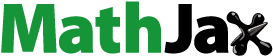
The academic community should be indebted to Professors Jun Cai and Yichun Chi for their meticulously written review paper (referred to as Cai & Chi, Citationin press in what follows) on optimal reinsurance, which summarises and synthesises the historical developments and recent advances in this burgeoning research field. In the current era, where risk management has found more and more applications, this review paper cannot be more timely and will definitely provide the impetus for future research in this and broader areas.
In keeping with Cai and Chi (Citationin press)'s notation and terminology, this discussion aims to complement their review paper by highlighting an important line of recent research and its prospective future directions: The study of risk-measure-based optimal reinsurance contracts with practical constraints. Given an objective functional (a notable example being a distortion risk measure; see Section 5 of Cai & Chi, Citationin press), a reinsurance premium principle, and a set of feasible ceded loss functions, a common theme of research in recent years has been to examine optimal reinsurance treaties in the presence of an external constraint that reflects different kinds of practical considerations. Various types of constraints accounting for a wide range of strategic and operational concerns have been studied in the literature, the most conspicuous ones being:
A reinsurance premium budget constraint, which specifies the amount that the insurer can allocate to reinsurance and reflects the financial limitations facing the insurer, as discussed in Section 5 of Cai and Chi (Citationin press). Such a constraint was imposed in, for example, Cui et al. (Citation2013), Zhuang et al. (Citation2016), and Cheung and Lo (Citation2017), and tackled using different techniques.
A reinsurer risk constraint, which limits the amount of risk (quantified by a certain risk measure) that the reinsurer is willing to be exposed to and recognises the bilateral nature of reinsurance. See, for example, Cheung et al. (Citation2012) and Lo (Citation2017b).
A unifying treatment of different external constraints that can be embedded in a risk-measure-based optimal reinsurance problem is provided in Lo (Citation2017a). Capitalizing on the statistical character inherent in such a constrained problem, Lo (Citation2017a) associated the marginal ceded loss function with the test function (i.e., the probability of rejecting the null hypothesis given the observed data) of an appropriate hypothesis test and studied the following constrained functional minimisation problem:
(1)
(1) where g and h are arbitrary integrable functions defined on the non-negative real line, π is a generic real constant, and f is the ceded loss function to be selected within
, the set of so-called non-decreasing and 1-Lipschitz functions (see Subsection 3.2 of Cai and Chi (Citationin press)). By specifying g and h appropriately, one can retrieve many risk measures and external constraints commonly encountered in practice. Via the use of a variation of the Neyman–Pearson Lemma in statistical hypothesis testing theory, Lo (Citation2017a) solved Problem (Equation1
(1)
(1) ) analytically and expeditiously, and provided a full characterisation of the optimal solutions.
It is imperative to point out that Lo (Citation2017a)'s Neyman–Pearson approach works well when there is a single external constraint, as in Problem (Equation1(1)
(1) ), but lends itself to multiple external constraints only in special cases. In an attempt to impose two constraints in a risk-measure-based optimal reinsurance model, Cai et al. (Citation2016) and Lo (Citation2017a) studied the following weighted Value-at-Risk (VaR) minimisation problem (see Section 6 of Cai & Chi, Citationin press):
(2)
(2) which involves minimising a weighted average of the insurer's VaR and reinsurer's VaR subject to their respective risk constraints, for arbitrary constants
,
,
, and has intrinsic connections to the notion of Pareto-optimal reinsurance contracts. Due to the special properties of the VaR, Problem (Equation2
(2)
(2) ) can be reduced to Problem (Equation1
(1)
(1) ), which carries only one external constraint for some appropriate choices of the functions g and h, depending on the range of values of λ, and thus easily solved by Lo (Citation2017a)'s Neyman–Pearson approach. Lo and Tang (Citation2019) extended Problem (Equation2
(2)
(2) ) to a weighted distortion risk measure minimisation problem and proposed a geometric approach to solving the resulting problem. Nevertheless, both of these weighted average minimisation problems are not entirely generalisations of Problem (Equation1
(1)
(1) ) in that the quantities that define the two constraints also appear in the objective function. Of more theoretical and practical interest is a general two-constraint optimal reinsurance problem of the form
(3)
(3) and its multiple-constraint counterpart
(4)
(4) where
are given integrable functions and
are given constants. Problems (Equation3
(3)
(3) ) and (Equation4
(4)
(4) ) are natural but practically important and mathematically challenging extensions of Problem (Equation1
(1)
(1) ) to two or more constraints. The appeal of these two problems lies in the interplay between the multiple constraints and their joint effect on the optimal solutions. We are particularly interested in whether one constraint will dominate or ‘compete’ with another constraint when it comes to designing the optimal contract for different values of
, and this may be a phenomenon reflecting the relative importance of different operational constraints facing an insurer. Technically, the presence of two or more constraints would invalidate the direct application of Lo (Citation2017a)'s Neyman–Pearson approach. While there are generalisations of the Neyman-Pearson Lemma to the case of multiple constraints in the statistics literature (see, for example, Proposition 6.1 of Shao, Citation2003), they are merely in the form of sufficient conditions rather than complete characterisations of the optimal solutions. To solve Problems (Equation3
(3)
(3) ) and (Equation4
(4)
(4) ) would probably require conceptually different and mathematically more sophisticated techniques, and I am hopeful that Professors Cai and Chi's review paper will provide a springboard for a solution, analytic or numerical, in the not too distant future.
