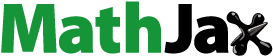
Abstract
Suppose that we observe , where
is an unknown vector with unknown precision τ. Estimating the regression coefficient
with known τ has been well studied. However, statistical properties such as admissibility in estimating
with unknown τ are not well studied. Han [(2009). Topics in shrinkage estimation and in causal inference (PhD thesis). Warton School, University of Pennsylvania] appears to be the first to consider the problem, developing sufficient conditions for the admissibility of estimating means of multivariate normal distributions with unknown variance. We generalise the sufficient conditions for admissibility and apply these results to the normal linear regression model. 2-level and 3-level hierarchical models with unknown precision τ are investigated when a standard class of hierarchical priors leads to admissible estimators of
under the normalised squared error loss. One reason to consider this problem is the importance of admissibility in the hierarchical prior selection, and we expect that our study could be helpful in providing some reference for choosing hierarchical priors.
1. Introduction
Consider a multivariate normal model,
(1)
(1) where
is a
observation vector,
is a p-dimensional vector of unknown parameters, and
is the unknown precision. Statistical properties such as admissibility for estimating
can be dated back to James-Stein (Citation1961) when the error variance is known, while the admissibility of generalisation of James-Stein estimator of
with unknown parameter τ was studied in Judge, Yancey, and Bock (Citation1983), Fraisse, Raoult, Robert, and Roy (Citation1990), Robert (Citation2007) and so on. For estimating
with the unknown nuisance parameter τ in the model (Equation1
(1)
(1) ), some authors, such as Strawderman (Citation1973), Maruyama and Strawderman (Citation2005) and Willing and Zhou (Citation2008) studied the minimaxity of Bayesian estimators of
under hierarchical priors. The admissibility of a generalised Bayesian estimator of
under a class of noninformative priors was recently studied in Han (Citation2009). With additional independent observation
Han (Citation2009) found a set of sufficient conditions for the joint priors of
, so that the generalised Bayesian estimator of
is admissible under the squared error loss. In practice, we often need to consider a normal linear regression model,
(2)
(2)
where is
design matrix with full column rank p,
It is of great interest to study the admissibility in estimating the unknown regression coefficients
with unknown τ in the normal linear regression model (Equation2
(2)
(2) ).
Several authors have described admissibility as a powerful tool for selecting satisfactory hierarchical generalised Bayesian priors. For example, Berger, Strawderman, and Tang (Citation2005) pointed out that the ‘use of objective improper priors in hierarchical modelling is of enormous practical importance, yet little is known about which such priors are good or bad. It is important that the prior distribution not be too diffuse, and study of admissibility is the most powerful tool known for detecting an over-diffuse prior’. For known precision or error variance, Brown (Citation1971) provided the necessary and sufficient condition of the admissible Bayes estimators under quadratic loss, based on a Markovian representation of the estimation problem. Recent papers related to the theoretical studies of the admissibility of estimators of can be found in Berger and Strawderman (Citation1996), Berger et al. (Citation2005), Berger, Sun, and Song (Citation2018), and so on.
However, most of the literature focussed on models of which variances are given, yet in practical problems, the precision or variance is often unknown. For the admissibility in the model (Equation2(2)
(2) ), to the best of our knowledge, very few results have been obtained because of the technical difficulty. The fundamental tool for proving admissibility for unknown precision is Blyth's method (Blyth, Citation1951), which proposed a sufficient admissibility condition, relating admissibility of an estimator with the existence of a sequence of prior distributions approximating this estimator. Based on Blyth's results, Han's (Citation2009) found sufficient conditions for the joint priors of
for model (Equation1
(1)
(1) ). Sometimes, those sufficient conditions are strict and difficult to satisfy. We will generalise the sufficient conditions for admissibility and apply these results to the normal linear regression model (Equation2
(2)
(2) ). Using the generalised conditions, a 2-level and 3-level hierarchical models with unknown precision τ are investigated when a standard class of hierarchical priors leads to admissible estimators of
under the normalised squared error loss. One motivation to consider this problem is the importance of admissibility in the hierarchical prior selection, and we expect that our study could be helpful to provide some reference for choosing hierarchical priors.
