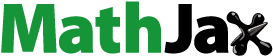
We congratulate the authors on a very interesting overview of sparse sufficient dimension reduction (SDR). Sparse SDR methods are discussed in both the classical n>p setting as well as the high-dimensional p>n setting. Related topics such as model-free variable selection and variable screening are also discussed in a most logical fashion. Last but not least, two new methodological contributions are made in this review paper. Namely, new variable screening methods are proposed as extensions of Yu et al. (Citation2016), and novel sparse SDR methods are discussed following the sparse SIR in Tan et al. (Citation2020).
While all the methods discussed in this review are in the frequentist domain, our comment will focus on sparse SDR through Bayesian methods. Reich et al. (Citation2011) proposed an SDR approach via Bayesian mixture modelling. Take single-index model as an example. Let be an i.i.d. sample from
. Let
be a basis for the central subspace and let
be the sufficient predictor. Then the conditional distribution of
given
can be modelled as
(1)
(1) where there are K normal mixture components and
denotes the weight of the kth component. By choosing the weights carefully, model (Equation1
(1)
(1) ) can be expressed as
Here
is a latent continuous variable,
are cutpoints. By placing a prior on
and the cutpoints, one can compute the conditional distributions and carry out the full Bayesian analysis. For SDR without sparsity, the prior for
is set as
,
. To introduce sparsity, a two-component mixture prior is assumed as
where 0<c<1 is a fixed constant and
is the prior inclusion probability. If
, then the jth predictor is included in the model. Otherwise the jth predictor is removed from the model. Reich et al. (Citation2011) also discussed a similar Bayesian mixture model for the multiple-index model, and the details are omitted.
Motivated by a frequentist SDR method recently proposed by Fang and Yu (Citation2020), Power and Dong (Citation2020) proposed a new Bayesian approach for sparse sufficient dimension reduction. Let ,
, and denote
as a partition for the support of Y. The classical sliced inverse regression (SIR) (Li, Citation1991) uses the kernel matrix
, where
with
and
for
. Note that
can be solved as an optimisation problem
(2)
(2) Fang and Yu (Citation2020) then applied the Mallows model averaging (MMA) of Hansen (Citation2007) to solve the least squares problem (Equation2
(2)
(2) ). Power and Dong (Citation2020) utilised Bayesian model averaging (BMA) (Raftery et al., Citation1997) to solve (Equation2
(2)
(2) ) instead. Similar to MMA, BMA works well for sparse models and may also adapt to models with dense signals. Furthermore, instead of solving for
,
, individually, we may solve for them jointly. Let
and
. Then we have
(3)
(3) To the best of our knowledge, there is no frequentist model averaging approach to solve (Equation3
(3)
(3) ). On the other hand, multi-response BMA (Brown et al., Citation1998) can be easily adapted to solve (Equation3
(3)
(3) ). As shown in Power and Dong (Citation2020), the multi-response BMA outperforms the frequentist MMA for SDR.
We congratulate the authors again for providing a stimulating review of existing sparse SDR techniques, which should motivate further development of new SDR methods. It is our belief that more Bayesian approaches may be applied for this cause.
References
- Brown, P. J., Vannucci, M., & Fearn, T. (1998). Multivariate Bayesian variable selection and prediction. Journal of the Royal Statistical Society: Series B (Statistical Methodology), 60(3), 627–641. https://doi.org/10.1111/rssb.1998.60.issue-3 doi: 10.1111/1467-9868.00144
- Fang, F., & Yu, Z. (2020). Model averaging assisted sufficient dimension reduction. Computational Statistics and Data Analysis, 152. https://doi.org/10.1016/j.csda.2020.106993
- Hansen, B. E. (2007). Least squares model averaging. Econometrica, 75(4), 1175–1189. https://doi.org/10.1111/ecta.2007.75.issue-4 doi: 10.1111/j.1468-0262.2007.00785.x
- Li, K. (1991). Sliced inverse regression for dimension reduction. Journal of the American Statistical Association, 86(414), 316–327. https://doi.org/10.1080/01621459.1991.10475035
- Power, M. D., & Dong, Y. (2020). Bayesian model averaging sufficient dimension reduction. Statistics and Probability Letters. Submitted.
- Raftery, A. E., Madigan, D., & Hoeting, J. A. (1997). Bayesian model averaging for linear regression models. Journal of the American Statistical Association, 92(437), 179–191. https://doi.org/10.1080/01621459.1997.10473615
- Reich, B. J., Bondell, H. D., & Li, L. (2011). Sufficient dimension reduction via Bayesian mixture modeling. Biometrics, 67(3), 886–895. https://doi.org/10.1111/biom.2011.67.issue-3 doi: 10.1111/j.1541-0420.2010.01501.x
- Tan, K., Shi, L., & Yu, Z. (2020). Sparse SIR: optimal rates and adaptive estimation. The Annals of Statistics, 48(1), 64–85. https://doi.org/10.1214/18-AOS1791
- Yu, Z., Dong, Y., & Shao, J. (2016). On marginal sliced inverse regression for ultrahigh dimensional model-free feature selection. The Annals of Statistics, 44(6), 2594–2623. https://doi.org/10.1214/15-AOS1424