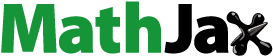
I congratulate and thank the author for providing a systematic and thorough review of both classical approaches and modern developments on the modelling of extremal events and tail dependence in the time series setting. It is an important area of research due to the massive demand from applications in various disciplines. For example, Elsner et al. (Citation2008) examined trends in upper and high quantiles of per-cyclone maximum wind speeds using satellite-derived data; Tsai (Citation2012) studied the relationship between the stock price index and the exchange rate in Asian markets when the exchange rates are extremely high; T. Zhang (Citation2020) considered inference about the temperature trend of the 1-in-100 hottest days and compared with that of the 1-in-20 hottest days. Unlike the usual concept of dependence that has been commonly quantified through the Pearson correlation coefficient and modelled by popular time series models such as the autoregressive and moving average model, studying the maxima and tail dependence generally requires developing a different but more suitable set of methodology and theory. In particular, one can easily construct examples for which the Pearson correlation coefficient can be arbitrarily close to one while still having zero tail dependence.
In addition to the influential tail quotient correlation coefficient (TQCC) test of Z. Zhang (Citation2008b) and Z. Zhang et al. (Citation2017), a distinguishable feature of the current review is its focus on the time series setting, which has been much less explored compared with the multivariate setting with independent observations. In particular, the author introduces the lag-k tail dependence measure of Z. Zhang (Citation2005) that naturally generalises the commonly used bivariate measure of Sibuya (Citation1960) to the time series setting and covers a number of extreme value time series models for the maxima and extreme observations. Note that conventional time series models such as ARMA and GARCH models are not ideal for describing extremal clusters and tail dependence in many applications due to their additive structures. To address this, the current paper reviews a list of alternative models that transform the conventional additive model into maximum-based ones to better model the maxima and extremal events. These include, but are not limited to, the moving maximum model of Hall et al. (Citation2002), the max-autoregressive moving average model of Davis and Resnick (Citation1989), the multivariate maxima of moving maxima process of Smith and Weissman (Citation1996) and its sparse extensions given by Z. Zhang (Citation2008a) and Tang et al. (Citation2013).
I shall here conclude the discussion by mentioning a potential research direction in this area. In particular, besides finding summary statistics and suitable models for describing tail dependence, another important problem is to develop limit theorems for quantities of interest under serial tail dependence. Such limit theorems can play critical roles in understanding the performance of parameter estimators and guiding the development of new statistical inference procedures. Existing results in this direction were mainly developed using the strong mixing framework of Rosenblatt (Citation1956) and its variants; see for example Drees (Citation2003), Chernozhukov (Citation2005), Davis and Mikosch (Citation2009), Drees and Rootzén (Citation2010), Davis et al. (Citation2018) and Hoga (Citation2018) among others. Therefore, an interesting research topic is to calculate the strong mixing coefficient and understand what the strong mixing condition means for processes described in the current review such as the moving maximum model of Hall et al. (Citation2002), the max-autoregressive moving average model of Davis and Resnick (Citation1989), and the sparse multivariate maxima of moving maxima process of Z. Zhang (Citation2008a) and Tang et al. (Citation2013). For example, when the coefficients in the moving maximum process of Hall et al. (Citation2002) satisfy a polynomial decay, then such results will enable us to identify the range of the polynomial rate with which the associated moving maximum process is strong mixing.
Recently, T. Zhang (Citation2020) proposed an alternative framework based on a new concept of tail adversarial stability for asymptotic theory of tail dependent time series. Unlike the strong mixing condition, the framework of T. Zhang (Citation2020) quantifies the degree of serial tail dependence by measuring the cumulative tail adversarial effect of coupled innovations and was shown to coordinate well with a lag-ℓ tail dependent martingale approximation scheme that can help lead to the desired limit theorem. In addition to its intuitive interpretability and mathematical convenience in developing limit theorems, the tail adversarial stability condition of T. Zhang (Citation2020) can also be easily verified for the moving maximum process of Hall et al. (Citation2002) and its extensions. To illustrate, we consider the moving maximum process
(1)
(1) where
is a sequence of independent unit Fréchet random variables and
is a sequence of nonnegative coefficients satisfying
. Then by elementary calculations, the tail adversarial stability condition required for an asymptotic theory of high quantile regression estimators as in T. Zhang (Citation2020) will be satisfied if
holds for some
. Compared to the condition
under which the moving maximum process (Equation1
(1)
(1) ) is well defined, such a condition seems to be reasonable and mild. Note that calculating the strong mixing coefficient for (Equation1
(1)
(1) ) may not be trivial and can possibly lead to stronger restrictions on the coefficients. Therefore, the tail adversarial stability framework of T. Zhang (Citation2020) seems to complement the existing literature on strong mixing by providing an alternative mathematical foundation for asymptotic theory of tail dependent time series, and it will be interesting to investigate how it can be used in various problems related to tail dependent time series to promote our understanding and scientific discoveries.
