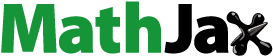
ABSTRACT
We examine the conditions under which descriptive inference can be based directly on the observed distribution in a non-probability sample, under both the super-population and quasi-randomisation modelling approaches. Review of existing estimation methods reveals that the traditional formulation of these conditions may be inadequate due to potential issues of under-coverage or heterogeneous mean beyond the assumed model. We formulate unifying conditions that are applicable to both types of modelling approaches. The difficulties of empirically validating the required conditions are discussed, as well as valid inference approaches using supplementary probability sampling. The key message is that probability sampling may still be necessary in some situations, in order to ensure the validity of descriptive inference, but it can be much less resource-demanding given the presence of a big non-probability sample.
1. Introduction
There is a resurgence of interest in the use of non-probability samples. See, for example, Baker et al. (Citation2013) and Elliott and Valliant (Citation2017) for two recent reviews. Such data may arise in situations where probability sampling is either infeasible or too costly. The observations may be obtained from the so-called big-data sources, such as payment transaction data via a specific platform, cellphone call data from a major provider of the service. These big-data non-probability samples can be much larger in size, compared to the more familiar non-probability samples collected from web panel surveys, quota sampling, etc.
Following Rubin (Citation1976) and Little (Citation1982), Smith (Citation1983) considers the so-called super-population (SP) approach to inference from non-probability sample. Under this approach, a prediction model is constructed for the outcome variable of interest, often conditional on some chosen covariates. In particular, Smith (Citation1983) observes an important distinction between analytic and descriptive inference. In analytic inference, the target is the model parameters that are of a theoretical nature; such parameters can never be observed directly no matter how large the sample is. Whereas the targets of descriptive inference are statistics of a given finite population, such that in principle they can be directly observed given a perfect census of the population.
Moreover, Smith (Citation1983) focuses on validity conditions, under which the non-probability sample observation mechanism can be ignored, in the sense that inference can be based on the observed distributions directly, such as the conditional distribution of the outcome variable given the covariates in the sample. The two key validity conditions under the SP approach can be roughly stated as follows: (i) the prediction model is correctly specified for the population units, (ii) the non-probability sample selection mechanism is non-informative, in the sense that the relevant distribution under the population model can be observed in the non-probability sample directly. Similar validity conditions for the SP approach apply in other situations, such as purposive sampling (Royall, Citation1970), missing data problems (Rubin, Citation1976).
In this paper, we concentrate on descriptive inference methods that depend on validity conditions in the sense of Smith (Citation1983). Of course, inference is also possible without such validity conditions. For instance, not missing-at-random models (Rubin, Citation1976) can be used to deal with informative missing data, where the unobserved full-sample outcome distribution is not the same as that among the respondent subsample. Or, the sample likelihood of Pfeffermann, Krieger, and Rinott (Citation1998) can be applied to survey data under informative sampling, where the distribution that holds in the population cannot be directly observed in the sample. See also Pfeffermann (Citation2017) for several other situations where this approach may be relevant. We do not consider such approaches here, which require explicitly modelling the informative observation mechanism of sample selection or measurement.
As reviewed by Elliott and Valliant (Citation2017), there exists another quasi-randomisation (QR) approach to non-probability samples. Under the QR approach, one hypothesises a randomisation model of the non-probability sample inclusion indicator, but treats the outcomes of interest as unknown constants in the population. Though it is clearly inspired by the randomisation approach based on probability sampling, the QR approach is also a model-based approach, based on a model of the sample inclusion indicator instead of a prediction model of the outcome variable under the SP approach. A key motivation is that the correct inclusion probability can be used for any outcome of interest, just like when it is known under probability sampling, whereas the SP approach by nature must be specified differently for different outcome variables. In the context of survey sampling, the QR approach was introduced to deal with nonresponse, where response to survey is modelled as the second phase of selection, in addition to the first phase of sample selection according to a probability sampling design (Oh & Scheuren, Citation1983).
According to Elliott and Valliant (Citation2017), two key validity conditions are required for the QR approach. (I) The non-probability sample does have a probability sampling mechanism, even though it is unknown. In particular, one assumes that this hypothesised sample inclusion probability is strictly positive for all the population units, so that the only difference to probability sampling is that the inclusion probability is unknown. (II) There exist a set of covariates that ‘fully govern the sampling mechanism’. In other words, the sample inclusion probability is a function of these covariates.
