solution since it depends on the model parameters and, hence, is not really a sample size. Consider the simple situation of a t-test, for instance. For a fixed value of t and fixed n, typical Bayesian analyses vary only by a term that depends on the prior.
, in this case, is a sum of terms that depend on the sample size, and also a term proportional to
, which can be arbitrarily large or small with high probability, depending on whether
is large or small.
PBIC, as with the original BIC, was designed for situations where each model receives the same prior weight. This is frequently not appropriate, especially in scenarios where there are many models of very differing dimensions, as illustrated by Chen and Feng in their discussion. Indeed, they point to early work on EBIC, which essentially brought prior probabilities into the picture, adding another term to BIC. The potential importance of doing this was also noted in the discussion of Peterson and Cavanaugh, and we will return to the discussion of EBIC there. Note, of course, that this introduction of prior probabilities can also be effected with PBIC.
Response to Bertrand Clarke
The name ‘unit information’ has historically come to mean the information contained in a single observation. Since is the information about
, which (in principle) is scaled by the sample size, using
as the unit information is reasonable.
The choice of prior is guided by three desiderata from the literature:
The prior should be centred at zero (or the appropriate null) and have the unit information as its scale parameter.
It should have Cauchy-like tails (a choice descended from Jeffreys).
It should result in a closed form expression, in order to be of comparable simplicity to BIC.
These desiderata essentially lead to the chosen prior.
The many interesting variants on BIC that are introduced in Section 2 of Clarke's Discussion are certainly worthy of study, but our rejoinder is not the appropriate place for that study. Whether the results of Section 5.5 hold when PBIC is replaced by (6) is, indeed, an interesting question.
The Section ‘Where to from Here’ is an interesting listing of possible approaches to tackle the (unsolved) general problem of defining ‘effective sample size '. It would be wonderful if one of these ideas solved the problem.
Response to Ruobin Gong and Minge Xie
As PBIC (or PBIC*) are approximations to a real Bayesian marginal likelihood, the Bayes factors of the null model to each alternative model can be reconstructed (as noted in the Discussion of Liu and Sun) and posterior model probabilities can then be constructed (given model prior probabilities) as noted in the Discussions of Chen and Feng and Peterson and Cavanaugh (both of which also suggest appropriate model prior probabilities). Thus one can attain a full Bayesian description of the model uncertainty.
But Gong and Xie are proposing more, namely to study the uncertainty in the choice of the prior distribution or (possibly) in the definition of the effective sample size when it is stochastic. These are certainly of interest but, as noted by the discussants themselves, would be difficult to achieve while retaining the computational simplicity of PBIC (and PBIC*).
Response to Jan Hannig
One of reasons we chose PBIC as the name is that almost all letters other than P – to preface BIC – had already been used; for instance Hannig's preferred CBIC has already been used in the literature (many publications) as ‘Conditional BIC’.
Not all of us are fans of local priors but, for those who are fans, it is very nice that Hannig was able to produce a ‘local’ version of PBIC.
We expected Hannig's discussion to be producing a ‘fiducial information criteria ', and hope FIC is yet to come!
Response to Jiming Jiang and Huan Nguyen
Jiang and Nguyen relate our work on PBIC to applications of information criteria more broadly defined in selection problems in mixed model analysis. They point out that, in the linear mixed model, not only is effective sample size an issue, but also counting the number of parameters becomes non-trivial, as we also discussed in Example 1.5. The mixed model is, indeed, a promising scenario for extension of these ideas.
Jiang and Nguyen ask if our definition of effective sample size has some general, intuitive explanation. The intuition for the linear model case is discussed, more generally, in Berger, Bayarri, and Pericchi (Citation2014). We have no intuition for the general case in Section 3.2, beyond that discussed therein.
The authors also rightly draw attention to the important idea of finite-sample performance of model selection criteria generally. We agree that this is highly relevant and this points towards opportunities for further research in studying such finite-sample performance of the PBIC and other information criteria. Jiang and Nguyen suggest to sidestep these issues by applying a different method which they developed and is called the fence method. Essentially the fence method is a two-step procedure where the most parsimonious model is chosen among a set of correct models. The set of ‘correct’ models is chosen by setting a fence, i.e., a threshold on the goodness-of-fit of the models under consideration, where in practice goodness-of-fit is taken to be . Given appropriate goodness-of-fit, parsimony is then used to select the best model, where parsimony is defined as model dimension. We fully agree that it only makes sense to select the best model among models that are in some sense good enough in capturing the data. Striking the balance between goodness-of-fit and parsimony is indeed what we are after, and we believe utilisation of effective sample size, through PBIC, can play an important role in finding that balance.