References
- Chernozhukov, V. (2005). Extremal quantile regression. The Annals of Statistics, 33(2), 806–839. https://doi.org/10.1214/009053604000001165
- Davis, R. A., Drees, H., Segers, J., & Warchoł, M. (2018). Inference on the tail process with application to financial time series modeling. Journal of Econometrics, 205(2), 508–525. https://doi.org/10.1016/j.jeconom.2018.01.009
- Davis, R. A., & Mikosch, T. (2009). The extremogram: A correlogram for extreme events. Bernoulli, 15(4), 977–1009. https://doi.org/10.3150/09-BEJ213
- Davis, R. A., & Resnick, S. I. (1989). Basic properties and prediction of max-arma processes. Advances in Applied Probability, 21(4), 781–803. https://doi.org/10.2307/1427767
- Drees, H. (2003). Extreme quantile estimation for dependent data, with applications to finance. Bernoulli, 9(4), 617–657. https://doi.org/10.3150/bj/1066223272
- Drees, H., & Rootzén, H. (2010). Limit theorems for empirical processes of cluster functionals. The Annals of Statistics, 38(4), 2145–2186. https://doi.org/10.1214/09-AOS788
- Elsner, J. B., Kossin, J. P., & Jagger, T. H. (2008). The increasing intensity of the strongest tropical cyclones. Nature, 455(7209), 92–95. https://doi.org/10.1038/nature07234
- Hall, P., Peng, L., & Yao, Q. (2002). Moving-maximum models for extrema of time series. Journal of Statistical Planning and Inference, 103(1–2), 51–63. https://doi.org/10.1016/S0378-3758(01)00197-5
- Hoga, Y. (2018). A structural break test for extremal dependence in β-mixing random vectors. Biometrika, 105(3), 627–643. https://doi.org/10.1093/biomet/asy030
- Rosenblatt, M. (1956). A central limit theorem and a strong mixing condition. Proceedings of the National Academy of Sciences of the United States of America, 42(1), 43–47. https://doi.org/10.1073/pnas.42.1.43
- Sibuya, M. (1960). Bivariate extreme statistics, I. Annals of the Institute of Statistical Mathematics, 11(3), 195–210. https://doi.org/10.1007/BF01682329
- Smith, R. L., & Weissman, I. (1996). Characterization and estimation of the multivariate extremal index. Technical report, University of North Carolina–Chapel Hill.
- Tang, R., Shao, J., & Zhang, Z. (2013). Sparse moving maxima models for tail dependence in multivariate financial time series. Journal of Statistical Planning and Inference, 143(5), 882–895. https://doi.org/10.1016/j.jspi.2012.11.008
- Tsai, I.-C. (2012). The relationship between stock price index and exchange rate in Asian markets: A quantile regression approach. Journal of International Financial Markets, Institutions and Money, 22(3), 609–621. https://doi.org/10.1016/j.intfin.2012.04.005
- Zhang, T. (2020). High-quantile regression for tail-dependent time series. Biometrika, forthcoming.
- Zhang, Z. (2005). A new class of tail-dependent time series models and its applications in financial time series. Advances in Econometrics, 20, 323–358.
- Zhang, Z. (2008a). The estimation of m4 processes with geometric moving patterns. Annals of the Institute of Statistical Mathematics, 60(1), 121–150. https://doi.org/10.1007/s10463-006-0078-0
- Zhang, Z. (2008b). Quotient correlation: A sample based alternative to Pearson's correlation. The Annals of Statistics, 36(2), 1007–1030. https://doi.org/10.1214/009053607000000866
- Zhang, Z., Zhang, C., & Cui, Q. (2017). Random threshold driven tail dependence measures with application to precipitation data analysis. Statistica Sinica, 27, 685–709. https://doi.org/10.5705/ss.202014.0421