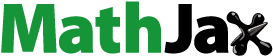
Abstract
Although advanced statistical models have been proposed to fit complex data better, the advances of science and technology have generated more complex data, e.g., Big Data, in which existing probability theory and statistical models find their limitations. This work establishes probability foundations for studying extreme values of data generated from a mixture process with the mixture pattern depending on the sample length and data generating sources. In particular, we show that the limit distribution, termed as the accelerated max-stable distribution, of the maxima of maxima of sequences of random variables with the above mixture pattern is a product of three types of extreme value distributions. As a result, our theoretical results are more general than the classical extreme value theory and can be applicable to research problems related to Big Data. Examples are provided to give intuitions of the new distribution family. We also establish mixing conditions for a sequence of random variables to have the limit distributions. The results for the associated independent sequence and the maxima over arbitrary intervals are also developed. We use simulations to demonstrate the advantages of our newly established maxima of maxima extreme value theory.
1. Introduction
Rigorous risk analysis helps to make better decisions and prevent great failures. Extreme value theory has been a powerful tool in risk analysis and is widely applied to risk analysis in finance, insurance, health, climate, and environmental studies. In classical extreme value theory, the sequence of data is assumed to have the same marginal distribution, and the limit distribution of the maxima is in one of the extreme value types if it exists. Galambos (Citation1978), de Haan (Citation1993), Beirlant et al. (Citation2004), de Haan and Ferreira (Citation2006), Leadbetter et al. (Citation2012) and Resnick (Citation2013) amongst many monographs are good literatures introducing the theoretical results in the classical extreme value theory. Mikosch et al. (Citation1997), Embrechts et al. (Citation1999), McNeil and Frey (Citation2000), Coles (Citation2001), Finkenstädt and Rootzén (Citation2004), Castillo et al. (Citation2005), Salvadori et al. (Citation2007) and Dey and Yan (Citation2016) introduce many applications of extreme value method to the areas of science, engineering, nature, finance, insurance and climate. For example, in financial applications, extreme value theory is one of the tools to calculate the Value-at-Risk (VaR) and Expected Shortfall (ES) (e.g., Rocco, Citation2014; Tsay, Citation2005). Chavez-Demoulin et al. (Citation2016) offer an extreme value theory (EVT)-based statistical approach for modelling operational risk and losses, by taking into account dependence of the parameters on covariates and time. Zhang and Smith (Citation2010) propose the multivariate maxima of moving maxima (M4) processes and apply the method to model jumps in returns in multivariate financial time series and predict the extreme co-movements in price returns. Daouia et al. (Citation2018) use the extreme expectiles to measure VaR and marginal expected shortfall. In the statistical inference of maximum likelihood estimation (MLE), a discussion on the properties of maximum likelihood estimators of the parameters in generalised extreme value (GEV) distribution was given by Smith (Citation1985). In the paper, it is shown that the classical properties of the MLE hold when the shape parameter , but not when
. Bücher and Segers (Citation2017) give a general result on the asymptotic normality of the maximum likelihood estimator for parametric models whose support may depend on the parameters.
In the age of Big Data, the advances of science and technology have been changing data generating processes in a more complex way. As a result, the data structures and dependence structures accompanied by the collected data can be very different from the existed assumptions in many commonly used models. In the literature, advanced statistical models and machine learning approaches have been proposed to fit such complex data or learn the underlying structures better. For example, the support vector machine, the deep learning method, and the random forest method have now been very well recognised and wildly used in data analysis. In extreme value analysis for more complex data, the same marginal distribution assumption and its derived extreme value distributions can be very restrictive and lack of data fitting power. Although statistical models, e.g., Heffernan et al. (Citation2007), Naveau et al. (Citation2011), Tang et al. (Citation2013), Malinowski et al. (Citation2015), Zhang and Zhu (Citation2016) and Idowu and Zhang (Citation2017), have been proposed to model extreme values observed from different data sources with different populations and max-domains of attraction, their probability foundations have not been established.
The definition of the classical maximum domain of attraction cannot be applied directly to the extreme values of data drawn from different populations mixed together. Note that we are not dealing with mixtures of distributions that may belong to a maximum domain of attraction of classical extreme value distribution. In this study, we are dealing with maxima of maxima in which the maxima resulted from each population has its limit extreme value distribution and norming and centering constants and convergence rate. For example, in many real-world applications, the risks one is exposed to usually come from different resources, and the risk at a given time is decided by the dominant one, i.e., not the added risk of all risks. Let us consider a specific example: Suppose a patient suffers two severe diseases. The risk of that the patient will die over a certain time may be best described by the maximum, not the sum, of two risk variables.
This work extends the definition of the maximum domain of attraction to maxima of maxima of sequences of random variables in which the mixing patterns change along with the sample size. The accelerated max-stable distribution (accelerated extreme value distribution) is expressed as a product of the classical extreme value distributions for the maxima of maxima resulted from different distributions. Some basic properties and theoretical results are provided. It can be seen that the classical extreme value distributions are special cases of our newly established family of accelerated max-stable distributions. The results obtained can be applied to more complex data, e.g., Big Data. The new results also establish the probability foundation of previously proposed statistical models in extreme time series modeling. Those models include Heffernan et al. (Citation2007) that introduces one scheme where the maxima are taken over random variables with different distributions, and Zhang and Zhu (Citation2016) that models intra-daily maxima of high-frequency financial data.
The structure of this paper is as follows. In Section 2, (1) we give a brief review of the classical extreme value theory; (2) we define our maxima of maxima of sequences of random variables; (3) we use examples to demonstrate the characteristics of the maxima of maxima; (4) we establish the convergence of maxima of maxima to the accelerated max-stable distributions; (5) we illustrate density functions of the new family of accelerated max-stable distributions and evaluate moments and tail equivalence. Simulations are used to demonstrate the advantages of the accelerated max-stable distribution family in terms of the estimation accuracy of high quantiles at different levels. We also apply this new accelerated max-stable distribution to the high quantiles of the daily maxima of 330 stock returns of S&P 500 companies. In Section 3, the convergence of joint probability for general thresholds and approximation errors are developed. In Section 4, theoretical results for weakly dependent sequences are derived. Section 6 concludes. Additional figures and technical proofs are included in the Appendix.