Thus there are two model-based approaches to inference from non-probability sample. Under the SP approach, one models the outcome variable conditional on the realised sample inclusion indicators, whereas under the QR approach, one models the sample inclusion indicators, but treats the outcomes as unknown constants. Although one may envisage the outcomes as the realised values of random variables, a fully specified model of the outcome variable will not be required under the QR approach, given suitable validity conditions. Similarly, although one acknowledges that the sample selection mechanism may be critical to the SP approach, a fully specified model of the inclusion indicator will not be required under the SP approach, given suitable validity conditions.
It is possible to construct estimators that combine both the models of outcome and sample inclusion indicator, in a manner such that the estimator is consistent as long as one of the two models hold. Over the recent years, it is becoming common to refer to this estimation approach as ‘doubly robust’ (Kang & Schafer, Citation2007; Kim & Haziza, Citation2014; Robins, Rotnitzky, & Zhao, Citation1994). Notice that the traditional generalised regression estimator in survey sampling is doubly robust in the same sense, except that here the randomisation mechanism is actually known. Nevertheless, it is a fact that in the debate between model-based and design-based inference from probability sampling, either side questioned the ‘robustness’ of the other.
The rest of the paper is organised as follows. In Section 2, we review the estimation methods from non-probability sample which do require validity conditions. Although these have been roughly stated above, a closer examination under both modelling perspectives reveals nuances across the different estimators. Moreover, we shall highlight the potential challenges of under-coverage and heterogeneous means beyond the assumed model. The traditional formulation of validity conditions is inadequate in both regards. We outline a set of unified validity conditions in Section 3, which are formulated non-parametrically and encompasses both the modelling approaches. Post-stratification and calibration estimators are considered in light of these conditions. However, as will be discussed, a key difficulty in practice is that the validity conditions may be impossible to verify empirically based only on the data used for the estimation. Finally, we outline shortly in Section 4 some valid approaches given a supplementary probability sampling of the outcome of interest, followed by a brief summary in Section 5.
The key message is that probability sampling may still be necessary in some situations, in order to ensure the validity of descriptive inference, but it can be much less resource-demanding given the presence of a big non-probability sample. In fact, the bigger the non-probability sample, the better it is.
2. Review of existing approaches
Denote by U the population of known size N. Let each population unit be associated with an outcome of interest, denoted by , for
. Denote by B the observed nonprobability sample of size
. A common assumption to all the estimators we discuss below is that B does not contain any out-of-scope units, such that
, and there are no duplicated units in B. Let
if
, and 0 if
. Let
be observed for all the units in B, and let
. To fix the idea, let
be the population total that is the target of descriptive inference. Let
in cases where any relevant covariates
are available in the sample B. Let
be the population totals and let
. Given
, one can have two situations depending on whether
are known or not. In the case they are unknown, it may still be possible that there exists a second probability sample S, for
, in which
is observed, so that
can be estimated based on the sample S.
2.1. B-sample expansion estimator
Consider first the most basic situation where only is observed, and no relevant covariates are available at all. Let
be the B-sample mean. The B-sample expansion estimator of Y is given by
(1)
(1) Under the SP approach, let
be the conditional expectation of
given
, for any
, where both
and
are treated as random variables. Provided the conditional expectation is the same as the unconditional expectation given either
or
, for any
, denoted by
(2)
(2) we have
such that the B-sample expansion estimator is prediction unbiased for Y. We shall refer to (Equation2
(2)
(2) ) as the SP assumption, which is a validity condition for the B-sample expansion estimator under the SP approach.
Under the QR approach, where is treated as a fixed constant, let
be the inclusion probability of any population unit that is associated with the value
. The notation ‘;’ is used here instead of ‘
’ because, strictly speaking,
is not a conditional probability now that
is not conceived as the realised value of a random variable under the QR approach. Now, provided the inclusion probability is the same for any
,
(3)
(3) we have
is unbiased for Y, since
In reality, p is unknown. It is natural to estimate it by
under (Equation3
(3)
(3) ), which yields (Equation1
(1)
(1) ) as the resulting plug-in estimator. It follows that the QR assumption (Equation3
(3)
(3) ) is the key validity condition, which ensures that the B-sample expansion estimator is consistent for Y, as
and
asympotically.