The paper is organised as follows. In Section 2, we introduce the sufficient conditions for admissibility of the generalised Bayesian estimators of for the model (Equation1
(1)
(1) ), which is studied by Han (Citation2009). In Section 3, we generalise the sufficient conditions for admissibility and apply these results to the normal linear regression model (Equation2
(2)
(2) ). 2-level and a 3-level hierarchical models with unknown precision τ are investigated in Sections 4 and 5, determining when a standard class of hierarchical priors leads to admissible estimators of
under the normalised squared error loss. Finally, some comments are made in Section 6.
2. Han's (Citation2009) results for (1)
Recall the model (Equation1(1)
(1) ) considered in Han (Citation2009), i.e.
where
and w are independent of each other. Let
denote an estimator of
. Correspondingly, the squared error loss function of
becomes
(3)
(3) Han (Citation2009) studied a class of prior density for
with assumption
(4)
(4) Consequently, the generalised Bayes estimator for the normal mean
is the posterior mean of
, given by
(5)
(5) where
Let
be the marginal likelihood function of
with the form
From Brown (Citation1971), the generalised Bayes estimator in (Equation5
(5)
(5) ) can be expressed as
(6)
(6) where ∇ denotes the gradient. Let S denote the ball of radius 1 at the origin in
and
be the complement of S, defined
. For the hierarchical Bayes model (Equation1
(1)
(1) ), Han (Citation2009) studied the admissible generalised Bayes estimators
under the following sufficient conditions.
Condition 1.
;
Condition 2.
;
Condition 3.
;
Condition 4.
;
Condition 5. For any positive constant B,
;
Condition 6. Define two sequences of functions
(7)
(7) Write
and
There is a constant C>0, such that
Theorem 2.1
Han, Citation2009
Consider the model (Equation1(1)
(1) ) with the prior densities
and
satisfying Conditions 1–6. If
is decreasing with respect to
the corresponding generalised Bayes estimator (Equation6
(6)
(6) ) for
is admissible under the squared error loss function (Equation3
(3)
(3) ).
3. Main results for (2)
We are primarily interested in the normal linear regression model (Equation2(2)
(2) ). For the model (Equation2
(2)
(2) ), we let
denote the least squared estimators of
, and
be the usual residual sum squares errors (SSE). Then
(8)
(8) independently. Here we obtain w automatically with m=n−p. For the model (Equation2
(2)
(2) ), consider the normalised squared error loss function of
given by
(9)
(9) The corresponding risk function of
is
(10)
(10) An estimator
is inadmissible if there exists another estimator whose risk function is nowhere bigger and somewhere smaller. If no such better estimator exists,
is admissible.
For the model (Equation2(2)
(2) ), to obtain the admissible estimator of
under the normalised squared error loss (Equation9
(9)
(9) ), we define
(11)
(11) where
is a
matrix such that
.
Lemma 3.1
For the model (Equation1(1)
(1) ), assume the estimator
in (Equation6
(6)
(6) ) is admissible under the loss function (Equation3
(3)
(3) ). Then the estimator
in (Equation11
(11)
(11) ) is admissible under the normalised squared error loss (Equation9
(9)
(9) ) for the model (Equation2
(2)
(2) ).
Proof.
Note that the model (Equation2(2)
(2) ) is equivalent to (Equation8
(8)
(8) ). It yields that
(12)
(12) It follows from the admissibility of
under the model (Equation1
(1)
(1) ) that the estimator
for
is admissible under the loss function
The proof of this lemma is completed.
Combining Theorem 1 with Lemma 1, we can reach the following theorem.
Theorem 3.2
For the model (Equation2(2)
(2) ) with the prior densities
and
satisfying Conditions 1–6, suppose that
is decreasing with respect to
then
defined in (Equation11
(11)
(11) ) is the admissible estimator of
under the normalised squared error loss (Equation9
(9)
(9) ).
Theorem 2.1 applies to the case where is spherically symmetric of
and decreases in
. As discussed in Han (Citation2009), this requirement is not unique and can be replaced by the following condition.
