Abstract
A new approach toward cancer therapy is the use of cancer vaccine, yet the different molecular bases of cancers, reduce the effectiveness of this approach. In this article, we aim to use matrix metalloproteinase-9 protein (MMP9) which is an essential molecule in the survival and metastasis of all types of cancers as a target for universal cancer vaccine design. The reference sequence of MMP9 protein was obtained from NCBI databases. Furthermore, the B-cell and T cell-related peptides were analyzed using the IEDB website and other related soft wares. The best candidate peptides were then visualized using chimera software. Three peptides were found to be good candidates for interactions with B cells (SLPE, RLYT, and PALPR), while 10 peptides were found as good targets for interactions with MHC1 and another 10 peptides founded suitable for interactions with MHC2 with population coverages of 94.77 and 90.67%, respectively. Finally, the immune response simulation and molecular docking were done using the C-IMMSIM simulator and AutoDock Vina to confirm the effectiveness of the proposed vaccine. By the end of this project: twenty-three peptide-based vaccine was designed for use as a universal cancer vaccine which has a high world population coverage for MHC1 (94.77%) and MHC2 (90.67%) related alleles.
1. Introduction
Cancer is a chronic disease with varying degrees of manifestations characterized by abnormal cell division and high mortality and morbidity rates, in addition, it is considered the second leading cause of death worldwide with an estimated 8 million death annually and 18 million new cases per year [Citation1–5]. Although the cancer survival rate increased dramatically over the years in many countries, yet more work are needed to improve the prognosis of patients suffering from this debilitating disease [Citation6,Citation7]. Cancer types can either be common or rare, depending on the number of cases associated with it. Usually, any cancer with a number of cases below 6 in 100,000 annually is considered a rare type [Citation8]. All types of cancers cause common clinical symptoms including weight loss, fatigue, pain, nausea, vomiting, and constipation, etc. [Citation9]. Additional symptoms are specific to the site of malignancy [Citation10]. Breast cancer is the most common type of cancer in women worldwide [Citation11]. Lung cancer is the second most common cancer in both men and women while colorectal is the third most common type of cancer in both sexes [Citation12].
The classical management for cancer composed of chemotherapy, radiotherapy, and surgery. Recently Immunotherapy started to shows very promising results in the field of cancer management. Different types of immunotherapy are available including cytokine therapy, checkpoint inhibitors, monoclonal antibodies, and therapeutic vaccines [Citation13,Citation14]. With the latter being the center of much research in recent years, cumulating in the licensing of Sipuleucel-T By the U.S. Food and Drug Administration (FDA) as the first dendritic cell-based therapeutic vaccine for prostate cancer in 2010 [Citation15,Citation16]. The vaccine can either be used alone or in combination with other modalities like immune checkpoint inhibitors (ICIs) which shows better results when compared with vaccine monotherapy [Citation17].
Most drug company invests in the common types of cancer like breast and colorectal cancer for financial and cost-effective reasons leaving the rare type of cancer like chondrosarcoma with no effective mode of treatment [Citation18–20]. This calls for a new strategy for the management of cancer as a whole rather than treating different types individually. Furthermore, although many trials of therapeutic and preventive drugs to cancer have been carried out throughout the years, yet none of them succeeded to obtain the same response from all types of cancers, moreover, the FDA has not approved any drug as a universal cancer vaccine agent [Citation21,Citation22]. The reason for this failure is the fact that no effective intervention is available that covers all pathological pathways of the different types of cancer caused by the massive tumor heterogeneity [Citation23].
There are approximately 28 subtypes of matrix metalloproteinase (MMPs). They are responsible for maintaining the components of the extracellular matrix (ECM) and controlling metastasis of cancerous cells. Matrix metalloproteinases-9 (MMP9) gene (MIM 120361) is located at chromosome 20q12-13. It encodes MMP9 protein, a major enzyme for the degradation and absorption of ECM, signaling of wound healing, bone resorption, angiogenesis, and plays a crucial role in various types of cancer progression. Such as breast cancer, colorectal cancer, papillary thyroid cancer, urinary cancers, lymphomas, and gastric cancer [Citation24–27]. In addition, MMP-9 is considered as a biomarker for inflammatory diseases like Rheumatoid arthritis and asthmas [Citation28,Citation29].
MMP-9 is present in normal tissues, however, it is overexpression has been noticed in different malignant tumors like osteosarcoma (73.9%), colorectal carcinoma (69.1%), breast (78.75%), pancreatic cancers (55.17%), and other types of cancers [Citation30–35]. therefore using it as a vaccine target will ensure a universal immune response against most types of cancer. in addition, animal studies in immunized mice with MMP-9 derived peptides resulted in significantly smaller tumor burden and improved immune response against cancer cells with the tested antibodies suppressing migration of both murine and human cancer cell lines in vivo, which suggest a possible outcome in human studies [Citation36].
To the best of our knowledge, this is the first immunoinformatics study to use the human MMP9 protein as an immunogenic target for peptide-based vaccine design for all types of cancer. Peptide-based vaccines are safe, cheaper, and less laboring making them a cost-effective method for vaccination [Citation37,Citation38]. In addition, reverse vaccinology techniques have proven their efficiency in different fields as evidenced by many of the designed vaccines been tested in clinical trials [Citation39–41].
2. Materials and methods
2.1. Sequences retrieval
The whole protein sequence of the MM9 in FASTA format, accessed on November 3, 2019 (NP-004985.2) was retrieved from the NCBI database (https://www.ncbi.nlm.nih.gov/protein) [Citation42]. On the other hand, the candidate epitopes were analyzed by IEDB analysis tools (http://www.iedb.org/) [Citation43].
2.2. Identification of conserved regions
Sequences were aligned to identify the conserved regions using the BioEdit tool package version 7.2.5. Furthermore, epitope conservancy investigation for each epitope was predicted by the IEDB analysis resource. (http://tools.immuneepitope.org/tools/conservancy) [Citation44].
2.3 B-cell epitope prediction
B-cell epitope is the part of a vaccine that interacts with the B-cell receptor. The epitopes of interest were investigated using numerous B-cell prediction algorisms to identify the antigenicity, flexibility, hydrophilicity, and surface accessibility, as the selection of the highest immunogenic protein sequence is a prerequisite for epitope-based peptide vaccine design [Citation45].
2.3.1. Prediction of linear B-cell epitopes
BepiPred from the immune epitope database (http://toolsiedb.ofg/bcell/) was used for linear B-cell epitopes prediction [Citation46], with a default threshold value of 0.35. All epitopes with values less than this threshold were chosen.
2.3.2. Prediction of surface accessibility
By using the Emini surface accessibility prediction tool of the immune epitope database (IEDB) (http://tools.immuneepitope.org/tools/bcell/iedb) [Citation47], the surface accessible epitopes were predicted from the available peptides in the conserved region, with a default threshold value of 1.000. We then selected all peptides with values less than this threshold for further analysis.