In summary, the B-sample expansion estimator (Equation1(1)
(1) ) can be motivated under both the SP and QR approaches, given validity conditions (Equation2
(2)
(2) ) and (Equation3
(3)
(3) ), respectively.
2.2. B-sample calibration estimator
Suppose relevant covariates are available in the sample B. The population totals X may be either known or unknown. In the latter case, suppose they can be estimated from a second probability sample S. The B-sample calibration estimator of Y is given by
(4)
(4) where
is some consistent S-sample estimator, as the S-sample size increases, and the weights
are calibrated in a way depending on the availability of X.
To actually compute the estimator (Equation4(4)
(4) ), one needs to choose a set of initial weights, denoted by
, and a distance function such as
between the initial and calibrated weights (Deville & Särndal, Citation1992). In the case of
(5)
(5) where
is the true B-sample inclusion probability, for
, the calibration estimator is consistent, as
and
, given mild regularity conditions in addition. However, insofar as one cannot manage to set the initial weights (Equation5
(5)
(5) ), the calibration estimator is unmotivated from the QR perspective.
Next, under the SP approach, suppose the SP assumption given by
(6)
(6) which relates the conditional expectation of
linearly to the given
, and
(7)
(7) by which the B-sample selection is non-informative given
. We have then
given
, regardless of the initial weights
. Otherwise, this expectation would tend to 0, provided
is an asymptotically unbiased estimator of X, under some suitable asymptotic setting. It follows that the assumptions (Equation6
(6)
(6) ) and (Equation7
(7)
(7) ) are the key validity conditions for the B-sample calibration estimator under the SP approach.
The estimator (Equation4(4)
(4) ) becomes the B-sample post-stratification estimator in the special case where
is the post-stratum dummy index. For the QR approach, one can set
to be the inverse post-stratum B-sample fraction, which is equivalent to introducing the QR assumption (Equation3
(3)
(3) ) in each post-stratum separately. This QR
assumption provides then a validity condition for the B-sample post-stratification estimator under the QR approach. For the SP approach, the two assumptions (Equation6
(6)
(6) ) and (Equation7
(7)
(7) ) remain formally the same.
2.3. B-sample inverse propensity weighting
Suppose relevant covariates are available in the sample B. The B-sample inverse propensity weighting (IPW) estimator is constructed under the QR approach. Suppose
(8)
(8) i.e., the B-sample inclusion probability is completely determined given
, in the strictly positive parametric form
, which may as well be referred to as the QR
assumption. Provided
is known for all the units in the population, η can be estimated, say, by a population estimating equation
where
. Otherwise, suppose
is observed in a second probability sample S, one can use the pseudo population estimating equation
(Kim & Wang, Citation2018), where
is the sampling weight, for
, or some S-sampling design-consistent adjustment of it. This requires that one is able to observe
for each unit i in S, in other words the two samples S and B can be matched, which is an important assumption in terms of application. To ensure that
is the same in both of these two estimating equations, i.e., whether
or just
, one needs to assume that S-sampling from U is non-informative for
, so that
(9)
(9) Notice that, given non-informativeness (Equation9
(9)
(9) ), we have
for all
, such that one can also use the unweighted S-sample estimating equation, which is given by
instead of the pseudo population estimating equation. Having obtained the parameter estimate
, one obtains
and the B-sample IPW estimator
(10)
(10) which is consistent for Y under mild regularity conditions, if
is consistent for η under some suitable asymptotic setting. It follows that the QR
assumption (Equation8
(8)
(8) ) is its key validity condition, whereas the non-informativeness assumption (Equation9
(9)
(9) ) is needed in addition when
is only available in a probability sample S instead of the population.
2.4. Another B-sample IPW estimator
Elliott and Valliant (Citation2017) discuss another IPW estimator (Equation10(10)
(10) ), where
is obtained with the help of a second so-called reference probability sample S, and is given by
(11)
(11) where
if
and 0 if
, and to fix the idea one may suppose
. First, the QR
assumption (Equation8
(8)
(8) ) is retained. The definition of
by (Equation11
(11)
(11) ) can then be motivated as follows:
provided the S-sample inclusion probability is also fully determined by
in the sense of (Equation8
(8)
(8) ). Thus the validity condition for the IPW estimator (Equation10
(10)
(10) ) based on (Equation11
(11)
(11) ) is that the QR
assumption (Equation8
(8)
(8) ) holds for both the samples, given the same
.
