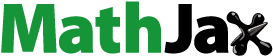
Abstract
Background
Combination HIV prevention approaches that include both biomedical and non-biomedical interventions often hold greater promise to improve health outcomes and reduce the risk of HIV transmission.
Objectives
Evaluate the relative properties of four leading candidate trial designs – ‘single-factor’, ‘multi-arm’, ‘all-in-one’, and ‘factorial’ designs – for assessing individual and/or combination prevention intervention approaches.
Methods
Monte-Carlo simulations are conducted, assuming a putative combination approach could choose its components from two candidate biomedical interventions, i.e. Treatment-as-Prevention (TasP) and Pre-exposure Prophylaxis (PrEP), and three candidate behavioral interventions, i.e. linkage-to-care, counseling, and use of condoms. Various scenarios for individual components’ effect sizes, their possible interaction, and the sample size based on real clinical studies are considered.
Results
The all-in-one and factorial designs used to assess a combination approach and the multi-arm design used to assess multiple individual components are consistently more powerful than single-factor designs. The all-in-one design is powerful when the individual components are effective without negative interaction, while the factorial design is more consistently powerful across a broad array of settings.
Conclusions
The multi-arm design is useful for evaluating single factor regimens, while the all-in-one and factorial designs are sensitive in assessing the overall efficacy when there is interest in combining individual component regimens anticipated to have complementary mechanisms. The factorial design is a preferred approach when assessing combination regimens due to its favorable power properties and since it is the only design providing direct insights about the contribution of individual components to the combination approach’s overall efficacy and about potential interactions.
Introduction
For more than two decades, several landmark clinical trials have been conducted evaluating biomedical interventions for the prevention of incident HIV infection among at-risk populations. Among those, in the setting of mother-to-child transmission are the evaluation of zidovudine in the AIDS Clinical Trials Group (ACTG) 076 trial,Citation1 nevirapine in the HIV Network for Prevention Trials (HIVNET) 012 trial,Citation2 antibiotics in the HIV Prevention Trial Network (HPTN) 024 trial,Citation3,Citation4 and the IMPAACT PROMISE Trial;Citation5 in the setting of heterosexual and/or homosexual transmissions are Treatment-as-Prevention (TasP) in the HPTN 052 trial,Citation6 Pre-exposure Prophylaxis (PrEP) in the Partners PrEP,Citation7 CDC 4940 TDF2,Citation8 iPrEx,Citation9 FemPrEP,Citation10 MTN 003 VOICE,Citation11 PROUD,Citation12 and iPERGAYCitation13 trials, as well as evaluation of microbicides to reduce heterosexual transmission in the HPTN 035Citation14 and the Microbicide Trials Network (MTN) 020 ASPIRE trial.Citation15 Other trials include PrEP evaluating injectables for Pre-exposure Prophylaxis (PrEP) in MSM (HPTN 083)Citation16 and in women (HPTN 084)Citation17 and those evaluating vaccines and monoclonal antibodies (HVTN 702, HVTN 703/HPTN 081, HVTN 704/HPTN 085 AMP, HVTN 705/HPX2008).Citation18–21 There have also been many trials that evaluated behavioral interventions. These include the evaluation of behavioral interventions among men having sex with men (MSM) in the HIVNET 015/EXPLORE trialCitation22 and community mobilization, mobile testing, same-day results and post-test support for HIV in HPTN 043/Project Accept Study.Citation23
Even with such promise provided by the TasP and PrEP regimens, their impact on reducing overall HIV incidence in real world settings would be compromised, for example, when proper uptake or adherence is not achieved. Therefore, combination prevention approaches that include both biomedical and behavioral intervention components have been advocated,Citation24 including those targeting associated social, economic, and other structural factors, such as the use of condoms, linkage-to-care, and HIV counseling, as resources and priorities are increasingly shifting towards community-level interventions to reduce HIV incidence. There is particular appeal to pursuing combination interventions having components with potential to work synergistically to increase their potency.
