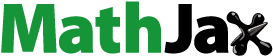
We congratulate Prof. Zhang for this timely review on recent advances in extreme value theory for heterogeneous populations and on time series models for extreme observations. This is a substantial effort. Not only does it give a summary of the state-of-the-art work in time series modelling of extremes but also suggests interesting methodological and applied research questions. Our discussion focuses on extremal dependence metrics and practical applications for the time series models.
1. Extremal dependence metrics
Extreme dependence metrics are important in extreme value modelling. Zhang (Citation2021) promotes tail quotient correlation coefficient (TQCC). We would like to highlight several other extremal dependence metrics in the extreme literature. Although not yet considered for heterogeneous populations, one may want to further explore their theoretical properties under the heterogeneous population assumption.
When analysing the extremal behaviour for a non-negative, d-dimensional regularly varying random vector , one canonical way is to apply a polar transformation (Resnick, Citation2007, Chapter 6.1),
, with a fixed norm
on
. In general, if
is regularly varying, then it is equivalent to say: there exists a probability measure S on the unit sphere,
, and a constant c>0, such that on
(the space of Radon measures on
),
where
denotes the vague convergence of measures on
,
is the measure on
with
, x>0, and
is a scaling function. The measure S is referred to as the spectral or angular measure. Heuristically, the angular measure S specifies possible directions of extremal events, thus laying the foundation of defining extremal dependence metrics.
When d = 2, for a regularly varying random vector with index
and angular measure S, the extremal dependence measure (EDM) is defined in Resnick (Citation2004) as
In particular,
if and only if S concentrates on the coordinate axes, referring to asymptotic independence.
Another dependence metric, the extremogram, has been introduced by Davis and Mikosch (Citation2009) in the context of regularly varying time series, i.e., for any , the lagged vector
satisfies on
,
where the limit measure
satisfies
, for any Borel set
. For
, the extremogram is defined as
Larsson and Resnick (Citation2012) compare the extremogram with EDM by rewriting
as a function of the angular measure,
, stemming from
. Here c_h>0 is a constant which may depend on h. When applied to max-autoregressive moving average (MARMA) processes the extremogram and the EDM may decay at different rates.
In the context of risk contagion, the presence of asymptotic independence may mask the underlying extremal dependence structure, which makes hidden regular variation (HRV) an important tool. Asymptotic independence means that the multivariate regular variation (MRV) limit measure concentrates on the coordinate axes. MRV may exist not only on the cone but also on a smaller cone, e.g.,
. Then the regular variation on the smaller cone
is the HRV. Das and Resnick (Citation2015) and Das and Resnick (Citation2017) considered several generative models with both MRV and HRV together with useful detection mechanisms of HRV. Such generative models can also be viewed as possible candidates for heterogeneous populations, but with different scopes than those reviewed in Zhang (Citation2021), where most of the discussion concentrates on the modelling of maxima. Recently, a grid estimator for the support of the angular measure has been proposed in Lehtomaa and Resnick (Citation2020), and it will be intriguing to inspect properties of all these methods under heterogeneous populations.
2. Practical applications
Zhang (Citation2021) suggests several time series model for extremes as alternatives to the widely used ARMA and GARCH models. These models are designed to characterise extremes or tail behaviours. To make them as accessible as ARMA and GARCH models in routine time series analyses, much methodological and computational developments are needed. We highlight several directions that may lead to fruitful and impactful research.
2.0.0.1 Threshold selection
Threshold selection, a notorious problem in the peaks over threshold (POT) method for modelling extremes, lingers in the time series modelling context. For instance, consider testing for tail (in)dependence between two series. The TQCC can be used to construct tests as suggested by Theorem 3.7–3.8. To compute the TQCC from an observed sample, however, a threshold has to be chosen. It is often selected as the 95th percentile, or the 99th percentile if the sample size is large. The threshold selection is an old problem and needs to be solved by striking a balance between the bias-variance trade-off. Some methods are based on a sequence of ordered hypothesis tests, in which case the false discovery rate may be controlled (Bader et al., Citation2018).
2.0.0.2 Parameter estimation
Parameter estimation is challenging for the time series models for multivariate extremes. Unless for a few special cases, the intractability of multivariate extreme density calls for alternative estimation approaches. These models, including the MARMA model, multivariate maxima and moving maxima (M4) model, and even their sparse versions, have a large number of parameters, which bring an extra difficulty. The generalised method of moments (GMM) applied to the tail dependence coefficients has been used in many of the works by Prof. Zhang. Alternative methods for intractable likelihood such as composite likelihood (Sang, Citation2016) and approximate Bayesian computation (Erhardt & Sisson, Citation2016) might help with parameter estimation in certain scenarios.