We make two observations. First, despite the superficial resemblance to the propensity scoring method of Rosenbaum and Rubin (Citation1983), the above argument for is not the same. As Rosenbaum and Rubin (Citation1983) state clearly before their first enumerated equation, ‘in this paper, the N units in the study are viewed as a simple random sample from some population’, where N is the size of the combined sample of treatment and non-treatment. The analogy to this combined sample is
here. However, it is generally untenable that
can be treated as a simple random sample from the population. Second, for any given probability sample S, it is possible to identify the variables that determine the designed inclusion probability, denoted by
, for
. There arises thus a question, ‘what if
differs considerably from
?’ Moreover, one may have more than one probability sample in which
is observed. There arises then a question, ‘which reference sample should one use?’
2.5. Sample matching estimator
Rivers (Citation2007) applies the SP approach in situations where a second probability sample S is available. Yang and Kim (Citation2018) study mass imputation methods, which include the matching estimator of Rivers (Citation2007) as a special case. The sample matching (SM) estimator is given by
(12)
(12) where
, for
based on a chosen metric
. That is,
is the nearest-neighbour (NN) imputation value from the B-sample for
.
To focus on the idea, assume for the moment exact matching is the case, where for all
and
. We have then
, which is the same as
as if the unit i were in B. Given the non-informativeness assumption (Equation7
(7)
(7) ) for the B-sample, which Yang and Kim (Citation2018) call the ‘ignorability’ assumption, we have
With respect to both the population model and the design of S, the SM estimator (Equation12
(12)
(12) ) is prediction unbiased for Y. Notice that in the case of S = U, the SM estimator is just an NN-imputation method. Whether S = U or not, the NN-imputed SM estimator is likely to be less efficient than a prediction-imputed SM estimator
whenever a correct parametric specification of the conditional mean (via β) is possible. The simulation results of Yang and Kim (Citation2018) show that NN-imputation is less efficient than imputation based on semi-parametric generalised additive models.
Now, it is not difficult to see that the consistency of the SM estimator (Equation12(12)
(12) ) can be established, given asymptotic exact matching instead, i.e.,
(13)
(13) for any
, as
and
. Yang and Kim (Citation2018) make the assumption of ‘common support’ to the same effect. To ensure that
does not change abruptly as the value
varies, Yang and Kim (Citation2018) assume that
is continuous differentiable. Or, one may adopt the SP
assumption below:
(14)
(14) (Chen & Shao, Citation2000, Theorem 1). It follows that the assumptions (Equation7
(7)
(7) ), (Equation13
(13)
(13) ) and (Equation14
(14)
(14) ) are the key validity conditions for the consistency of the SM estimator (Equation12
(12)
(12) ).
We make two observations. First, an attractive feature of the NN-imputation is that the imputed sample S looks more realistic and natural than, say, by the regression prediction imputation. However, unless the S-sampling is non-informative, the NN-imputed S-sample will not resemble the true S-sample that could have been observed, since
where the inequality is the case unless S-sampling is non-informative in the sense of (Equation7
(7)
(7) ). Second, for any other covariate
, including when
contains the S-sample design variables, we have
unless
and
are conditionally independent of each other given
. This is a general problem for statistical matching of variables associated with distinct units, i.e.,
associated with
for some
and
associated with the same value
but for some different unit in S. The following example illustrates both remarks above.
Example
Let be independent of
, for any
. Then, the SP
assumption (Equation14
(14)
(14) ) holds trivially, as long as the marginal expectation
exists. Next, suppose simple random sample B, so that the non-informative assumption (Equation7
(7)
(7) ) holds, and
regardless of the exact matching assumption. Suppose stratified simple random S-sampling with two strata of different sampling fractions, so that the S-sample inclusion probability is not a constant. Then, the S-sampling is informative (given
) as long as the population stratum means are different, since
where
is the true S-sample mean that is unknown, since
is not observed in S. It follows that the NN-imputed S-sample
would look like a sample generated by simple random sampling, rather than the actual stratified sampling. Moreover, the SM-estimator of stratum means, corresponding to say
, respectively, will be biased for the population stratum means.
3. More generally on validity conditions
Non-informative selection in form of (Equation7(7)
(7) ) or (Equation9
(9)
(9) ) is a critical condition for all the methods in Section 2, which make use of auxiliary variable
. Two kinds of possible violation of these assumptions are worth noting.