While most HIV prevention trials focused on the evaluation of one intervention, more recently, the PopART Trial (HPTN 071) conducted in Zambia and South Africa was designed to assess a combination of universal voluntary HIV counseling and testing provided at household level, linkage of HIV infected individuals to care and early initiation of antiretroviral therapy (ART) for all those testing HIV-positive.Citation25
There is an increasing need for trials such as PopART to assess combination intervention approaches that aim to optimize preventive and treatment efforts.Citation26 While such approaches have been efficiently and effectively evaluated in other settings such as the Women’s Health Initiative Trial,Citation27 challenges remain in designing and implementing combination intervention approaches in the HIV prevention setting. They tend to be scientifically and logistically complex to properly design and implement. These challenges, together with inadequate insights about the statistical properties of various designs for evaluating combination regimens, are contributing reasons for why few trials have been conducted for multi-component combination intervention approaches.
To gain more insight about which clinical trial design may be preferable in practice when assessing multiple intervention approaches in HIV prevention, we chose four leading candidate designs in this paper, and conducted extensive Monte-Carlo simulation studies to investigate evaluate their relative performance. These four leading candidate designs are:
Single-factor design. Each individual prevention intervention component is assessed in a separate, controlled randomized trial.
Factorial design. All possible combinations of multiple components are assessed in one controlled, randomized trial.
Multi-arm design. Individual prevention intervention components are assessed in one controlled, randomized trial.
All-in-one design. A regimen with ‘all’ prevention intervention components in the experimental arm is assessed in one controlled, randomized trial.
To enhance the generalizability of results, multiple scenarios were considered to assess the influence of the magnitude of true levels of effect, the impact of interaction between factors and the impact of trial size.
Methods
Monte-Carlo simulation studies were conducted to investigate properties of the four leading candidate trial designs. To enable the simulation studies to be realistic regarding prevention intervention components that might be included in a combination prevention intervention approach, we considered two key biomedical interventions: Pre-exposure Prophylaxis (PrEP) uisng antiretroviral therapy (ART) offered to eligible HIV-negative participants; and Treatment-as-Prevention (TasP) using ART offered to eligible HIV-positive participants. Three key behavioral interventions also were considered: Linkage-to-Care (LtC), Counseling, and Condom Use.
The relative properties of the four candidate trial designs were evaluated across a range of settings for the true effects of the five interventions. PrEP and TasP were assumed to have moderate and high efficacy, with corresponding hazard ratios (HR) set to be 0.56 (iPrEx study)Citation9 and 0.3 (HPTN 052),Citation28 respectively. Linkage-to-care, counseling, and consistent condom use are common behavioral interventions that could further improve the effectiveness when pursuing treatment-as-prevention (HIVNET 016 A).Citation29–32 The HRs of LtC, Counseling, and Condom Use were set to be 0.9, 0.8, and 0.7, respectively. In this paper, this serves as the base setting, labeled Setting I, and consists of two biomedical interventions with moderate and strong efficacies, as well as three behavioral interventions with a range of weak efficacies.
To gain additional insights, properties of the four candidate trial designs were assessed in three more settings:
In Setting IIa, the effect of PrEP was reduced from HR = 0.56 to HR = 0.78, while the other interventions retained their effects from Setting I.
In Setting IIb, LtC and Counseling were assumed to have no effects, (i.e. HR = 1.0), while the other three interventions retained their effects from Setting I.
Finally, in Setting III, while the most effective biomedical intervention, TasP, retained its full HR = 0.3 effect and the most effective behavioral intervention, Condom Use, retained its full HR = 0.7 effect, the effect of PrEP was meaningfully attenuated to HR = 0.9, and the LtC and Counseling interventions were assumed to have no effects, (i.e. HR = 1.0).
In summary, Setting I represents a scenario where the five interventions have their broadest effects; in turn, across the five interventions, there is increased heterogeneity of efficacy in the two scenarios in Setting II, and there is the most heterogeneity of efficacy in Setting III. More details to describe these settings can be found in Appendix.
As discussed in the Introduction, the four leading candidate designs with control arms having placebo (added to standard-of-care) were: ‘single-factor’, ‘two-way factorial’, ‘multi-arm’, and ‘all-in-one’ designs. In the single-factor design, the simplest design, participants receive either a single intervention or placebo. Participants in a factorial design receive one of following four options: a biomedical intervention, a behavioral intervention, the combination of that biomedical and that behavioral intervention, or neither intervention. In the multi-arm design, participants receive one of three options: a biomedical intervention, a behavioral intervention, or neither intervention. In the all-in-one design, participants receive either all five behavioral and biomedical interventions or none of these interventions.