2. Accelerated max-stable distribution for independent sequences
2.1. A brief review of classical univariate extreme value theory
In classical extreme value theory, the central result is the Fisher-Tippett theorem which specifies the form of the limit distribution for centered and normalised maxima. Let be a sequence of independent and identically distributed (i.i.d.) non-degenerate random variables (rvs) with common distribution function F and
be the sample maxima. The Fisher-Tippett theorem states that: If for some norming constants
and centering constants
we have
(1)
(1) for some nondegenerate H, where
stands for convergence in distribution, then H belongs to one type of the following three cumulative distribution functions (cdf's):
(2)
(2) Conversely, every extreme value distribution in (Equation2
(2)
(2) ) can be a limit in (Equation1
(1)
(1) ), and in particular, when H itself is the cdf of each
, the limit is itself. We say that F belongs to the maximum domain of attraction of the extreme value distribution of H, and denote as
when (Equation1
(1)
(1) ) holds. H is also called the max-stable distribution since for any
, there are constants
and
such that
. Due to this property, the equivalence of extreme value distribution or max-stable distribution in practice is mutually implied.
2.2. Maxima of maxima
Suppose that the independent mixed sequence of random variables is composed of k subsequences
;
,
as
and
. Denote
as the maximum of the jth subsequence,
. Suppose
, where
is one of the three types of extreme value distributions, i.e.,
has the following limit distribution with some norming constants
and centering constants
(3)
(3) Define
, i.e.,
is the maxima of k maxima of
s. Throughout the paper,
is termed as the maxima of maxima. Questions can be asked: (1) whether or not Equation (Equation1
(1)
(1) ) holds with appropriately chosen norming constants
; (2) if Equation (1) holds, whether or not
are equivalent to any of
; (3) whether or not
is a function of
; (4) if all Equations (1)–(3) hold, which one is the best method to be used in practice. This paper intends to answer these four questions.
Practical examples related to the above defined process can be numerous. For example, (1) the maximum temperature of the US in a day can be described by the maximum of maxima of regional maximum temperatures. In each region, the maximum temperature is the maximum temperature recordings among all weather stations in the region. Considering the regions' spatial and geographical patterns, the regional maxima certainly follow different extreme value distributions from one region to another region. The US temperature maxima are the maxima of regional maxima, and should be modelled by a distribution function that is a function of the regional extreme value distribution functions. (2) Considering the daily risk of high-frequency trading in a stock market, one can partition the data into hourly data (from 9:00 am to 4:00 pm). Suppose each hourly maxima of negative returns can be approximately modelled by an extreme value distribution of
. It is clear that
is better modelled by a function of
i.e., not a single
. We use the following simple example with k = 2 to illustrate the idea.
Example 2.1
The sequence is generated by
, where
,
, and
and
are two distribution functions. Assume
and
are independent. Then
.
Remark
The form is the simplest case in the general mixture models introduced in Zhao and Zhang (Citation2018). It is also the simplest case in the copula structured M4 models studied by Zhang and Zhu (Citation2016).
For illustrative purpose of Example 2.1, let's consider two scenarios. Suppose and
for
. Here
represents the uniform distribution on the interval
. The superscript
stands for the kth sample sequence. In Scenario 1, Figure illustrates two different simulated sequences of
, where
, and the maxima of
for n = 100 and a particular k, e.g., k = 1. Next, we repeatedly generate
such sequences
. By taking the maxima
, the histogram of
is displayed in Figure (a) with a = −2.2 and b = 2.2. In Scenario 2, by replacing the marginal distribution of
with
, the histogram of
is shown in Figure (b). It is clear that although
is independent and identically distributed (i.i.d.), one can see that the distribution of
looks quite different from the three types of extreme value distributions.
Figure 1. Simulated mixed sequences from normal and uniform distributions and their maxima (marked with black dots). In (a), the maximum is from the uniform distribution; in (b), the maximum is from .
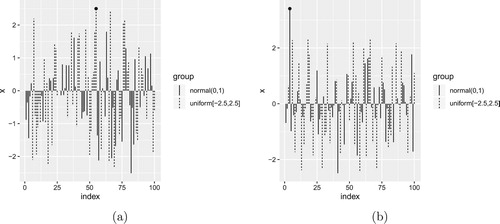
In Example 2.1, the larger values of two paired underlying subsequences are observed while the smaller values are covered up by larger ones and are never observed. The sample sizes from the two subsequences are the same. However, in general mixed sequences the ratios of sample sizes from two subsequences can be any value between 0 and infinity and can vary as the total sample size grows. As a result, we can see many kinds of different patterns different from Figure .
In practice, data generating processes are naturally formed spatially and temporarily from underlying physical processes of studies. Here we provide two data generating processes in simulation.
For a given sample size n, we set the numbers
and
satisfying
and assume that
,
. Then we generate the specified
and
observations from two populations respectively, stack them in a sequence, and perform a random permutation of the combined sequence. In a physical process, the procedure can be designed as: we mix
yellow balls and
white balls in a bag. Then we draw balls sequentially. If a yellow ball is drawn, generate a number from the first population, otherwise from the second population.
Alternatively, suppose
,
are norming constants defined in Equation (Equation3
(3)
(3) ) with known population distributions in simulation, we can set
and let
, and then solve
and
to generate the observations as the last step.
Example 2.2
Using the sampling scheme designed above. Suppose there are two sequences and
with
and
.
is mixed with these two sequences. Let
be the maxima of the jth realisation of the sequence,
, n = 300. With
,
are calculated and the histogram is shown in Figure (a). The case of
and
is shown in Figure (b).
The histograms in Figure look different from any of the three types of extreme value distributions discussed in (Equation2(2)
(2) ). One feature is that they can be bimodal. On the other hand, the classical GEV distributions are all unimodal. Figure shows two specific examples of choices of
and
. In more general situations, the ratios of
and
can be any values in
. The ratio
may also change as n increases. In Figures and , the left parts of the distributions are dominated by the Weibull type induced by the uniform distribution, and the right parts resemble the Gumbel type induced by the normal distribution. The reason is that when we look at the maxima of
, there are two populations competing with each other. Taking (b) in Figure as an example, the winners from
form the steep peak on the left; and the winners from
form the smoother peak on the right.