First, Kim and Rao (Citation2018) point out an important issue that has not received sufficient attention in these methods, namely B-sample under-coverage is the case if some population units have in fact zero chance of being included in it. Under the SP approach, extrapolation of the conditional distribution of in the B-sample to these population units can only be based on subjective beliefs but not empirical evidence. The QR approach is equally affected, since randomisation inference would have been invalidated even if
were known for all the B-sample units, let alone when it is unknown and needs to be estimated. To address the issue, Kim and Rao (Citation2018) consider a two-phase SM estimator. Let the S-sample be partitioned into
and
, such that
and
. First, estimate this unobserved partition via the B-sample support:
Each S-sample unit that is unsupported in the B-sample ε-neighbourhood is assigned to
. Let us suppose this partition estimator is consistent in the following sense:
as
and
. Next, the two-phase SM estimator is given as
where
. In other words, the under-coverage is dealt with by the calibration of the weights
. This can be motivated, provided the conditional mean
can be linearly related to
, and the relationship is the same for the units with
, i.e., the under-coverage is non-informative for the SP linear model.
Second, insofar one requires either an assumption of SP (Equation7
(7)
(7) ) or QR
(Equation9
(9)
(9) ), there is always the possibility of heterogeneous mean, beyond what is controlled by the chosen
. Let
be of the size
. Under the SP approach, which models the mean
of unit i by
, heterogeneous mean is the case if
, despite
(15)
(15) and
is statistically correct in that the
's average to
over all the units in
. Under the QR approach, heterogeneous mean is the case if
, despite
(16)
(16) Let us illustrate the concept of heterogeneous mean with a simple example.
Example
Let , such that
, for all
. Let
be a partition. Let
be of size
and with mean
, for all
; let
be of size
and with mean
, for all
. Suppose
. Let
. Then,
for any
, but we still have
, satisfying (Equation15
(15)
(15) ).
Heterogeneous mean affects the SP and QR approaches differently. Given (Equation15(15)
(15) ), assuming
for
is prediction unbiased, despite heterogeneous mean, since
Meanwhile, given (Equation16
(16)
(16) ), assuming
for
yields
in which case the IPW estimator under the QP approach may be biased, despite the model of
is statistically correct in the sense of (Equation16
(16)
(16) ).
The discussion above suggests that the formulation of validity conditions in Section 2 is inadequate in the presence of under-coverage and mean heterogeneity. Below we first reformulate the validity conditions, which cover both the SP and QR approaches, despite the presence of under-coverage and mean heterogeneity. We elaborate and illustrate these conditions for the post-stratification and calibration estimators. Finally, we discuss the difficulties of verifying these validity conditions empirically.
3.1. Non-parametric asymptotic (NPA) non-informativeness
We start by noticing that the B-sample mean equals to the population mean, denoted by , provided
where
and
denote, respectively, expectation and covariance with respect to the empirical distribution function that places point mass 1/N on each population unit. This provides an empirical formulation of the non-informativeness of the B-sample observation mechanism with respect to the outcome of interest. Similar expressions have appeared in various discussions of the potential sample mean bias due to the observation mechanism, such as unequal probability sampling (Rao, Citation1966), survey nonresponse (Bethlehem, Citation1988) or big data (Meng, Citation2018). It motivates the following non-parametric asymptotic (NPA) non-informativeness assumption in the absence of any covariates:
(17)
(17) The NPA assumption (Equation17
(17)
(17) ) encompasses both the SP and QR approaches. For the SP approach, taking the conditional expectation of
's conditional on the
's yields
given NPA non-informative B-selection, where
given non-negligible B-selection in addition. Under this condition, the B-sample expansion estimator (Equation1
(1)
(1) ) is asymptotically prediction unbiased from the SP perspective. For the QR approach, taking the expectation of
s with the
s being constants yields
In particular, the NPA assumption (Equation17
(17)
(17) ) allows for
, so that the B-sample expansion estimator (Equation1
(1)
(1) ) remains consistent from the QR perspective, even in the presence of under-coverage of the units with
or non-representative units with
.
Example
Let be a partition. Let
be of size
, where
for
; let
be of size
, where
for
. Despite under-coverage of
, the first NPA condition implies
, given the second condition
.
3.2. Post-stratification estimator
Consider post-stratification by , for
. Provided the assumption (Equation17
(17)
(17) ) holds within each post-stratum, the B-sample post-stratification estimator is asymptotically unbiased from both the SP and QR perspectives. Below we consider the QR approach. The SP approach is a special case of the calibration estimator discussed in Section 3.3.
