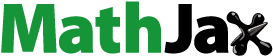
Abstract
This paper is devoted to study the proportional reinsurance/new business and investment problem under the mean-variance criterion in a continuous-time setting. The strategies are constrained in the non-negative cone and all coefficients in the model except the interest rate are stochastic processes adapted the filtration generated by a Markov chain. With the help of a backward stochastic differential equation driven by the Markov chain, we obtain the optimal strategy and optimal cost explicitly under this non-Markovian regime-switching model. The cases with one risky asset and Markov regime-switching model are considered as special cases.
1. Introduction
Insurers usually control their risks via some business activities, such as investing in a financial market, purchasing reinsurance, and acquiring new business. The problem of finding the optimal reinsurance/new business and investment strategies has been investigated in a vast of literature under various criteria, among which minimising ruin probability, maximising expected utility of terminal wealth, and minimising the mean-variance cost are most popular.
Browne (Citation1995) first studied the optimal investment problem for an insurer aiming to maximise the terminal utility function and minimise the ruin probability under a diffusion risk model. Under a similar diffusion model, Promislow and Young (Citation2005) obtained the optimal investment and quota-share reinsurance strategies minimising the probability of ruin. Yang and Zhang (Citation2005) derived the optimal reinsurance-investment strategy maximising the expected utility of terminal wealth under a jump-diffusion model. Chen, Li, and Li (Citation2010) studied the reinsurance-investment problem with a dynamic value-at-risk (VaR) constraint and got the optimal strategy minimising the probability of ruin via the dynamic programming technique and Lagrange multiplier method. More recent work on minimising ruin probability and maximising utility includes Yi, Li, Viens, and Zeng (Citation2013), Liang and Bayraktar (Citation2014) and Xu, Zhang, and Yao (Citation2017), among others.
Since the pioneer work of Markowitz (Citation1952), the mean-variance optimisation problem has become a key topic in modern portfolio selection theory. By embedding the original mean-variance problem into a linear-quadratic (LQ, for short) control problem, Li and Ng (Citation2000) and Zhou and Li (Citation2000) derived the pre-commitment optimal solution to the dynamic mean-variance problem in a multi-period model and a continuous-time model, respectively. Bäuerle (Citation2005) studied the optimal proportional reinsurance problem under the benchmark and the mean-variance criterion. Bai and Zhang (Citation2008) considered the optimal reinsurance/new business and investment problem with no-shorting constraint under the mean-variance criterion. They considered both classical and diffusion risk models in the Markovian framework and solved the problem through the viscosity solution to the Hamilton–Jacobi–Bellman equation. Similarly, with the help of stochastic LQ control theory and viscosity solution, Bi (Citation2013) studied the optimal investment and reinsurance problem for an insurer under the mean-variance criterion with non-negative constraint on the strategies in a jump-diffusion financial market. By using the martingale method, Bi, Meng, and Zhang (Citation2014) investigated the mean-variance optimal investment and reinsurance problem with bankruptcy prohibition. For more recent work on mean-variance reinsurance/new business and investment problem, we refer the reader to Shen and Zeng (Citation2015), Zeng, Li, and Gu (Citation2016), Wang, Wang, and Wei (Citation2019), etc.
In this paper, we study the mean-variance reinsurance/new business and investment problem under a non-Markovian regime-switching model. It is well-known that in the Markov regime-switching model the coefficients are deterministic functions of a Markov chain. Due to its flexibility, the Markov regime-switching model is usually used to capture the business cycle and changes in the environment, etc. Zhang and Siu (Citation2012) studied the optimal proportional reinsurance and investment problem with no short-selling constraint in a Markovian regime-switching models. Chen and Yam (Citation2013) considered the mean-variance reinsurance-investment problem under a regime-switching model by using the similar method in Zhou and Yin (Citation2003). In contrast to the Markov regime-switching model, the coefficients are stochastic processes adapted to the filtration generated by a Markov chain (or jointly by a Markov chain and a Brownian motion, see Siu & Shen, Citation2017) in the non-Markovian regime-switching model. The advantage of this kind of model is that it may capture the path-dependence and memory effect in the financial market, since the parameters depend on not only the current state but also the historical information of the Markov chain. Under non-Markovian regime-switching models, Shen, Wei, and Zhao (Citation2018) investigated the mean-variance asset-liability management problem; Wang and Wei (Citation2019) studied the mean-variance portfolio selection problem via mean-field formulation.