As in any combination prevention intervention approach, potential interaction may exist between individual intervention components. In our simulation studies, the influence of interaction between interventions was considered. For a given intervention, the presence of another intervention could have no effect on the efficacy of that given intervention, or an enhancing effect (i.e. a synergistic effect or ‘positive’ interaction), or an attenuating effect (i.e. an antagonistic effect or ‘negative’ interaction). More details regarding the modeling of interaction terms can be found in the Appendix.
For an intervention ‘package’ (defined either as a single intervention or a combination of interventions) to be deemed to have a clinically meaningful and statistically reliable impactful, the upper bound of the confidence interval of its estimated HR should be less than a ‘margin’ or ‘cut-off’ that is formulated in the context of the off-target effects (i.e. safety) as well as the costs and complexity of the delivery of that package. To be specific, the cutoff for a given intervention package is calculated using a multiplicative factor of 0.9 for each behavioral intervention and a multiplicative factor of 0.5 for each biomedical intervention in that package.
In each Monte-Carlo simulation, the sample size was set to be 5000 participants, with an underlying constant hazard rate corresponding to a 2% annual HIV incidence in the control arm. To explore the influence of the total amount of information, three different censoring distributions were used that led to an average of 1.6, 4.26 and 7.2 years of follow-up (i.e. an average of 8000, 21300 and 36000 person-years of follow-up respectively). For each design, simulations were performed 1000 times. The analyses were based on the Cox proportional hazards models for the primary outcome of time-to-HIV-infection, subject to censoring. Hence, an individual efficacy is expressed by hazards ratio. For example, a hazards ratio of 0.3 for the TasP in the single-factor design means that there would be a (1-0.3)×100%. i.e. 70%, risk reduction of HIV acquisition associated with the TasP for HIV-negative partners of sero-discordant couples. For simplicity, we assumed that the primary analysis would be based on the principle of intention-to-treat (ITT), and censoring would be non-informative.
To assess the population impact of the various designs in real-world settings, the Public Health Impact (PHI) score was calculated for a given design, by taking the sum over all selections of interventions that would be possible under that design, where each term in that sum is the product of the probability that the set of interventions would be established to be effective and the true hazard ratio when that set of interventions would be used. In essence, the PHI provides the average relative reduction in the hazard that would be achieved by using that specific candidate trial design to evaluate that specific set of interventions in the specific clinical setting being considered.
For illustration, in the single factor design, participants receive either a single intervention or placebo. The only possible policy recommendations based on this trial would be to recommend the intervention or not. So, if is the underlying HR of an intervention B, the PHI associated with a single-factor design trial evaluating intervention B would be,
More technical details of model formulation and the definition of PHI score can be found in the Appendix. Simulations are performed in the statistical software R (v3.4.1).
Results
provides results for the situation in which a trial would have 8000 person-years follow-up and a control arm event rate of 2% per year, and where the trial would be conducted in Setting I, where true HRs of PrEP, TasP, LtC, Counseling, and Condom Use are 0.56, 0.3, 0.9, 0.8, and 0.7, respectively. Each entry in the table is based on the results of 1000 simulated trials conducted according to a trial design specific to the rows of the table and, for the rows corresponding to the factorial design, according to whether there is an interaction (as specified by the columns of the table) between the two regimens being assessed. In each of these situations, the table provides the normally distributed estimate of the logarithm of the HR, (denoted β̂); the precision of that estimate that is provided by its standard error (denoted se(β̂)), and the probability the trial establishes the component regimen (or the combination in the setting of the ‘all-in-one’ design) to have a clinically meaningful and statistically reliable impactful, achieved when the upper bound of the corresponding confidence interval in a simulated trial is less than the cut-off, ‘c’.
Table 1 Simulation results of setting ITable Footnote*
The β̂ column in the ‘No interaction’ situation provides the expected result that the estimates from the Cox proportional hazards analyses are essentially unbiased in the trial design setting corresponding to every row of the table. In the factorial design setting, if one fits a main effects model when there truly would be negative or positive interactions between the component interventions, the main effects parameter being estimated would be a weighted average of the true effect of the component intervention across the settings where the other component intervention is or is not being delivered.