2.3.3. Prediction of epitopes antigenicity
The Kolaskar and Tongaonkar antigenicity methods were used to determine the antigenic sites in MMP-9 protein. We used a default threshold value of 1.028. It is available at (http://tools.immuneepitope.org/bcell/) [Citation48], all antigenic peptide with values less than this threshold were selected for further analysis.
2.4 T-cell epitope prediction
2.4.1. Mhc class I binding predictions:
Peptide binding to MHC class I molecules was analyzed by the IEDB MHC I prediction tool (http://tools.iedb.org/mhc1.); based on Artificial Neural Network (ANN). This tool has different algorithms to show the ability of the submitted sequence to bind to a specific MHC class 1 molecule [Citation43,Citation49–51]. All epitope lengths were set as nine amino acids, all conserved epitopes that bind to alleles at score equal or less than 100 half-maximal inhibitory concentration (IC50) are selected for further analysis [Citation52].
2.4.2. Mhc class II binding predictions
Peptide binding to MHC class II molecules was analyzed by the IEDB MHC II prediction tool (http://tools.iedb.org/mhcII) [Citation53,Citation54], the three major types of HLA alleles (DR, DQ, and DP) were chosen for analysis based on NN-align, where all peptides with IC50 less than 500 were chosen for a further workout.
2.5. Population coverage
Calculating HLA-alleles distribution among the world population is critical for effective multi-epitope vaccine design; therefore, worldwide population coverage analysis of the above-mentioned MHC classes I and II epitopes was done by using the IEDB tool. (http://tools.iedb.org/tools/population/iedb_input) [Citation55].
2.6. Protein 3D structure visualization
The full 3D structure of MM9 was generated by RaptorX (http://raptorx.uchicago.edu/) [Citation56]. The accession number of the reference sequence is NP-004985.2, then UCSF Chimera version 1.14 was used to visualizing the positions of suggested peptides [Citation57]. The generated protein model was then verified using the Ramachandran plot from the (model panel) tool in chimera software.
2.7. Allergenicity test
AllerTOP v. 2.0 was used to check the Allergenicity of MMP9 protein. This method is based on auto cross-covariance (ACC) transformation of protein sequences into uniform equal-length vectors. It depends on five E descriptors of amino acids (amino acid hydrophobicity, molecular size, helix-forming propensity, the relative abundance of amino acids, and β-strand forming propensity). The proteins are classified depending on training sets containing different known and unknown allergens [Citation58]. It is available at https://www.ddg-pharmfac.net/AllerTOP/index.html.
2.8. Antigenicity test
VaxiJen v. 2.0 was used to check the antigenicity of the chosen peptides with a cut point of 0.5. It is an alignment-free approach for insilico antigen identification, which is based on the ACC method. It has a prediction accuracy ranging from 69 to 97% [Citation59]. The software is available at http://www.ddg-pharmfac.net/vaxijen/VaxiJen/VaxiJen.html.
Furthermore, ANTIGENpro from SCRATCH protein predictor server was used to validate these antigenicity results, which is a sequenced based predictive model to predict if certain proteins are protectives [Citation60]. http://scratch.proteomics.ics.uci.edu/.
2.9. Prediction of interferon-gamma inducing epitopes
IFNepitope was used to predict the MHC2 related peptides with a capacity to activate interferon-gamma cytokine productions. It is based on two models: Motif based and SVM based models [Citation61].
The software is available at http://crdd.osdd.net/raghava/ifnepitope/index.php.
2.10. Molecular docking
The MMP-9 protein sequence was visualized in UCSF Chimera. The proposed peptides were then selected and saved as PBD. Furthermore, we choose four peptides from the proposed MHC1 and MHC2 peptides targets, that have the highest population coverages and the largest number of HLA alleles combined to it.
2.10.1. Ligand preparation
The four chosen peptides were prepared using AutoDock Tools 1.5.6 as following, first, we extracted water molecules, then we added polar hydrogen molecules, and lastly, we add charges using the Kollman charge method [Citation62].
2.10.2. Target predation
The 3 D structure of HLA-A*02:01 (6TDR) was retrieved from protein databank (http://www. rcsb.org/ pdb/home/home.do). Afterword, its file was prepared using AutoDock tools 1.5.6.
2.10.3. Insilico docking
Molecular docking was performed using AutoDock Vina 4.0 and PyMOL soft wares, with the default Grid parameters [Citation63]. AutoDock Vina is based on Genetic Algorithm; that combines energy evaluation through grids of affinity potential to calculate the suitable binding position for a ligand on a given protein, It is more accurate when compared with the previous versions of Docking software like AutoDock 4 [Citation64]. The obtained results were retrieved as binding energy. We then used Chimera software to visualize the pose with the least binding energy.
2.11. Immune response simulation
C-IMMSIM model was used to stimulate the immune system response to the proposed vaccine.
We submitted the MMP9 reference sequence to the server and run the simulations with the following prefixed parameters: Random Seed: 12345, Simulation Volume: 10, and Simulation Steps: 100 [Citation65]. http://150.146.2.1/C-IMMSIM/index.php?page=1.
3. Results
3.1. Prediction of B-cell epitope
The metalloproteinase-9 protein (MMP-9) reference sequence was examined by using BepiPred linear epitope prediction, Kolaskar and Tongaonkar antigenicity and Emini surface accessibility methods in IEDB, to calculate the probability of specific regions in the protein which binds to B-cell receptor, being in the surface of the protein and being immunogenic, respectively.
In BepiPred linear epitope prediction, which was the first method used to determine the B-cell epitopes, the average score of MMP-9 peptides was 0.321, with a minimum score of −0.006 and a maximum score of 3.227. Basically, all values that equal to or greater than the default threshold 0.350 were potentially linear epitopes, as shown in and .
Figure 1. Bepipred linear epitope prediction, the yellow peaks above the red line (threshold) are proposed to be a part of B-cell epitopes and the green peaks are not a part.
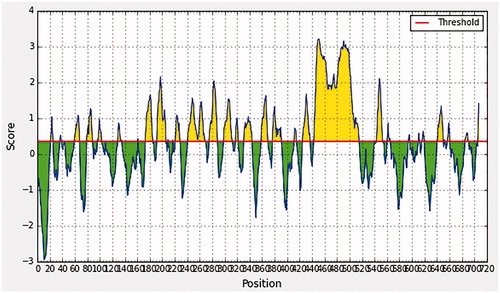
Table 1. Describe the predicted linear B-cell epitopes.
In Emini surface accessibility prediction, the average surface accessibility predictions of the protein were as 1.000, with a maximum of 6.034 and a minimum of 0.031, so all the values equal or greater than the default threshold 1.000 were potentially in the surface. Furthermore in Kolaskar and Tongaonkar antigenicity prediction’s the average of antigenicity was 1.028, with a maximum of 1.307 and a minimum of 0.869; all values equal to or greater than the default threshold 1.028 were potential antigenic determinants. The results of conserved predicted B-cell epitopes that passed both Emini & kolaskar tests are shown in and .
Figure 2. Emini surface accessibility, yellow peaks above the red line (threshold) are proposed to be a part B-cell epitopes while green peaks are not a part.