We assume that the insurer can purchase proportional reinsurance and access a financial market consisting of a riskless asset and multiple risky assets. The claim process of the insurer and the price processes of the risky assets are correlated and modelled by drifted and geometric Brownian motions, respectively. The coefficients in the model depend on the historical information of a Markov chain. Similar to Bai and Zhang (Citation2008), we impose the non-negative constraint on the retention level and the investment strategy. Since our model is non-Markovian, the viscosity method used in their paper fails. Instead, we follow the method proposed by Hu and Zhou (Citation2005) which studied a stochastic LQ control problem with control constrained in a cone. By using a backward stochastic differential equation (BSDE, for short) driven by the Markov chain, we obtain the optimal strategy and efficient frontier in closed-form. We also study the Markov regime-switching model as a special case, where the BSDE degenerates to a system of ordinary differential equations (ODE, for short). It is worthy of noting that under the Markov regime-switching model, Chen and Yam (Citation2013) gave a condition under which the optimal reinsurance and investment strategy without constraints are indeed non-negative. We show that our results are consistent with theirs.
The remainder of this paper is organised as follows. In Section 2, we introduce some notations and formulate the mean-variance problem. In Section 3, we show the main results of the paper. In Section 4, we consider two special cases with one risky asset and Markovian regime-switching model. Finally, Section 5 concludes the paper.
2. The model
2.1. Notation
Let be a fixed time duration, where
. Let
be a filtered probability space on which an
-dimensional standard Brownian motion
and an irreducible continuous-time Markov chain
with finite states are defined. Here, the filtration
is the augmentation under
of the natural filtration generated by
and
. We also assume that the Brownian motion and the Markov chain are independent of each other.
Without loss of generality, let be the state pace of
, where
are the ith unit column vectors in
. Let
be the Q-matrix of the Markov chain
at time t. We assume that the entries in
are uniformly bounded and continuous. Denote by
the augmentation under
of the natural filtration generated by the Markov chain
.
From Elliott, Aggoun, and Moore (Citation2008, Appendix B), we have the following semi-martingale representation of the Markov chain :
(1)
(1) where
is a martingale valued in
.
Similar to Cohen and Elliott (Citation2008), we define
For a matrix
with proper dimension, we denote
.
Throughout this paper, we will use the same notations as in Hu and Zhou (Citation2005). We list here for the reader's convenience. Denote the inverse of a non-singular square matrix by
and the n-dimensional identity matrix by
. The transpose and the norm of any vector or matrix
are denoted by
and
, respectively. If
, it means that
is a
-dimensional real matrix in which all entries are non-negative. We denote the set of symmetric
(square) matrices by
. We list the following spaces about random variables or stochastic processes involved in this paper. For
, a positive integral number k,
, etc., let
is called uniformly positive definite, if there exists a deterministic constant c>0 such that
for a.e.
and
-a.s.. For any real number we define
, and especially,
and
.
2.2. Mean-variance reinsurance and investment problem
Assume that the insurer is allowed to invest the surplus into a financial market, consisting of a risk-free asset and n risky assets. The price of the risk-free asset satisfies
(2)
(2) where the interest rate
is a deterministic, uniformly bounded, scalar-valued function. For
, the price of the kth risky asset
is given by
(3)
(3) where
is the expected return rate of the kth risky asset and
is the volatility rate.
is usually called the risk premium vector at time t. Usually,
are more popular and reasonable for every investor or insurer. For convenience, denote by
in following.
Following Promislow and Young (Citation2005), we model the claim process according to a Brownian motion with drift as
(4)
(4) where
are bounded
-adapted stochastic processes. We assume that
. In this paper, the claim process
and the risky assets are correlated while they are independent to each other in Chen and Yam (Citation2013).Footnote1
We assume that the premium is paid continuously at rate with safety loading
. Then before introducing reinsurance and investment, the surplus process
is given by
(5)
(5) To control the original insurance risk, we assume the insurer can purchase proportional reinsurance or acquire new business as described in Bäuerle (Citation2005) and Bai and Zhang (Citation2008). Let the retention level for the original insurer/ceded company at time t be
. Then for the claim Y arriving at time t, the part of the claim the insurer pays is
and that paid by the reinsurance/ceded company is
. In this paper, we only consider the cheap reinsurance. Then, the insurer pays reinsurance premiums continuously at rate
. After the purchase of reinsurance, the surplus process
becomes
(6)
(6) Since
can be interpreted as acquiring new business, we restrict the reinsurance strategy
in this paper.