Condition 7. Denote
(13)
(13)
(14)
(14)
(15)
(15)
(16)
(16) We have
(17)
(17)
(18)
(18)
As an immediate corollary, we have the following result.
Theorem 3.3
For the model (Equation2(2)
(2) ), assume that the prior densities
and
satisfy Conditions 1–7. Then estimator
in (Equation11
(11)
(11) ) for
is admissible under the normalised squared error loss (Equation9
(9)
(9) ).
It might be difficult to show that the is a decreasing function of
. Interestingly, this requirement can be relaxed to the requirement that
is a decreasing function of its component,
, for
.
Lemma 3.4
For the model (Equation1(1)
(1) ) with given τ,
is a decreasing function of
for
then Condition 7 holds.
Proof.
For any given , there is an orthogonal matrix
, such that
Without loss of generality, we can transform the coordinate system of
such that
Then,
It is easy to verify that the ith coordinate of
is
(19)
(19) Since
is a function of
,
is an odd function for
when
. It yields
. Therefore,
and
.
Let be the joint density of
, i.e.
Using (Equation19
(19)
(19) ), we get
(20)
(20) By the Divergence Theorem (Katz, Citation2005),
(21)
(21) where
is the boundary of S. Combining (Equation20
(20)
(20) ) and (Equation21
(21)
(21) ), we get
Then, we have
Clearly,
Since
can be written by
and
is symmetric about
, then
Therefore,
Since
is an even function for
and decreasing in
, then
. Therefore, we have
. With the same argument as above, we have
for any
.
Consequently, we obtain the following result.
Theorem 3.5
For the model (Equation2(2)
(2) ), assume that the prior densities
and
satisfy Conditions 1–6. If for any given
is decreasing in
. the estimator
in (Equation11
(11)
(11) ) for
is admissible under the normalised squared error loss (Equation9
(9)
(9) ).
Sometimes, is not strictly a decreasing function of its component,
, for
, but it could be a decreasing function of the components of some given orthogonal transformation. The following lemma shows that such cases also work.
Lemma 3.6
Consider the model (Equation1(1)
(1) ). Suppose there is an orthogonal matrix
such that
and
is a decreasing function of
for
then Condition 7 holds.
Proof.
It is easy to verify that the Jacobian of the transformation is
, and
. Note that
where
is the normal density function of
with mean
and variance
, and
. Similarly, we have
Since
s a decreasing function of
, for
from Lemma 3.4, for any
and w, we have
i.e.
. With the same argument as above, we have
for any
.
Accordingly, we get the following result.
Theorem 3.7
For the model (Equation2(2)
(2) ), assume that the prior densities
and
satisfy Conditions 1-6. If there is an orthogonal matrix
such that
and
is a decreasing function of
for
the estimator
in (Equation11
(11)
(11) ) for
is admissible under the normalised squared error loss (Equation9
(9)
(9) ).
In the next two sections, we will apply the above results to a 2-level and a 3-level hierarchical model, with unknown variance and a standard class of hierarchical priors.
4. Admissibility for a 2-level hierarchical model
4.1. g-Prior
For the model (Equation2(2)
(2) ), we consider the following class of hierarchical prior for
,
(22)
(22) where
. Zellner (Citation1986) proposed this form of the conjugate Normal-Gamma family with k=1. Many authors followed his work, for example, Eaton (Citation1989), Berger, Pericchi, and Varshavsky (Citation1998), Liang, Paulo, Molina, Clyde, and Berger (Citation2008) and Bayarri, Berger, Forte, and Garcła-Donato (Citation2012). From the perspective of model selection, g acts as a dimensionality penalty (Liang et al., Citation2008). For the choice of g, we study two cases:
Case 1. g is a known positive constant.
Recommendations for g have included the following: Kass & Wasserman's (Citation1995) unit information prior (g=n), Foster & George's (Citation1994) risk inflation criterion (), Fernández, Ley, & Steel's (Citation2001) benchmark prior (
) and so on.
Case 2. g is an unknown parameter, and the prior of g is .
