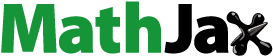
We first want to commend (Shao, Citation2021) for a timely paper that reviews the methodological and theoretical advances in statistical inference after covariate-adaptive randomisation in the last decade. The paper clearly presents the important considerations and pragmatic recommendations when analysing data obtained from covariate-adaptive randomisation, which provides principled guidelines for the practice.
The aim of our remaining comments is to extend the discussion on the invariance property in Shao (Citation2021). That is, the asymptotic distribution of an estimator remains the same under different covariate-adaptive randomisation schemes. For ease of reading, we follow the notation in Shao (Citation2021) whenever possible and focus on the case of two treatment arms (i.e., ). The ideas can be extended to the case of multiple treatment arms.
For continuous or binary outcomes, Shao (Citation2021) describes three post-stratified estimators for the population mean difference :
where is the support of
, and all other quantities are defined in Sections 5.2 and 6.1 of Shao (Citation2021). These post-stratified estimators enjoy the invariance property; that is, their asymptotic distributions are not affected by the covariate-adaptive randomisation. This is a very appealing property as (i) the same inference procedure can be universally applied to different covariate-adaptive randomisation schemes; and (ii) valid inference of the treatment effect can be obtained when complicated covariate-adaptive randomisation schemes such as the Pocock and Simon's minimisation are employed.
It is well-known that the post-stratified estimator is algebraically equivalent with the estimator of θ from fitting the following working model
where
are unknown parameters, and
is the support of
with one level dropped to avoid degeneracy. See, for example, Fuller (Citation2009, Chapter 2.2.3). Analogously,
is algebraically equivalent with the estimator of θ from fitting the following working model
and
is algebraically equivalent with the estimator of θ from fitting the following working model
where
are unknown parameters.
These three invariant estimators can each be obtained as the estimator of θ from fitting the following working model, with properly specified
and
being functions of
, and
and
being their sample means,
(1)
(1)
where
are unknown parameters. Here, the set of covariates included in
and
are non-overlapping to avoid degeneracy, where
has full interactions with
, while
does not have interactions with
. Specifically,
can be obtained with
being a column vector of all dummy variables for
and
being empty,
can be obtained with
and
being empty, and
can be obtained with
and
.
In fact, model (Equation1(1)
(1) ) defines a general class of estimators of the treatment effect
,
(2)
(2)
where
are the least squares estimates from fitting the working model (Equation1
(1)
(1) ). Similar to the proof of Theorem 2 in Ye et al. (Citation2020), we can show that the class of estimators defined in (Equation2
(2)
(2) ) are consistent and asymptotically normal and have asymptotic distributions invariant to the covariate-adaptive randomisation schemes, as long as
includes the dummy variables for all joint levels of
as a sub-vector. The key step in the proof is to show that for
,
(3)
(3)
where
and
are the probability limits of
defined as
Taking the derivatives and rearranging give
,
, and
Because
is discrete and
contains all joint levels of
as a sub-vector, we have that (Equation3
(3)
(3) ) holds.
Equation (Equation2(2)
(2) ) provides a more complete characterisation of the estimators satisfying the invariance property, compared with the ANHECOVA estimator in Ye et al. (Citation2020) derived from (Equation1
(1)
(1) ) without the term
However, we should note that although the class of estimators defined in (Equation2
(2)
(2) ) enjoy the invariance property, they may be less efficient than the simple mean difference
under some data generating process. This stresses the advantage of the ANHECOVA estimator in Ye et al. (Citation2020) that is guaranteed to be more efficient than the simple mean difference
.
Disclosure statement
No potential conflict of interest was reported by the author(s).
Additional information
Notes on contributors
Ting Ye
Ting Ye is a Postdoctoral Fellow at the Department of Statistics, The Wharton School, University of Pennsylvania. She obtained a Ph.D. in Statistics at the University of Wisconsin-Madison in 2019. Her research interest centers around developing pragmatic and robust statistical methods to analyze complex datasets, and advancing causal inference in biomedical and social sciences.
Yanyao Yi
Yanyao Yi is a Research Scientist at the Global Statistical Sciences, Eli Lilly and Company. He obtained a Ph.D. in Statistics at the University of Wisconsin-Madison in 2019. His research mostly focuses on clinical trials.
References
- Fuller, W. A. (2009). Sampling statistics. Wiley.
- Shao, J. (2021). Inference after covariate-adaptive randomisation: Aspects of methodology and theory. Statistical Theory and Related Fields, 5. https://doi.org/10.1080/24754269.2021.1871873
- Ye, T., Shao, J., Yi, Y., & Zhao, Q. (2020). Principles for covariate adjustment in analysing randomized clinical trials. arXiv:2009.11828.