Let be the amount of the insurer's wealth invested in the kth risky asset at time t. In this paper, short-selling of the risky assets is not allowed, i.e. it must be satisfied that
The process
is called an investment portfolio of the insurer at the risk financial market. Then the joint strategy of the reinsurance and risk investment can be described by a
-dimensional stochastic process, denoted by
. Let
be the wealth of the insurer, who adopts the reinsurance strategy
and the investment portfolio
. Given an investment portfolio
, the amount of the insurer's wealth invested in the free-risk asset can be determined by
. Therefore, for an initial wealth
, the dynamics of the wealth process
is given by the following stochastic differential equation:
(7)
(7) where
Assumption 2.1
are
-a.s. predictable processes.
is uniformly non-degenerate, i.e. there exists a constant
such that
(8)
(8)
Remark 2.1
By some elementary matrix operations, we can show the following results under Assumption 2.1:
For all
,
and
are positive definite;
There are two positive constants
and
such that for a.e.
and
-a.s.
(9)
(9)
Definition 2.2
A strategy is admissible if
We denote by
the set of all admissible strategies.
Similar to Hu and Zhou (Citation2005), we consider the following mean-variance optimisation problem.
Definition 2.3
The mean-variance reinsurance and investment problem is a constrained stochastic optimisation problem such that for any given :
(10)
(10) Moreover, the problem is called feasible if there is at least one strategy satisfying all the constraints of (Equation10
(10)
(10) ). The problem is called finite if it is feasible and the infimum
is finite.
Remark 2.2
The restriction of the targeted payoff is natural as the latter can always be achieved by putting all the money in the bank and conducting proportional reinsurance policies with the retention ratio
for all
(i.e. the insurer transfers all of the claim to the reinsurer).
Similar to Hu and Zhou (Citation2005, Theorem 6.1), if we choose and adopt a family of admissible strategies
, by adjusting the coefficient
, it is easy to get the following proposition about the feasibility of the problem.
Proposition 2.4
The mean-variance reinsurance-investment problem (Equation10(10)
(10) ) is always feasible for every
.
3. Solution to the problem
3.1. Preliminaries
In this section, some mathematical preliminaries are presented. In the subsequent analysis, a vital technical tool is Tanaka's formula.
Lemma 3.1
Tanaka's formula
Let be a continuous semi-martingale. Then
where
is an increasing continuous process, called the local time of
at 0, satisfying
(11)
(11)
For , define
(12)
(12) Let
(13)
(13)
(14)
(14) where
and
For the convenience of analysis,
is sometimes partitioned as
where
and
.
Lemma 3.2
Under Assumption 2.1. we have for a.e. and
-a.s.
(15)
(15) and
(16)
(16) where
and
Proof.
Since and
is positive definite (see Remark 2.1), it is easy to find that
and the equality holds if and only if
. Therefore (Equation15
(15)
(15) ) is obtained.
For the second part of the lemma, firstly by noting ,
and (Equation9
(9)
(9) ), we obtain for a.e.
and
-a.s.,
where the last inequality is from that
If
we have for a.e.
and
-a.s.,
and
But
. Thus the minimum value
will be obtained somewhere in the bounded range
, that is to say
Since
(17)
(17) it is not difficult to get that
. Moreover, by (Equation9
(9)
(9) ), we have for a.e.
and
-a.s.
Therefore
(18)
(18) Moreover, considering
, we obtain (Equation16
(16)
(16) ). Consequently, by recalling Assumption 2.1,
is finite.
Remark 3.1
If the claim process is independent of the risky assets, i.e. , it is easy to see
Then
Given
, we consider the SDE
(19)
(19)
Lemma 3.3
SDE (Equation19(19)
(19) ) has a unique solution
(20)
(20) where
(21)
(21)
Proof.
Firstly, we introduce two linear SDEs as follows
(22)
(22) and
(23)
(23) It is easy to get the unique solution to (Equation22
(22)
(22) )
Considering Assumption 2.1, it is easy to obtain that
and
. It is well-known that the unique continuous
-adapted solution to (Equation23
(23)
(23) ) is given by
(24)
(24) Define
The fact that
and
implies that
By applying Itô formula to
, the resulted SDE is exactly (Equation19
(19)
(19) ).