By integrating out the latent variable g, one can get the conditional prior of given
,
(23)
(23) which can be represented as a mixture of g priors.
For Case 2, some priors have been previously considered. Here are two examples.
Example 4.1
, i.e.
(24)
(24) As discussed by Berger and Strawderman (Citation1996), it results in the multivariate t-prior for
given
, namely
(25)
(25) Zellner-Siow (Citation1980) studied the multivariate cauchy prior for
, which is one special case of (Equation25
(25)
(25) ) with
and
.
Example 4.2
Robust prior (Bayarri et al., Citation2012):
(26)
(26)
where ,
, and
. The prior (Equation26
(26)
(26) ) has its origins in the robust prior introduced by Strawderman (Citation1971), Berger (Citation1980) and Berger (Citation1985). As Bayarri et al. (Citation2012) discussed, the priors proposed by Liang et al. (Citation2008) are particular cases with
(the hyper-g prior) and
(the hyper-g/n prior). The prior in Cui and George (Citation2008) has
.
For the robust prior (Equation26(26)
(26) ), it is not straightforward to obtain the closed form of the marginal conditional prior for
given τ. Alternatively, we attempt to get the boundary of the marginal density of
given τ.
Lemma 4.3
Define
(27)
(27) where
and
then there are two positive constants
and
such that
for any u>0.
The proof is given in the Appendix. Applying this lemma to (Equation23(23)
(23) ), the resulting prior for
given τ for robust prior (Equation26
(26)
(26) ) with
has the boundary
(28)
(28) where p>2.
4.2. Admissibility
We apply the results in Section 3 to determine when the hierarchical priors (Equation22(22)
(22) ) result in admissible estimators of
under the normalised squared error loss (Equation9
(9)
(9) ).
Theorem 4.4
Case
For the model (Equation2
(2)
(2) ) under the hierarchical prior (Equation22
(22)
(22) ) with a given g. If
the estimator
in (Equation11
(11)
(11) ) for
is admissible under the normalised squared error loss (Equation9
(9)
(9) ).
The proof of Theorem 4.4 is similar to the proof of Theorem 4.5 later, thus it is omitted. As discussed by George and Foster (Citation2000), the choice of g effectively controls model selection, with large g typically concentrating the prior on parsimonious models with a few large coefficients, whereas small g tends to concentrate the prior on saturated models with small coefficients. Herein, we consider Case 1 from the perspective of admissibility, not the model selection. From Theorem 4.4, the choice of fix g has no effect on the admissibility of estimators of
.
Next, we consider Case 2. The prior density of g satisfies the following conditions:
Condition A1.
is a continuous function in
;
Condition A2.
,
, as
;
Condition A3.
,
, as
for some constant C>0.
Clearly, two examples of discussed in Section 4.1 satisfy Condition A1–A3 with appropriate a and b.
Theorem 4.5
Case
For the model (Equation2
(2)
(2) ) with the hierarchical prior (Equation22
(22)
(22) ), assume
satisfies Condition A1–A3. If
a>k−1 and
the estimator
in (Equation11
(11)
(11) ) for
is admissible under the normalised squared error loss (Equation9
(9)
(9) ).
Proof.
It is convenient to write , where
is the matrix of eigenvectors corresponding to
with
. Define
. From (Equation23
(23)
(23) ), the conditional prior of
given
is
which is a decreasing function of
, for
. From Theorem 3.7, we just need to verify Condition 1–6. For Condition 1,
If
, there is a positive constant C, such that
By polar coordinate transformation
, the integration over
becomes
which is finite if
, i.e.
Since
satisfies Condition A1-A3, there are some postive constants
,
and
such that
which is finite if a>k−1, and k+b>1.
For Condition 2,
which is finite if
, a>k−2 and k+b>2.
For Condition 3,
which is finite if
, a>k−3 and k+b>3.
For Condition 4, note that
By Cauchy-Schwarz inequality, it yields
Therefore,
which is finite if
, a>k−1, and k+b>1.
For Condition 5,
By polar coordinate transformation
, the integration over
becomes
Therefore,
which is finite if
, a>−1, and b>1.