Figure (a) shows the distribution of for the sequence which is mixed with
and a Fréchet distribution. In (b), (c) and (d), they show the combinations of one Fréchet distribution and one Weibull distribution. Notice that in panel (b), the distribution looks left-skewed and is very similar to a Weibull distribution. However, with the effect of the Fréchet distribution, it actually has an infinite right endpoint.
Figure 4. Histograms of . (a)
and Fréchet combination. (b)–(d) Some combinations of Fréchet and Weibull.
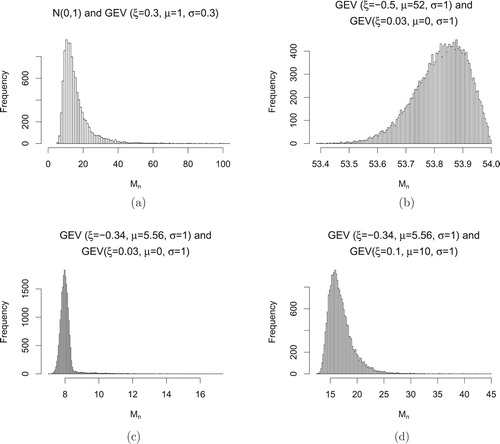
In Figure , histograms of are created such that the independent sequences of random variables
are generated by comparing the pairs of observations from normal and Weibull distribution. They can be unimodal or bimodel, left-skewed or right-skewed. If we use the GEV family to characterise the distributions of
in these examples, it may not capture the shape of the distribution properly. For example, if we look at the left part of the distribution in Figure (d), it resembles a Weibull distribution that has a finite right endpoint. However, because of the effect of the normal distribution on the right tail, the shape changes suddenly to be similar to a Gumbel distribution with infinite right endpoint. If we fit a GEV distribution to
, the left part with more sample data may have a large effect on the fitted distribution and we may underestimate the long tail on the right.
2.3. Convergence to the accelerated max-stable distribution
Throughout the paper, is the right endpoint of a cdf F and let
;
is restricted to k = 2. For k>2, relative results can be derived with additional notations. The following theorem shows that under certain conditions on the norming constants
and
, we can choose one set of the norming constants for the global maximum
to derive its limit distribution. Theorem 2.1 can be directly derived from Khintchine's theorem.
Theorem 2.1
If and
satisfy (Equation3
(3)
(3) ) for j = 1, 2, the limit distribution of
as
can be determined in the following cases:
Case 1. | If | ||||
Case 2. | If |
Notice that the limit in Case 1 is the product of two extreme value distributions, . Although it is in the product form, sometimes it can still be reduced to the three classical extreme value distributions. For example,
is still a Fréchet type. However, in some situations, when the conditions in Case 1 are satisfied, the limit product form cannot be reduced to any one of the three extreme value distributions. We next present several examples to illustrate these possibilities.
Example 2.3
Fréchet and Gumbel
Suppose is a Fréchet distribution function, and
is the standard Gumbel distribution function. By choosing
,
we have
(6)
(6) Then when
, we have
Example 2.4
Fréchet and Fréchet
Suppose and
are two Fréchet distribution functions such that
, which means that the tail of
is heavier than the tail of
. By choosing norming constants
,
and
,
we have
(7)
(7) and
(8)
(8) If
, then
(9)
(9) If
, then
(10)
(10)
In Example 2.4, the sequence is mixed with two Fréchet distributions with different shape parameters. The limit distribution of for this mixed sequence is the product of two Fréchet distributions, which is different from any of the three types of extreme value distributions.
Example 2.5
Uniform and normal
Suppose is the function of the uniform distribution
,
is the distribution function of
. By choosing
(11)
(11) and
we have
(12)
(12) for x<0, and
(13)
(13) Then
Since
for any x, we have
(14)
(14)
Example 2.6
Weibull and Weibull
Suppose and
,
(15)
(15)
(16)
(16) are two polynomial functions with common finite endpoint
,
. We can choose
,
,
,
, and
(17)
(17)
(18)
(18) If
, then
Example 2.7
Normal and Pareto
Suppose is the standard normal distribution function of
,
,
, K>0 is a Pareto distribution function. Let
Then
Furthermore, if
, then
Example 2.8
Cauchy and uniform distribution
is the standard Cauchy distribution function, and
, let
Then
and
In Example 2.8, the limit distribution for the normalised is 0 when x<0, and the limit distribution for the normalised
is 1 when x>0. Thus, the product is the same as the former one.
In Examples 2.3 and 2.5, we showed that when n is sufficiently large (goes to infinity), the distribution of will be dominated by the subsequence whose marginal distribution has a heavier tail. In Examples 2.4 and 2.6, if the ratio
converges to a constant, then one subsequence is never dominated by another, and the limit is of the product form that cannot be reduced to a classical extreme value distribution if
.
We now introduce the accelerated max-stable distribution (AMSD) or the accelerated extreme value distribution (AEVD). We consider the convergence of the probability related to the normalised maxima and
of two subsequences separately. By the relationship
, we can use the accelerated max-stable distribution to approximate the distribution of
. The classical extreme value distributions will be special cases in the accelerated max-stable distribution family.
Definition 2.1
Let and
be two max-stable distribution functions, we call
the accelerated max-stable distribution (AMSD/AEVD) function, which is the product of two max-stable distribution functions. More generally, we also say that
belongs to the accelerated max-stable distribution family if it is the product of k max-stable distribution functions,
.
Remark
If Z follows an accelerated max-stable distribution , then Z can be expressed as
, where each
follows a max-stable distribution. By taking maxima of
,
values are accelerated by other components
s to get observed Z values. On the other hand, we have
and
where
stands for the survival function, i.e.,
The above inequalities may be regarded as accelerated survival rates. This observation motivates us to call the new distribution as the accelerated max-stable (extreme value) distribution. In the view of risk analysis, the systemic risk of Z is accelerated from individual risks of
s given a fixed confidence level.
