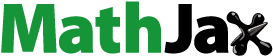
Abstract
In this thesis, we construct test statistic for association test and independence test in high dimension, respectively, and study the corresponding theoretical properties under some regularity conditions. Meanwhile, we propose a nonparametric variable screening procedure for sparse additive model with multivariate response in untra-high dimension and established some screening properties.
With rapid advances of modern technology, high-dimensional data have been frequently collected at relatively low cost in many scientific areas such as microarray analysis, tumour classification, biomedical imaging and finance. This type of data tends to have a dimension comparable to, or much larger than, the sample size. Note that the classical statistical methods are investigated under the scenario where the dimension is fixed. When it comes to high-dimension case, these procedures are challenged simultaneously by the following three perspectives: computational expediency, statistical accuracy and algorithmic stability. Therefore, more and more statisticians are pursuing new methods to address the high-dimensional problems. Under such a circumstance, we conduct our research on high-dimensional problems as follows: high-dimensional association test, nonparametric variable screening in ultra-high dimension and independence test for high-dimensional data. By investigating the existing approaches, we construct some new statistic and further establish the corresponding asymptotic theories.
The first chapter introduces the research background of this thesis, and further summarises the innovations given in this thesis.
The second chapter of this thesis is about high-dimensional association test. Here the hypothesis we are interested in is
where denotes the covariance matrix of
and
. The fact that
only implies the absence of linear relationship rather than independence between random vectors
and
(except when they are from a multivariate normal distribution). This test problem can also be expressed equivalently as
where
is the trace of a matrix, and
is called the ‘covariance’ of random vectors
and
in Escoufier (Citation1973). It is worth noting that Székely et al. (Citation2007) defined the distance covariance with the Euclidean distance. It is easy to obtain
when we replace the Euclidean distance with half of its square.
is thus proposed as an unbiased estimator of
. Based on
, a new test statistic
is introduced for association test in high dimension. This proposed test procedure enjoys three characteristics as follows. First, it has a wide scope of practical application. That is, it only requires that p + q tends to infinity, which contains two scenarios: On the one hand, p and q can diverge at the same time, on the other hand, only p or q diverges. Second, it expands the theoretical results of Srivastava and Reid (Citation2012) and Li et al. (Citation2017). Both of these two papers assume that the vector
is from a multivariate normal distribution. Furthermore, the asymptotic distribution under the local alternative is out of their consideration in these two articles. In this part, we obtain the limiting distribution under bothr the null hypothesis and the local alternative without imposing the assumption that
is from a multivariate normal distribution. Specially, on one hand, when
and
are independent, the proposed test statistic
converges to the standard normal
in distribution. On the other hand,
converges to
in distribution under the local alternatives. Third, we describe the assumptions given in the theorems under some particular model structure. This helps us to have a more intuitive understanding of these conditions.
The third part of this thesis is about nonparametric variable screening in ultrahigh-dimensional additive models. Due to the absence of a priori information about the model structure, a more flexible class of nonparametric models such as the additive model can be used to significantly increase the flexibility of parametric models, especially for the ultrahigh-dimensional data with much challenge to check model assumptions. Inspired by Fan et al. (Citation2011), we propose a nonparametric screening procedure based on RV correlation constructed in Escoufier (Citation1973). This procedure works as follows: for each predictor , we obtain a normalised B-spline basis
and compute the corresponding RV correlation
between the multivariate response
and this basis
. Then we rank the importance of
according to the RV (correlation of vectors) correlation
. The screening procedure enjoys two advantages from both practical and theoretical viewpoints. First, it can be directly applied to multivariate additive model, which makes additive models much more applicable. Second, the theoretical properties of the proposed screening measure, such as Sure Screening Property, False Selection Rate and Ranking Consistency Property, are obtained under some regularity conditions. Furthermore, to enhance its finite sample performance, two iterative feature screening procedures are also proposed.
Testing the independence between the random vectors and
is of importance in both statistical theory and applications. Thus, the fourth chapter of this thesis is about independence test in high dimension. Székely et al. (Citation2007) proposed
, the distance correlation between random vectors
and
, to measure all types of dependence between random vectors in arbitrary, not necessarily with equal dimensions. In other word,
is zero if and only if
and
are independent. Furthermore, they established the asymptotic properties of the proposed test statistic when the dimension is fixed. Later, Székely and Rizzo (Citation2013) discovered that, as p, q tend to infinity, the empirical distance correlation of the two vectors
and
converges to one even though they are independent. Therefore, the two authors extended the distance correlation with a modified version in high dimension. They introduced a new test statistic based on the modified distance correlation. They also derived that this statistic converges to Student t, as dimensions tend to infinity. Again using the modified distance correlation, we construct a new test statistic and obtain its limiting properties. This test procedure in this part has four features as follows. First only one dimension diverges. Without loss of generality, we assume the dimension p tends to infinity and the dimension q is fixed. This scenario can be seen as an extension of Székely and Rizzo (Citation2013) in practical applications. Second, the asymptotic distribution of our proposed test statistic is established under null hypothesis and the local alternative hypothesis, which generalises the work of Székely and Rizzo (Citation2013) in statistical theory. Third, to address the problem of ‘the curse of dimensionality’, we adopt the power enhancement technique proposed in Fan et al. (Citation2015) to boost the empirical power of our test even in high dimension. The simulation results show that our proposed method outperform some existing ones in empirical power. Last, similar to that in the first part of this thesis, some computation results of the assumptions imposed in the theorems are given under some particular models, which may shed light on the assumptions.
The fifth chapter summarises the work done in the thesis and shows some directions of the relevant future work.
Disclosure statement
No potential conflict of interest was reported by the authors.
Additional information
Notes on contributors
Yongshuai Chen
Yongshuai Chen is a young teacher of Capital University of Economics and Business.
Hengjian Cui
Hengjian Cui is a Professor of Capital Normal University.
References
- Escoufier, Y. (1973). Le traitement des variables vectorielles. Biometrics, 29(4), 751–760. https://doi.org/10.2307/2529140
- Fan, J., Feng, Y., & Song, R. (2011). Nonparametric independence screening in sparse ultra-high-dimensional additive models. Journal of the American Statistical Association, 106, 544–557. https://doi.org/10.1198/jasa.2011.tm09779
- Fan, J., Liao, Y., & Yao, J. (2015). Power enhancement in high-dimensional cross-sectional tests. Econometrica, 83(4), 1497–1541. https://doi.org/10.3982/ECTA12749
- Li, W., Chen, J., & Yao, J. (2017). Testing the independence of two random vectors where only one dimension is large. Statistics, 51(1), 141–153. https://doi.org/10.1080/02331888.2016.1266988
- Srivastava, M. S., & Reid, N. (2012). Testing the structure of the covariance matrix with fewer observations than the dimension. Journal of Multivariate Analysis, 112, 156–171. https://doi.org/10.1016/j.jmva.2012.06.004
- Székely, G. J., & Rizzo, M. L (2013). The distance correlation t-test of independence in high dimension. Journal of Multivariate Analysis, 117, 193–213. https://doi.org/10.1016/j.jmva.2013.02.012
- Székely, G. J., Rizzo, M. L., & Bakirov, N. K. (2007). Measuring and testing independence by correlation of distances. Annals of Statistics, 35(6), 2769–2794. https://doi.org/10.1214/009053607000000505