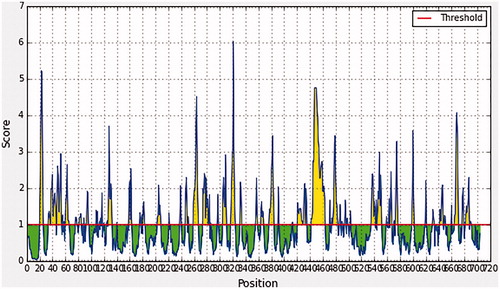
Figure 3. Kolaskar and Tongaonkar antigenicity prediction, yellow peaks above the red line (Threshold) are proposed to be a part of B-cell epitope while green peaks are not a part.
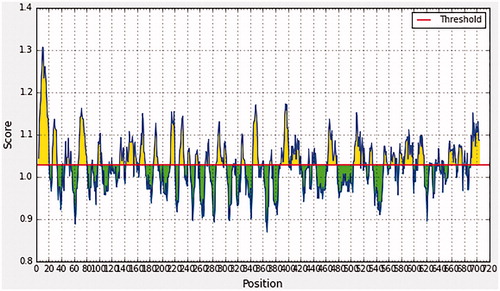
Table 2. List of conserved B-cell epitopes with their surface accessibility and antigenicity scores.
3.2. Prediction of T-cell epitope
3.2.1. MHC-I binding profile prediction for T cytotoxic cell conserved epitopes
227 epitopes were anticipated to interact with different MHC-1 alleles. The core epitopes (YRYGYTRVA/YGYTRVAEM) were noticed to be the dominant binders (highest population coverage and lowest IC-50) with seven alleles for each (HLA-C*06:02,HLA-C*07:01,HLA-C*12:03,HLA-C*14:02,HLA-B*27:05,HLA-C*07:02,HLA-B*39:01/HLA-C*03:03,HLA-C*14:02,HLA-C*12:03,HLA-C*06:02,HLA-B*08:01,HLA-C*15:02,HLA-C*07:01). Followed by (YLYRYGYTR, WRFDVKAQM, ALWSAVTPL, LLLQKQLSL, LIADKWPAL, KLFGFCPTR, MYPMYRFTE, FLIADKWPA) which binds with [Citation1,Citation3,Citation5,Citation8] alleles ().
Table 3. List of most promising epitopes that had a good binding affinity with MHC-I alleles in terms of IC50.
3.2.2. MHC-II binding profile prediction for T helper cell conserved epitopes
479 conserved predicted epitopes were found to interact with MHC-II alleles. The core epitopes (KMLLFSGRRLWRFDV) is thought to be the top binder as it interacts with 18 alleles; (HLA-DRB1*01:01,HLA-DRB1*03:01,HLA-DRB1*04:02,HLA-DRB1*07:01,HLA-DRB1*04:04,HLA-DRB1*08:01,HLA-DRB1*09:01,HLA-DRB1*13:01,HLA-DRB1*11:01,HLA-DRB1*13:02,HLA-DRB1*12:01,HLA-DRB1*15:01,HLA-DRB1*16:02,HLA-DRB3*01:01,HLA-DRB4*01:03,HLA-DRB5*01:01,HLA-DRB3*03:01,HLA-DRB4*01:01). Followed by (GRGKMLLFSGRRLWR, RGKMLLFSGRRLWRF, GKMLLFSGRRLWRFD, TFTRVYSRDADIVIQ, AVIDDAFARAFALWS, FARAFALWSAVTPLT, MLLFSGRRLWRFDVK, GNQLYLFKDGKYWRF, NQLYLFKDGKYWRFS) which binds to [Citation14,Citation15,Citation17,Citation18] alleles ().
Table 4. List of the most promising core sequence epitopes that had a strong binding affinity with MHC-II in terms of IC50.
3.2.3. Population coverage analysis
Population coverage test was performed, to detect the world Coverage of the epitopes that bind to MHC1 and MHC11 alleles, which founded to exhibit exceptional coverage with percentages of 94.77 and 90.67%, respectively.
Population coverage for MHC1: The interactions between the following 10 peptides with the most frequent MHC class I alleles (YRYGYTRVA, YGYTRVAEM, YLYRYGYTR, WRFDVKAQM, ALWSAVTPL, LLLQKQLSL, LIADKWPAL, KLFGFCPTR, MYPMYRFTE, and FLIADKWPA), represent a considerable coverage against the whole world population. The maximum population coverage percentage over these epitopes worldwide was found to be 62.74% for YRYGYTRVA (; ).
Figure 4. Illustrates the global coverage for the top 10 MHC-I peptides (YRYGYTRVA, YGYTRVAEM, YLYRYGYTR, WRFDVKAQM, ALWSAVTPL, LLLQKQLSL, LIADKWPAL, KLFGFCPTR, MYPMYRFTE, and FLIADKWPA). Note: In the graph, the line (-o-) represents the cumulative percentage of population coverage of the epitopes; the bars represent the population coverage for each epitope.
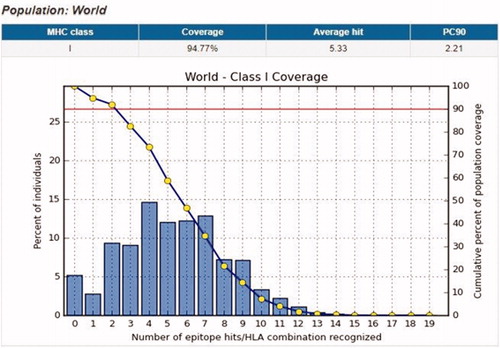
Table 5. Global population coverage of promising epitopes binding to MHC class I alleles.
Population coverage for MHC11: In the case of MHC class II, 10 epitopes were found to interact with the most frequent MHC class II alleles (KMLLFSGRRLWRFDV, GRGKMLLFSGRRLWR, RGKMLLFSGRRLWRF, GKMLLFSGRRLWRFD, TFTRVYSRDADIVIQ, AVIDDAFARAFALWS, FARAFALWSAVTPLT, MLLFSGRRLWRFDVK, GNQLYLFKDGKYWRF, NQLYLFKDGKYWRFS), inferring a massive global coverage. The highest population coverage percentage of these epitopes worldwide was that of KMLLFSGRRLWRFDV with a percentage of 83.74% (; ).
Figure 5. Illustrates the global proportion for the top 10 MHC-II epitopes (KMLLFSGRRLWRFDV, GRGKMLLFSGRRLWR, RGKMLLFSGRRLWRF, GKMLLFSGRRLWRFD, TFTRVYSRDADIVIQ, AVIDDAFARAFALWS, FARAFALWSAVTPLT, MLLFSGRRLWRFDVK, GNQLYLFKDGKYWRF, NQLYLFKDGKYWRFS). Notes: In the graph, the line (-o-) represents the cumulative percentage of population coverage of the epitopes; the bars represent the population coverage for each epitope.
