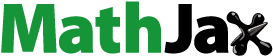
In the past decade, significant progress has been made regarding inference under covariate-adaptive randomisation. We thank Prof. Shao for a timely review of the growing literature about the topic. The paper is focused on the most important and commonly used class of covariate-adaptive randomisation methods, i.e., those balancing discrete covariates. The recent advances in robust inference are emphasised and discussed in detail. Several types of outcomes, such as continuous and time-to-event data, are covered. We here provide some additional recent results from the following five perspectives.
1. Balance discrete covariates with covariate-adaptive randomisation
In practice, covariate-adaptive randomisation is usually based on discrete covariates. Two of the most commonly used methods are stratified permuted block design and Pocock and Simon's minimisation. Y. Hu and Hu (Citation2012) proposed a unified framework of covariate-adaptive design that is able to control various types of imbalances, including within-stratum, within-covariate-margin, and overall imbalances. In particular, by choosing different weights in the imbalance measure, the proposed method includes stratified randomisation and minimisation as special cases. The theoretical balancing properties were given in Y. Hu and Hu (Citation2012) under some strong conditions that do not apply to minimisation. To the best of our knowledge, the theoretical results of minimisation were first developed in Ma et al. (Citation2015). The general results under widely satisfied conditions are completely established in a recent paper by F. Hu and Zhang (Citation2020). The proposed methods were also generalised to the cases of unequal allocation and multiple treatments (F. Hu & Zhang, Citation2020; Z. Liu et al., Citation2017).
Although the original paper of Y. Hu and Hu (Citation2012) did not explicitly study the inferential properties under the proposed designs, some model-based inference results were derived in Ma et al. (Citation2015). The robust inference under Hu and Hu's method is recently discussed in Ma, Tu, et al. (Citation2020).
2. Balance continuous covariates with covariate-adaptive randomisation
In clinical trials, it is common that some important baseline covariates are continuous. In practice, these continuous covariates are usually discretised in order to be used for stratified randomisation or minimisation. To avoid information loss due to discretization and uncertainties in determining the cut-off points, some emerging methods that directly handle continuous covariates are gaining popularity. See F. Hu et al. (Citation2014) for more discussions.
Therefore, it is of interest to consider inference under covariate-adaptive randomisation that balances continuous covariates. Compared to the methods dealing with discrete covariates, however, the results are somewhat limited. In X. Li et al. (Citation2019), a covariate-adaptive randomisation method is proposed to balance the means of a univariate covariate, and the corresponding inferential properties are given following the work of Ma et al. (Citation2015). More recently, Ma, Qin, et al. (Citation2020) study inference for general covariate-adaptive randomisation. Besides stratification and minimisation, the results also apply to the methods balancing for continuous covariates, including some classical optimality-based methods such as Atkinson's -biased coin design (Atkinson, Citation1982), as well as some newly proposed method (Qin et al., Citation2018). It should be noted that the works mentioned above fall in the category of model-based inference, and it would be interesting also to consider robust inference under these general covariate-adaptive randomisation methods.
3. Data quality issues
In the literature, most studies concerning inference under covariate-adaptive randomisation assume that the data quality is perfect. However, some data quality issues are inevitable in clinical trials and other comparative studies. Some recent works have been devoted to study the impact of different types of imperfect data on covariate-adaptive randomisation.
When discrete covariates are used in covariate-adaptive randomisation, the misclassification in the covariates has a twofold impact: it impairs the intended covariate balance and causes concerns over the validity of inference. In the linear model framework, the impact of misclassification on covariate-adaptive randomised trials has been studied in Wang and Ma (Citation2020) from both design and inference perspectives. For generalised linear models, some limited simulation results are available (Fan et al., Citation2018), and we are working on providing rigorous theoretical justifications by extending some recent results without considering covariate misclassification (Y. Li et al., Citation2020).
Another topic that draws some attention recently is about unobserved covariates in covariate-adaptive randomised experiments. There have been concerns on the use of covariate-adaptive randomisation if some important covariates cannot be observed. In the recent work of Y. Liu and Hu (Citation2020), the balancing properties of unobserved covariates are studied theoretically when stratification and covariates-adaptive randomisation are used. The results provide some foundation to study further the impact of unobserved covariates on inference following these randomisation procedures (Y. Liu & Hu, Citation2021).
Other examples of data quality issues include missing data and non-compliance. Interested readers can consult Wang et al. (Citation2019) and Bugni and Gao (Citation2021) for more discussions.
4. Combine covariate-adaptive randomisation with other adaptive designs
Covariate-adaptive randomisation can not only be used standalone, but can also be used in more complex settings with other adaptive design features, such as interim analyses and seamless trials. Building upon recent advances in inference under covariate-adaptive randomisation, some theoretical foundations have been established for implementing covariate-adaptive randomisation with some commonly used adaptive designs, such as sequential monitoring (Zhu & Hu, Citation2019) and sample size re-estimation (X. Li et al., Citation2021). Also, Ma, Wang, et al. (Citation2020) considered the adaptive seamless phase II/III clinical trials with covariate-adaptive randomisation and provided an adjusted test to control the type I error rate and improve the power. Since multiple treatments are usually involved in seamless trials, the multiple-comparison issue was also addressed for covariate-adaptive randomisation, which is an important problem in its own right.