Consider first the hypothetical estimator with known :
To fix the idea for variance estimation, suppose independent Bernoulli distribution of
with probability
, where
. The variance of
is then given by
An unbiased estimator of the first term of the variance, denoted by
is given by
where
. An unbiased estimator of the second term, denoted by
is given by
where the second equality follows given the additional QR
assumption, i.e.,
for
. Putting
and
together, we obtain
Now, the post-stratification estimator, denoted by
, is obtained from
on replacing
by
, where
is the observed size of
and
is the known post-stratum population size. Expanding
around
(i.e., linearisation) would yield an asymptotically valid estimator of the unconditional variance of
.
3.3. Calibration estimator
The post-stratification estimator is infeasible, in cases when the B-sample contains empty cells, or when the population size is not all known. Let
be a vector of many-to-one mappings of
, such that the population total
is known, and the sample total
has only non-zero components.
As discussed for the calibration estimator in Section 2, generally one is not able to set the initial weight to be the inverse of B-sample inclusion probability in practice. Suppose one simply starts with initial equal weights for all
. The linear calibration estimator (Deville & Särndal, Citation1992) is given by
where the weights
minimise the distance to
as measured by
subjected to the constraints
, where
and
. It follows that
, for
, since the only thing that matters to the calibration constraints is
now that
for
and, given whatever
, the term
is minimised at
for
.
As the first validity condition for , suppose there exists a vector
, such that
(18)
(18) for each t-value, as
, where
, and
is the population size of
. The condition (Equation18
(18)
(18) ) is analogous to the SP
assumption (Equation6
(6)
(6) ), where the covariate
is replaced by
here. Moreover, it relaxes the model (Equation6
(6)
(6) ) of the conditional mean, allowing for potential heterogeneous mean similar to (Equation15
(15)
(15) ). Now that
, we have
Given (Equation18
(18)
(18) ),
as
. Moreover, we have
as
, given
(19)
(19) for any given t, which is an adaption of the NPA non-informativeness assumption (Equation17
(17)
(17) ) to the present setting. It follows that the two assumptions (Equation18
(18)
(18) ) and (Equation19
(19)
(19) ) are the key validity conditions for the calibration estimator to be consistent for Y.
For variance estimation, suppose again independent Bernoulli distribution of with probability
, where
. An approximate variance estimator for the calibration estimator
can then be given as
where
, and
.
3.4. Validation of non-informative B-sample selection
Of the validity conditions discussed above, the critical assumption is non-informative B-sample selection, which can be stated in various forms. For instance, given the non-informativeness assumption (Equation17(17)
(17) ), an additional assumption like (Equation18
(18)
(18) ) can in principle to validated empirically. However, the non-informativeness condition may not hold exactly, and it is generally impossible to verify only based on the data used for the estimation. Below we discuss the issue in more detail.
Consider first the propensity model under the QR approach. Suppose known
to avoid additional complications otherwise, the census score equation is
which is always satisfied by
, i.e., the saturated model. Insofar as a non-saturated model of
does not fit perfectly to the data, one can always attribute its cause to the non-saturated functional form of
, instead of rejecting the assumption that the set of covariates
‘fully govern the sampling mechanism’. In this sense, the validity of the latter assumption cannot be refuted empirically.
Next, for the SP approach, where both and
are treated as random, assume the B-sample inclusion probability
depends on
, where
is known for
to avoid extra complications. For goodness-of-fit checks, let
be a known covariate, which is distinct from
. We have
where
and
. The two observed checks are
Setting
, which fits the assumption
, both the two checks are satisfied given
, i.e., the B-sample expansion estimate of
is perfect for all x. This would suggest that the NPA assumption (Equation17
(17)
(17) ) holds for
given
and may be considered to support the plausibility of the NPA assumption (Equation17
(17)
(17) ) for
given
, provided
is known to be correlated with
, but not otherwise. However, in situations where such a covariate
is available, it seems natural that it should be used in the estimation of Y to start with. The two checks amount then to the case of
and are satisfied trivially by setting
. Thus one is faced with a dilemma, where building the best model for estimation would at the same time reduces the ability to verify it.