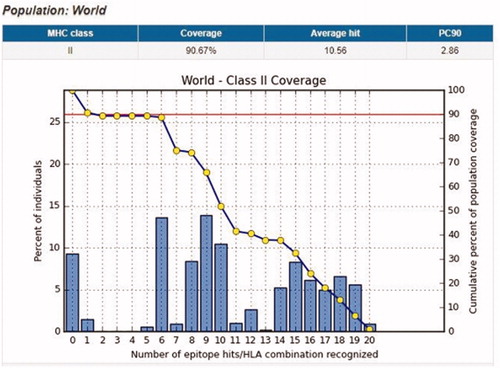
Table 6. Global population coverage of promising epitopes in isolated MHC class II.
3.2.4. Antigenicity analysis
Antigenicity analysis of the 10 chosen peptides of MHC1 and MHC2 by VaxiJen software shows four peptides to be probably antigenic in MHC1 (YLYRYGYTR, WRFDVKAQM, LIADKWPAL, and MYPMYRFTE) while only one peptide was found to be antigenic in MHC2 (RRLWRFDVKAQMVDP) (). Furthermore, ANTIGEN pro predicts MMP-9 protein probability of antigenicity to be 0.827661.
Table 7. List of antigenic peptides inMHC1 and MHC2 as predicted by VaxiJen software.
3.2.5. Prediction of interferon-gamma inducing epitopes:
IFNepitope server showed three peptides in MHC2 with an ability to induce interferon-gamma production (GPFLIADKWPALPRK, GRGKMLLFSGRRLWR, and FARAFALWSAVTPLT) ().
Table 8. List of MHC2 related peptides that can induce interferon-gamma production.
3.2.6. Structure modeling and validation by chimera
The MMP-9 protein structure was predicted by RaptorX and then analyzed by chimera software, moreover, the chosen peptides related to B cell, MHC1, and MHC2 peptides were then visualized () Furthermore, the modeling was verified using the Ramachandran plot analysis tool in chimera software, which shows that most of the amino acid residues are located in the allowed region ().
Figure 6. Present 3D structure of metalloproteinase-9 protein visualizing the top 10 T-cell peptides binding to MHC class I using chimera (version 1.14), yellow color indicate the chosen peptides while the purple color indicates the rest of the molecules.
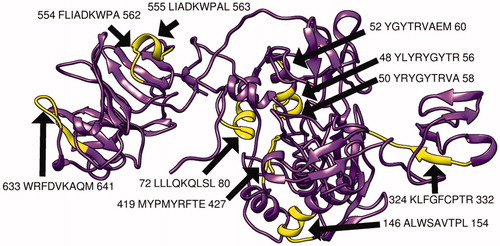
Figure 7. Present 3D structure of metalloproteinase-9 protein visualizing top 10 T-cell peptides binding to MHC class II, using chimera (version 1.14), yellow color indicate the chosen peptides while the purple color indicates the rest of the molecules.
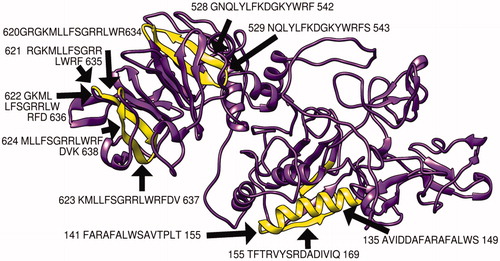
Figure 8. Present 3D structure of metalloproteinase-9 protein visualizing the most promising B-cell peptides that have high surface accessibility and antigenicity scores, using chimera (version 1.14), yellow color indicate the chosen peptides while the purple color indicates the rest of the molecules.
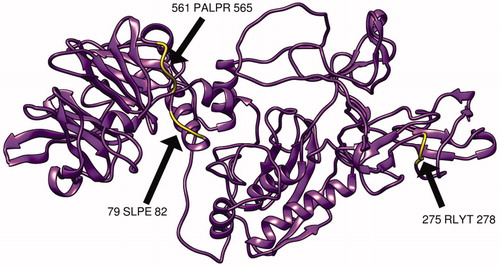
Figure 9. Ramachandran plot analysis of MMP9 protein showing most of the torsion angles located at allowed region (the blue dots represent torsion angles; the green lines indicate the allowed region. (Phi) ϕ And (psi) ψ are torsion angles. The torsion angle about the N–C bond is called ϕ and that about the C–C bond is ψ. This analysis predicted by chimera version 1.14.
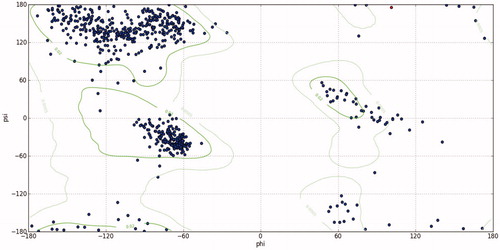
3.2.7. Conservation analysis
BioEdit analysis showed most of the amino acids in MMP-9 proteins to be conserved, except in three positions (110, 279, and 574) where the original wild amino acids in these position changes to Leucine, Arginine, and Proline, respectively ().
3.2.8. Characterization of the immune response of the vaccine
To investigate the immune responses created by the final chimeric vaccine construct, the immune simulator C-IMMSIM Online server-generated such simulations, which resemble the real responses produced by the immune system. These responses suggest a high spike in the initiation of secondary immune responses. Relatively, high levels of IgM were recorded in the primary response in the first days of vaccination, preceded by the surge in B-cell populations. Furthermore, the IgG1 + IgG2, IgG + IgM antibodies along with IgM were found to be increased along with the formation of T and B memory cells (). As the memory further intensifies, a projected response was reported in the cytotoxic and helper cell populations ()). Furthermore, cytokines like IL-6, IL-12, and interferon-g are reported to increase in the first days after the introduction of the vaccine to reach a level of 250,000 ng/ml by the end of the second week. Moreover, IL-2 was noticed to peak after 7 days of vaccine administration ().
Figure 11. Immune response to MMP-9 as predicted by the C-IMMSIM Online server. The plot shows the simulated levels of memory cells, B cell, and T cells after the introduction of MMP-9 protein to the human body. Act = active, Intern = internalized the Ag, Pres II = presenting on MHC II, Dup = in the mitotic cycle, Anergic = anergic, Resting = not active.
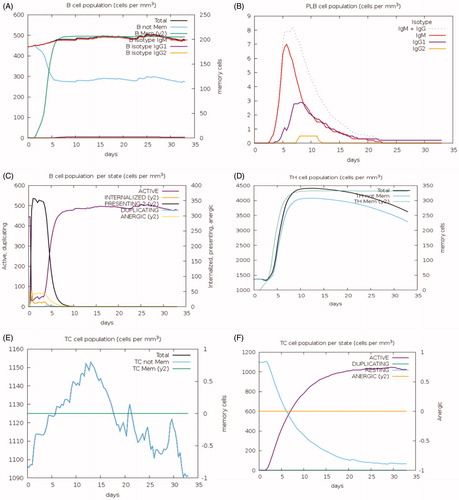
3.2.9. Docking
Four peptides were chosen for docking with HLA-A2A01 the result was: nine possible positions for each peptide with MHC1 and MHC2 proteins, we then selected the positions with least energy as our final model. The results of the least energy exposure for MHC1 peptides were as following: ALWSAVTPL: −9.7, LLLQKQLSL: −8.6. Furthermore, the results of the least energy exposure for MHC2 peptides were as following: KMLLFSGRRLWRFDV: −8.8, GRGKMLLFSGRRLWR: −7.8. The following are 3D representations of the docking of four peptides with MHC1 and MHC2 proteins ().