The se(β̂) column is very informative about the relative efficiencies of these candidate trial designs. While the single-factor design would be quite efficient for assessment of the effect of an individual component in the trial (in the absence of other components), the factorial design provides very similar efficiency for the assessment of the biomedical component and only modestly reduced efficiency for the assessment of the behavioral component, (where this reduction results from the biomedical component’s influence in reducing the number of events in the trial). Hence, the increased efficiency of the factorial design relative to the single factor design is immediately apparent, nearly providing the ability to address the effects of two component interventions for the cost of the single factor trial that addresses the effect of only one component intervention. To provide improved intuition for these relative results in the single factor design and factorial design settings, it is noteworthy that the standard error of the estimate, se(β̂), for the effect of a component intervention is approximated by the square root of [4/L], where L is the number of participants in each trial having primary outcome events.
Since the multi-arm trial is sharing the events among three arms, each pairwise comparison in that trial design setting would have approximately two-thirds the number of events of the corresponding pairwise comparison in the fixed sample design setting.
The column for the probability of establishing effect by ruling out the relevant cut-offs does reflect the considerable sensitivity of the all-in-one design in Setting I where all components contribute benefits and where some are highly effective. This column also suggests that the factorial design and multi-arm design will be more efficient than the single-factor design. This is confirmed by . These three tables provide the Public Health Impact (PHI) for Settings I, IIb and III, respectively. The results in Setting IIa are not presented since those results are quite similar to those for Setting IIb. These tables also address the influence of total information on the relative properties by addressing situations where individual trials would have either 8000, 21300 or 36000 person-years follow-up on average.
Table 2 Public health impact of setting ITable Footnote*
Table 3 Public health impact of setting IIbTable Footnote*
Table 4 Public health impact of setting IIITable Footnote*.
The ‘column mean’ rows of provide a summary measure of the ‘average’ public health impact of each of these candidate trial designs, by taking an average over the six pairwise situations when one evaluates a biomedical and a behavioral intervention. In turn, the column mean row for the single factor design gives one-half weight to the two biomedical rows and one-half weight to the three behavioral rows.
Discussion
The results of these simulations directly address the properties in HIV prevention research of four candidate trial designs, were they to be implemented in specific settings where the components or combinations would have levels of benefit that, while not yet being reliably established, truly would be as assumed in these simulations. Importantly, the insights of these assessments would generalize to other HIV and non-HIV clinical settings, including present day HIV prevention research where the benefits of some prevention interventions already have been reliably established, as discussed in the Introduction. For example, in a setting where TasP and Condom Use would be viewed to be part of established standard-of-care, evidence-based advances in prevention could be achieved through clinical trials in which such standard-of-care would be provided to all study participants, with a placebo-controlled randomization to additional components, such as vaccines, monoclonal antibodies, PrEP or microbicides, using one of these leading candidate trial designs. In such a setting, given the reduced rate of transmission that would be expected in this established standard-of-care control arm, feasibility considerations would lead to even greater need for designs are that would provide timely and reliable results in an efficient manner.
When pursuing efficiency as well as reliability, the results in provide motivation to consider alternatives to the single-factor trial design in settings where there would be more than one new component intervention. If mechanisms of these experimental interventions are not complementary, the simulation results indicate the ‘multi-arm design’ consistently has better properties than the single-factor design, especially in larger trials having more events.
If mechanisms of experimental interventions are complementary, the all-in-one and factorial designs enable assessments of efficacy when of these interventions are used in combination. It is apparent from that factorial design is robustly superior to the single factor design. The properties of the all-in-one design are very sensitive to the level of heterogeneity of efficacy of the component interventions. When that heterogeneity is not large, as in , the all-in-one design has the greatest power. However, its properties are inferior when there is considerable heterogeneity of efficacy of component interventions, as seen in .
The presence of interactions does have effects on the relative properties of the multi-arm design and the factorial design. In all , the factorial design is more sensitive than the multi-arm design when there is no interaction, especially in smaller trials. Since the presence of interaction has greater influence on the power of the factorial design than on the power of the multi-arm design, the factorial design tends to be more powerful than the multi-arm design when there is positive interaction, while the reverse is true when there is negative interaction.
The power of the all-in-one design is meaningfully impacted by the presence of interaction, yet that design, like the multi-arm design, does not provide insights about which interventions experience interaction or, in turn, about the magnitude of that interaction. The factorial design not only has favorable efficiency properties, but also is the only design that would be able to identify the presence of interaction and its magnitude. Importantly, it also is the only design having the significant scientific and regulatory advantage of providing direct insights about contributions of components.
