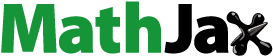
Abstract
The sample moment can be used to estimate the population third central moment, , in the Johnson’s modified t-statistic for skewed distributions. However, moment estimator is non-unique and insufficient for the parameter of population. In this paper, we display the maximum likelihood estimator (MLE) of
in modified t-statistic as parent distributions are asymmetrical. A Monte Carlo study shows that the MLE procedure is more powerful than Student’s t-test and ordinary Johnson’s modified t-test for a variety of positively skewed distributions with small sample sizes.
PUBLIC INTEREST STATEMENT
The effect of skewness of a random variable on test statistics has been a popular research topic in the statistics field. Student’s t-test is commonly adopted to test the null hypothesis. However, Student’s t-test may have power loss when the researches are focused on positively skewed data. This study proposed Johnson’s modified t-test with the maximum likelihood estimator (MLE) of the third central moment for positively skewed data. After controlling Type I error, half Johnson’s modified t-test could more significant than Student’s t-test for a demonstration of laboratory mice data. Johnson’s modified t-test with the MLE procedure is worth recommending for a variety of positively skewed distributions with small sample sizes.
1. Introduction
The central limit theorem is widely used when a random sample is drawn from a non-normal population with mean and variance
. It assumes that the mean
of a population is to be estimated. In practice, a random sample of size
would typically be taken from the population, and then the sample mean would be computed to estimate
. The sample mean can be defined as a random variable. Then, it varies from sample to sample and cannot be deterministically predicted. The notation
is used when the sample mean is defined as a random variable, and
for the corresponding values where
. The random variable
follows a sample distribution with mean
and standard deviation
. According to the central limit theorem, the sample mean
can be approximated by a normal distribution with mean
=
and standard deviation
=
for a large sample size
, where
is the standard deviation of the population. By this theorem, the test statistic
can be used to test the hypothesis that the mean of a non-normal population is
when it is known that standard deviation is
and the sample size is large.
The Student’s t-test was proposed to overcome the inefficiency of the z-test with small samples. The sample variance is used for the population variance if
is unknown. The Student’s t-test (i.e.,
) can be used for hypotheses where the sample standard deviation
is used to estimate
. It performs well when
is finite and the sample size is large. It is now assumed that the distribution of a random variable, such as the random variable
, should be studied. The first two moments (i.e., the mean and the variance) can be obtained as a step toward understanding the distribution, and the unbiased estimators for the mean and the variance can be obtained from a random sample. However, there are several situations that require higher-order moments. For a scenario where the sample size is small and the parent distribution is asymmetrical (e.g., Gamma distribution), Johnson (Citation1978) proposed a modified procedure for the Student’s t-test using the first few terms of the inverse Cornish–Fisher expansion, proposed by Cornish and Fisher (Citation1937), as follows:
where is the population third central moment. It can be estimated by the sample third central moment, denoted by
. When the hypothesis
is stated, the ordinary Johnson’s modified t-statistic is
where .
Under violations of both normality and variance homogeneity, Cressie and Whitford (Citation1986) examined the problem of using the conventional Student’s t-test with inappropriate standard deviation. The Welch's t-test is most frequently used to tackle the violations of classical assumptions. Alternatively, this situation can be improved by correcting the t variables using transformations, such as Johnson’s transformation and Hall’s transformation proposed by Hall (Citation1983).
For the asymmetric distribution of upper-tailed tests, Sutton (Citation1993) verified that Johnson’s -test could be used, as Student’s t-test lacks statistical power. Furthermore, it reduces the probability of Type I error. However, Johnson’s
-test may yield incorrect results if skewness is inflated and the sample size is small.
To test the mean of a positively skewed distribution with the upper-tailed test, Chen (Citation1995) conducted a novel testing procedure using the Edgeworth expansion under several positively skewed distributions, such as Gamma, Weibull, exponential, and lognormal. According to the results of a simulation study, the new test statistic is more powerful than Student’s t-values and Johnson’s -values regardless of which positively skewed distribution and critical value were selected.
To estimate the mean of asymmetric distributions, Johnson (Citation1978) proposed some modified t-tests that can be widely applied to the original distributions, from normal distributions to asymmetric distributions, for example, to exponential distributions with sample size as small as 13. In several real situations, owing to the cost limitations of the sampling procedures, when the sample size is small, the deviation of the original distribution may be larger than that in Johnson’s study. In this case, Johnson’s test may lack accuracy. To resolve this, Sutton (Citation1993) proposed an improved comprehensive test method to improve Johnson’s tail t-test. Chen (Citation1995) proposed an upper-tailed test method for the average of a positively skewed distribution. According to a Monte Carlo study, Chen’s test proved to be more accurate than Johnson’s modified t-test and Sutton’s compound test for various positively skewed distributions and small samples. Above related studies used sophisticated mathematical expansion to improve the accuracy of Johnson’s test.