Figure 13. First peptide ALWSAVTPL docking with HLA-A*0201, showing the position with the least energy exposure (−9.7). Blue and cyan colors indicate MHC protein, while white and red color represents the binding peptide. The docking was done using AutoDock Vina and visualized using Chimera version.1.14.
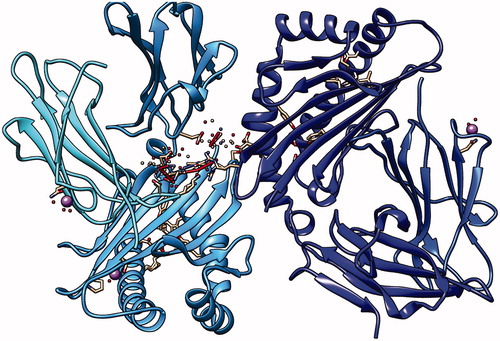
Figure 14. Second peptide LLLQKQLSL docking with HLA-A*0201, showing the position with the least energy exposure (−8.6). Blue and cyan colors indicate MHC protein, while white and red color represents the binding peptide. The docking was done using AutoDock Vina and visualized using Chimera version.1.14.
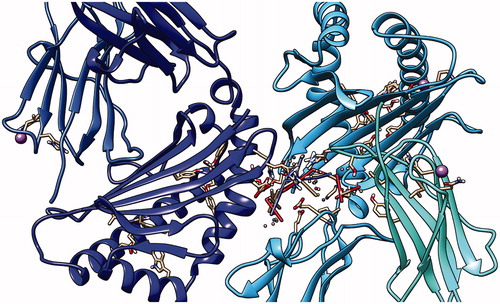
Figure 15. Third peptide KMLLFSGRRLWRFDV docking with HLA-A*0201, showing the position with the least energy exposure (−8.8). Blue color indicates MHC protein, while white and red color represents the binding peptide. The docking was done using AutoDock Vina and visualized using Chimera version 1.14.
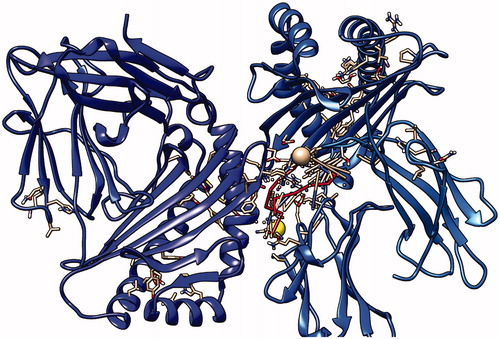
Figure 16. Fourth peptide GRGKMLLFSGRRLWR docking with HLA-A*0201, showing the position with the least energy exposure (−7.8). Blue color indicates MHC protein, while white and red color represents the binding peptide. The docking was done using AutoDock Vina and visualized using Chimera version.1.14.
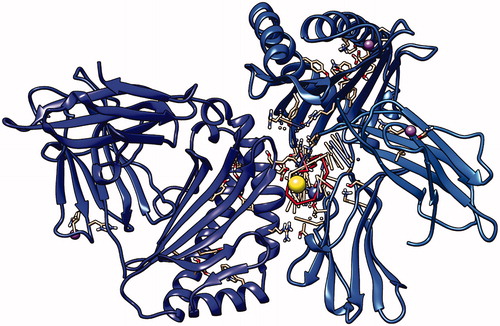
4. Discussion
Therapeutic and preventive multi Epitope peptides vaccines are the next generation of cancer immunotherapy. Production and testing of vaccines are expensive and time-consuming procedures, hence the development of immunoinformatic studies to tackle these challenges associated with the classical production of vaccines [Citation66,Citation67]. Many researchers have tried to design universal cancer vaccines using different approaches, yet until now, the FDA has not approved any of these drugs [Citation68]. The current work used immunogenic protein (matrix metalloproteinase-9) that is essential for the survival of all type of cancer as a target for vaccine design, where three Peptides found to be a good candidate for interactions with B cells, in addition, 10 peptides found as a good target for interactions both MHC1 and MHC2. The different soft wares used for the analysis are summarized in .
Most cancer vaccines depend on T cell interactions but recent research indicates the significant role B cell plays in the immunology of cancer, B cell produces antibodies and help in the regulation of other immune reaction [Citation69]. Furthermore, several B cell-based cancer vaccine studies have shown Promising results in chronic lymphocytic leukemia and other types of tumors [Citation70]. In this article, Linear B cell epitopes of matrix metalloproteinase-9 were analyzed by the BepiPred linear epitope prediction tool. After checking for conservation using BioEdit software (). These epitopes were further checked for surface accessibility and antigenicity by using the Emini surface accessibility prediction method and Kolaskar and Tongaonkar antigenicity method respectively () The following peptides have the highest score and were approved as the most promising B-cell epitopes (SLPE, RLYT, and PALPR) ( and ).
T-cell receptor can only identify epitopes if it is presented on major histocompatibility complex (MHC), Epitopes bind to MHC through the binding groove of MHC class I and class II by interacting with the amino acids residues located in the pockets on the floor of MHC [Citation71,Citation72].
Cytotoxic T cell (CD8) can only recognize antigen when it is presented on MHC1, and so we analyzed the peptides that have the highest population coverage and the best binding MHC1 and found the following 10 candidates (YRYGYTRVA, YGYTRVAEM, YLYRYGYTR, WRFDVKAQM, ALWSAVTPL, LLLQKQLSL, LIADKWPAL, KLFGFCPTR, MYPMYRFTE, FLIADKWPA) (; ). It is interesting to note that among the associated alleles related to these peptides, we found the most frequently reported allele in Caucasians which is HLA-A*0201 [Citation73]. This further proves the significant coverage of these peptides.
In the case of T-helper cells, it can only recognize antigen when it is presented in MHC2. Therefore, we predicted the peptides of MMP9 that can firmly bind with MHC2, which also hold the highest population coverage and end up with the following 10 peptides sequence (KMLLFSGRRLWRFDV, GRGKMLLFSGRRLWR, RGKMLLFSGRRLWRF, GKMLLFSGRRLWRFD, TFTRVYSRDADIVIQ, AVIDDAFARAFALWS, FARAFALWSAVTPLT, MLLFSGRRLWRFDVK, GNQLYLFKDGKYWRF, and NQLYLFKDGKYWRFS) (; ).
The population coverage of the epitopes and their respective HLA alleles are crucial, as HLA alleles are highly polymorphic in nature [Citation74]. We aim for this vaccine to benefit patients from different parts of the world, therefore, we analyzed the whole word population coverage for our predicted peptides, where we found MHC1 and MHC2 related peptides to have population coverage of 94.77 and 90.67%, respectively (). Therefore, our vaccine is assumed to elicit a strong comprehensive immune response against most types of cancer in the majority of the vaccinated population.