Combining these restrictions, we can find that when , a>k−1 and k+b>3, Conditions 1–5 hold. As Han (Citation2009) discussed, Condition 6 is very mild. Proceeding in an analogous way on page 47 of Han (Citation2009), Condition 6 holds. By Theorem 3.7, the estimator
in (Equation11
(11)
(11) ) for
is admissible.
We are also interested in admissible estimators under Inv-Gamma and robust prior for g. Using Theorem 4.5, we have the following results.
Theorem 4.6
For the model (Equation2(2)
(2) ) with the hierarchical prior (Equation22
(22)
(22) ), assume
is
. If
and
the estimator
in (Equation11
(11)
(11) ) for
is admissible.
Proof.
By Theorem 4.5 with any constant a>k−1 and b=v+1, the result holds.
Theorem 4.7
For the model (Equation2(2)
(2) ) with the hierarchical prior (Equation22
(22)
(22) ), assume
is robust prior (Equation26
(26)
(26) ). If
and
the estimator
in (Equation11
(11)
(11) ) for
is admissible.
Proof.
From Theorem 4.5 with a=0 and , the proof is completed.
5. Admissibility for a 3-level hierarchical model
We also study a 3-level hierarchical model and determine which elements of the hierarchical prior class lead to admissible estimators of the under normalised squared error loss.
5.1. The model and priors
Consider the following 3-level hierarchical model
(29)
(29) where
is a given
matrix with full rank s,
is the
unknown vector,
and
are a
and
known covariate matrix, respectively, and λ is an unknown hyperparameter. To simplify the computation, without loss of generality, we set
and
.
Assume , and the prior of g satisfies the Condition A1–A3. The prior
satisfies the following conditions.
Condition B1.
is a continuous function in
;
Condition B2.
,
, as
;
Condition B3.
,
as
for some constant C>0.
5.2. Admissibility
The following lemma is needed.
Lemma 5.1
For the 3-level hierarchical model (Equation29(29)
(29) ), assume
satisfies Condition A1–A3, and
satisfies Condition B1–B3. Then
(30)
(30)
Proof.
Note that
Define
we have
Then the marginal distribution of θ given τ,
which is proportional to (Equation30
(30)
(30) ). The proof is completed.
Theorem 5.2
For the 3-level hierarchical model (Equation29(29)
(29) ), assume
satisfies Condition A1–A3, and
satisfies Condition B1–B3. Then, the estimator
in (Equation11
(11)
(11) ) for
is admissible if
and one of the following conditions holds,
.
Proof.
It is convenient to write , where
is the matrix of eigenvectors corresponding to
with
. Herein, we denote
. Therefore, from Lemma 5.1,
which is a decreasing function of
, for
. In addition, there are two positive constant
and
, such that
For the technical reasons, we first consider Condition 2. Note that
The integration over
is finite if k<1. For simplicity, denote
and
. If
, we have l>0.
Note that
(31)
(31) Clearly,
which is finite if a>h−1 and
. Clearly,
which is finite if b>1−h+l and
. Similarly, it is easy to verify that
is finite if a>h−1, b>1−h+l,
and
. Therefore, (Equation31
(31)
(31) ) is finite if
and
.
Similarly, for Condition 3,
(32)
(32) where
is a postive constant.
Clearly, if
. As in the proof of Condition 2, (Equation32
(32)
(32) ) is finite if
,
and
.
For Condition 4, from Lemma 5.1, note that
We will consider two cases, i.e. p>s and
respectively. If p>s,
. It yields
In the second step, we apply the Cauchy-Schwartz inequality. Therefore,
(33)
(33) As in the proof of Condition 3, (Equation33
(33)
(33) ) is finite if
, b>1−k,
and
.
If p=s, there is a positive constant , such that
Therefore, using the Cauchy-Schwartz inequality,
where
. Thus,
(34)
(34) where
is a positive constant. Note that the integration over
is finite if
.
If
which is finite if
and
Meanwhile,
which is finite if
and
.
For Condition 5, note that
By polar coordinate transformation
, the integration over
becomes
where
is a positive constant. Therefore,
(35)
(35) which is finite if
,
and
.