Response to Brunero Liseo
Using constant priors for the common parameters in the models under consideration is, indeed, restrictive. But allowing other priors would change the default nature of PBIC – a feature of BIC that we were trying to mimic. In the paper, we did say that the first step is to transform all parameters so that they reside in , mentioning that this was important for using the Laplace approximation; it is also important in making the use of a constant prior for common parameters more palatable.
Liseo asks if alternatives to the mixing prior in (9) are possible. The mixing prior, with Beta parameters a=0.5 and b=1, is precisely the distribution so that the prior closely matches a Cauchy prior and, at the same time, the marginal can be computed exactly. There are other priors in the class for which the marginal can be computed exactly but, because of the pioneering work of Jeffreys on this, matching a (unit information) Cauchy prior seemed most natural.
The more general definition of TESS in Section 3.2 can differ in important ways from the specific definition for Linear Models given in (14). For instance, in Example 5.2, TESS depends explicitly on , which does not result if the definition in Section 3.2 is used. On the other hand, they do coincide in Example 3.3. This lack of agreement of the general definition and the linear model definition is the reason we do not feel that the general definition is the final answer. Also, it is possible that an optimal general definition of effective sample size could depend on unknown parameters, but this would not be particularly useful. Solving this problem of a general definition would, as Liseo notes, open up the use of PBIC for very general situations, including dependent data and time series modelling, outside of the linear model.
Response to Sifan Liu and Dongchu Sun
We did, indeed, mean that PBIC could be used to compute an (approximate) Bayes factor via the formula . Thanks for writing this out; we seem to have neglected to explicitly give the formula in the paper. We agree with the other comments in the discussion also.
Response to Ryan Peterson and Joseph Cavanaugh
Adding in model prior probabilities, resulting in Equation (1) in the discussion, is certainly needed if the model prior probabilities are not equal. And the discussion of Peterson and Cavanaugh does a nice job of pointing out why equal model prior probabilities is not usually a good idea when the model space is large.
In selection from among P variables, for instance, the most common default prior recommended today gives equal weight of to each model size, with that mass divided up equally among all models of a given size. This is equivalent to the EBIC suggestion in the discussion, with
. It is also equivalent to use of the alternative suggestion
, if w is viewed as unknown and given a uniform prior. Additional discussion of these issues can be found in Scott and Berger (Citation2010).
Response to Jun Shao and Sheng Zhang
The idea of benchmarking the importance of variables has been used before, in situations where carrying out a traditional Bayesian variable selection analysis is difficult; see, e.g., Linkletter, Bingham, Hengartner, Higdon, and Ye (Citation2006). Our view is that this is likely to be most useful when one wants to judge – at the same time – if a variable has ‘no or minor effect '. In traditional Bayesian model uncertainty analysis, this requires two steps: finding the posterior probability that the variable has no effect and looking at the posterior distribution of effect size, given that the variable has an effect. We still find it useful to do the more complex traditional analysis, but Shao and Zhang do a nice job of developing and illustrating the benchmarking approach.
References
- Berger, J., Bayarri, M. J., & Pericchi, L. R. (2014). The effective sample size. Econometric Reviews, 33, 197–217. doi: 10.1080/07474938.2013.807157
- Bollen, K. A., Harden, J. J., Ray, S., & Zavisca, J. (2014). BIC and alternative Bayesian information criteria. Selection of Structural Equation Models: A Multidisciplinary Journal, 21(1), 1–19. doi: 10.1080/10705511.2014.856691
- Bollen, K. A., Ray, S., Zavisca, J., & Harden, J. J. (2012). A comparison of Bayes factor approximation methods including two new methods. Sociological Methods and Research, 41, 294–324. doi: 10.1177/0049124112452393
- Linkletter, C., Bingham, D., Hengartner, N., Higdon, D., & Ye, K. Q. (2006). Variable selection for Gaussian process models in computer experiments. Technometrics, 48, 478–490. doi: 10.1198/004017006000000228
- Scott, J. G., & Berger, J. O. (2010). Bayes and empirical-Bayes multiplicity adjustment in the variable-selection problem. The Annals of Statistics, 38, 2587–2619. doi: 10.1214/10-AOS792