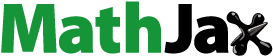
We thank Professor Jun Shao for organizing this interesting discussion. We also thank the six discussants for many insightful comments and suggestions. Assembling data from different sources has been becoming a very popular topic nowadays. In our review paper, we have mainly discussed many integration methods when internal data and external data share a common distribution, though the external data may not have information for some underlying variables collected in the internal study. Indeed the common distribution assumption is very strong in practical applications. Due to the technology advance, the collection of data is getting much easier, for example, by using i-phone, satellite image, etc. As those collected data are not obtained by well-designed probability sampling, inevitably, they may not represent the general population. As a consequence, there probably exists a systematic bias. In the survey sampling literature, how to combine survey sampling data with non probability sampling data has also got very popular (Chen et al., Citation2020). Without bias correction, most existing methods may produce biased results if the common distribution assumption is violated. One has to be careful to assess the impartiality before data integration.
Before we respond to the common concern by the reviewers on the heterogeneity among different studies, we first outline the possible distributional shifts or changes in each source data. In the machine learning literature, the concepts of covariate shift, label shift, and transfer learning have been widely used (Quiñonero-Candela et al., Citation2009). We briefly highlight those concepts in terms of statistical joint density or conditional density.
Covariate shift: Let Y and X be, respectively, the outcome and a vector of covariates in Statistic terminology, or a label variable and a vector of features in Machine Learning Languish. Suppose we have two data-sets:
where
and
are the joint density function of
, the conditional density function of Y given X = x, the marginal density function of X, the conditional density function of X given Y = y, and the marginal density function of Y, respectively. The subscript k = 0 and 1 correspond to the training data and the testing data, respectively. The covariate-shift assumption is
where the conditional density of Y given X remains unchanged from the training data to the testing data, but the marginal covariate distribution shifts. The most popular assumption on the shifted covariate distribution is
where
is a known density ratio.
Label shift: The popular label shift assumption in machine learning is
If the outcome Y is the status of a disease and X is symptoms, a problem of interest is to predict the disease status given the symptoms. In machine learning literature, people may make the anticasual assumption that it is the disease status causes the symptoms. In the label shift assumption, the conditional density of X given Y does not change between different studies, however, the marginal distribution of the disease status Y changes in different studies.
Transfer learning: Let be the conditional means for
. Suppose a parametric model is assumed for
in the training data, say,
, where
is known up to unknown finite dimensional parameter θ. A popular assumption in transfer learning is
where g is a monotone function depending on an unknown parameter η. For a low dimensional covariate case, one may assume
. In the high dimensional covariate case, on the other hand, one may assume
to be 0 for most components of Δ. Then penalized likelihood methods can be applied to select those non-zero components.
1. Response to Professor Lawless
We would like to thank Professor Lawless for his insightful comments. We totally agree with his view on testing the compatibility before combining internal and external data together.
Suppose the internal data and external summarized information derived from
are available. Since
is not available, we may not be able to test
even if
are available. The best we can do is to test the joint distributions of
from the internal data and external data are the same if both of them are available. If a small portion of external data
is also available, certainly it is possible to test the distributional agreement between two sources of data.
The most popular approach in meta analysis is to estimate the mean treatment effect over different but similar studies. The basic assumption is that the true mean is the same across different studies, but to reflect the possible discrepancy among studies, a possible unexplained variation is assumed in each study. In general raw data from each study are unavailable except for summarized information. Later on, meta analysis was extended to the regression case, for example,
where
is the i-th outcome in j-th study,
;
. Again to allow the variation in each study, one may assume
. According to our understanding (easily can be wrong), even if the covariate distributions of
are quite different among studies, the regression coefficients β and γ can be estimated without any problem. On the other hand, if one is interested in estimating the marginal parameter such as the mean of
, then the simple combination of
(the sample version of
) is meaningless since
s vary across studies.
Professor Lawless has further pointed out the possibility of combining information for the formulation of predictive models. By discovering some new covariates, one may gain substantial gains in predictive performance. Nevertheless, the general methodology works are not well developed yet. A recent work by Efron (Citation2020) has indicated that in general the prediction problem is easier than the attribute estimation. Moreover, in the discussion of Professor Efron's paper, Xie and Zheng (Citation2020) disclosed that one may have a correct coverage for the prediction for a future value of the response even if the underlying model is incorrect as long as the independent and identically distributed structure remains true. However, a correct model will produce a confidence interval with the narrowest width.
2. Response to Professor Han
Building on the early work by Sheng et al. (Citation2021), Professor Han has suggested a calibration method in the covariate shift problem. Moreover, if the dimension of the covariate is large, Chen et al. (Citation2021) and Zhai and Han (Citation2022) have used a penalized likelihood method to regularize the underlying parameters. Definitely, the use of summarized information in high-dimensional parameter problems is welcome.
Professor Han has discussed a different approach to combine information. Let Y be a response variable, and X and Z be two covariates, where both X and Z are available in the internal data and only X and Y are available in the external data. In essence, they (Taylor et al., Citation2022) assume
(1)
(1)
and
where
is a known link function, and
and
are unknown parameters. Under the assumption that X and Z are independent or at least uncorrelated and that the covariate effects are closed to 0, they show
approximately, where α is an unknown scale parameter. Based on the external information
, they fit a model
(2)
(2)
The information gain in the newly formed model comes from the fact that it has a scale parameter α only instead of the vector parameters β in the original model. On the other hand, the compatibility of these two models is hard to satisfy, and more systematic works are needed.