For the independent sequence of random variables with two subsequences
and
defined as above, suppose (Equation3
(3)
(3) ) is satisfied with j = 1, 2 and norming constants
, i.e.,
(19)
(19) then
(20)
(20)
Definition 2.2
Suppose an independent sequence of random variables satisfies (Equation19
(19)
(19) ) and (Equation20
(20)
(20) ). We call the underlying distribution,
, of
belongs to the competing-maximum domain of attractions of
and
, and denote as
.
We note that a max-stable distribution may also be decomposed into a product of two max-stable distributions. As a result, the max-stable distribution family can be thought as a family that is embedded in the accelerated max-stable distribution family. This observation can be seen in Theorem 2.1 that the limits of under two different conditions belong to the accelerated max-stable distribution family. In other words, the accelerated max-stable distributions form an expanded family of distributions that can describe the limiting distribution of the normalised maxima for more general sequences.
For k = 2 and , AMSDs/AEVDs can have the following six possible combinations:
Case 1. |
| ||||
Case 2. |
| ||||
Case 3. |
| ||||
Case 4. |
| ||||
Case 5. |
| ||||
Case 6. |
|
It is easy to see that the classical extreme value distributions are special cases of the AMSD family. For any a>0, b>0 satisfying we have
Since
and
are max-stable distributions, for any
and
, there are constants
,
,
,
such that
.
In Equation (Equation20(20)
(20) ), we considered the convergence of
instead of the traditional
. If
and
are sufficiently large, by (Equation19
(19)
(19) ) we have
and
, then
(21)
(21) where
is of the same type as
, j = 1, 2.
To close this section, we remark that (Equation21(21)
(21) ) is the basis of applying the newly introduced AMSD/AEVD family to real data. Based on (Equation21
(21)
(21) ), in practice, we don't need to worry about the values of
,
,
,
,
,
, as they are absorbed in
and
, see also Coles (Citation2001). In our examples, we have used some fixed numbers for
and
. They are just for simulation convenience. When n tends to infinity, the values of
and
will depend on n.
The next section presents density functions and shapes from which one can see the flexibility of applying the new distribution to real data modelling.
2.4. Density functions and density plots
The density function of the accelerated max-stable distribution requires some discussion of the support region of the cumulative distribution function. We can express the two terms in the product using the form of the generalised extreme value distribution,
(22)
(22) where
and
. We include the special case
as the limit of
for
. Denote the density function as
and let
Since
and
are symmetric, we only present one of them. We have the following six cases for the density functions.
Case 1. |
| ||||
Case 2. |
| ||||
Case 3. |
| ||||
Case 4. |
| ||||
Case 5. |
| ||||
Case 6. |
If |
In Figures and , four density plots of Weibull-Gumbel type are shown. In Figure , panel (a) is the density plot of Fréchet-Fréchet type; and panel (b) is the density plot of Fréchet-Gumbel type. We can observe that they capture the shapes of the histograms shown in Figures and .
Figure 6. Density plots of the accelerated max-stable distributions with Weibull-Gumbel combinations. (a) ,
,
,
,
,
. (b)
,
,
,
,
,
.
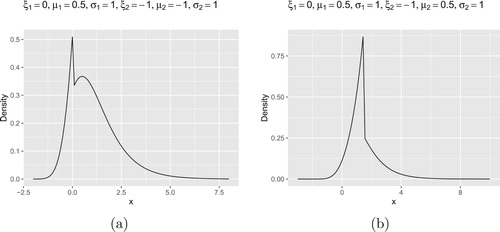
Figure 7. Density plots of the accelerated max-stable distributions with Weibull-Gumbel combinations. (a) ,
,
,
,
,
. (b)
,
,
,
,
,
.
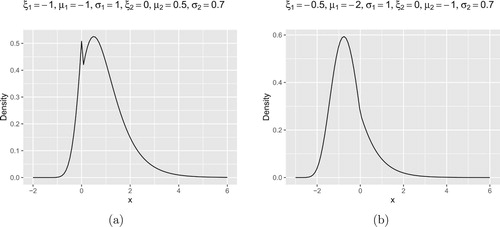
Figure 8. (a) Density plot of the accelerated max-stable distribution with Fréchet-Fréchet combinition. ,
,
,
,
,
. (b) Density plot of the accelerated max-stable distributions with Fréchet-Gumbel combinition.
,
,
,
,
,
.
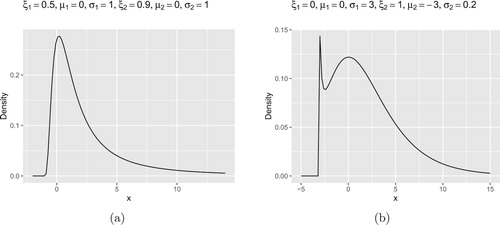
In Figure (b), it is for , i.e., the combination of two Gumbel distributions. In this case, the density plot is bimodal, which is different from that of a Gumbel distribution. Suppose that
and
,
and
, then we have some norming constants
and
such that
(23)
(23) Here the limit product form requires that the two scale parameters
. Otherwise, the product
reduces to the Gumbel type.
2.5. Tail equivalence and the existence of moments
In this section, we discuss some results of tail-equivalence, and which moments are finite for certain AMSDs/AEVDs.
Definition 2.3
Two cdf's F and H are called tail-equivalent if they have the same right endpoint, i.e., if , and
(24)
(24) for some constant
.
We have the following facts.
Fact 2.1
It is clear that the product distribution of a Weibull distribution and another type of extreme value distribution is tail equivalent to
.
Fact 2.2
Suppose , let
be the kth moment of X, then
is finite only if
.
Suppose , then
has a heavier tail than
. Let
be the kth moment of
. We know that
only if
. This implies that
has the same right-tail heaviness as
.
Fact 2.3
If , then
and
are tail-equivalent.
Fact 2.4
Suppose . Let
be the kth moment of X. Then
is finite only if
.
Fact 2.5
and
are tail-equivalent.
Fact 2.6
If has a heavier tail than
, then the accelerated max-stable distribution
is tail-equivalent to
.
3. Joint convergence and approximation errors
3.1. Convergence of joint probability for general thresholds
It may also be interesting to consider the limits of for some sequences
and
not necessarily of the form
or even not dependent on x. Here
and
are the lengths of the two subsequences, we may write them specifically as
and
since they vary with the total length n. When choosing
for j = 1, 2, it becomes the problem we discussed before. The question is:
Which conditions on and
ensure that the limit of
for
exists for appropriate constants
and
?