In the two applications presented in Section 5, the extreme value analyses started from the residuals of univariate GARCH models with t-distributed innovations. This is basically a two-step inference procedure. Such procedure is commonly used in copula modelling where the marginal models are fitted first and then the copula model is fitted with pseudo-observations obtained based on the marginal fits (e.g., Joe, Citation2005). When making inferences about the parameters in the second stage, the uncertainty in the estimation of the first-stage parameters may play a role. The rank-based bootstrap procedure takes the GARCH residuals as given, which may underestimate the uncertainty if the first-stage parameter estimation uncertainty does have an effect on the second stage.
2.0.0.3 Model diagnostics
ARMA and GARCH models in time series analyses have many standard diagnostic tools. Examples are sample autocorrelation functions, sample partial autocorrelation functions, residual quantile–quantile plots and the Ljung–Box test (Ljung & Box, Citation1978). For extreme value time series models to be as popular as ARMA and GARCH models, similar diagnostic tools need to be developed. The quantile–quantile plot has been the most used because, often times, the models have a sequence of hidden Fréchet or Gumbel variables that can be recovered once the parameters are estimated. Practitioners need tools to determine the order of models (e.g., MARMA or M4), to assess the goodness-of-fit of the hypothesised model and to compare across models (nested or non-nested). The connection from diagnostic tools (Davis & Mikosch, Citation2009; Ledford & Tawn, Citation2003) other than TQCC to the times series models remains to be established.
2.0.0.4 Software implementation
Despite the many software packages for extreme value analysis (e.g. https://cran.r-project.org/web/views/ExtremeValue.html), none provides implementations for the models for time series extremes that are meant to be alternatives to the ARMA and GARCH models. The situation did not change from the review of Zhang et al. (Citation2016) to the current review. From this perspective, it would be of great value to both the methodological community and applied community of time series regressions of extremes to have the models available for practical applications, including threshold selection, parameter estimation, model diagnostics and beyond. We look forward to the days when people use these models as competently and conveniently as they do for ARMA and GARCH models.
References
- Bader, B., Yan, J., & Zhang, X. (2018). Automated threshold selection for extreme value analysis via ordered goodness-of-fit tests with adjustment for false discovery rate. The Annals of Applied Statistics, 12(1), 310–329. https://doi.org/10.1214/17-AOAS1092
- Das, B., & Resnick, S. I. (2015). Models with hidden regular variation: Generation and detection. Stochastic Systems, 5(2), 195–238. https://doi.org/10.1287/14-SSY141
- Das, B., & Resnick, S. I. (2017). Hidden regular variation under full and strong asymptotic dependence. Extremes, 20(4), 873–904. https://doi.org/10.1007/s10687-017-0290-8
- Davis, R. A., & Mikosch, T. (2009). The extremogram: A correlogram for extreme events. Bernoulli, 15(4), 977–1009. https://doi.org/10.3150/09-BEJ213
- Erhardt, R., & Sisson, S. A. (2016). Modeling extremes using approximate Bayesian computation. In D. Dey & J. Yan (Eds.), Extreme value modeling and risk analysis: Methods and applications (pp. 281–306). CRC Press.
- Joe, H. (2005). Asymptotic efficiency of the two-stage estimation method for copula-based models. Journal of Multivariate Analysis, 94(2), 401–419. https://doi.org/10.1016/j.jmva.2004.06.003
- Larsson, M., & Resnick, S. I. (2012). Extremal dependence measure and extremogram: The regularly varying case. Extremes, 15(2), 231–256. https://doi.org/10.1007/s10687-011-0135-9
- Ledford, A. W., & Tawn, J. A. (2003). Diagnostics for dependence within time series extremes. Journal of the Royal Statistical Society: Series B (Statistical Methodology), 65(2), 521–543. https://doi.org/10.1111/rssb.2003.65.issue-2
- Lehtomaa, J., & Resnick, S. I. (2020). Asymptotic independence and support detection techniques for heavy-tailed multivariate data. Insurance: Mathematics and Economics. 93: 262–277. https://doi.org/10.1016/j.insmatheco.2020.05.002.
- Ljung, G. M., & Box, G. E. P. (1978). On a measure of lack of fit in time series models. Biometrika, 65(2), 297–303. https://doi.org/10.1093/biomet/65.2.297
- Resnick, S. (2004). The extremal dependence measure and asymptotic independence. Stochastic Models, 20(2), 205–227. https://doi.org/10.1081/STM-120034129
- Resnick, S. I. (2007). Heavy-tail phenomena: Probabilistic and statistical modeling. Springer Science & Business Media.
- Sang, H. (2016). Composite likelihood for extreme values. In D. Dey & J. Yan (Eds.), Extreme value modeling and risk analysis: Methods and applications (pp. 239–256). CRC Press.
- Zhang, Z. (2021). On studying extreme values and systematic risks with nonlinear time series models and tail dependence measures. Statistical Theory and Related Fields. Forthcoming.
- Zhang, Z., Peng, L., & Idowu, T. (2016). Max-autoregressive and moving maxima models for extremes. In D. Dey & J. Yan (Eds.), Extreme value modeling and risk analysis: Methods and applications (pp. 153–178). CRC Press.