3. Response to Professors Zhou and Song
In addition to echoing the same message that the heterogeneity among different studies and batches of data, Professors Zhou and Song have laid out many challenging issues in fusing different data sources, including the issue that the number of data batches tends to infinity, one-round communication and unlimited rounds of communications in the case that the number of data batches increases. Indeed, if the size of data gets very large, bias becomes critical since variance gets almost negligible.
Moreover, Professors Zhou and Song have given many useful references in the machine learning literature on information borrowing but accounting for individualized heterogeneity. It is indispensable to develop new and optimal algorithms to deal with large data and high dimensional problems. The three future directions outlined by Professors Zhou and Song are important and welcome.
4. Response to Professor Ning
Whether conventional statistical methods borrowing information from similar studies work depends on the testing conclusion of the hypothesis whether internal data and summarized external information are compatible. Professor Ning has advocated a systematic way to do so by using a similar principal to transfer learning. To accomplish this, Chen et al. (Citation2021) used the penalized likelihood method. More researches in this direction are welcome. Of course, user-friendly softwares are urgent to be developed.
5. Response to Professor Chen
We sincerely thank Professor Chen for the three crucial technical problems concerning empirical likelihood for estimation equations. Qin & Lawless (1994) assumed that the estimating function is smooth enough so that the usual Taylor series approximation method applies. With non-smooth estimating equations, the profile empirical likelihood function become a zigzag function and the Taylor series approximation method fails to work, making it challenging to establish the limiting distribution of the maximum empirical likelihood estimator (MELE). With the help of advanced empirical process theory, enough smoothness of the expected estimating function
are sufficient to guarantee the standard limiting distributions of the MELE and the empirical likelihood ratio (Molanes Lopez et al., Citation2009). About the global consistency of the MELE, although of great importance to the theoretical completeness of empirical likelihood, this fundamental property has not been rigorously established yet in the literature. We appreciate that Professor Chen has outlined a proof for the global consistency.
The last issue is on the efficiency issue of nonparametric maximum likelihood estimator (MLE). Many thanks to Professor Chen for pointing out our mistake in Section 3.4: , which does not hold. With slight modification, the conclusion there is still true. As Professor Chen suggested, we replace
by the true density function of X, say
. Define a enlarged parametric density function
which clearly includes
as a special case. In addition, we require that
satisfy
(3)
(3)
and
, where
is the true value of θ. In contrast to Back and Brown (Citation1992), our construction of the enlarged density is not from the exponential family.
Define . Given n observations
from
, the log-likelihood function is
Let
be the MLE under the parametric model
. Then under certain regularity conditions,
By equality (Equation3
(3)
(3) ),
where
takes expectation with respect to
. By this result and tedious algebra, we find that
where
is the asymptotic variance of the MELE
.
Consequently,
which implies that under the parametric model
, the MLE of θ has the asymptotic variance V. Thus, under only the general estimating equation model
, the best estimator of θ should also have an asymptotic variance at least as large as V. Because the MELE of θ of Qin & Lawless (1994) has the asymptotic variance V, we conclude that it achieves the semiparametric lower information bound, as claimed in Section 3.3 of our review paper.
6. Conclusion
The simple integration method may produce biased results in the presence of distribution shifts. When assembling information from different data sources, one has to understand the data generating process, accordingly, to make judiciously choices on different modelling methods. More importantly, characterizing the selection bias plays an extremely important role in data fusing.
Disclosure statement
No potential conflict of interest was reported by the author(s).
References
- Back, K., & Brown, D. P. (1992). GMM, maximum likelihood, and nonparametric efficiency. Economics Letters, 39(1), 23–28. https://doi.org/10.1016/0165-1765(92)90095-G
- Chen, Y., Li, P., & Wu, C. (2020). Doubly robust inference with non-probability survey samples. Journal of the American Statistical Association, 115(532), 2011–2021. https://doi.org/10.1080/01621459.2019.1677241
- Chen, Z., Ning, J., Shen, Y., & Qin, J. (2021). Combining primary cohort data with external aggregate information without assuming comparability. Biometrics, 77(3), 1024–1036. https://doi.org/10.1111/biom.v77.3
- Efron, B. (2020). Prediction, estimation and attribution. Journal of the American Statistical Association, 115(530), 636–655. https://doi.org/10.1080/01621459.2020.1762613
- Molanes Lopez, E. M., Van Keilegom, I., & Veraverbeke, N. (2009). Empirical likelihood for non-smooth criterion functions. Scandinavian Journal of Statistics, 36(3), 413–432. https://doi.org/10.1111/sjos.2009.36.issue-3
- Quiñonero-Candela, J., Sugiyama, M., Schwaighofer, A., & Lawrence, N. D. (2009). Dataset shift in machine learning. MIT Press.
- Sheng, Y., Sun, Y. F., Huang, C. Y., & Kim, M.-K. (2021). Synthesizing external aggregated information in the presence of population heterogeneity: a penalized empirical likelihood approach. Biometrics, 78(2), 679–690. https://doi.org/10.1111/biom.v78.2
- Taylor, J. M. G., Choi, K., & Han, P. (2022). Data integration – exploiting ratios of parameter estimates from a reduced external model. Biometrika. https://doi.org/10.1093/biomet/asac022
- Xie, M. G., & Zheng, Z. (2020). Discussion of professor Bradley efron's article on ‘prediction, estimation, and attribution’. Journal of the American Statistical Association, 115(530), 667–671. https://doi.org/10.1080/01621459.2020.1762614
- Zhai, Y., & Han, P. (2022). Data integration with oracle use of external information from heterogeneous populations. Journal of Computational and Graphical Statistics. https://doi.org/10.1080/10618600.2022.2050248