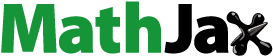
We congratulate Professor Shao on his exciting and thought-provoking paper and appreciate the Editor's invitation to discuss it. This paper provided a comprehensive review of the methodology and theory for statistical inference under covariate-adaptive randomisation. Covariate-adaptive randomisation is widely used in the design stage of clinical trials to balance baseline covariates that are most relevant to the outcomes. Researchers often use linear regression or analysis of covariance (ANCOVA) to analyse the experimental results in the analysis stage. However, the validity of the resulting inferences is not crystal clear because the usual modelling assumptions might not be justified by covariate-adaptive randomisation. It is essential to develop a model-assisted methodology and theory for statistical inference under covariate-adaptive randomisation, allowing the working model to be arbitrarily misspecified. Professor Shao's paper discussed recent developments in this aspect and made recommendations on using valid and efficient inference procedures under covariate-adaptive randomisation.
As pointed out by Professor Shao, Ye, Yi, et al. (Citation2020) proposed a model-assisted regression approach and showed that the resulting regression-adjusted average treatment effect estimator is more efficient than (as least as efficient as) the difference-in-means estimator, without any modelling assumptions on the potential outcomes and covariates. In other words, the model-assisted inference is efficient and robust to model misspecification.
The efficiency gain and robustness of regression adjustment have been widely investigated under simple randomisation. When there are two treatment arms (treatment and control), Yang and Tsiatis (Citation2001) examined three commonly used regression models for estimating the average treatment effect:
where
is the observed outcome,
is the treatment assignment indicator,
is the vector of covariates, and
is the vector of covariate means. They showed that these three ordinary-least-squares (OLS) estimators of τ are consistent for the average treatment effect and the third OLS estimator is the most efficient. In fact, as shown by Wang, Ogburn, et al. (Citation2019), when
(equal allocation), the second OLS estimator is as efficient as the third one and the usual OLS variance estimator derived from the second regression is also consistent. Thus, we can use standard statistical software, such as lm in R, to construct confidence intervals or tests.
Recently, the efficiency and robustness of regression adjustment under covariate-adaptive randomisation have witnessed significant advances from different perspectives (Bugni et al., Citation2018, Citation2019; Ma et al., Citation2020b; Wang, Susukida, et al., Citation2019; Ye & Shao, Citation2018; Ye, Shao, et al., Citation2020). Under stratified randomisation and a finite population framework, Liu and Yang (Citation2020) also proposed a regression-adjusted average treatment effect estimator and showed that it is more efficient than (as least as efficient as) the stratified difference-in-means estimator,
In practice when there exist small strata, the stratum-specific regression-adjusted vectors
proposed by Ye, Yi, et al. (Citation2020) might lead to inferior performance due to overfitting (Liu & Yang, Citation2020). To solve this problem, two independent works Ma et al. (Citation2020b) (for two treatment arms) and Ye, Shao, et al. (Citation2020) (for multiple treatment arms) suggested to use the stratum-common regression-adjusted vectors:
where
denotes sample in stratum
, and
is the sample mean of the covariates under treatment arm a within stratum
. The resulting regression-adjusted average treatment effect estimator is
where
is the sample mean of the covariates within stratum
. Ma et al. (Citation2020b) and Ye, Shao, et al. (Citation2020) answered a question raised in Wang, Ogburn, et al. (Citation2019),
It is an open question, to the best of our knowledge, as to what happens when more variables than the stratification indicators are included in the ANCOVA model under such randomisation schemes, in terms of consistency of the ANCOVA estimator and how to compute its asymptotic variance under arbitrary model misspecification.