All peptides were visualized using Chimera software () which shows the predicted structures and positions of these peptides in MMP9 protein (yellow color), then Ramachandran plot analysis shows most of the amino acids residue located in the allowed regions which validate the accuracy of the generated structure () [Citation75].
Allergenicity is an important factor in vaccine design as the allergic reaction could be fatal to the patients and hence we tested the allergenicity of our proposed vaccine using AllerTOP software which confirms the nonallergenic of the vaccine [Citation76]. Furthermore, the antigenicity is a significant factor to put into account when choosing a target for vaccine design [Citation77], therefore we tested the antigenicity of mhc1 and mhc2 peptides using VaxiJen software which shows 4 peptides to be antigenic in mhc1 (YLYRYGYTR, WRFDVKAQM, LIADKWPAL, MYPMYRFTE) and one peptide to be antigenic in mhc2 (RRLWRFDVKAQMVD) (). In addition, the antigenicity of the whole protein was checked using ANTIGENpro which is more accurate and specific than VaxiJen and the resulted probability (0.827661) indicated the high antigenicity of the protein [Citation60,Citation78].
Interferon gamma is a cytokine that plays an essential role in innate and adaptive immunity. It is produced from T cell upon activation by specific peptides which lead to an improvement in cancer elimination by inducing ischemia and improving recognition of the tumor cells by CD4 and CD8 cells [Citation79]. we tested the ability of our chosen peptide to induce secretion of interferon-gamma through IFNepitope software which indicates that there are 3 peptides in MHC 2 to positively affect the interferon-gamma production (GPFLIADKWPALPRK, GRGKMLLFSGRRLWR, FARAFALWSAVTPLT) ().
Molecular docking and validation were done using AutoDock Vina after protein and ligand preparation using AutoDock tools. The docking shows the best combination possible between the peptides and the MHC protein. Here we choose the best four peptides for docking (ALWSAVTPL: −9.7, LLLQKQLSL: −8.6, KMLLFSGRRLWRFDV: −8.8, and GRGKMLLFSGRRLWR: −7.8.) and we showed the best model with least energy exposure using Chimera. This will help in future studies regarding the design of the MMP-9 vaccine ().
Immune response simulation was done using the C-IMMSIM server which is an agent-based simulator of the immune response against viral and drugs. After submitting the reference sequence, we have the result of both cellular and humoral response, which shows an increase in IgM antibodies after less than 5 days since the beginning of vaccination followed by fast drop then a rise in IgG antibodies after it, which is a known immune response phenomenon in vaccination [Citation80] ().
Interesting findings were the rise in memory cells for more than a month since the beginning of infection suggesting a possibility of developing a lasting immunity after using this vaccine ().
The associated cytokine changes prove the vast immunogenic reactions induced by the vaccine especially the rise in IL2, in 7 days after the vaccine induction, which plays a significant role in enhancing the immune response against the cancer antigens through potentiating the role of natural killer cells NKL, B cell, and T cell, therefore inducing regression in cancer patients () [Citation81,Citation82].
In a study done by Roomi et al. where they used MMP9 as an anticancer vaccine in mice, they found an 80% reduction in tumor volume after using this vaccine [Citation83]. Which further proves the predicted effectiveness of our proposed vaccine. We took further steps in our work to analyze this target using the human database.
Previous research by Dosset et al. designed a universal peptide-based cancer vaccine using telomerase reverse transcriptase (TERT) which showed improve response only against CD4 and CD8. With no testing for the possible response from B cells which was covered in our current research [Citation84].
This study is limited due to the few numbers of reference sequence we were able to acquire from NCBI, along with the six alleles (HL-DRB3*03:01, HL-DRB3*02:01, HL-DRB4*01:03, HL-DRB3*01:01, HL-DRB4*01:01, HL-DRB5*01:01) that was not applicable for prediction in IEDB. In addition to the limited reported therapeutic activity of peptide vaccines in general [Citation85].
In the end, 23 peptides were chosen as a target for universal cancer vaccine design, yet more clinical studies are required to confirm and validate these results, in order to move another step towered the goal of eradicating cancer.
5. Conclusion
The current work designs multi epitopes peptide-based universal cancer vaccine using MMP9 protein as a target where three Peptides were found to be good candidates for interactions with B cells, and 10 peptides were found as a good target for interactions with each MHC1 and MHC2.
Acknowledgment
The authors acknowledge the Deanship of Scientific Research at the University of Bahri for supportive cooperation.
Disclosure statement
The authors declare that no conflict of interest exists in this work.
References
- Siegel RL, Miller KD, Jemal A. Cancer statistics, 2019. CA Cancer J Clin. 2019;69(1):7–34.
- Nagai H, Kim YH. Cancer prevention from the perspective of global cancer burden patterns. J Thorac Dis. 2017;9(3):448–451.
- Doytchinova IA, Flower DR. In silico prediction of cancer immunogens: current state of the art. BMC Immunol. 2018;19(1):11.
- Siegel R, Ma J, Zou Z, et al. Cancer statistics, 2014. CA Cancer J Clin. 2014;64(1):9–29.
- Bray F, Ferlay J, Soerjomataram I, et al. Global cancer statistics 2018: GLOBOCAN estimates of incidence and mortality worldwide for 36 cancers in 185 countries. CA Cancer J Clin. 2018;68(6):394–424.
- Miller KD, Nogueira L, Mariotto AB, et al. Cancer treatment and survivorship statistics, 2019. CA A Cancer J Clin. 2019;69(5):363–385.
- Allemani C, Matsuda T, Di Carlo V, et al. Global surveillance of trends in cancer survival 2000-14 (CONCORD-3): analysis of individual records for 37 513 025 patients diagnosed with one of 18 cancers from 322 population-based registries in 71 countries. Lancet (London, England). 2018;391(10125):1023–1075.
- Pillai RK, Jayasree K. Rare cancers: challenges & issues. Indian J Med Res. 2017;145(1):17–27.
- Miaskowski C, Lee KA. Pain, fatigue, and sleep disturbances in oncology outpatients receiving radiation therapy for bone metastasis: a pilot study. J Pain Symptom Manag. 1999;17(5):320–332.
- Scheel BI, Holtedahl K. Symptoms, signs, and tests: The general practitioner's comprehensive approach towards a cancer diagnosis. Scand J Prim Health Care. 2015;33(3):170–177.
- Momenimovahed Z, Salehiniya H. Epidemiological characteristics of and risk factors for breast cancer in the world. Breast Cancer (Dove Med Press)). 2019;11:151–164.
- Siegel RL, Miller KD, Jemal A. Cancer statistics, 2020. CA A Cancer J Clin. 2020;70(1):7–30.
- Kamta J, Chaar M, Ande A, et al. Advancing cancer therapy with present and emerging immuno-oncology approaches. Front Oncol. 2017;7:64.
- Falzone L, Salomone S, Libra M. Evolution of cancer pharmacological treatments at the turn of the third millennium. Front Pharmacol. 2018;9:1300.
