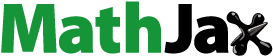
Combining information from similar studies has attracted substantial attention and continues to become increasingly important to assemble quality evidence in comparative effectiveness research. To my knowledge, this is the first paper to systematically review classical and up-to-date methods on how different statistical methods, such as meta-analysis, empirical likelihood (EL), renewal estimation and incremental inference, can be applied to incorporate information from multiple sources. This review paper succinctly presents both basic and advanced issues and will be greatly beneficial for researchers who are interested in this field.
Because of the wide array of related methods, this paper consists of cohesive but relatively independent sections. Although it is a review paper, the focus and contents are quite different from those of original papers. For example, an optimal combination of two estimators from two independent studies is derived by two methods from different perspectives: a linear combination with the smallest asymptotic variance and the maximum likelihood method. Another example is how to select a more efficient way to synthesize auxiliary information from other studies. In Section 5 of the review paper, two different sets of constraints, in which one involves parameter of interest and the other does not, have been presented and compared in terms of efficiency improvement. Both statistical intuition and theoretical justification are provided, which help readers create a better way to combine aggregate information for improved efficiency in practice. Such insightful discussions are not easily found elsewhere. The paper also nicely derives the conclusion that, similar to parametric-likelihood-based meta-analysis, the calibration methods (e.g., EL and generalized method of moments (GMM)) based on aggregate information have no efficiency loss compared to these methods using all individual data. Such deep insight into these methods greatly promotes their use for information calibration, since it is always challenging to obtain individual-level data.
As stated in the title, this review paper mainly focuses on statistical methods using calibration information from similar studies. One crucial assumption of these methods is homogeneity between the cohort with individual data (e.g., target cohort) and these similar studies (e.g., external sources). When the calibration information from the external sources are not comparable with those of the target cohort, such calibration methods may result in severe bias in estimation and misleading conclusions (Chen et al., Citation2021; Huang et al., Citation2016). One way to address this issue is to test the comparability by comparing calibration information between the target cohort and external sources before combining such information. Using the setup in Section 4 of the review paper as an example, assume that the auxiliary information from external sources is the mean of Y by subgroups (e.g., subgroups determined by covariates such as age and gender),
(1)
(1)
Then the compatibility can be checked by testing the difference between the sample average of outcomes within
from the individual-level data of the target cohort and the auxiliary information from external sources using a Wald-type test. However, the conclusion of this strategy is borrowing/no borrowing, which does not fully make use of comparable/discrepant degree between the two different sources.
Chen et al. (Citation2021) proposed a penalized constraint EL to accommodate the potential discrepancy between the target cohort and auxiliary information from external sources, and Li et al. (Citation2022) used a similar principal in transfer learning. Basically, the penalized likelihood allows us to determine the degree of information borrowing from the external sources by making use of a penalty term with a tuning parameter. Although the method with the penalty on discrepancy was developed under the EL framework, it can be easily extended to other estimating methods such as GMM. Using the setup in Section 4 of the review paper as an illustrating example, define the mean of Y in the subgroup of the target cohort as
, i.e.,
(2)
(2)
When integrating the auxiliary information with the target cohort, the comparability assumption would be violated if there existed at least one k such that
. We introduce a K-dimensional vector parameter
to incorporate the potential incomparable information for
:
. We need to estimate
and identify non-zero
s to adaptively combine the auxiliary information from the external sources.
Note that Equation (Equation2(2)
(2) ) can be expressed equivalently to
Define
, where
is the score equation, and
is the estimating equation to link the target cohort with auxiliary information. We can then construct a penalized GMM objective function, which combines the above estimating equations and a penalty term with a tuning parameter γ on the potential differences characterized by
,
(3)
(3)
Here,
is the sample variance of the estimating equations with initial but consistent estimators
:
. The penalty function could be the smoothly clipped absolute deviation (SCAD) penalty or least absolute shrinkage and selection operator (LASSO), which have been well studied in the literature and have many desirable properties (Fan & Li, Citation2001; Tibshirani, Citation1996). The tuning parameter
can be selected by minimizing the Bayesian information criterion (BIC)-like criterion (Kai et al., Citation2011; Wang et al., Citation2007). It will be interesting to investigate how this framework can be used in various methods reviewed by Qin et al. to combine information from multiple sources without assuming comparability.
Although there is a substantial amount of literature on statistical methods using calibration information from other sources, user-friendly computational tools for implementation are limited. We can look forward in the future to having well-documented and publicly available software such as R packages for practical applications in information integration, which are expected to have direct and high impact on comparative effectiveness research.
Disclosure statement
No potential conflict of interest was reported by the author(s).
References
- Chen, Z., Ning, J., Shen, Y., & Qin, J. (2021). Combining primary cohort data with external aggregate information without assuming comparability. Biometrics, 77(3), 1024–1036. https://doi.org/10.1111/biom.v77.3
- Fan, J., & Li, R. (2001). Variable selection via nonconcave penalized likelihood and its oracle properties. Journal of the American Statistical Association, 96(456), 1348–1360. https://doi.org/10.1198/016214501753382273
- Huang, C.-Y., Qin, J., & Tsai, H.-T. (2016). Efficient estimation of the Cox model with auxiliary subgroup survival information. Journal of the American Statistical Association, 111(514), 787–799. https://doi.org/10.1080/01621459.2015.1044090
- Kai, B., Li, R., & Zou, H. (2011). New efficient estimation and variable selection methods for semiparametric varying-coefficient partially linear models. Annals of Statistics, 39(1), 305–332. https://doi.org/10.1214/10-AOS842
- Li, S., Cai, T. T., & Li, H. (2022). Transfer learning for high-dimensional linear regression: prediction, estimation, and minimax optimality. Journal of the Royal Statistical Society. Series B, Statistical Methodology, 84(1), 149–173. https://doi.org/10.1111/rssb.v84.1
- Tibshirani, R. (1996). Regression shrinkage and selection via the lasso. Journal of the Royal Statistical Society, Series B, 58(1), 267–288. https://doi.org/10.1111/j.2517-6161.1996.tb02080.x
- Wang, H., Li, R., & Tsai, C. L. (2007). Tuning parameter selectors for the smoothly clipped absolute deviation method. Biometrika, 94(3), 553–568. https://doi.org/10.1093/biomet/asm053