If we can prove the uniqueness of the solution, then the proof of the lemma will be accomplished. To this end, first suppose that and
are two continuous adapted solution to SDE (Equation19
(19)
(19) ). Denote
. Now in order to generate a linear SDE for
, the following procedure was implemented. Set
Then
is a continuous adapted solution to the following linear SDE:
Therefore,
, which implies that
is the unique the solution to SDE (Equation19
(19)
(19) ).
Next, we introduce the following backward stochastic differential equation driven by the Markov chain
(25)
(25) where
.
By Lemma 3.2 and Cohen and Elliott (Citation2010, Theorem 3.11), we have the following result:
Lemma 3.4
There exists a unique solution to the BSDE (Equation25
(25)
(25) ) such that
(26)
(26) and
for some constants
.
3.2. Main results
In this section, we give explicit solutions to the mean-variance reinsurance-investment problem (Equation10(10)
(10) ) in terms of the solution to the BSDE (Equation25
(25)
(25) ).
It is well-known that the Lagrange multiplier method is the standard method to cope with the constraint equation on the mean-variance problem (Equation10
(10)
(10) ). By inserting the Lagrange multiplier
, we can consider the following cost functional:
(27)
(27) Similar to Hu and Zhou (Citation2005), we may first solve the following unconstrained problem parameterized by the Lagrange multiplier
:
(28)
(28) Define
.
Theorem 3.5
Let be the solution to BSDE (Equation25
(25)
(25) ). Then the strategy
(29)
(29) is optimal for the problem (Equation28
(28)
(28) ). Moreover, the corresponding cost is
(30)
(30)
Proof.
First note that Lemma 3.4 ensures that (Equation25(25)
(25) ) has an unique bounded, uniformly positive solution
. Let
be the solution to (Equation7
(7)
(7) ) under an arbitrary admissible strategy
. Noting that the admissible strategy satisfies
, by the theory of stochastic differential equation (SDE, for short), there is a unique solution
for the SDE (Equation7
(7)
(7) ).
Set . By Itô's formula and (Equation7
(7)
(7) ), we have
(31)
(31) Therefore, the cost function (Equation27
(27)
(27) ) can be written as
Applying Tanaka's formula (3.1) to
, we have
(32)
(32) and
(33)
(33) where
is the local time of
at 0. Applying Itô's formula, we get
(34)
(34) where we have used the fact that
by virtue of (Equation11
(11)
(11) ). Similarly, we have
(35)
(35) Applying Itô's formula to
, we have (after some reorganisation)
(36)
(36) Similarly, we can derive
(37)
(37) Next, we define, for any integer
, the following stopping time
as follows
(38)
(38) where
. Obviously,
, is an increasing sequence of stopping times converging to T almost surely.
Summing (Equation36(36)
(36) ) and (Equation37
(37)
(37) ), we get
(39)
(39) Integrating the above formula from 0 to
, and then taking expectation, after arranging we get
(40)
(40) where
(41)
(41) Now let us send
. In addition, noticing that
,
, and
applying the dominated convergence theorem, from (Equation27
(27)
(27) ) we get
(42)
(42) The next step is to show that
for any
. Specifically, the analysis is as follows.
If for some t, then set
. In this case, notice
if only if
. Fixing t given before, substituting
for
, and noticing the definition of
, then
where the equality holds at
,
. Then, the equality in the above holds if and only if the strategy takes
If
for some t, then set
. In this case,
where the equality holds at
Finally, when
, then
with the equality if and only if
.
According to the above analysis, we have
Combining the above analysis and (Equation42
(42)
(42) ), we find that for all admissible strategies
,
(43)
(43) with the equality sign holding if and only if the strategy
adopts
as follows
(44)
(44) i.e. the expression (Equation29
(29)
(29) ). Thus (Equation30
(30)
(30) ) follows from that
where
.
According to the definition of the admissible strategy, we need to show that the strategy defined by (Equation29
(29)
(29) ) should belong to
if it is admissible. Noting the definition of
, and Assumption 2.1 and Remark 2.1, we have
(45)
(45) Therefore
is uniformly bounded.
Now, under the state feedback strategy (Equation44(44)
(44) ), the stochastic differential equation (Equation31
(31)
(31) ) is written as
(46)
(46) with
. By Lemma 3.3, (Equation46
(46)
(46) ) indeed admits a unique solution
. Recalling that
, it is easy to see that
.