Acknowledgments
Support from a Centers of Actuarial Excellence (CAE) Research Grant (2018–2021) from the Society of Actuaries is gratefully acknowledged. Any opinions, finding, and conclusions or recommendations expressed in this material are those of the author and do not necessarily reflect the views of the SOA.
Disclosure statement
No potential conflict of interest was reported by the author(s).
Additional information
Funding
Notes on contributors
Ambrose Lo
Ambrose Lo is currently Associate Professor of Actuarial Science with tenure at the Department of Statistics and Actuarial Science, The University of Iowa. He earned his B.S. in Actuarial Science and Ph.D. in Actuarial Science from The University of Hong Kong in 2010 and 2014, respectively. He joined The University of Iowa as Assistant Professor of Actuarial Science in August 2014 and was tenured and promoted to Associate Professor in July 2019. His research interests lie in dependence structures, quantitative risk management as well as optimal (re)insurance.
References
- Cai, J., & Chi, Y. (in press). Optimal reinsurance designs based on risk measures: A review. Statistical Theory and Related Fields.
- Cai, J., Lemieux, C., & Liu, F. (2016). Optimal reinsurance from the perspectives of both an insurer and a reinsurer. ASTIN Bulletin, 46(3), 815–849. https://doi.org/10.1017/asb.2015.23
- Cheung, K. C., Liu, F., & Yam, S. C. P. (2012). Average value-at-Risk minimizing reinsurance under Wang's premium principle with constraints. ASTIN Bulletin, 42(2), 575–600. https://doi.org/10.2143/AST.42.2.2182809
- Cheung, K. C., & Lo, A. (2017). Characterizations of optimal reinsurance treaties: A cost-benefit approach. Scandinavian Actuarial Journal, 2017(1), 1–28. https://doi.org/10.1080/03461238.2015.1054303
- Cui, W., Yang, J., & Wu, L. (2013). Optimal reinsurance minimizing the distortion risk measure under general reinsurance premium principles. Insurance: Mathematics and Economics, 53(1), 74–85. https://doi.org/10.1016/j.insmatheco.2013.03.007
- Lo, A. (2017a). A Neyman–Pearson perspective on optimal reinsurance with constraints. ASTIN Bulletin, 47(2), 467–499. https://doi.org/10.1017/asb.2016.42
- Lo, A. (2017b). A unifying approach to risk-measure-based optimal reinsurance problems with practical constraints. Scandinavian Actuarial Journal, 2017(7), 584–605. https://doi.org/10.1080/03461238.2016.1193558
- Lo, A., & Tang, Z. (2019). Pareto-optimal reinsurance policies in the presence of individual risk constraints. Annals of Operations Research, 274(1–2), 395–423. https://doi.org/10.1007/s10479-018-2820-4
- Shao, J. (2003). Mathematical statistics (2nd ed.). Springer.
- Zhuang, S. C., Weng, C., Tan, K. S., & Assa, H. (2016). Marginal indemnification function formulation for optimal reinsurance. Insurance: Mathematics and Economics, 67, 65–76. https://doi.org/10.1016/j.insmatheco.2015.12.003