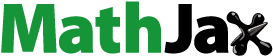
It is our pleasure to have an opportunity of making comments on this fine work in that the authors present a comprehensive review on empirical likelihood (EL) methods for integrative data analyses. This paper focuses on a unified methodological framework based on EL and estimating equations (EE) to sequentially combine summary information from individual data batches to obtain desirable estimation and inference comparable to those obtained by the EL method utilizing all individual-level data. The latter is sometimes referred to as an oracle estimation and inference in the setting of massively distributed data batches. An obvious strength of this review paper concerns the detailed theoretical properties in connection to the improved estimation efficiency through the utility of auxiliary information.
In this paper, the authors consider a typical data integration situation where individual-level data from the Kth data batch is combined with certain ‘good’ summary information from the previous K−1 data batches. While appreciating the theoretical strengths in this paper, we notice a few interesting aspects that are worth some discussions.
Distributed data structures: In practice, both individual data batch size and the number of data batches may appear rather heterogeneous, requiring different theory and algorithms in the data analysis. Such heterogeneity in distributed data structures is not well aligned with the methodological framework reviewed in the paper. One important practical scenario is that the number of data batches tends to infinity. Such setting may arise from distributed data collected from millions of mobile device users, or from electronic health records (EHR) data sources distributed across thousands of hospitals. In the presence of massively distributed data batches, a natural question pertains to a trade-off between data communication efficiency and analytic approximation accuracy. Although one-round data communication is popular in this type of integrative data analysis, multiple rounds of data communication may be also viable in the implementation via high-performance computing clusters. Our experience suggests that sacrifice in the flexibility of data communication (e.g., limited to one-round communication in the Hadoop paradigm), although enjoys computational speed, may pay a substantial price on the loss of approximation accuracy, leading to potentially accumulated estimation bias when the number of data batches increases. This issue of estimation bias is a technical challenge in nonlinear models due to the invocation of approximations to linearize both estimation procedure and numerical search algorithm. On the other hand, relaxing the restrictions on data communication, such as the operations within the lambda architecture, can help reduce the approximation error and lower estimation bias. Clearly, the latter requires more computational resources.
This important issue was investigated by Zhou et al. (Citation2022) that studied asymptotical equivalence between distributed EL estimator and oracle EL estimator under both one-round communication and unlimited rounds of communication when the number of distributed data batches increases perpetually. They found that under one-round communication, if the number of data batches, K, increases with the sample size n at a slow order of with
and all individual batch sizes increase (i.e.,
), their proposed distributed EL estimator is asymptotically equivalent to the oracle EL estimator in the mode of convergence in distribution. Interestingly, they found that if there is no limit on communication, both technical conditions above can be removed, and moreover, under much weaker conditions the distributed EL estimator and the oracle EL estimator are asymptotically equivalent in the mode of convergence in probability. The latter is a stronger convergence result than the former. Furthermore, assisted by the ADMM algorithm, even if there exist serious unbalanced covariate distributions in several data batches, the distributed EL estimator can still work well, while the conventional meta methods fail miserably.
Heterogeneity: Good theoretical properties, including estimation consistency and asymptotic normality as well as estimation efficiency, are reviewed in this paper. We notice that these theoretical properties are established under a big assumption of a homogeneous underlying data generating mechanism and a homogeneous statistical model across all data batches. In practice, this assumption can be easily violated, especially when the number of data batches increases. Generally speaking, bias and variance trade-off is a common criterion in statistical analysis. With distributed data, heterogeneity issues are unavoidable. Aggregating information from heterogeneous data batches using a homogeneous modelling approach could suffer severe estimation bias and failure in inference. This is the well-known fact that the bias of an estimator is in fact a more dominant issue than its variance when the volume of the data at hand is big. Thus, investigating similarity among available data batches is a critical step in the early stage of analyzing distributed data.
Addressing both data and model heterogeneity has been extensively considered in the literature of distributed data analyses. For example, federated learning (McMahan et al., Citation2017) aims to find effective methods to borrow information across similar datasets while accounting for individualized heterogeneity. Li et al. (Citation2020) utilized a proximal notion specific to each local objective to tackle heterogeneity in federated network learning; Collins et al. (Citation2021) and Fallah et al. (Citation2020) considered federated learning of a shared data representation or models across data batches; Smith et al. (Citation2017) focused on studying heterogeneous models via multi-task learning (or meta learning) by shared sparsity across different models.
As mentioned above, unbalanced covariate distributions or uneven dimensions of covariates across data batches are pervasive in practice. Little work is available in the literature to handle this technical challenge. Zhou et al. (Citation2022) considered a simple case of unbalanced covariate distribution with the same dimension of covariates across data batches. With the help of the ADMM algorithm, their proposed distributed EL estimator worked well. In addition, some researchers studied non-IID data in the development of distributed estimation and inference. For example, renewable estimation and incremental inference proposed by Luo et al. (Citation2022) allow to sequentially update both estimation and inference for clustered data steams; Wang et al. (Citation2012) proposed an integrative analysis of distributed longitudinal data; Hector and Song (Citation2021) considered a distributed generalized method of moments (GMM) for multi-dimensional outcomes with a diverging dimension; and Tang et al. (Citation2020) utilized the confidence distribution approach to establish a distributed lasso estimation in distributed datasets, just to name a few.