Diaconis and Efron (Citation1983) proposed the time-consuming computer intensive method carried out to evaluate the small-sample behavior of the modifications in terms of Type I error rate and statistical power. However, relatively few studies have considered the statistical properties of different estimators of in the ordinary Johnson’s modified t-statistic. In this study, the maximum likelihood estimator (MLE) of
is proposed in such a modified t-statistic for asymmetrical parent distributions. A Monte Carlo simulation is performed to examine the statistical power of the MLE in the context of Johnson’s modified t-statistic for each scenario. It is demonstrated that this procedure is more powerful than both Student’s t-test and ordinary Johnson’s modified t-test for a variety of positively skewed distributions and small sample sizes.
2. MLE of μ3 for the upper-tailed test
Skewness can be used to measure the level of asymmetry of a probability distribution. The skewness coefficient can be positive or negative and is denoted by . It has a greater effect on a t-type variate compared with the kurtosis coefficient. Neyman and Pearson (Citation1928) and Pearson (Citation1928) demonstrated that the power of the short right tail in the sampling distribution of the Student’s t-test is small for upper-tailed tests of the population mean. Sutton (Citation1993) performed a Monte Carlo analysis to examine the statistical properties of Student’s t-test and Johnson’s modified t-test for skewed distributions. Sutton demonstrated that the power performance of Johnson’s modified t-test was better than that of the conventional t-test in several cases. When skewness was high, the Type I error was inaccurate for both tests, as the sample size was not sufficiently large. However, both procedures indicated a tendency for greater accuracy (in the Type I error) with an increase in sample size and a decrease in skewness.
In a field such as statistics, all inventions are necessarily conceptual. MLEs are arguably the most valuable invention in the history of statistics. Although MLEs are often mathematically non-trivial, and the likelihood equations are tractable only if they are specifically based on a given distribution, MLEs are still widely used in a large number of models. In general, maximum likelihood estimation can also be a different numerical application. This study begins with a familiar model, namely, the exponential family, as it is relatively simple from a computational perspective. The definition of the exponential family is as follows:
Definition 2.1. Let where
Suppose
is a probability mass function (or probability density function) that belongs to the one-parameter exponential family with natural parameter space
where
is called the natural parameter of
,
is called the natural statistic,
is the cumulant generating function, and
is the carrier density.
For simplicity, it is assumed that the shape parameters are known. Moreover, for completeness, the theorem on MLEs for belongs to the exponential family with parameter
is stated as follows:
Theorem 2.1. Let If
is the MLE of the parameter
, then
Here, three positively skewed distributions are considered: (i) a Weibull distribution, (ii) a Gamma distribution, and (iii) an exponential distribution. Of course, they belong to the one-parameter exponential family. The MLEs of the unknown parameters of these distributions are, according to Theorem 2.1, as follows.
Remark: (i) Weibull distribution ()
The density of a Weibull distribution with variable
, where
, is given by
where and
. Furthermore,
and
, where
is known.
(2) The MLE of
satisfies
. Then
Remark: (ii) Gamma distribution ()
(1) The density of a Gamma distribution with variable
, where
, is given by
where and
. Furthermore,
and
, where
is known.
(2) The MLE of
satisfies
. Then
Remark: (iii) Exponential distribution ()
(1) The density of an exponential distribution with variable
, where
, is given by
where and
. Furthermore,
and
.
(2) The MLE of
satisfies
. Then
According to the invariance property of MLE, it is convenient to derive the MLE of , denoted as
(see Appendix A) in each case. Then, the test statistic is
The decision rule for testing versus
is to reject
when
under a significance level of
. The theoretical derivation of
is provided in Appendix B.
3. Monte Carlo simulation
Chen (Citation1995) proposed a new procedure for the upper-tailed test of the means of positively skewed distributions. Monte Carlo analysis can be used to investigate the new procedure’s statistical properties in each case. Here, random samples are generated from positively skewed distributions with a range of values. These distributions are the Weibull (
), Gamma (
), Gamma (
), Gamma (
), Gamma (
), Gamma (
) and exponential (
) corresponding to the
values are 0.63, 0.87, 1.00, 1.32, 1.63, 1.83, and 2.00, respectively.
