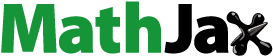
Abstract
In this thesis, we establish non-linear wavelet density estimators and studying the asymptotic properties of the estimators with data missing at random when covariates are present. The outstanding advantage of non-linear wavelet method is estimating the unsoothed functions, however, the classical kernel estimation cannot do this work. At the same time, we study the larger sample properties of the ISE for hazard rate estimator.
AMS 2000 Subject Classifications:
Fields such as finance, insurance and geology are full of random phenomena. We deal with these problems based on a large amount of statistical data and analyse the development law of the variables we care about. The density function and hazard rate function can fully reflect the distribution law of samples. The asymptotic properties of density estimator and hazard rate estimator are important research topics in Statistics. There are many literatures on the estimation of density function and hazard rate function under complete data. However, in many practical applications, incomplete data are encountered due to various causes, such as right-censored and/or left-truncated data as well as missing data. For example, in questionnaire survey or interview, loss of questionnaire or non-response will lead to data loss. When there are missing data, standard estimation methods cannot be applied directly, specially, for the unknown function with finite discontinuous. In this thesis, we employ nonlinear wavelet estimation method to deal with this puzzle. The major advantage of the wavelet method is its adaptability (in the minimax sense) to the degree of smoothness of the underlying unknown cure.
The random variables with a continuous distribution function (df) F and density function f. For density function
, we have the following wavelet expression:
(1)
(1) where
and
are the wavelet coefficients of f, and the system
is an orthonormal basis for the space
. The proposed nonlinear wavelet estimated of f is defined by
(2)
(2) where
is a threshold,
is a truncation parameter,
and
are estimators of wavelet coefficients
and
, respectively, with the estimator
of F.
A popular stochastic measure of the distance between any unknown function f and its estimator is the integral square error (ISE), which is often used to study the performance of the estimator, defined by
and the mean integral squared error (MISE), i.e.,
where
is a weight function.
The MISE of kernel estimator for density function is as follows:
(3)
(3) where n denotes sample size,
is bandwidth of the kernel estimator, r is order of kernel,
and
are constants depending on both the kernel and density function. The MISE expansion for kernel estimator generally fails if f does not have r derivatives. However, the asymptotic MISE for non-linear wavelet estimator is the same in both smooth and unsmooth density cases, a fact that is not true for the kernel method.
It is worth pointing out that there is no result available in the literature for non-linear wavelet density estimation with missing data. Based on the widespread existence of missing data and the advantage of non-linear wavelet method, we consider the asymptotic properties and finite sample performance of non-linear estimation and hazard rate estimation with missing data. In regression analysis, data missing is often divided into two cases: missing response variables and missing covariables. At the same time, there are three types of data missing mechanisms: missing completely at random, missing at random (MAR) and missing at non-random. In this paper, we focus on the situation of responses missing at random when covariables are present. Our contribution includes the following aspects.
In Chapter 1, we mainly introduce the research background, research content and innovation points, and review the large sample properties for nonlinear wavelet estimators of density function and kernel estimators of hazard rate function under complete data and incomplete data in a large number of literatures.
In Chapter 2, we for the first time establish the asymptotic expansion of the non-linear wavelet density estimator with data MAR when covariates are present. Under some assumptions, we discuss the asymptotic expansion for MISE of non-linear wavelet density estimator and prove the asymptotic expansion of MISE is still true for the unknown density estimator with finite discontinuous points. In addition, we discuss the asymptotic normality of the non-linear wavelet density estimator.
In Chapter 3, based on the definition of the estimator of df F in Wang and Qin (2009), we construct the non-linear wavelet density estimator with missing at random when covariates are present, which is different with the estimator In Chapter 2. We prove the uniform convergence rate of global
error for estimator in Besove space, which contains unsmoothed functions. Also, we establish data simulation to investigate average mean square error (AMSE) of the estimator with different missing rates. It can be seen that the estimator performs better as sample size increasing and/or as missing rate decreasing.
In Chapter 4, we first employ calibration, imputation and inverse probability weighting method and propose three kinds of non-linear wavelet estimators of lifetime density function with censoring indicator of right-censored data MAR. The asymptotic normality of estimators and asymptotic expansion of MISE for the estimator are proved. At the same time, we confirm that the asymptotic expansion of MISE for the estimator with finite discontinuous points still holds. In addition, we consider the influence of censoring rate, missing rate and sample size on the estimators through simulation analysis, and observe the asymptotic normality of the estimators from the Q–Q plots. The conclusion is that the performance for calibration estimator is best and the performance for inverse probability weighting estimator is worst. Moreover, the quality of fitting for the estimators gets better as increasing of the sample size and the results get better as decreasing of censoring rate and missing rate.
In Chapter 5, we establish the kernel estimator of hazard rate function for the lifetime with censoring indicator of right-censored data MAR, and first study the asymptotic normality for ISE of the estimator and show the asymptotic expansion of MISE for the estimator, which improve the related results with data MAR. From one simulation, we analyse the AMSE of the estimator with different missing rate and censoring rate, and analyse the finite sample performance of the estimator from average curves. It is easy to see that the AMSE of estimator decreases with the increasing of sample size and increases with the increasing of missing rate and censoring rate, as well the average cure of the estimator performs better as a larger sample size.
The innovations of this thesis are described as follows. Firstly, we extend the asymptotic properties of the non-linear wavelet density estimators with complete data to data MAR. We discuss the asymptotic expansion of MISE and the asymptotic normality for the estimators as well as the uniform convergence rate of error in Besov space including discontinuous functions. Secondly, we expand the related results of non-linear wavelet density estimators with data missing random to censoring indicator of right-censored data MAR. We confirm that the asymptotic expansion of MISE still holds for the unknown density estimator with finite discontinuous points. Thirdly, we improve the asymptotic properties of hazard rate estimators under complete data or right-censored data to censoring indicator of right-censored data MAR. Consequently, the asymptotic normality for ISE and the asymptotic expansion for MISE of the estimator are verified.
In this thesis, we establish non-linear wavelet density estimators and study the asymptotic properties of the estimators with data MAR when covariates are present. The outstanding advantage of non-linear wavelet method is estimating the unsmoothed functions, however, the classical kernel estimation cannot do this work. At the same time, we study the large sample properties of the ISE for hazard rate estimator.
The estimation methods and theoretical results mentioned in this thesis are based on independent identically distributed sample. But in many practical fields, we often encounter some mixing sequences, for instance, α-mixing, ρ-mixing and ϕ-mixing. The traditional methods are no longer applicable, which requires us to develop new estimation methods. That is what we are going to do.