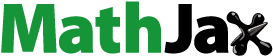
High-dimensional data are frequently collected in a large variety of areas such as biomedical imaging, functional magnetic resonance imaging, tomography, tumor classifications, and finance. With recent explosion of scientific data of unprecedented size and complexity, feature ranking and screening are playing an increasingly important role in many scientific studies. There are many significant breakthroughs in this filed. Starting from Zhu et al. (Citation2011), the first paper on model free marginal screening, Li et al. (Citation2020) provided a thorough review of sparse sufficient dimension reduction.
Both marginal Dantzig selector (Yu, Dong, & Shao,Citation2016) and forward trace pursuit (Yu, Dong, & Zhu, Citation2016) can be used for feature screening. Marginal screening is however the first step of forward trace pursuit. Trace pursuit is to connect marginal screening with forward regression. It is also worth mentioning that forward trace pursuit can be used as an initial screening step to speed up computation in the case of ultrahigh dimensionality. Compared with existing screening method in the literature, trace pursuit has a lot of advantages. For details, one can refer to Yu, Dong, and Zhu (Citation2016).
Tan et al. (Citation2020) established the mini-max lower bound for sparse sliced inverse regression (SIR for short), which has never been done in the area of sparse sufficient dimension reduction. Thinking that an optimal estimation of sparse SIR is computationally intractable, they proposed the computationally feasible counterpart for sparse SIR, which however can not maintain the optimal rate. To overcome this issue, Tan et al. (Citation2020) proposed a refined sparse SIR estimate, which is rate-optimal yet computational intractable. However, they proved that the computationally feasible counterpart based on the adaptive estimation procedure is nearly rate-optimal. Lasso-SIR (Lin et al., Citation2019) was shown to be rate optimal only when , however, the sparse SIR approach is rate optimal even when
. Therefore, the sparse SIR estimator certainly possesses a much wider range of applications, which, in my view, is a big breakthrough in sparse sufficient dimension reduction.
Disclosure statement
No potential conflict of interest was reported by the author(s).
References
- Li, L., Wen, X. M., & Yu, Z. (2020). Review of sparse sufficient dimension reduction. Statistical Theory and Related Fields.
- Lin, Q., Zhao, Z., & Liu, J. (2019). Sparse sliced inverse regression via Lasso. Journal of the American Statistical Association, 114(528), 1726–1739. https://doi.org/10.1080/01621459.2018.1520115
- Tan, K., Shi, L., & Yu, Z. (2020). Sparse SIR: Optimal rates and adaptive estimation. The Annals of Statistics, 48(1), 64–85. https://doi.org/10.1214/18-AOS1791
- Yu, Z., Dong, Y., & Zhu, L. X. (2016). Trace pursuit: A general framework for model-free variable selection. Journal of the American Statistical Association, 111(514), 813–821. https://doi.org/10.1080/01621459.2015.1050494
- Yu, Z., Dong, Y., & Shao, J. (2016). On marginal sliced inverse regression for ultrahigh dimensional model-free feature selection. The Annals of Statistics, 44(6), 2594–2623. https://doi.org/10.1214/15-AOS1424
- Zhu, L. P., Li, L., Li, R., & Zhu, L. X. (2011). Model-free feature screening for ultrahigh-dimensional data. Journal of the American Statistical Association, 106(496), 1464–1475. https://doi.org/10.1198/jasa.2011.tm10563