- Cheever MA, Higano CS. PROVENGE (Sipuleucel-T) in prostate cancer: the first FDA-approved therapeutic cancer vaccine. Clin Cancer Res. 2011;17(11):3520–3526.
- Ahmed MS, Bae Y-S. Dendritic cell-based therapeutic cancer vaccines: past, present and future. Clin Exp Vaccine Res. 2014;3(2):113–116.
- Zhao J, Chen Y, Ding Z-Y, et al. Safety and efficacy of therapeutic cancer vaccines alone or in combination with immune checkpoint inhibitors in cancer treatment. Front Pharmacol. 2019;10:1184.
- Gradishar WJ, Anderson BO, Balassanian R, et al. Breast cancer, version 4.2017, NCCN clinical practice guidelines in oncology. J Natl Compr Canc Netw. 2018;16(3):310–320.
- Siegel RL, Miller KD, Jemal A. Colorectal cancer mortality rates in adults aged 20 to 54 years in the United States, 1970-2014. Jama. 2017;318(6):572–574.
- Biermann JS, Chow W, Reed DR, et al. NCCN guidelines insights: bone cancer, version 2.2017. J Natl Compr Canc Netw. 2017;15(2):155–167.
- Maeda H, Khatami M. Analyses of repeated failures in cancer therapy for solid tumors: poor tumor-selective drug delivery, low therapeutic efficacy and unsustainable costs. Clin Transl Med. 2018;7(1):11.
- Dalgleish AG, Stern PL. The failure of radical treatments to cure cancer: can less deliver more? Ther Adv Vaccines Immunother. 2018;6(5-6):69–76.
- Aurisicchio L, Pallocca M, Ciliberto G, et al. The perfect personalized cancer therapy: cancer vaccines against neoantigens. J Exp Clin Cancer Res. 2018;37(1):86.
- Felizi RT, Veiga MG, Carelli Filho I, et al. Association between matrix metallopeptidase 9 polymorphism and breast cancer risk. Rev Bras Ginecol Obstet. 2018;40(10):620–624.
- Wang R-X, Chen S, Huang L, et al. Predictive and prognostic value of Matrix metalloproteinase (MMP) - 9 in neoadjuvant chemotherapy for triple-negative breast cancer patients. BMC Cancer. 2018;18(1):909.
- Schlom J, Arlen PM, Gulley JL. Cancer vaccines: moving beyond current paradigms. Clin Cancer Res. 2007;13(13):3776–3782.
- Zarkesh M, Zadeh-Vakili A, Akbarzadeh M, et al. The role of matrix metalloproteinase-9 as a prognostic biomarker in papillary thyroid cancer. BMC Cancer. 2018;18(1):1199.
- Maguire EM, Pearce SWA, Xiao R, et al. Matrix metalloproteinase in abdominal aortic aneurysm and aortic dissection. Pharmaceuticals (Basel). 2019;12(3):118.
- Tokito A, Jougasaki M. Matrix metalloproteinases in non-neoplastic disorders. IJMS. 2016;17(7):1178.
- Huang H. Matrix metalloproteinase-9 (MMP-9) as a cancer biomarker and MMP-9 biosensors: recent advances. Sensors. 2018;18(10):3249.
- Baroncini LA, Nakao LS, Ramos SG, et al. Assessment of MMP-9, TIMP-1, and COX-2 in normal tissue and in advanced symptomatic and asymptomatic carotid plaques. Thromb J. 2011;9(1):6.
- Liu Y, Wang Y, Teng Z, et al. Matrix metalloproteinase 9 expression and survival of patients with osteosarcoma: a meta-analysis. Eur J Cancer Care. 2017. DOI:10.1111/ecc.12364
- Li H, Qiu Z, Li F, et al. The relationship between MMP-2 and MMP-9 expression levels with breast cancer incidence and prognosis. Oncol Lett. 2017;14(5):5865–5870.
- Yang B, Tang F, Zhang B, et al. Matrix metalloproteinase-9 overexpression is closely related to poor prognosis in patients with colon cancer. World J Surg Onc. 2014;12(1):24.
- Jakubowska K, Pryczynicz A, Januszewska J, et al. Expressions of matrix metalloproteinases 2, 7, and 9 in carcinogenesis of pancreatic ductal adenocarcinoma. Dis Markers. 2016;2016:9895721.
- Waheed Roomi M, Efremov E, Niedzwiecki A, et al. Matrix metalloproteinases-9 as a promising target for anti-cancer vaccine: inhibition of melanoma tumor growth in mice immunized with syngeneic MMP-9 peptides. World Cancer Res J. 2019;6:e1421.
- Li W, Joshi M, Singhania S, et al. Peptide vaccine: progress and challenges. Vaccines (Basel). 2014;2(3):515–536.
- Rahat MA. Targeting angiogenesis with peptide vaccines. Front Immunol. 2019;10:1924.
- Okuno K, Sugiura F, Hida J-i, et al. Phase I clinical trial of a novel peptide vaccine in combination with UFT/LV for metastatic colorectal cancer. Exp Ther Med. 2011;2(1):73–79.
- Obara W, Kanehira M, Katagiri T, et al. Present status and future perspective of peptide-based vaccine therapy for urological cancer. Cancer Sci. 2018;109(3):550–559.
- Sawada Y, Yoshikawa T, Nobuoka D, et al. Phase I trial of a glypican-3-derived peptide vaccine for advanced hepatocellular carcinoma: immunologic evidence and potential for improving overall survival. Clin Cancer Res. 2012;18(13):3686–3696.
- O'leary NA, Wright MW, Brister JR, et al. Reference sequence (RefSeq) database at NCBI: current status, taxonomic expansion, and functional annotation. Nucleic Acids Res. 2016;44(D1):D733–D745.
- Kim Y, Ponomarenko J, Zhu Z, et al. Immune epitope database analysis resource. Nucleic Acids Res. 2012;40:W525–30.
- Bui HH, Sidney J, Li W, et al. Development of an epitope conservancy analysis tool to facilitate the design of epitope-based diagnostics and vaccines. BMC Bioinformatics. 2007;8:361.
- Larsen JE, Lund O, Nielsen M. Improved method for predicting linear B-cell epitopes. Immunome Res. 2006;2:2.
- Jespersen MC, Peters B, Nielsen M, et al. BepiPred-2.0: improving sequence-based B-cell epitope prediction using conformational epitopes. Nucleic Acids Res. 2017;45(W1):W24–W29.
- Emini EA, Hughes JV, Perlow DS, et al. Induction of hepatitis A virus-neutralizing antibody by a virus-specific synthetic peptide. J Virol. 1985;55(3):836–839.
- Kolaskar AS, Tongaonkar PC. A semi-empirical method for prediction of antigenic determinants on protein antigens. FEBS Letters. 1990;276(1–2):172–174.
- Kolaskar AS, Tongaonkar PC. A semi-empirical method for prediction of antigenic determinants on protein antigens. FEBS Lett. 1990;276(1–2):172–174.