Remark 3.2
It is easy to find that
(47)
(47) from (Equation30
(30)
(30) ).
Now, we can show the optimal strategy and efficient frontier of the problem (Equation10(10)
(10) ).
Theorem 3.6
Let be the unique solution to the BSDE (Equation25
(25)
(25) ). Then the efficient strategy corresponding to
as a feedback of the wealth process, for the problem (Equation10
(10)
(10) ) is
(48)
(48) where
(49)
(49) Moreover, the efficient frontier is
(50)
(50)
Proof.
It follows from (Equation16(16)
(16) ) that
. Thus
in (Equation49
(49)
(49) ) is well defined.
First, we intend to directly solve the problem (Equation10(10)
(10) ) for
. Let
be the wealth process corresponding to the efficient strategy
. By linearity, it is easy to see that
where
and
are given by as follows, respectively
(51)
(51) and
(52)
(52) Applying Itô's formula, for
, we obtain
Integrating from 0 to T and taking expectation, it yields that
(53)
(53) Noting
we have
(54)
(54) and
(55)
(55) So if
, under the constraint
in (Equation10
(10)
(10) ), by (Equation55
(55)
(55) ), we immediately obtain that the corresponding efficient strategy
which means that all the wealth to be put in the bank (i.e. the risk-free asset) and all the risk of the insurance business to be passed to the reinsurer. By (Equation54
(54)
(54) ) and
,
. Obviously it is easy to get the corresponding variance
.
Putting into (Equation49
(49)
(49) ) and (Equation48
(48)
(48) ), we can easily obtain that
and
. Moreover, by substituting
for
in (Equation50
(50)
(50) ), we have
That is to say that, according to Definition 2.3, (Equation50
(50)
(50) ) and (Equation48
(48)
(48) ) are indeed the efficient frontier and the efficient strategy corresponding to
. In the following, we only consider for any fixed
.
As described in Hu and Zhou (Citation2005)), by applying the duality theorem, we have for ,
where the last equality is from (Equation47
(47)
(47) ). Recalling (Equation30
(30)
(30) ), by making use of method of completing the square, we have, for any
Considering (Equation16
(16)
(16) ), under the constrained condition
, it is apparent that for
,
where
. As
, we also can get for
,
Then for
, considering (Equation20
(20)
(20) ) in Lemma 3.3, we have
(56)
(56) By Theorem 3.5 and substituting
for λ in (Equation29
(29)
(29) ), we have
The proof is completed.
4. Two special cases
4.1. One risky asset
In this section, we consider i.e. there is only one risky asset. Then (Equation3
(3)
(3) ), (Equation4
(4)
(4) ) and (Equation7
(7)
(7) ) are rewritten as follows
and
where
. In this case, we have,
From Proposition 3.2, we have
where
In the next Lemma 4.1, we get the minimum value
and
for the case with n = 1.
Lemma 4.1
If
then
(57)
(57) where we have suppressed the variable t;
If
we have for
(58)
(58)
Proof.
To get the the infimum of for
, we consider the the following optimisation problem
By Karush–Kuhn–Tucker conditions, it holds that
i.e.
It is easy to get
The proof is completed.
Through applying Lemma 4.1 to Theorem 3.6 and 4.2, we can obtain the explicit expression of the optimal strategy in the case of one risk-free asset.
4.2. Markovian regime-switching model
In this section, we consider the Markovian regime-switching model, that is to say we suppose that all of random coefficients mentioned before in the paper are functions of the state of the Markov chain. To be precise, let
where
are deterministic and bounded. Then other notations are changed correspondingly, for instance,
and
For the BSDE (Equation25
(25)
(25) ), then we have
where
In the following, to ease the presentation, sometimes we simplify the notations slightly by omitting the tilde character above those deterministic bounded functions.
By Cohen and Szpruch (Citation2012), there exists a unique function such that
(59)
(59) and
(60)
(60) where
and
System (Equation60
(60)
(60) ) is a homogeneous linear system of variable-coefficient first-order ordinary differential equations with continuous coefficients. Then, by the classical theory in the ODEs (see, e.g. Walter, Citation1998, P. 162), indeed there is exactly one solution to the system (Equation60
(60)
(60) ). If
are constants. Let
where
Then system (Equation60
(60)
(60) ) has the following unique solution
where
are the eigenvalues and corresponding eigenvectors of
and the constants
is determined by
Applying (Equation59(59)
(59) ) to (Equation48
(48)
(48) ) and (Equation50
(50)
(50) ) in Theorem 3.6, it easily to get the following result.