Some conditions on tails and
are required to ensure that
converges to a non-trivial limit, i.e., a number in
.
Theorem 3.1
Suppose is an independent sequence of random variables which is mixed with two subsequences
and
with underlying distributions
and
,
and
as
. Let
and
and
are two sequences of real numbers such that
(25)
(25) Then
(26)
(26) Conversely, if (Equation26
(26)
(26) ) holds for some
, then so does (Equation25
(25)
(25) ).
Remark
Since is the probability that
exceeds level
, Equation (Equation25
(25)
(25) ) means that the expected number of exceedences of
by
and
by
in total converges to τ. When the sequence is generated from one distribution
, Theorem 3.1 can be reduced to the classical result by choosing
. That is
(27)
(27) if and only if
(28)
(28) as
.
The following corollary gives the conditions such that we can choose one of and
to be applied to
, and derive a similar limit of
. The condition involves both the ratio of two tail probabilities
and
.
Corollary 3.1
Let ,
. Suppose that there exist two sequences
and
such that
(29)
(29) Then
(30)
(30) Moreover, if
, where
, then
(31)
(31)
Specifically, if we choose ,
, and suppose that
(32)
(32)
(33)
(33) then
and
belong to the GEV distribution family and the limit in (Equation31
(31)
(31) ) becomes
.
The following is an example of mixed sequence and the limit properties of the maxima of subsequences and the global maxima.
Example 3.1
Suppose is a sequence of random variables combining two subsequences
and
. Suppose
, where
,
and
are i.i.d. from a Pareto distribution with
and a Fréchet distribution with
respectively.
Since for each
so that Type II (Fréchet) limit applies. For
we have
, so that
(34)
(34) Putting
for
,
(35)
(35) On the other hand,
, i.e.,
.
Then we have for ,
(36)
(36) Since
When
, the condition
in Corollary 3.1 is satisfied, hence
(37)
(37) Since
we also have
(38)
(38)
3.2. Approximation error
The convergence results are usually accompanied by the question of the approximation error. Suppose and
, writing
and
, then by Theorem 3.1 we have
(39)
(39) The approximation can be decomposed into several parts. We have
and
We denote
Then
The following result gives the bound for the approximation error.
Theorem 3.2
Let be an independent sequence of random variables mixed with two subsequences
and
, which satisfies
and
,
,
are defined as above, then
with
where the first bound is asymptotically sharp, in the sense that if
then
. Furthermore, for
,
with
.
If for
, then (Equation39
(39)
(39) ) holds. By Lemma A.1, (Equation39
(39)
(39) ) holds also if
and
are replaced by different constants
and
, satisfying
and
. However, the speed of convergence to zero of
(thus the speed of
to
) can be very different for different choices of norming constants.
4. Weakly dependent sequences
In this section, we extend the independent sequences to weakly dependent sequences. For a sequence of random variables with identical distribution, it is stationary if
and
have the same joint distribution for any choice of n,
, and m. For the mixed sequence, we will provide some alternatives so that the desired results still hold. We assume that the dependence between
and
falls off in some specific way as
increases.
4.1. Review of some weakly dependent conditions
Some weakly dependent conditions in the literature can be generalised to the scenarios of mixed sequences. For m-dependent sequence ,
and
are independent if
. Another commonly used condition is the strong mixing condition first introduced by Rosenblatt (Citation1956). A sequence of random variables
is said to satisfy the strong mixing condition if for some
and
for any p and k, where
as
;
is the σ-field generated by the indicated random variables. The function
does not depend on the sets A and B, so the strong mixing condition is uniform.
For normal sequences, the correlation between and
may be a better measure of dependence. We can also use the dependence restriction
where
as
.
Since the event is the same as
. We may restric the events on this type of event. Following Leadbetter et al. (Citation2012), we use
to denote
. The following condition D is a weakened condition of strong mixing.
The condition will be said to hold if for any integers
and
for which
, and any real u,
(40)
(40) where
as
.
Under the condition D, the Extremal Types Theorem also holds. Since we usually deal with the event for some levels
, the condition can still be weakened. The condition
is defined as follows.
The condition will be said to hold if for any integers
(41)
(41) for which
, we have
(42)
(42) where
as
for some sequence
.
The condition guarantees that
. We still need a further assumption to have the opposite inequality for the upper limit. Here we present the
condition used in Watson (Citation1954) and Loynes (Citation1965). This condition bounds the probability of more than one exceedance among
, therefore no multiple points in the point process of exceedances.
The condition will be said to hold for the sequence of random variables
, if
(43)
(43) as
, (where [ ] denotes the interger part).
If both conditions and
are satisfied, we have
is equivalent to
for
.
4.2. Weakly dependent mixed sequences
To generalise the results from non-mixed sequences to mixed sequences, we need to modify the conditions of and
. We use
to denote the vector of levels
when the sequence
is composed of two subsequences
and
,
. We further assume that
as
,
, so that
.
Before introducing the more general condition, we introduce some new notations. Let
. Here
indicates that the event A is true, otherwise
. The notation
represents the threshold for
, which depends on the subsequence that
belongs to. For example, if
and
, then
represents
. After introducing this notation, we can state the condition
as follows.
The condition will be said to hold for the mixed sequence of random variables
with two subsequences
and
if for any integers
(44)
(44) for which
, we have
where
as
for some sequence
.
Similarly, we can also extend the condition for mixed sequences, which is denoted as
.
The condition will be said to hold for the mixed sequence of random variables
and levels
if
(45)
(45) where
and
denotes the integer part.
Equation (Equation45(45)
(45) ) means that
. It can be observed that if
holds for the mixed sequence
, then
also holds for the subsequence
, for j = 1, 2. The same conclusion is also true for the condition
.
After introducing the conditions and
, we have the extended results for mixed sequences. We assume that the two subsequences
and
are independent with each other. Also, for any interval
with
members, there are
members from
and
members from
. We assume that the proportion of each subsequence
and
, where
.