- Nielsen M, Lundegaard C, Worning P, et al. Reliable prediction of T-cell epitopes using neural networks with novel sequence representations. Protein Sci. 2003;12(5):1007–1017.
- Peters B, Sette A. Generating quantitative models describing the sequence specificity of biological processes with the stabilized matrix method. BMC Bioinformatics. 2005;6:132.
- Sidney J, Assarsson E, Moore C, et al. Quantitative peptide binding motifs for 19 human and mouse MHC class I molecules derived using positional scanning combinatorial peptide libraries. Immunome Res. 2008;4:2.
- Wang P, Sidney J, Dow C, et al. A systematic assessment of MHC class II peptide binding predictions and evaluation of a consensus approach. PLoS Comput Biol. 2008;4(4):e1000048.
- Wang P, Sidney J, Kim Y, et al. Peptide binding predictions for HLA DR, DP and DQ molecules. BMC Bioinformatics. 2010;11:568.
- Bui HH, Sidney J, Dinh K, et al. Predicting population coverage of T-cell epitope-based diagnostics and vaccines. BMC Bioinformatics. 2006;7:153.
- Wang S, Li W, Liu S, et al. RaptorX-Property: a web server for protein structure property prediction. Nucleic Acids Res. 2016;44(W1):W430–W435.
- Pettersen EF, Goddard TD, Huang CC, Couch GS, et al. UCSF Chimera-a visualization system for exploratory research and analysis. J Comput Chem. 2004;25(13):1605–1612.
- Dimitrov I, Bangov I, Flower DR, et al. AllerTOP v.2-a server for in silico prediction of allergens. J Mol Model. 2014;20(6):2278.
- Doytchinova IA, Flower DR. VaxiJen: a server for prediction of protective antigens, tumour antigens and subunit vaccines. BMC Bioinf. 2007;8(1):4.
- Magnan CN, Zeller M, Kayala MA, et al. High-throughput prediction of protein antigenicity using protein microarray data. Bioinform. (Oxford, England). 2010;26(23):2936–2943.
- Dhanda SK, Vir P, Raghava GP. Designing of interferon-gamma inducing MHC class-II binders. Biol Direct. 2013;8:30.
- Morris GM, Huey R, Lindstrom W, et al. AutoDock4 and AutoDockTools4: automated docking with selective receptor flexibility. J Comput Chem. 2009;30(16):2785–2791.
- DeLano WL. Pymol: an open-source molecular graphics tool. CCP4 Newslett Protein Crystallogr. 2002;40:82–92.
- Trott O, Olson AJ. AutoDock Vina: improving the speed and accuracy of docking with a new scoring function, efficient optimization, and multithreading. J Comput Chem. 2010;31(2):455–461.
- Rapin N, Lund O, Bernaschi M, et al. Computational immunology meets bioinformatics: the use of prediction tools for molecular binding in the simulation of the immune system. Plos One. 2010;5(4):e9862.
- Khan M, Khan S, Ali A, et al. Immunoinformatics approaches to explore Helicobacter pylori proteome (virulence factors) to design B and T cell multi-epitope subunit vaccine. Sci Rep. 2019;9(1):13321.
- Abdelmageed MI, Abdelmoneim AH, Mustafa MI, et al. Design of a multiepitope-based peptide vaccine against the E protein of human COVID-19: an immunoinformatics approach. Biomed Res Int. 2020;2020:2683286.
- Lokhov PG, Balashova EE. Design of universal cancer vaccines using natural tumor vessel-specific antigens (SANTAVAC). Hum Vaccin Immunother. 2015;11(3):689–698.
- Wennhold K, Shimabukuro-Vornhagen A, von Bergwelt-Baildon M. B cell-based cancer immunotherapy. Transfus Med Hemother. 2019;46(1):36–46.
- Biagi E, Rousseau R, Yvon E, et al. Responses to human CD40 ligand/human interleukin-2 autologous cell vaccine in patients with B-cell chronic lymphocytic leukemia. Clin Cancer Res. 2005;11(19 Pt 1):6916–6923.
- Zvyagin IV, Tsvetkov VO, Chudakov DM, et al. An overview of immunoinformatics approaches and databases linking T cell receptor repertoires to their antigen specificity. Immunogenetics. 2020;72(1-2):77–84.
- Kennedy PR, Barthen C, Williamson DJ, et al. HLA-B and HLA-C differ in their nanoscale organization at cell surfaces. Front Immunol. 2019;10:61.
- Pelte C, Cherepnev G, Wang Y, et al. Random screening of proteins for HLA-A*0201-binding nine-amino acid peptides is not sufficient for identifying CD8 T cell epitopes recognized in the context of HLA-A*0201. J Immunol. 2004;172(11):6783–6789.
- Glanville KP, Coleman JRI, Hanscombe KB, et al. Classical human leukocyte antigen alleles and C4 haplotypes are not significantly associated with depression. Biol Psychiatry. 2020;87(5):419–430.
- Hollingsworth SA, Karplus PA. A fresh look at the Ramachandran plot and the occurrence of standard structures in proteins. Biomol Concepts. 2010;1(3–4):271–283.
- Chung EH. Vaccine allergies. Clin Exp Vaccine Res. 2014;3(1):50–57.
- Servín-Blanco R, Zamora-Alvarado R, Gevorkian G, et al. Antigenic variability: obstacles on the road to vaccines against traditionally difficult targets. Hum Vaccin Immunother. 2016;12(10):2640–2648.
- Pourseif MM, Yousefpour M, Aminianfar M, Moghaddam G, et al. A multi-method and structure-based in silico vaccine designing against Echinococcus granulosus through investigating enolase protein. Bioimpacts. 2019;9(3):131–144.
- Castro F, Cardoso AP, Gonçalves RM, et al. Interferon-gamma at the crossroads of tumor immune surveillance or evasion. Front Immunol. 2018;9:847.
- Toman M, Celer V, Kavanová L, et al. Dynamics and differences in systemic and local immune responses after vaccination with inactivated and live commercial vaccines and subsequent subclinical infection with PRRS virus. Front Immunol. 2019;10:1689.
- Choudhry H, Helmi N, Abdulaal WH, et al. Prospects of IL-2 in cancer immunotherapy. Biomed Res Int. 2018;2018:9056173.
- Sun Z, Ren Z, Yang K, et al. A next-generation tumor-targeting IL-2 preferentially promotes tumor-infiltrating CD8+ T-cell response and effective tumor control. Nat Commun. 2019;10(1):3874.
- Roomi MW, Niedzwiecki A, Rath M. Peptide vaccines directed against human metalloproteinases (MMPs) with anti-tumor efficacy in vitro and in vivo. J Cell Med Nat Health (CM&NH). 2018.
- Dosset M, Godet Y, Vauchy C, et al. Universal cancer peptide-based therapeutic vaccine breaks tolerance against telomerase and eradicates established tumor. Clin Cancer Res. 2012;18(22):6284–6295.
- Bezu L, Kepp O, Cerrato G, et al. Trial watch: Peptide-based vaccines in anticancer therapy. Oncoimmunology. 2018;7(12):e1511506.