Combining the above results, Conditions 2–5 hold if satisfy the conditions as this theorem states. For Condition 1,
(36)
(36) where
is a positive constant. If
(Equation36
(36)
(36) ) can be proceeded as (Equation34
(34)
(34) ). If
it is easy to verify that (Equation36
(36)
(36) ) is finite if
,
Proceeding in an analogous way on page 47 of Han (Citation2009), Condition 6 also holds. By Theorem 3.7, estimator (Equation11
(11)
(11) ) for
are admissible.
6. Comments
In Section 2, we listed the sufficient conditions for admissibility of the estimators of with unknown τ, which was developed by Han (Citation2009). In Section 3, we generalise the sufficient conditions for admissibility and apply these results to the normal linear regression model (Equation2
(2)
(2) ). We have to admit that those sufficient conditions are still not optimal enough. Sometimes, we can't obtain satisfactory results utilising the conditions directly. In our paper, we consider
for the prior of τ. The condition of k for admissibility is
Unfortunately, we can't prove the admissibility for the boundary point
which is of great interest since it is the natural extension of Stein's harmonic prior (Stein, Citation1981) to the unknown variance problem. In follow-up work, we will try to explore the more powerful sufficient conditions for admissibility of the estimators of
with unknown τ. One promising method for this problem may be by Blyth's method (Blyth, Citation1951), discovering an appropriate sequence of finite measures.
Disclosure statement
No potential conflict of interest was reported by the authors.
Additional information
Funding
Notes on contributors
Chengyuan Song
Chengyuan Song is a PhD candidate in the College of Statistics, East China Normal University, Shanghai, China. His research interests include Bayesian statistics.
Dongchu Sun
Dr. Dongchu Sun received his PhD. in 1991 from Department of Statistics, Purdue University, under the guidence of Professor James O. Berger.
References
- Bayarri, M. J., Berger, J. O., Forte, A., & Garcła-Donato, G. (2012). Criteria for Bayesian model choice with application to variable selection. The Annals of Statistics, 40, 1550–1577. doi: 10.1214/12-AOS1013
- Berger, J. O. (1980). A robust generalized Bayes estimator and confidence region for a multivariate normal mean. The Annals of Statistics, 8, 716–761. doi: 10.1214/aos/1176345068
- Berger, J. O. (1985). Statistical decision theory and Bayesian analysis (2nd ed.). New York: Springer-Verlag Inc.
- Berger, J. O., Pericchi, L. R., & Varshavsky, J. A. (1998). Bayes factors and marginal distributions in invariant situations. Sankhya, Series A, Indian Journal of Statistics, 60, 307–321.
- Berger, J. O., & Strawderman, W. E. (1996). Choice of hierarchical priors: Admissibility in estimation of normal means. The Annals of Statistics, 24, 931–951. doi: 10.1214/aos/1032526950
- Berger, J. O., Strawderman, W., & Tang, D. (2005). Posterior propriety and admissibility of hyperpriors in normal hierarchical models. The Annals of Statistics, 33, 606–646. doi: 10.1214/009053605000000075
- Berger, J. O., Sun, D., & Song, C. (2018). An objective prior for hyperparameters in normal hierarchical models. Submitted.
- Blyth, C. (1951). On minimax statistical decision procedures and their admissibility. The Annals of Mathematical Statistics, 22, 22–42. doi: 10.1214/aoms/1177729690
- Brown, L. D. (1971). Admissible estimators, recurrent diffusions, and insoluble boundary value problems. The Annals of Mathematical Statistics, 42, 855–903. doi: 10.1214/aoms/1177693318
- Cui, W., & George, E. I. (2008). Empirical Bayes vs. fully Bayes variable selection. Journal of Statistical Planning and Inference, 138, 888–900. doi: 10.1016/j.jspi.2007.02.011
- Eaton, M. L. (1989). Group invariance applications in statistics. Institute of Mathematical Statistics, 1, 1–133.