4. Using additional probability sample of outcomes
So far we have only considered the situations, where the outcome values of interest are only observed in the non-probability sample B. Obviously, the situation changes completely, given in addition a probability sample of outcomes. Below we discuss shortly two different approaches to inference in the absence of any relevant covariates. The ideas remain the same in situations with additional covariates.
The first approach aims at consistent estimation combing the two samples, as for example, discussed in Tam and Kim (Citation2018a, Citation2018b), where the probability sample is taken from the whole population and overlaps with the B-sample. These authors also discussed additional issues such as measurement errors or nonresponse. Here we discuss the situation where the probability sample is taken from the B-sample complement population. Given the non-probability sample observations , one may treat
as fixed, and select a second supplementary sample from the rest of the population, denoted by
. Given the S-sample observations of the outcome, denoted by
, it is straightforward to obtain a test for
vs.
, given as
where
is an S-sample estimator of the population mean outside of the B-sample, i.e.,
and
is the associated variance estimator. If
is not rejected, then there is the possibility of using
as an estimate on its own, without regular concurrent surveys in future. This would achieve the greatest cost savings. To this end, one may consider S as a particular form of audit sampling, whose aim is to validate the big-data estimate
and to provide a meaningful accuracy measure that can accommodate its potential bias. Zhang (Citation2019) develops an approach to audit sampling inference for big data statistics.
Let . A consistent estimator of
using both samples is given by
where
is the S-sample inclusion probability, and the validity of
now derives from probability sampling of S, regardless of how the B-sample is generated. The relative efficiency (RE) against the setting without the B-sample can be given by
where
is a hypothetical probability sample from the whole population U, which has the same sample size and the same sampling design as S. One may refer to this as the split-population approach to inference, which is an age-old idea for combining survey sampling with administrative data. The efficiency gain would be substantial provided the B-sample is large. In fact, the larger the B-sample, the greater is the efficiency gain.
Under the second approach to inference, consider a composite estimator given by
where γ is the composition weight, for
. Notice that when
, the composite estimator is just the split-population estimator
above, which is consistent for
. As γ increases from
towards one, one risks introducing greater bias, insofar as
. However, the composite estimator may yield a smaller mean squared error (MSE) of estimation, provided this is desirable. One is then essentially trading the increasing bias
against the decreasing stand error
, as γ increases. The composite estimator that achieves the minimum MSE is given by
Estimating
by
in application, one can use
The validity of the composite approach derives from probability sampling of S, regardless of how the B-sample is generated. Again, the bigger the B-sample, the better it is.
5. Summary
All the estimators from non-probability sample observations reviewed in Section 2 are model based, whether the modelling is carried out under the SP or QR approach. Two features regarding the model covariate , for
, are worth recapitulating:
compared to the situation with known
, making use of an additional probability sample
entails a loss of efficiency, as can be expected;
the availability of an additional probability sample without the outcome variable is not a principal advantage, since it does not simplify the validity conditions compared to the situation where
is known, but it does resolve the practical difficulty when
is unavailable yet some functions of
are needed for descriptive inference.
The situation changes completely, given in addition a probability sample of outcomes. The probability sample then enables valid descriptive inference in combination with the non-probability probability sample. Depending on the circumstances, the probability sample can either be selected from the whole population, or just the rest population outside the non-probability sample.
Finally, in situations where the non-probability sample is large, the cost savings will be the greatest if it can replace regular survey sampling altogether. Use of an additional probability audit sample is needed to validate the non-probability sample estimate, in spite of possible failure of its underlying model assumptions, and to provide a meaningful accuracy measure that can accommodate its potential bias.
Disclosure statement
No potential conflict of interest was reported by the author.
ORCID
Li-Chun Zhang http://orcid.org/0000-0002-3944-9484
Additional information
Notes on contributors
Li-Chun Zhang
Li-Chun Zhang is Professor of Social Statistics at University of Southampton, Senior Researcher at Statistics Norway, and Professor of Official Statistics at University of Oslo. His research interests include finite population sampling design and coordination, graph sampling, sample survey estimation, non-response, measurement errors, small area estimation, index number calculations, editing and imputation, register-based statistics, analysis of integrated data, statistical matching, record linkage, population size estimation.
References
- Baker, R., Brick, J. M., Bates, N. A., Battaglia, M., Couper, M. P., Dever, J. A., …Tourangeau, R. (2013). Report of the AAPOR task force on non-probability sampling (Technical Report). Deerfield, IL: American Association for Public Opinion Research.