Theorem 4.2
The efficient strategy corresponding to as a feedback of the wealth process, for the problem (Equation10
(10)
(10) ) is
(61)
(61) where
and the efficient frontier
(62)
(62)
Remark 4.1
For the case with the one risky asset (i.e. n = 1), by Theorem 4.2, the efficient strategy corresponding to is (Equation61
(61)
(61) ) with
and the efficient frontier is
(63)
(63)
Remark 4.2
If the interest rate in Chen and Yam (Citation2013) is deterministic and in our paper, then the models in our paper are the same. In this case,
and the optimal strategy obtained in Chen and Yam (Citation2013) is given by (Equation61
(61)
(61) ) with
and
Under the deterministic interest rate, the assumption in Chen and Yam (Citation2013, Theorem 5.1), which becomes
always holds. It follows from Remark 4.1 that our results are the same.
5. Conclusion
We have investigated an optimal proportional reinsurance and investment problem for an insurer under the mean-variance criterion. We assumed that the claim process of the insurer and the prices of risky assets are correlated and the coefficients (except the interest rate) in the model are stochastic processes adapted to the filtration generated by a Markov chain. Such a non-Markovian model can capture the path-dependence and memory effects in the financial market. Furthermore, we considered the non-negative constraint on the reinsurance and investment strategies. By solving a unconstrained optimisation problem parameterized by the Lagrange multiplier, we obtained the optimal strategy in terms of the unique solution to a BSDE driven by the Markov chain.
Some relevant problems are worthy of being discussed in further. First, we can assume that the interest rate is also a stochastic process. Second, we may consider the non-cheap reinsurance. Third, we only studied the pre-commitment strategy in this paper and hope to investigate the time-consistent equilibrium strategy in our future works.
Disclosure statement
No potential conflict of interest was reported by the author(s).
Additional information
Funding
Notes on contributors
Liming Zhang
Liming Zhang is a Ph.D. candidate, School of Statistics, East China Normal University.
Rongming Wang
Dr Rongming Wang holds a Ph.D. from East China Normal University. He is now a professor at East China Normal University. His research interests include financial risk management, insurance actuarial and mathematical finance.
Jiaqin Wei
Dr Jiaqin Wei holds a Ph.D. from East China Normal University. He is now a research professor at East China Normal University. His research interests include actuarial science and mathematical finance.
Notes
1 For example, Credit Default Swap (CDS) is a popular credit derivative to enhance the credit ratings of the reference risky assets. Thus, the claim processes of insurers providing CDS protections are related to the financial risks.
References
- Bai, L., & Zhang, H. (2008). Dynamic mean-variance problem with constrained risk control for the insurers. Mathematical Methods of Operations Research, 68(1), 181–205. doi: 10.1007/s00186-007-0195-4
- Bäuerle, N. (2005). Benchmark and mean-variance problems for insurers. Mathematical Methods of Operations Research, 62(1), 159–165. doi: 10.1007/s00186-005-0446-1
- Bi, J., & Guo, J. (2013). Optimal mean-variance problem with constrained controls in a jump-diffusion financial market for an insurer. Journal of Optimization Theory and Applications, 157(1), 252–275. doi: 10.1007/s10957-012-0138-y
- Bi, J., Meng, Q., & Zhang, Y. (2014). Dynamic mean-variance and optimal reinsurance problems under the no-bankruptcy constraint for an insurer. Annals of Operations Research, 212(1), 43–59. doi: 10.1007/s10479-013-1338-z
- Browne, S. (1995). Optimal investment policies for a firm with a random risk process: Exponential utility and minimizing the probability of ruin. Mathematics of Operations Research, 20(4), 937–958. doi: 10.1287/moor.20.4.937
- Chen, S., Li, Z., & Li, K. (2010). Optimal investment–reinsurance policy for an insurance company with var constraint. Insurance: Mathematics and Economics, 47(2), 144–153.
- Chen, P., & Yam, S. C. P. (2013). Optimal proportional reinsurance and investment with regime-switching for mean–variance insurers. Insurance: Mathematics and Economics, 53(3), 871–883.