Theorem 4.1
Let be a weakly dependent mixed sequence of random variables with two subsequences
and
, with sample size proportions
and
as
,
. Suppose that
and
hold for
, then for
(46)
(46) if and only if
(47)
(47)
Based on Theorem 4.1, we have the following corollary.
Corollary 4.1
The same conclusions hold with (i.e.,
if and only if
if the requirements that
,
hold are replaced by the condition that, for arbitrarily large
, there exists a vector of levels
such that
, which satisfy
with
and
hold.
Theorem 4.1 tells us the property of the joint probability given the tail properties of
and
.
is the mean exceedances of the two thresholds by the corresponding subsequences in total. Theorem 4.1 is the generalisation of Theorem 3.1 under the condition that the mixed sequence is weakly dependent within each subsequence.
4.3. Associated independent sequences
The ‘independent sequence associated with ’ can be used to study the maxima of dependent sequence. It was first introduced by Loynes (Citation1965). For a weakly dependent sequence of random variables
, the notation
is used to be the independent sequence with the same marginal distribution as
, and write
. When
is mixed with two subsequences
and
with different marginal distributions, we still have the associated independent subsequences
and
, and we write
, for i = 1, 2.
The following Theorem 4.2 tells us that, under the weakly dependent conditions, and
have the same limit if it exists. By Theorem 4.3, we can choose the same norming constant as the independent sequence to derive the same limit of
and
.
Theorem 4.2
Let be a mixed sequence of random variables with two subsequences
and
, independent with each other. Suppose
and
hold for a vector of levels
. Then
if and only if
. The same holds with
if the condition
and
are replaced by the requirement that for arbitrarily large
there exists
such that
, which satisfy
with
and
hold.
Theorem 4.3
Suppose that and
hold for the mixed sequence of random variables
, with
for each real x. Then
(48)
(48) if and only if
(49)
(49) for some non-degenerate continuous distribution function
.
With the results in this section, for weakly dependent sequences with conditions and
being satisfied, we can treat them as independent sequences when studying the limit distribution of the maxima. In the next section, some numerical experiments and estimation results are presented.
5. Numerical experiments
5.1. Simulation
We study the accuracy of the accelerated max-stable distributions in estimating the high quantiles of the simulated data. They are compared to the results using the classical GEV distribution alone. To simulate the data, we first generate two sequences from two different GEV distributions with parameters and
, denoting them as
and
, here n = 2000. We pair them and find their maxima,
, then fit the accelerated max-stable distribution and GEV distribution separately to the sequence
using maximum likelihood method. Using each fitted distribution, we generate a new sequence
and calculate the proportion of
that exceeds the 90th, 95th and 99th percentiles of the original sequence
. The simulation scenarios cover all the possible combinations of three types of extreme value distributions. For each combination scenario, the process is repeated 100 times and the standard deviations of the estimated proportions are shown in the parentheses. The results are in Table .
Table 1. The proportions of the simulated data based on the fitted accelerated max-stable distributions and GEV distributions that exceeds the 90th, 95th and 99th percentiles of the original data .
From Table , for the 90th percentile, we can observe that accelerated max-stable distributions perform better than the GEV alone, and the exceeding proportion is closer to the theoretical value 0.1. The same is true for the 95th percentiles. For both of these two percentiles, the proportions are larger than the theoretical value 0.1 and 0.05 in general, with the GEV distribution deviating more. This observation implies that both estimations overestimate the true values. For the 99th percentiles, we observe that the differences are not large overall. With a few cases (2nd and 3rd), the accelerated max-stable distribution outperforms the GEV distribution. Also, the proportions for accelerated max-stable distributions are all larger than 0.01 and those for GEV distributions are mostly smaller than 0.01. This phenomenon implies that the accelerated max-stable distribution may overestimate the 99th percentiles. On the other hand, the GEV distribution may underestimate the 99th percentiles.
5.2. Real data
In this section, we apply both AMSD/AEVD and GEV fitting to stock data. The data contains the daily closing prices of 330 S&P 500 companies. Based on the closing prices, we calculate the daily negative log returns using the formula . Here
represents the stock's closing price of one company on day i. For each day i, we obtain the 330 negative log returns and calculate the maximal value of them, denoting it as
. The time range is from 3 January 2000 to 30 December 2016, which contain 4277 trading days in the data. The histogram showing the distribution of
is in Figure .
Figure 10. The histogram of the daily maxima of negative log returns of 330 stocks in the S&P 500 companies list.
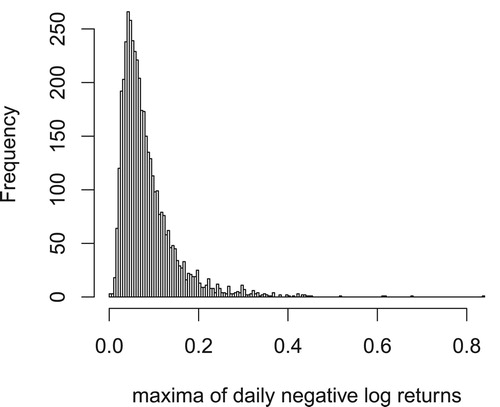
We find the 90th, 95th and 99th sample percentiles of , which are 0.1545, 0.2 and 0.3229, respectively. Here the daily maximal negative log returns have some time dependency. However, for the purpose of demonstration, we treat them as independent and fit the AMSD/AEVD and the GEV distribution to
. Based on the fitted distributions, we generate random samples with the same size and find the proportions of the samples that exceed the three percentiles. The proportions are shown in Table .
Table 2. The proportions of the simulated samples generated from the fitted distributions that exceed the 90th, 95th, and 99th sample percentiles of the maximal daily negative log returns.
Table clearly reveals that the AMSD/AEVD performs better than the GEV alone. The modelling performance may be further improved if time series dependence is implemented in the model fitting, e.g., the AcF model proposed by Zhao et al. (Citation2018) and Mao and Zhang (Citation2018). We will leave this task as a future project.