Further research would be useful in addressing the impact of stratification in the design and analysis, and in evaluating the properties of higher-level factorial design, such as a three-way factorial design. It also would be of interest to explore the impact on efficiency of allowing participants to select which factors to which they would choose to be randomized, as was allowed in the Women’s Health Initiative that used a ‘flexible’ factorial design to evaluate the effects of vitamins, diet and hormone replacement therapy.Citation27
Conclusion
When the main interest is in assessing each individual intervention component, the single-factor and multi-arm designs provide important design options, as they are simple and straightforward, although multiple trials may have to be performed. If participants would be eligible for more than one experimental intervention component, the multi-arm design would provide increased statistical and operational efficiency in comparisons with the control arm and would also provide important insights about the head-to-head comparisons of the experimental arms.
When there is interest in combining individual component regimens anticipated to have complementary mechanisms, alternatives to the single-factor design are needed. The all-in-one design has high power when the efficacies of the individual component interventions are consistently high, but not necessarily so when some components are rather ineffective or there are negative interactions.
The factorial design is more robustly powerful than the all-in-one design over a broad array of settings. It also has improved efficiency relative to the multi-arm design, even though the total number of events when using the factorial design would be reduced by the simultaneous use of more than one experimental intervention. The factorial design is the only trial design that provides insights about the interaction of intervention effects, which would be of considerable clinical importance in guiding decisions about whether to make simultaneous use of the experimental interventions. Unlike the all-in-one design, the factorial design also provides important clinical and regulatory insights about the contributing effects of individual interventions when the combination of those interventions has been established to be efficacious. Based on these considerations, it is a preferable trial design when there is interest in regimens based on combinations of individual components.
These conclusions about the favorable properties of factorial designs, based on consideration of HIV prevention research, could be generalized to other research settings, such as the COVID-19 pandemic where combinations of interventions also would be of interest. In the COVID-19 setting, factorial designs could provide reliable insights in a timely manner about the safety and efficacy of urgently needed prevention and therapeutic combination regimens. Further, their insights about the contribution of component interventions would be invaluable to the feasibility of achieving the wide scale implementation of effective COVID-19 interventions.
Disclosure statement
No financial interest or benefit has arisen from the direct applications of the research work presented in this paper.
Additional information
Funding
Notes on contributors
Ying Qing Chen
Ying Qing Chen, PhD, is a Professor of Biostatistics at the Vaccine and Infectious Disease Division and the Public Health Sciences Division, Fred Hutchinson Cancer Research Center in Seattle, Washington, USA.
Lili Peng
Lili Peng, MS, was a Statistical Research Associate at the Vaccine and Infectious Disease Division, Fred Hutchinson Cancer Research Center in Seattle, Washington, USA.
Yixin Wang
Yixin Wang, PhD, was a Post-Doctoral Fellow at the Vaccine and Infectious Disease Division, Fred Hutchinson Cancer Research Center in Seattle, Washington, USA.
Eline Appelmans
Eline Appelmans, MD, was a Research Associate and Project Manager at the Vaccine and Infectious Disease Division, Fred Hutchinson Cancer Research Center in Seattle, Washington, USA.
Sayan Dasgupta
Sayan Dasgupta, PhD, is a Staff Scientist at the Vaccine and Infectious Disease Division, Fred Hutchinson Cancer Research Center in Seattle, Washington, USA.
Thomas R. Fleming
Thomas R. Fleming, PhD, is a Professor of Biostatistics and Statistics at the Departments of Biostatistics and Statistics, University of Washington in Seattle, Washington, USA.
References
- Connor EM, Sperling RS, Gelber R, et al. Reduction of maternal-infant transmission of human immunodeficiency virus type 1 with zidovudine treatment. Pediatric AIDS Clinical Trials Group Protocol 076 Study Group. N Engl J Med. 1994;331(18):1173–1180.
- Jackson JB, Musoke P, Fleming T, et al. Intrapartum and neonatal single-dose nevirapine compared with zidovudine for prevention of mother-to-child transmission of HIV-1 in Kampala, Uganda: 18-month follow-up of the HIVNET 012 randomised trial. Lancet (London, England). 2003;362(9387):859–868.