- Cohen, S. N., & Elliott, R. J. (2008). Solutions of backward stochastic differential equations on Markov chains. Communications on Stochastic Analysis, 2(2), 251–262. doi: 10.31390/cosa.2.2.05
- Cohen, S. N., & Elliott, R. J. (2010). Comparisons for backward stochastic differential equations on Markov chains and related no-arbitrage conditions. The Annals of Applied Probability, 20(1), 267–311. doi: 10.1214/09-AAP619
- Cohen, S. N., & Szpruch, L. (2012). On Markovian solutions to Markov chain bsdes. Numerical Algebra, Control and Optimization, 2(2), 257–269. doi: 10.3934/naco.2012.2.257
- Elliott, R. J., Aggoun, L., & Moore, J. B. (2008). Hidden Markov models: Estimation and control. New York, NY: Springer.
- Hu, Y., & Zhou, X. Y. (2005). Constrained stochastic LQ control with random coefficients, and application to portfolio selection. SIAM Journal on Control and Optimization, 44(2), 444–466. doi: 10.1137/S0363012904441969
- Li, D., & Ng, W. L. (2000). Optimal dynamic portfolio selection: Multiperiod mean-variance formulation. Mathematical Finance, 10(3), 387–406. doi: 10.1111/1467-9965.00100
- Liang, Z., & Bayraktar, E. (2014). Optimal reinsurance and investment with unobservable claim size and intensity. Insurance: Mathematics and Economics, 55, 156–166.
- Markowitz, H. (1952). Portfolio selection. The Journal of Finance, 7(1), 77–91.
- Promislow, S. D., & Young, V. R. (2005). Minimizing the probability of ruin when claims follow Brownian motion with drift. North American Actuarial Journal, 9(3), 110–128. doi: 10.1080/10920277.2005.10596214
- Shen, Y., Wei, J., & Zhao, Q. (2018). Mean–variance asset–liability management problem under non-Markovian regime-switching models. Applied Mathematics and Optimization. doi:10.1007/s00245-018-9523-8.
- Shen, Y., & Zeng, Y. (2015). Optimal investment–reinsurance strategy for mean–variance insurers with square-root factor process. Insurance: Mathematics and Economics, 62, 118–137.
- Siu, T. K., & Shen, Y. (2017). Risk-minimizing pricing and esscher transform in a general non-Markovian regime-switching jump-diffusion model. Discrete and Continuous Dynamical Systems-series B, 22(7), 2595–2626. doi: 10.3934/dcdsb.2017100
- Walter, W. (1998). Ordinary differential equations. New York, NY:Springer-Verlag.
- Wang, H., Wang, R., & Wei, J. (2019). Time-consistent investment-proportional reinsurance strategy with random coefficients for mean–variance insurers. Insurance: Mathematics and Economics, 85, 104–114.
- Wang, T., & Wei, J. (2019). Mean–variance portfolio selection under a non-Markovian regime-switching model. Journal of Computational and Applied Mathematics, 350, 442–455. doi: 10.1016/j.cam.2018.10.040
- Xu, L., Zhang, L., & Yao, D. (2017). Optimal investment and reinsurance for an insurer under Markov-modulated financial market. Insurance: Mathematics and Economics, 74, 7–19.
- Yang, H., & Zhang, L. (2005). Optimal investment for insurer with jump-diffusion risk process. Insurance: Mathematics and Economics, 37(3), 615–634.
- Yi, B., Li, Z., Viens, F. G., & Zeng, Y. (2013). Robust optimal control for an insurer with reinsurance and investment under Heston's stochastic volatility model. Insurance: Mathematics and Economics, 53(3), 601–614.
- Zeng, Y., Li, D., & Gu, A. (2016). Robust equilibrium reinsurance-investment strategy for a mean–variance insurer in a model with jumps. Insurance: Mathematics and Economics, 66, 138–152.
- Zhang, X., & Siu, T. K. (2012). On optimal proportional reinsurance and investment in a Markovian regime-switching economy. Acta Mathematica Sinica, English Series, 28(1), 67–82. doi: 10.1007/s10114-012-9761-7
- Zhou, X. Y., & Li, D. (2000). Continuous-time mean-variance portfolio selection: A stochastic LQ framework. Applied Mathematics and Optimization, 42(1), 19–33. doi: 10.1007/s002450010003
- Zhou, X. Y., & Yin, G. (2003). Markowitz's mean-variance portfolio selection with regime switching: A continuous-time model. SIAM Journal on Control and Optimization, 42(4), 1466–1482. doi: 10.1137/S0363012902405583