- Fernández, C., Ley, E., & Steel, M. F. J. (2001). Benchmark priors for Bayesian model averaging. Journal of Econometrics, 100, 381–427. doi: 10.1016/S0304-4076(00)00076-2
- Foster, D. P., & George, E. I. (1994). The risk inflation criterion for multiple regression. The Annals of Statistics, 22, 1947–1975. doi: 10.1214/aos/1176325766
- Fraisse, A., Raoult, J., Robert, C., & Roy, M. (1990). Une condition nécessaire d'admissibilité et ses conséquences sur les estimateurs a˙ rétrécisseur de la moyenne d'un vecteur normal. Canadian Journal of Statistics, 18, 213–220. doi: 10.2307/3315452
- George, E. I., & Foster, D. P. (2000). Calibration and empirical Bayes variable selection. Biometrika, 87, 731–747. doi: 10.1093/biomet/87.4.731
- Han, X. (2009). Topics in shrinkage estimation and in causal inference (PhD thesis). Warton School, University of Pennsylvania.
- James, W., & Stein, C. (1961). Estimation with quadratic loss.Proceedings Fourth Berkeley Symposium in Mathematical Statistics and Probability (Vol. 1, pp. 361–3679).
- Judge, G., Yancey, T., & Bock, M. (1983). Pre-test estimation under squared error loss. Economics Letters, 11, 347–352. doi: 10.1016/0165-1765(83)90028-9
- Kass, R. E., & Wasserman, L. (1995). A reference Bayesian test for nested hypotheses and its relationship to the Schwarz criterion. Journal of the American Statistical Association, 90, 928–934. doi: 10.1080/01621459.1995.10476592
- Katz, V. J. (2005). The history of Stokes' theorem. Mathematics Magazine, 52, 146–156. doi: 10.1080/0025570X.1979.11976770
- Liang, F., Paulo, R., Molina, G., Clyde, M., & Berger, J. O. (2008). Mixtures of g priors for Bayesian variable selection. Journal of the American Statistical Association, 103, 410–423. doi: 10.1198/016214507000001337
- Maruyama, Y., & Strawderman, W. (2005). A new class of generalized Bayes minimax ridge regression estimators. The Annals of Statistics, 33, 1753–1770. doi: 10.1214/009053605000000327
- Robert, C. (2007). The Bayesian choice: From decision-theoretic foundations to computational implementation. New York: Springer.
- Stein, C. (1981). Estimation of the mean of a multivariate normal distribution. The Annals of Statistics, 9, 1135–1151. doi: 10.1214/aos/1176345632
- Strawderman, W. (1971). Proper Bayes minimax estimators of the multivariate normal mean. The Annals of Mathematical Statistics, 42, 385–388. doi: 10.1214/aoms/1177693528
- Strawderman, W. E. (1973). Proper Bayes minimax estimators of the multivariate normal mean vector for the case of common unknown variances. The Annals of Statistics, 1, 1189–1194. doi: 10.1214/aos/1176342567
- Willing, R., & Zhou, G. (2008). Generalized Bayes minimax estimators of the mean of multivariate normal distribution with unknown variance. Journal of Multivariate Analysis, 99, 2208–2220. doi: 10.1016/j.jmva.2008.02.016
- Zellner, A. (1986). On assessing prior distributions and Bayesian regression analysis with g-prior distributions. In: Goel, P. and Zellner, A., Eds., Bayesian Inference and Decision Techniques: Essays in Honor of Bruno de Finetti (pp. 233–243). New York: Elsevier Science Publishers, Inc.
- Zellner, A., & Siow, A. (1980). Posterior odds ratios for selected regression hypotheses. In J. M. Bernardo, M. H. DeGroot, D. V. Lindley, & A. F. M. Smith (Eds.), Bayesian statistics (pp. 585–603). Valencia: University Press.
Appendix
A.1. Proof of Lemma 4.3
To simplify the computation, without loss of generality, we set c=1. Let , then
One just needs to consider
(A1)
(A1)
The integral can be written by
For the lower bound of
and
, we have
where
and
. For the upper bound of
and
, we have
where
and
. Therefore, let
and
. We get (EquationA1
(A1)
(A1) ). The lemma is proved.