- Guay LA, Musoke P, Fleming T, et al. Intrapartum and neonatal single-dose nevirapine compared with zidovudine for prevention of mother-to-child transmission of HIV-1 in Kampala, Uganda: HIVNET 012 randomised trial. Lancet (London, England). 1999;354(9181):795–802.
- Goldenberg RL, Mwatha A, Read JS, et al. The HPTN 024 Study: the efficacy of antibiotics to prevent chorioamnionitis and preterm birth. American Journal of Obstetrics and Gynecology 2006;194(3):650–661.
- Fowler MG, Qin M, Fiscus SA, et al. Benefits and Risks of Antiretroviral Therapy for Perinatal HIV Prevention. N Engl J Med. 2016;375(18):1726–1737.
- Cohen MS, Chen YQ, McCauley M, et al. Prevention of HIV-1 infection with early antiretroviral therapy. N Engl J Med. 2011;365(6):493–505.
- Pattacini L, Murnane PM, Baeten JM, Partners PrEP Study Team, et al. Antiretroviral pre-exposure prophylaxis does not enhance immune responses to HIV in exposed but uninfected persons. J Infect Dis. 2015;211(12):1943–1952.
- Thigpen MC, Kebaabetswe PM, Paxton LA, et al. Antiretroviral preexposure prophylaxis for heterosexual HIV transmission in Botswana. N Engl J Med. 2012;367(5):423–434.
- Grant RM, Lama JR, Anderson PL, et al. Preexposure chemoprophylaxis for HIV prevention in men who have sex with men. N Engl J Med. 2010;363(27):2587–2599.
- Van Damme L, Corneli A, Ahmed K, et al. Preexposure prophylaxis for HIV infection among African women. N Engl J Med. 2012;367(5):411–422.
- Marrazzo JM, Ramjee G, Richardson BA, et al.; VOICE Study Team. Tenofovir-based preexposure prophylaxis for HIV infection among African women. N Engl J Med. 2015;372(6):509–518.
- Mccormack S, Dunn DT, Desai M, et al. Pre-exposure prophylaxis to prevent the acquisition of HIV-1 infection (PROUD): effectiveness results from the pilot phase of a pragmatic open-label randomized trial. The Lancet. 2016;387(10013):53–60.
- Molina JM, Capitant C, Spire B, et al.; ANRS IPERGAY Study Group. On-Demand Preexposure Prophylaxis in Men at High Risk for HIV-1 Infection. N Engl J Med. Dec 3 2015;373(23):2237–2246.
- Guffey MB, Richardson B, Husnik M, et al.; HIV Prevention Trials Network (HPTN) 035 Study Team. HPTN 035 phase II/IIb randomised safety and effectiveness study of the vaginal microbicides BufferGel and 0.5% PRO 2000 for the prevention of sexually transmitted infections in women. Sex Transm Infect. Aug 2014;90(5):363–369.
- Baeten JM, Palanee-Phillips T, Brown ER, et al. Use of a vaginal ring containing dapivirine for HIV-1 prevention in women. N Engl J Med. Dec 1 2016;375(22):2121–2132.
- HPTN. HPTN 083: a phase 2b/3 double blind safety and efficacy study of injectable cabotegravir compared to daily oral Tenofovir Disoproxil Fumarate/Emtricitabine (TDF/FTC), for pre-exposure prophylaxis in HIV-uninfected cisgender men and transgender women who have sex with men. https://www.hptn.org/research/studies/hptn083#block-views-block-study-related-publications-block-1. Published July 20, 2020. Accessed November 1, 2018.
- HPTN. HPTN 084: a phase 3 double blind safety and efficacy study of long-acting injectable cabotegravir compared to daily oral TDF/FTC for pre-exposure prophylaxis in HIV-uninfected women. https://www.hptn.org/research/studies/hptn083#block-views-block-study-related-publications-block-1. Published July 20, 2020. Accessed November 1, 2018.
- HVTN. uhambo(HVTN 702): a pivotal phase 2b/3 multi-site, randomized, double-blind, placebo-controlled clinical trial to evaluate the safety and efficacy of ALVAC-HIV (vCP2438) and Bivalent Subtype C gp120/MF59 in preventing HIV-1 infection in adults in South Africa. http://uhambo.org.za/. Published July 20, 2020. Accessed December 2, 2018.
