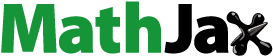
Qin, Liu and Li (henceforth QLL) review methods for combining information using empirical likelihood and related approaches; many of these ideas originated in the earlier work of Jing Qin. I thank the authors for their review, and for the opportunity to contribute to its discussion. I have little to say about technical aspects, which are well established but will comment briefly on broader aspects of data integration, and implications for methods like those in the article. I will focus on settings where there is a response variable Y and covariates X, Z and assume the target of inference is either the distribution of Y given X, Z or the ‘marginal’ distribution
of Y given X. In health research Y might represent (time to) the occurrence of some specific event, and X, Z covariates, exposures or interventions. The distribution
is important for individual-level decisions; in settings where X represents interventions
is relevant in randomized trials and comparative effectiveness research.
The authors consider two main topics in data integration: (i) the use of external auxiliary data to augment the analysis of a specific ‘internal’ study, and (ii) the combination of data from separate studies with a view to estimation or tests for common parameters or features. They focus on situations where, aside from the internal study in the topic (i), data are in summarized form; they note results showing that combining summary information from each study is asymptotically as efficient as combining individual-level data and show that EL methods have this property. However, these results make strong assumptions about distributions of variables being the same in the different studies; failures of these assumptions for methods like those in the article can lead to biased estimation or to estimates whose interpretation is unclear. Various authors (e.g. Estes et al., Citation2018; Han and Lawless 2016; Han & Lawless, Citation2019) have for example shown that for topic (i), estimates of covariate effects in a model for using augmentation with external data on
can be significantly biased when the distributions of
in the internal study and external data source differ. For topic (ii), heterogeneity of parameter values across studies is well recognized in meta-analysis, and is often addressed by incorporating random study effects (QLL, Section 2.2; DerSimonian & Laird Citation1986); however, interpretation of combined estimates is often problematic.
For the topics considered, there is in general no substitute for a comparison of data sources so as to assess their compatibility. Individual-level data are typically needed to compare distributions across data sources and to validate assumptions used for data integration, though in some settings this can be done with sufficiently granular summary data. Consider topic (i) and an internal study that records data , with the focus on inference for
; external auxiliary summary data are available for (
. It is possible (e.g. Han & Lawless, Citation2019; Kundu, Tang, & Chatterjee, Citation2019) to test whether the joint distribution of
is the same in the internal study population and the population by providing a summary auxiliary information. However, aside from settings where these two populations are the same (e.g. as in the use of calibration in survey methodology, or in two-phase studies where phase 1 and phase 2 samples are drawn from the same population), this is rarely the case; distributions of
usually differ, and the conditional distributions
may also differ. At a minimum, checks of compatibility should involve a comparison of summary external data with analogous data from the internal study. This might require ingenuity or additional auxiliary data in some settings, for example, if the internal study is a case–control study providing information on
and the external data provide information on
.
Topic (ii), discussed in QLL Section 6, has a huge literature, much of it under the heading of meta-analysis. Consider studies as in QLL and estimation of a regression coefficient
based on a (generalized) linear model for
; this is common in meta-analyses of randomized trials. Distributions or models that condition on multiple covariates are more likely to be similar across populations than marginal models (e.g. Keiding & Louis, Citation2016), so let's assume the conditional distributions
are the same across studies, where Z represents some ‘maximal’ set of covariates. If there is an XZ interaction in the distribution
then the coefficients
differ unless
has the same distribution in each study population. This is uncommon even when X represents a randomly assigned treatment in each study and so is independent of Z. Variation in treatment effects
motivates random effects meta-analysis where, as outlined by QLL (Section 2.2), studies
are assumed to target a common estimand θ, but the values
may vary across studies according to a model with
. Parameters
and τ can be estimated (DerSimonian & Laird Citation1986) but a key question is how to interpret
, especially if the
do not measure exactly the same thing. In the illustration here, regression coefficients
represent marginal effects of X in each study population. One might say this means the same thing in each population but if the distribution of Z and the
vary substantially across studies, combining them may make little sense. DerSimonian and Laird (Citation2015) view meta-analysis ‘as providing an overall summary of what has been learned, as well as a quantitative measure of how results differ, above and beyond sampling error’ but recognize problems associated with very diverse results, and the need to examine individual study conditions to assess sources of differences. Meta regression (e.g. Thompson and Higgins (Citation2002)), in which study-level covariates are used to explain variation in estimates across studies, can be applied more broadly to data integration that is based on summary measures. Analyses of variation in results across studies provide the most valuable insights in some situations.
Finally, it is not the focus of QLL, but many authors have recently discussed the combination of information for the formulation of predictive models (e.g. Gu et al., Citation2021; Kundu, Tang, & Chatterjee, Citation2019). Models that give predictive probabilities are best compared using scoring rules that address both calibration and sharpness (e.g. Gneiting & Katzfuss, Citation2014); the Brier Score is a common measure for assessing predictive distributions for binary outcomes Y such as experiencing (Y = 1) or not experiencing (Y = 0) some event by a given time t. Papers often focus on improvements in the estimation of regression coefficients from combining data or models from different sources. However, for well-calibrated models, substantial gains in predictive performance arise mostly from the discovery and incorporation of new covariates or covariate effects with high explanatory power. Increases in the precision of estimation usually have a small effect and gains in predictive performance from data integration alone tend to be modest (e.g. see Kundu, Tang, & Chatterjee, Citation2019).
Disclosure statement
No potential conflict of interest was reported by the author(s).
References
- Dersimonian, R., & Laird, N. M. (1986). Meta-analysis in clinical trials. Controlled Clinical Trials, 7, 177–188.
- DerSimonian, R., & Laird, N. M. (2015). Meta-analysis in clinical trials revisited. Contemporary Clinical Trials, 45(2), 139–145. https://doi.org/10.1016/j.cct.2015.09.002
- Estes, J. P., Mukherjee, B., & Taylor, J. M. G. (2018). Empirical Bayes estimation and prediction using summary-level information from external big data sources adjusting for violations of transportability. Statistics in Biosciences, 10(3), 568–586. https://doi.org/10.1007/s12561-018-9217-4
- Gneiting, T., & Katzfuss, M. (2014). Probabilistic forecasting. Annual Review of Statistics and Its Application, 1(1), 125–151. https://doi.org/10.1146/statistics.2013.1.issue-1
- Gu, T., Taylor, J. M. G., & Mukherjee, B. (2021). A meta-inference framework to integrate multiple external models into a current study. Biostatistics (Oxford, England), https://doi.org/10.1093/biostatistics/kxab017.
- Han, P., & Lawless, J. F. (2019). Empirical likelihood estimation using auxiliary summary information with different covariate distributions. Statistica Sinica, 29(3), 1321–1342. https://doi.org/10.5075/ss.202017.0308
- Keiding, N., & Louis, T. A. (2016). Perils and potentials of self-selected entry to epidemiological studies and surveys (with discussion). Journal of the Royal Statistical Society: Series A, 179(2), 319–376. https://doi.org/10.1111/rssa.12136
- Kundu, P., Tang, R., & Chatterjee, N. (2019). Generalized meta-analysis for multiple regression models across studies with disparate covariate information. Biometrika, 106(3), 567–585. https://doi.org/10.1093/biomet/asz030
- Thompson, S. G., & Higgins, J. P. T. (2002). How should meta-regression analyses be undertaken and interpreted? Statistics in Medicine, 21(11), 1559–1573. https://doi.org/10.1002/(ISSN)1097-0258