It should be noted that studies on test procedures use Student’s t-test () and Johnson’s modified t-test (
). For all tests, the rejection regions are based on the
-distribution. The notation of the parameters of the distribution is consistent with that in Mood, Graybill, and Boes (Citation1974). In this study, Monte Carlo samples of size 100,000 were generated for each simulation. The comparisons of the tests are based on the same conditions (i.e., sample size) to calculate the Type I error rate and the statistical power. For upper-tailed tests, let
, where
and
are the true mean and standard deviation, respectively, and
= 0.5, 1.0, 1.5, 2.0, 2.5 for each scenario.
4. Simulation results
Tables and show the empirical results of the Type I error rates for Student’s t-test (the number at the top of each set) and Johnson’s modified t-test ( are the numbers in the middle and bottom of each set, respectively). The procedure indicates a tendency for greater accuracy of Type I error rates when the sample size increases and skewness decreases. It is evident that the Type I error rates of Student’s t-test may differ at significant levels of 0.01 and 0.05.
Table 1. Comparison of type I error rates for student’s t-test and Johnson’s modified t-tests for upper-tailed rejection areas when is true at
Table 2. Comparison of type I error rates for Student’s t-test and Johnson’s modified t-tests for upper-tailed rejection areas when is true at
It should be noted that when the skewness coefficient is less than 2.00 and , the Type I error rates can be approximately doubled if
for testing
and
. Furthermore, they can be approximately 50% larger if
. However, the Type I error rate for
indicates a slight inflation at the significant level of
or
when skewness is not severe and the sample size is as small as 20. The inflation of the Type I error rate increases as the sample size increases.
Tables and show the comparison of the power of Student’s -test, Johnson’s modified
-test, and Johnson’s modified
-test using the
-critical point (
). In all the cases, as skewness and the value of
vary, the statistical power of Johnson’s modified
-test is higher than that of the Student’s
-test and Johnson’s modified
-test.
Table 3. Power comparison of student’s t-test and Johnson’s modified t-tests for upper-tailed rejection areas when and
is true at
Table 4. Power comparison of student’s t-test and Johnson’s modified t-tests for upper-tailed rejection areas when and
is true at
5. Demonstration using real data
The real data used here to illustrate the t-tests are from an experiment to determine the nitrogen binding capacity of laboratory mice (Dolkart, Halpern, & Perlman, Citation1971). The design was set by a control group of 20 normal mice and an experimental group of 19 diabetic mice. Both groups were treated with bovine serum albumin (BSA) for 28 days. The amount of BSA nitrogen bound was measured on the 29th day with micrograms per milliliter of undiluted mouse serum. The two group data were used to test whether the average amount of BSA nitrogen bound in the normal control group is better than that in the experimental group (known average binding capacity is 112.72). Both tests and
were used to test
against
. In a demonstration of laboratory mice data, we have
= 1.504 and kurtosis = 1.976 for the binding capacity of the experimental group. The goodness-of-fit test for the distribution fitting was used, and the result (p-value = 0.426) indicates that there is no significant evidence to reject the null hypothesis. This implies that the experimental group data are from the exponential distribution. The MLE of
for
is considered under the exponential distribution assumption. Then, the data were tested by each Johnson’s
-test, and
=2.56 and
=3.20 are obtained. The values of
and
should be compared with the critical value in Student’s t tables for 19 degrees of freedom at a significance level of 5% (i.e.,
). It was found that the data supported
rather than
, and thus it is concluded that the normal mice have a significantly higher binding capacity than the diabetic mice at the critical point
=1.729. The p-values of tests were also calculated: 0.006 and 0.001 corresponding respectively to
=2.56 and
=3.20. The p-value of
represents a more significant impact on the dataset than that of
.
6. Conclusion and future work
This study was concerned with the MLE of in Johnson’s modified t-test and the
-test of the means of positively skewed distributions. An empirical study indicated that the
-test is accurate in terms of the Type I error rate when the sample size is small and skewness is not severe. Moreover, the
-test is more powerful than the
-test and
-test given that the sampling distributions are known.
When skewed or known distributions are used, the parameters can be inferred by the MLE method more effectively than by moment estimators, as expected for known distributions. In this study, the distributions were selected with shape and scale parameters, and it was assumed that the shape parameters were known for simplicity in the setting of skewness in the simulations.
In practice, Johnson’s modified t-test is preferable when the distribution is unknown, except for its asymmetry. Therefore, the population third central moment (i.e., ) is estimated by the sample third central moment in the calculation of Johnson’s modified t-test (i.e., frequentist) rather than by distribution-based estimators (such as MLEs). However, one may calculate the skewness coefficient of the empirical data and test them for distribution-based fit before applying the
-test. It is suggested that both the
and
tests be performed and their results be compared for minimally skewed empirical data. Moreover, the
-statistic greatly depends on the shape of the parent distribution through the goodness of fit test. Furthermore, it involves the scale for the MLE of the parent distribution. To derive a robust and powerful test, future studies should examine another estimator for
of Johnson’s modified t-test with fewer restrictions.