- Bethlehem, J. (1988). Reduction of nonresponse bias through regression estimation. Journal of Official Statistics, 4, 251–260.
- Chen, J. H., & Shao, J. (2000). Nearest neighbor imputation for survey data. Journal of Official Statistics, 16, 113–131.
- Deville, J.-C., & Särndal, C.-E. (1992). Calibration estimators in survey sampling. Journal of the American Statistical Association, 87, 376–382. doi: 10.1080/01621459.1992.10475217
- Elliott, M. R., & Valliant, R. (2017). Inference for nonprobability samples. Statistical Science, 32, 249–264. doi: 10.1214/16-STS598
- Kang, J. D. Y., & Schafer, J. L. (2007). Demystifying double robustness: A comparison of alternative strategies for estimating a population mean from incomplete data (with discussion). Statistical Science, 22, 523–539. doi: 10.1214/07-STS227
- Kim, J. K., & Haziza, D. (2014). Doubly robust inference with missing data in survey sampling. Statistica Sinica, 24, 375–394.
- Kim, J.-K., & Rao, J. N. K. (2018). Data integration for big data analysis in finite population inference. Talk presented at SSC2018. Montreal.
- Kim, J.-K., & Wang, Z. (2018). Sampling techniques for big data analysis in finite population inference. Retrieved from arXiv:1801.09728v1
- Little, R. J. A. (1982). Models for nonresponse in sample surveys. Journal of the American Statistical Association, 77, 237–250. doi: 10.1080/01621459.1982.10477792
- Meng, X. L. (2018). Statistical paradises and paradoxes in big data (I): Law of large populations, big data paradox, and 2016 US presidential election. The Annals of Applied Statistics, 12, 685–726. doi: 10.1214/18-AOAS1161SF
- Oh, H. L., & Scheuren, F. J. (1983). Weighting adjustments for unit non-response. In W. G. Madow, I. Olkin & D. B. Rubin (Eds.), Incomplete data in sample surveys (Vol. 2): Theory and bibliographies (pp. 143–184). New York: Academic Press.
- Pfeffermann, D. (2017). Bayes-based non-Bayesian inference on finite populations from non-representative samples. Calcutta Statistical Association Bulletin, 69, 1–29. doi:10.1177/0008068317696546
- Pfeffermann, D., Krieger, A. M., & Rinott, Y. (1998). Parametric distributions of complex survey data under informative probability sampling. Statistica Sinica, 8, 1087–1114.
- Rao, J. N. K. (1966). Alternative estimators in PPS sampling for multiple characteristics. Sankhya, 28, 47–60.
- Rivers, D. (2007). Sampling for web surveys. Proceedings of the survey research methods section. American Statistical Association.
- Robins, J. M., Rotnitzky, A., & Zhao, L. P. (1994). Estimation of regression coefficient when some regressors are not always observed. Journal of the American Statistical Association, 89, 846–866. doi: 10.1080/01621459.1994.10476818
- Rosenbaum, P., & Rubin, D. (1983). The central role of the propensity score in observational studies for causal effects. Biometrika, 70, 41–55. doi: 10.1093/biomet/70.1.41
- Royall, R. M. (1970). On finite population sampling theory under certain linear regression models. Biometrika, 57, 377–387. doi: 10.1093/biomet/57.2.377
- Rubin, D. B. (1976). Inference and missing data. Biometrika, 63, 581–592. doi: 10.1093/biomet/63.3.581
- Smith, T. M. F. (1983). On the validity of inferences from non-random sample. Journal of the Royal Statistical Society, Series A, 146, 394–403. doi: 10.2307/2981454
- Tam, S.-M., & Kim, J.-K. (2018a). Big data ethics and selection-bias: An official statistician's perspective. Statistical Journal of the IAOS, 34, 577–588. doi:10.3233/SJI-170395
- Tam, S.-M., & Kim, J.-K. (2018b). Mining big data for finite population inference. Talk presented at BigSurv18. Barcelona.
- Yang, S., & Kim, J.-K. (2018). Integration of survey data and big observational data for finite population inference using mass imputation. Retrieved from https://arxiv.org/abs/1807.02817v1
- Zhang, L.-C. (2019). Proxy expenditure weights for consumer price index: Audit sampling inference for big data statistics. Retrieved from https://arxiv.org/abs/1906.11208