As shown by Ma et al. (Citation2020b) and Ye, Shao, et al. (Citation2020), is the OLS estimator of τ in the regression with treatment-by-covariates interactions,
(1)
(1)
where
includes all stratum indicators and additional covariates
. From a practical point of view, researchers often use a simpler regression (often called ANCOVA):
(2)
(2)
Ma et al. (Citation2020b) showed that, for equal allocation, i.e.,
, the OLS estimator derived from regression (Equation2
(2)
(2) ) is as efficient as (asymptotically) that obtained from regression (Equation1
(1)
(1) ). More importantly, the OLS variance estimator derived from regression (Equation2
(2)
(2) ) is also consistent. These properties hold for almost all covariate-adaptive randomisation including minimisation. Therefore, it is valid and efficient to use ANCOVA under covariate-adaptive randomisation with two treatment arms and equal allocation, even if the linear model is misspecified. However, for clinical trials with more than two treatment arms, the equivalence of the point estimators obtained from regressions (Equation1
(1)
(1) ) and (Equation2
(2)
(2) ) does not hold anymore (Ye, Shao, et al., Citation2020). As shown by Ye, Shao, et al. (Citation2020), asymptotically, regression (Equation1
(1)
(1) ) is more efficient than (at least as efficient as) regression (Equation2
(2)
(2) ), and a nonparametric variance estimator should be used for valid inferences. Another contribution of Ye, Shao, et al. (Citation2020) is that they established the joint asymptotic normality of
, where K is the number of treatment arms. The joint asymptotic normality is useful for testing more general treatment effects.
At the end of the discussion, we would like to point out that regression adjustment for covariate-adaptive randomisation has been extended to high-dimensional settings where the number of covariates is comparable to or even larger than the sample size, see Ma et al. (Citation2020a) for more details.
Disclosure statement
No potential conflict of interest was reported by the author(s).
Additional information
Funding
Notes on contributors
Hanzhong Liu
Hanzhong Liu, Associate Professor, Center for Statistical Science, Department of Industrial Engineering, Tsinghua University, Beijing, China.
References
- Bugni, F. A., Canay, I. A., & Shaikh, A. M. (2018). Inference under covariate-adaptive randomization. Journal of the American Statistical Association, 113(524), 1784–1796. https://doi.org/https://doi.org/10.1080/01621459.2017.1375934
- Bugni, F. A., Canay, I. A., & Shaikh, A. M. (2019). Inference under covariate-adaptive randomization with multiple treatments. Quantitative Economics, 10(4), 1747–1785. https://doi.org/https://doi.org/10.3982/QE1150
- Liu, H., & Yang, Y. (2020). Regression-adjusted average treatment effect estimates in stratified randomized experiments. Biometrika, 107(4), 935–948. https://doi.org/https://doi.org/10.1093/biomet/asaa038
- Ma, W., Tu, F., & Liu, H. (2020a). A general theory of regression adjustment for covariate-adaptive randomization: Ols, lasso, and beyond. arXiv:2011.09734.
- Ma, W., Tu, F., & Liu, H. (2020b). Regression analysis for covariate-adaptive randomization: A robust and efficient inference perspective. arXiv:2009.02287.
- Wang, B., Ogburn, E. L., & Rosenblum, M. (2019). Analysis of covariance in randomized trials: More precision and valid confidence intervals, without model assumptions. Biometrics, 75(4), 1391–1400. https://doi.org/https://doi.org/10.1111/biom.v75.4
- Wang, B., Susukida, R., Mojtabai, R., Amin-Esmaeili, M., & Rosenblum, M. (2019). Model-robust inference for clinical trials that improve precision by stratified randomization and adjustment for additional baseline variables. arXiv:1910.13954.
- Yang, L., & Tsiatis, A. A. (2001). Efficiency study of estimators for a treatment effect in a pretest–posttest trial. The American Statistician, 55(4), 314–321. https://doi.org/https://doi.org/10.1198/000313001753272466
- Ye, T., & Shao, J. (2018). Robust tests for treatment effect in survival analysis under covariate-adaptive randomization. arXiv:1811.07232.
- Ye, T., Shao, J., & Zhao, Q. (2020). Principles for covariate adjustment in analyzing randomized clinical trials. arXiv:2009.11828.
- Ye, T., Yi, Y., & Shao, J. (2020). Inference on average treatment effect under minimization and other covariate-adaptive randomization methods. arXiv:2007.09576.