6. Conclusions
This paper extends the classical extreme value theory to maxima of maxima of time series with mixture patterns depending on the sample size. It has been shown that the classical extreme value distributions are special cases of the accelerated max-stable (extreme value) distributions (AMSDs/AEVDs). Some basic probabilistic properties are presented in the paper. These properties can be used as the probability foundation of recently proposed statistical models for extreme observations. The AMSDs may shed the light of extreme value studies and inferences. Many of existing theories in classical extreme value literature can be renovated in a much more general setting. Many real applications, e.g., risk analysis and portfolio management, systemic risk, etc. can be reanalysed and better results can be expected. Under the newly introduced framework, many new statistical models can be introduced and explored.
Acknowledgments
The authors thank Editor Jun Shao and two referees for their valuable comments. The work by Cao was partially supported by NSF-DMS-1505367 and Wisconsin Alumni Research Foundation #MSN215758. The work by Zhang was partially supported by NSF-DMS-1505367 and NSF-DMS-2012298.
Disclosure statement
No potential conflict of interest was reported by the author(s).
Additional information
Funding
Notes on contributors
Wenzhi Cao
Wenzhi Cao is a PhD student from the statistics department at the University of Wisconsin-Madison. Cao received his bachelor degree in Mathematics from Nankai University. Cao's research areas include extreme value theory and machine learning.
Zhengjun Zhang
Zhengjun Zhang is Professor of Statistics at the University of Wisconsin. Zhang's main research areas of expertise are in financial time series and rare event modelling, virtual standard cryptocurrency, risk management, nonlinear dependence, asymmetric dependence, asymmetric and directed causal inference, gene-gene relationship in rare diseases.
References
- Beirlant, J., Goegebeur, Y., Segers, J., & Teugels, J. (2004). Statistics of extremes: Theory and applications. Wiley Series in Probability and Statistics. Wiley.
- Bücher, A., & Segers, J. (2017). On the maximum likelihood estimator for the generalized extreme-value distribution. Extremes, 20(4), 839–872. https://doi.org/https://doi.org/10.1007/s10687-017-0292-6
- Castillo, E., Hadi, A. S., Balakrishnan, N., & Sarabia, J. M. (2005). Extreme value and related models with applications in engineering and science. Wiley Series in Probability and Statistics. Wiley.
- Chavez-Demoulin, V., Embrechts, P., & Hofert, M. (2016). An extreme value approach for modeling operational risk losses depending on covariates. Journal of Risk and Insurance, 83(3), 735–776. https://doi.org/https://doi.org/10.1111/jori.v83.3
- Coles, S. (2001). An introduction to statistical modeling of extreme values. Springer.
- Daouia, A., Girard, S., & Stupfler, G. (2018). Estimation of tail risk based on extreme expectiles. Journal of the Royal Statistical Society: Series B (Statistical Methodology), 80(2), 263–292. https://doi.org/https://doi.org/10.1111/rssb.12254
- de Haan, L. (1993). Extreme value statistics. In J. Galambos, J. Lechner, & E. Simiu (Eds.), Extreme value theory and applications (pp. 93–122). Kluwer Academic Publisher.
- de Haan, L., & Ferreira, A. (2006). Extreme value theory: An introduction. Springer Verlag.
- Dey, D. K., & Yan, J. (2016). Extreme value modeling and risk analysis: Methods and applications. Chapman & Hall/CRC.
- Embrechts, P., Resnick, S. I., & Samorodnitsky, G. (1999). Extreme value theory as a risk management tool. North American Actuarial Journal, 3(2), 30–41. https://doi.org/https://doi.org/10.1080/10920277.1999.10595797
- Finkenstädt, B., & Rootzén, H. E. (2004). Extreme values in finance, telecommunications, and the environment. Chapman & Hall/CRC.
- Galambos, J. (1978). The asymptotic theory of extreme order statistics. Technical Report.
- Heffernan, J. E., Tawn, J. A., & Zhang, Z. (2007). Asymptotically (in)dependent multivariate maxima of moving maxima processes. Extremes, 10(1–2), 57–82. https://doi.org/https://doi.org/10.1007/s10687-007-0035-1
- Idowu, T., & Zhang, Z. (2017). An extended sparse max-linear moving model with application to high-frequency financial data. Statistical Theory and Related Fields, 1(1), 92–111. https://doi.org/https://doi.org/10.1080/24754269.2017.1346852
- Leadbetter, M. R., Lindgren, G., & Rootzén, H. (2012). Extremes and related properties of random sequences and processes. Springer Science & Business Media.
- Loynes, R. M. (1965). Extreme values in uniformly mixing stationary stochastic processes. The Annals of Mathematical Statistics, 36(3), 993–999. https://doi.org/https://doi.org/10.1214/aoms/1177700071
- Malinowski, A., Schlather, M., & Zhang, Z. (2015). Marked point process adjusted tail dependence analysis for high-frequency financial data. Statistics and Its Interface, 8(1), 109–122. https://doi.org/https://doi.org/10.4310/SII.2015.v8.n1.a10
- Mao, G., & Zhang, Z. (2018). Stochastic tail index model for high frequency financial data with Bayesian analysis. Journal of Econometrics, 205(2), 470–487. https://doi.org/https://doi.org/10.1016/j.jeconom.2018.03.019
- McNeil, A. J., & Frey, R. (2000). Estimation of tail-related risk measures for heteroscedastic financial time series: An extreme value approach. Journal of Empirical Finance, 7(3–4), 271–300. https://doi.org/https://doi.org/10.1016/S0927-5398(00)00012-8
- Mikosch, T., Embrechts, P., & Klüppelberg, C. (1997). Modelling extremal events for insurance and finance. Springer Verlag.
- Naveau, P., Zhang, Z., & Zhu, B. (2011). An extension of max autoregressive models. Statistics and Its Interface, 4(2), 253–266. https://doi.org/https://doi.org/10.4310/SII.2011.v4.n2.a19
- Resnick, S. I. (2013). Extreme values, regular variation and point processes. Springer.
- Rocco, M. (2014). Extreme value theory in finance: A survey. Journal of Economic Surveys, 28(1), 82–108. https://doi.org/https://doi.org/10.1111/joes.2014.28.issue-1
- Rosenblatt, M. (1956). A central limit theorem and a strong mixing condition. Proceedings of the National Academy of Sciences USA, 42(1), 43–47. https://doi.org/https://doi.org/10.1073/pnas.42.1.43
- Salvadori, G., De Michele, C., Kottegoda, N. T., & Rosso, R. (2007). Extremes in nature: An approach using copulas. Springer(Complexity).