5. Robust inference under covariate-adaptive randomisation
Very recently, the topic of robust inference under covariate-adaptive randomisation has drawn a lot of attention. Compared with model-based inference, robust inference does not require a correctly-specified data generation model and is thus more flexible in certain scenarios. When the average treatment effect is of interest, several approaches have been proposed to achieve robust inference. In addition to the results already mentioned in the review, the topic is also tackled from the perspective of regression adjustment. Ma, Tu, et al. (Citation2020) assessed several of the most commonly used regression models for estimating and inferring the treatment effect and showed that all these regression-based estimators can consistently estimate the treatment effect, although the efficiency is different. Consistent non-parametric variance estimators were also proposed to facilitate valid inference. Furthermore, when the additional baseline covariates are high-dimensional, it is desirable to use Lasso and other modern regression and machine learning techniques to further improve the efficiency (H. Liu et al., Citation2020).
Acknowledgments
This work was partly supported by National Natural Science Foundation of China (Grant Nos. 11801559, 12031005).
Disclosure statement
No potential conflict of interest was reported by the author(s).
Additional information
Funding
Notes on contributors
Wei Ma
Wei Ma is Assistant Professor at Institute of Statistics and Big Data, Renmin University of China.
Li-Xin Zhang
Li-Xin Zhang is Professor at School of Mathematical Sciences, Zhejiang University.
Feifang Hu
Feifang Hu is Professor at Department of Statistics, George Washington University.
References
- Atkinson, A. C. (1982). Optimum biased coin designs for sequential clinical trials with prognostic factors. Biometrika, 69(1), 61–67. https://doi.org/https://doi.org/10.1093/biomet/69.1.61
- Bugni, F. A., & Gao, M. (2021). Inference under covariate-adaptive randomization with imperfect compliance. arXiv:2102.03937.
- Fan, L., Yeatts, S. D., Wolf, B. J., McClure, L. A., Selim, M., & Palesch, Y. Y. (2018). The impact of covariate misclassification using generalized linear regression under covariate–adaptive randomization. Statistical Methods in Medical Research, 27(1), 20–34. https://doi.org/https://doi.org/10.1177/0962280215616405
- Hu, F., Hu, Y., Ma, Z., & Rosenberger, W. F. (2014). Adaptive randomization for balancing over covariates. Wiley Interdisciplinary Reviews: Computational Statistics, 6(4), 288–303. https://doi.org/https://doi.org/10.1002/wics.1309
- Hu, F., & Zhang, L. X. (2020). On the theory of covariate-adaptive designs. arXiv:2004.02994.
- Hu, Y., & Hu, F. (2012). Asymptotic properties of covariate-adaptive randomization. Annals of Statistics, 40(3), 1794–1815. https://doi.org/https://doi.org/10.1214/12-AOS983
- Li, X., Ma, W., & Hu, F. (2021). Sample size re-estimation for covariate-adaptive randomized clinical trials. Statistics in Medicine. https://doi.org/https://doi.org/10.1002/sim.8939
- Li, X., Zhou, J., & Hu, F. (2019). Testing hypotheses under adaptive randomization with continuous covariates in clinical trials. Statistical Methods in Medical Research, 28(6), 1609–1621. https://doi.org/https://doi.org/10.1177/0962280218770231
- Li, Y., Ma, W., Qin, Y., & Hu, F. (2020). Testing for treatment effect in covariate-adaptive randomized clinical trials with generalized linear models and omitted covariates. arXiv:2009.04136.
- Liu, H., Tu, F., & Ma, W. (2020). A general theory of regression adjustment for covariate-adaptive randomization: OLS, Lasso, and beyond. arXiv:2011.09734.
- Liu, Y., & Hu, F. (2020). Balancing unobserved covariates with covariate-adaptive randomized experiments. Journal of the American Statistical Association, in press. https://doi.org/https://doi.org/10.1080/01621459.2020.1825450
- Liu, Y., & Hu, F. (2021). The impact of unobserved covariates on covariate-adaptive randomized experiments. Working paper.
- Liu, Z., Ban, T., & Huang, T. (2017). General covariate-adaptive randomization targeting unequal allocation ratio. Journal of Statistical Planning and Inference, 191(2), 68–80. https://doi.org/https://doi.org/10.1016/j.jspi.2017.05.008
- Ma, W., Hu, F., & Zhang, L. X. (2015). Testing hypotheses of covariate-adaptive randomized clinical trials. Journal of the American Statistical Association, 110(510), 669–680. https://doi.org/https://doi.org/10.1080/01621459.2014.922469
- Ma, W., Qin, Y., Li, Y., & Hu, F. (2020). Statistical inference for covariate-adaptive randomization procedures. Journal of the American Statistical Association, 115(531), 1488–1497. https://doi.org/https://doi.org/10.1080/01621459.2019.1635483
- Ma, W., Tu, F., & Liu, H. (2020). Regression analysis for covariate-adaptive randomization: A robust and efficient inference perspective. arXiv:2009.02287.
- Ma, W., Wang, M., & Zhu, H. (2020). Seamless phase II/III clinical trials with covariate adaptive randomization. Statistica Sinica. https://doi.org/10.5705/ss.202019.0354.
- Qin, Y., Li, Y., Ma, W., & Hu, F. (2018). Pairwise sequential randomization and its properties. arXiv:1611.02802v2.
- Wang, B., Susukida, R., Mojtabai, R., Amin-Esmaeili, M., & Rosenblum, M. (2019). Model-robust inference for clinical trials that improve precision by stratified randomization and adjustment for additional baseline variables. arXiv:1910.13954.
- Wang, T., & Ma, W. (2020). The impact of misclassification on covariate-adaptive randomized clinical trials. Biometrics. https://doi.org/10.1111/biom.13308
- Zhu, H., & Hu, F. (2019). Sequential monitoring of covariate-adaptive randomized clinical trials. Statistica Sinica, 29(1), 265–282. https://doi.org/10.5705/ss.202016.0330.