Additional information
Funding
Notes on contributors
I-Shiang Tzeng
I-Shiang Tzeng is a biostatistician at Taipei Tzu Chi Hospital, Buddhist Tzu Chi Medical Foundation, New Taipei city, Taiwan. In the past years, he was a doctoral researcher in the National Translational Medicine and Clinical trial Resource Center (NTCRC) composed by Academia Sinica, National Taiwan University and National Yang-Ming University, Taiwan. He served as a bioinformatics and biostatistics consultant in NTCRC. He is also an adjunct assistant professor in the Department of Statistics, National Taipei University, Taiwan. His area of research includes biostatistics and epidemiologic method and further studies proposing the potential powerful method for age-period-cohort (APC) analysis. Futhermore, his research interests include the field of machine learning from biological issues to medical issues in all potential applications.
References
- Student. (1908). The probable error of a mean. Biometrika, 6, 1–13.
- Chen, L. (1995). Testing the mean of skewed distributions. Journal of the American Statistical Association, 90, 767–772.
- Cornish, E. A., & Fisher, R. A. (1937). Moments and cumulants in the specification of distributions. Review of the International Statistics Institute, 5, 307–327.
- Cressie, N. A. C., & Whitford, H. J. (1986). How to use the two sample t test? Biometrical Journal, 28, 131–148.
- Diaconis, P., & Efron, B. (1983). Computer-intensive methods in statistics. Scientific American, 248, 116–130.
- Dolkart, R. E., Halpern, B., & Perlman, J. (1971). Comparison of antibody responses in normal and alloxan diabetic mice. Diabetes, 20, 162–167.
- Hall, P. (1983). Inverting an edgeworth expansion. The Annals of Statistics, 11, 569–576.
- Johnson, N. J. (1978). Modified t tests and confidence intervals for asymmetrical populations. Journal of the American Statistical Association, 73, 536–544.
- Mood, A. M., Graybill, F. A., & Boes, D. C. (1974). Introduction to the theory of statistics. New York, NY: John Wiley.
- Neyman, J., & Pearson, E. S. (1928). On the use and interpretation of certain test criteria for purposes of statistical inference, part I. Biometrika, 20A, 175–240.
- Pearson, E. S. (1928). The distribution of frequency constants in small samples form symmetrical populations. Biometrika, 20A, 356–360.
- Sutton, C. D. (1993). Computer-intensive methods for tests about the mean of an asymmetrical distribution. Journal of the American Statistical Association, 88, 802–810.
- Welch, B. L. (1947). The generalization of “Student’s” problem when several different population variances are involved. Biometrika, 34, 28–35.
Appendix A
(i) Weibull ()
First, initial moments can be calculated using
Second,
Hence, MLE of ,
is
where is MLE of
(ii) Gamma ()
First, initial moments can be calculated using
Second,
Hence, MLE of ,
is
where
is MLE of
(iii) Exponential ()
First, initial moments can be calculated using
Second,
Hence, MLE of ,
is
where
is MLE of
Appendix B
Derivation of and
in
Let is defined as a random variable follows a sample distribution with mean
=
and standard deviation
=
for a large sample size
, where
is the standard deviation of population. First, we consider the Student’s t-test
where the sample standard deviation is used to estimate
. According to Cornish–Fisher expansion under the assumption of all moments of a population exists; then
where is defined as a random variable follows a standard normal distribution. Let
is defined as the population third central moment and
is the third central moment of
which equal to
; then
The Cornish–Fisher expansion of which ignoring higher-order terms is
Let
,
be a normal variable independent of
. Replacing the values of
and
by their respective expansions and rewriting
, where
is the correlation between
and
, the Cornish–Fisher expansion of
is
where and
is the fourth central moment of
Substitute to
, then
Select and
through constraints as follows
Substitute to constant term of
then
Hence, according to Johnson’s method to modify t by and
as
where and
related with
,
, and
.
And ,
, then
In our study, let the modified t variable of as follows:
Let ,
, then we represent the above statistic as follows:
Use MLE of ,
to estimate
,
, respectively. Then
We know MLE of is equal to
. And then
To demonstrate the use of the variable in testing of real data, we assume to test the hypothesis
against
. The reject criteria could be
where the critical value, is obtained from the Student’s
-distribution.