- Smith, R. L. (1985). Maximum likelihood estimation in a class of nonregular cases. Biometrika, 72(1), 67–90. https://doi.org/https://doi.org/10.1093/biomet/72.1.67
- Tang, R., Shao, J., & Zhang, Z. (2013). Sparse moving maxima models for tail dependence in multivariate financial time series. Journal of Statistical Planning and Inference, 143(5), 882–895. https://doi.org/https://doi.org/10.1016/j.jspi.2012.11.008
- Tsay, R. S. (2005). Analysis of financial time series (Vol. 543). John Wiley & Sons.
- Watson, G. S. (1954). Extreme values in samples from m-dependent stationary stochastic processes. The Annals of Mathematical Statistics, 25(4), 798–800. https://doi.org/https://doi.org/10.1214/aoms/1177728670
- Zhang, Z., & Smith, R. L. (2010). On the estimation and application of max-stable processes. Journal of Statistical Planning and Inference, 140(5), 1135–1153. https://doi.org/https://doi.org/10.1016/j.jspi.2009.10.014
- Zhang, Z., & Zhu, B. (2016). Copula structured m4 processes with application to high-frequency financial data. Journal of Econometrics, 194(2), 231–241. https://doi.org/https://doi.org/10.1016/j.jeconom.2016.05.004
- Zhao, Z., & Zhang, Z. (2018). Semi-parametric dynamic max-copula model for multivariate time series. Journal of the Royal Statistical Society: Series B (Statistical Methodology), 80(2), 409–432. https://doi.org/https://doi.org/10.1111/rssb.12256
- Zhao, Z., Zhang, Z., & Chen, R. (2018). Modeling maxima with autoregressive conditional Fréchet model. Journal of Econometrics, 207(2), 325–351. https://doi.org/https://doi.org/10.1016/j.jeconom.2018.07.004
Appendix
A.1. Proofs of Theorems and Propositions
A.1.2. Proof of Fact 2.2
The density of is
(A1)
(A1) Thus
(A2)
(A2) Dividing the integral into two parts, we get
(A3)
(A3) First, let us consider
. Since
and
is continuous on
, it is bounded on
. This implies that
(A4)
(A4) Next, let us consider
. We have
Notice that
(A5)
(A5) Therefore
only if
and
, i.e.,
.
A.1.3. Proof of Fact 2.3
We need to consider .
Since and
as
, we have the Taylor expansions
Therefore
(A6)
(A6) This proves that
and
are tail-equivalent.
A.1.4. Proof of Fact 2.4
The density of is
Thus
(A7)
(A7) Dividing the above equation into two parts, we get
(A8)
(A8) For the first part, since
and
is continuous on
, it is bounded on
. Thus
.
For the second part,
(A9)
(A9) Since
(A10)
(A10) we have
if and only if
.
A.1.5. Proof of Fact 2.5
We need to consider .
Since and
, we have the Taylor expansions
Thus
(A11)
(A11) This implies that
and
are tail-equivalent.
A.1.6. Proof of Theorem 3.1
If (Equation25(25)
(25) ) holds, we must have
Then
(A12)
(A12) which is equivalent to
Conversely, if (Equation26
(26)
(26) ) holds, which is equivalent to
(A13)
(A13) we must have
and
. Otherwise, suppose
, then there is a sequence of indexes
and
such that
for
. This means that
which is contradictory to (EquationA13
(A13)
(A13) ). We have
(A14)
(A14) and Equation (Equation25
(25)
(25) ) holds.
A.1.7. Proof of Corollary 3.1
Since
(A15)
(A15) (Equation30
(30)
(30) ) is a direct result of Theorem 3.1.
If , then
, where
. Therefore,
A.1.8. Proof of Theorem 3.2
Since and
, the result follows from Lemma A.2.
A.1.9. Proof of Theorem 4.1
For fixed k, write , suppose that there are
members from
and
members from
among
. If (Equation47
(47)
(47) ) holds, by assumption we have
and
thus
(A16)
(A16) Since
we have
(A17)
(A17) where
.
Condition implies that
as
. By (EquationA16
(A16)
(A16) ) and (EquationA17
(A17)
(A17) ), we have
Since
implies
and
, Lemma A.3 holds for each subsequence. We have
Letting
we have
.
Conversely, if (Equation46(46)
(46) ) holds,
(A18)
(A18) Since
we have
. By letting
in (EquationA18
(A18)
(A18) ),
from which (multiplying k on all sides and let
) we have
.
A.1.10. Proof of Corollary 4.1
Suppose by
and
, we have
By Theorem 4.1,
. Then
By letting
, we have
Conversely, we still have
Since the above inequality holds for arbitrary large
we must have
.
A.1.11. Proof of Theorem 4.2
For , the condition
may be rewritten as
with
, this holds if and only if
. The same is true for
by condition
and
. When
, the result follows from Corollary 4.1.
A.1.12. Proof of Theorem 4.3
If , the equivalence follows from Theorem 4.2, with
.
If , the continuity of G shows that, if
,there exists
such that
.
and
hold for
and
or
depending on the assumption made, so that
. If (Equation49
(49)
(49) ) holds, then we have
thus
and
(since one of the inequalities must hold and also implies another). By Theorem 4.2, (Equation48
(48)
(48) ) holds. The converse direction can be proved similarly.
A.2. Lemmas
Lemma A.1
Khintchine, Theorem 1.2.3 in Leadbetter et al. (Citation2012)
Let be a sequence of cdf's and H a nondegenerate cdf. Let
and
be constants such that
(A19)
(A19) Then for some nondegenerate cdf
and constants
,
,
(A20)
(A20) if and only if
(A21)
(A21) for some a>0 and b, and then
(A22)
(A22)
Lemma A.2
Lemma 2.4.1 in Leadbetter et al. (Citation2012)
If
then
(A23)
(A23) and further
(A24)
(A24) uniformly for x in bounded intervals.
If
then
(A25)
(A25) with
.
Lemma A.3
Lemma 3.3.2 in Leadbetter et al. (Citation2012)
If holds, for a fixed integer k, we have
(A26)
(A26)