Implementation: One noted aspect missing in this paper is the lack of review on algorithms and software packages related to implementation. There are some R software packages available in the literature, such as R package DDIMM (Hector & Song, Citation2020) to perform data integration with dependent data sources, and R package metafuse (Tang & Song, Citation2016) to fuse heterogeneous parameters across independent data sources into subgroups. Both algorithms and software packages play important roles in translational research, which leads to broader impacts.
Some future directions: The authors have built up an interesting framework that may motivate many important future research problems. With our limited knowledge in this field, we humbly suggest three. First, despite the unified framework that seems appealing in the low-dimensional case, high-dimensional data would present a great challenge related to potentially heavy computational burdens, in addition to notoriously hard problems regarding post-model selection inference. Second, in the big-data era, data with specific structures like spatially and/or temporally correlated data are pervasive. Extending the low-dimensional framework to handle distributed spatio-temporal data is an important direction. Third, for massive distributed datasets, outliers or contaminated data are ubiquitous. It is important to develop robust distributed EL methods to obtain reliable and stable results in both estimation and inference.
Disclosure statement
No potential conflict of interest was reported by the author(s).
Additional information
Funding
References
- Collins, L., Hassani, H., Mokhtari, A., & Shakkottai, S. (2021). Exploiting shared representations for personalized federated learning. Proceedings of the 38th International Conference on Machine Learning, PMLR 139 (pp. 2089–2099). https://proceedings.mlr.press/v139/collins21a.html
- Fallah, A., Mokhtari, A., & Ozdaglar, A. (2020). Personalized federated learning with theoretical guarantees: A model-agnostic meta-learning approach. Advances in Neural Information Processing Systems, 33, 3557–3568. https://proceedings.neurips.cc/paper/2020/file/24389bfe4fe2eba8bf9aa9203a44cdad-Paper.pdf
- Hector, E. C., & Song, P. X.-K. (2020). Doubly distributed supervised learning and inference with high-dimensional correlated outcomes. Journal of Machine Learning Research, 21(173), 1–35. http://jmlr.org/papers/v21/19-996.html
- Hector, E. C., & Song, P. X.-K. (2021). A distributed and integrated method of moments for high-dimensional correlated data analysis. Journal of the American Statistical Association, 116(534), 805–818. https://doi.org/10.1080/01621459.2020.1736082
- Li, T., Sahu, A. K., Zaheer, M., Sanjabi, M., Talwalkar, A., & Smith, V. (2020). Federated optimization in heterogeneous networks. Proceedings of Machine Learning and Systems, 2, 429–450. https://proceedings.mlsys.org/paper/2020/file/38af86134b65d0f10fe33d30dd76442e-Paper.pdf
- Luo, L., Zhou, L., & Song, P. X.-K. (2022). Real-time regression analysis of streaming clustered data with possible abnormal data batches. Journal of the American Statistical Association, 1–42. To appear. https://doi.org/10.1080/01621459.2022.2026778
- McMahan, B., Moore, E., Ramage, D., & Hampson, S. (2017). Communication-efficient learning of deep networks from decentralized data. Artificial intelligence and statistics, PMLR (pp. 1273–1282), Lauderdale, FL, USA. https://proceedings.mlr.press/v54/mcmahan17a.html
- Smith, V., Chiang, C.-K., Sanjabi, M., & Talwalkar, A. S. (2017). Federated multi-task learning. Advances in Neural Information Processing Systems, Curran Associates, Inc. (vol. 30), Long Beach, CA, USA. https://proceedings.neurips.cc/paper/2017/file/6211080fa89981f66b1a0c9d55c61d0f-Paper.pdf
- Tang, L., & Song, P. X. K. (2016). Fused lasso approach in regression coefficients clustering: Learning parameter heterogeneity in data integration. The Journal of Machine Learning Research, 17(113), 3915–3937. http://jmlr.org/papers/v17/15-598.html
- Tang, L., Zhou, L., & Song, P. X.-K. (2020). Distributed simultaneous inference in generalized linear models via confidence distribution. Journal of Multivariate Analysis, 176, Article 104567. https://doi.org/10.1016/j.jmva.2019.104567
- Wang, F., Wang, L., & Song, P. X.-K. (2012). Quadratic inference function approach to merging longitudinal studies: Validation and joint estimation. Biometrika, 99(3), 755–762. https://doi.org/10.1093/biomet/ass021
- Zhou, L., She, X., & Song, P. X.-K. (2022). Distributed empirical likelihood approach to integrating unbalanced datasets. Statistica Sinica. https://doi.org/10.5705/ss.202020.0330