- HPTN Ha. AMP(HVTN 703/HPTN 081): a phase 2b study to evaluate the safety and efficacy of VRC01 broadly neutralizing monoclonal antibody in reducing acquisition of HIV-1 infection in women in sub-Saharan Africa. https://ampstudy.org.za/about. Published July 20, 2020. Accessed December 2, 2018.
- HPTN Ha. AMP(HVTN 704/HPTN 085): a phase 2b study to evaluate the safety and efficacy of VRC01 broadly neutralizing monoclonal antibody in reducing acquisition of HIV-1 infection among men and transgender persons who have sex with men. https://ampstudy.org/. Published July 20, 2020. Accessed December 2, 2018.
- HVTN. IMBOKODO (HVTN 705/HPX2008): a multicenter, randomized, double-blind, placebo-controlled phase 2b efficacy study of a heterologous prime/boost vaccine regimen of Ad26.Mos4.HIV and aluminum phosphateadjuvanted Clade C gp140 in preventing HIV-1 infection in adult women. https://www.imbokodo.org.za/. Published July 20, 2020. Accessed December 2, 2018.
- Koblin B, Chesney M, Coates T. Effects of a behavioural intervention to reduce acquisition of HIV infection among men who have sex with men: the EXPLORE randomised controlled study. Lancet (London, England). 2004;364(9428):41–50.
- Coates TJ. An expanded behavioral paradigm for prevention and treatment of HIV-1 infection. Journal of Acquired Immune Deficiency Syndromes 2013;63:S179–S182.
- UNAIDS Report on the Global AIDS Epidemic Geneva; 2010.
- Hayes RA, Beyers N, Sabapathy K, et al.; HPTN 071 (PopART) Study Team. HPTN 071 (PopART): rationale and design of a cluster-randomised trial of the population impact of an HIV combination prevention intervention including universal testing and treatment - a study protocol for a cluster randomised trial. Trials 2014;15:57.
- Floyd S, Ayles H, Schaap A, et al.; HPTN 071 (PopART) Study Team. Towards 90-90: findings after two years of the HPTN 071 (PopART) cluster-randomized trial of a universal testing-and-treatment intervention in Zambia. PLoS One. 2018;13(8):e0197904.
- Design of the Women's Health Initiative clinical trial and observational study. The Women's Health Initiative Study Group. Controlled Clinical Trials Feb 1998;19(1):61–109.
- Jia Z, Mao Y, Zhang F, et al. Antiretroviral therapy to prevent HIV transmission in serodiscordant couples in China (2003-11): a national observational cohort study. Lancet (London, England). Oct 5 2013;382(9899):1195–1203.
- Ahmed S, Lutalo T, Wawer M, et al. HIV incidence and sexually transmitted disease prevalence associated with condom use: a population study in Rakai. Uganda. Aids Nov 9 2001;15(16):2171–2179.
- Kumwenda N, Hoffman I, Chirenje M, et al. HIV incidence among women of reproductive age in Malawi and Zimbabwe. Sex Transm Dis. Nov 2006;33(11):646–651.
- Kranzer K, Zeinecker J, Ginsberg P, et al. Linkage to HIV care and antiretroviral therapy in Cape Town, South Africa. PLoS One. Nov 2 2010;5(11):e13801
- Maheu-Giroux M, Tanser F, Boily MC, Pillay D, Joseph SA, Barnighausen T. Determinants of time from HIV infection to linkage-to-care in rural KwaZulu-Natal, South Africa. Aids. Apr 24 2017;31(7):1017–1024.
Appendix
Interaction
We further consider scenarios which involve interaction between the effects of the biomedical and the behavioral intervention. We consider three different scenarios of interaction
No interaction: When the mitigating effects of the biomedical and behavioral interventions on HIV transmission are multiplicative, or that there is no interaction between their effects when used in a combination therapy.
Positive interaction: There is a positive interaction between the effects of a biomedical and behavioral intervention when they are used in a combination therapy. Throughout the simulation study, the positive interaction scenarios are created by the rule that in presence of a biomedical intervention, the effect of the behavioral intervention is squared. In Design D, when all five interventions are present, only the effect of the behavioral intervention Condoms is squared in the positive interaction scenario.
Negative interaction: There is a negative interaction between the effects of a biomedical and behavioral intervention when they are used in a combination therapy. Throughout the simulation study, the negative interaction scenarios are created by the rule that in presence of a biomedical intervention, the effect of the behavioral intervention is reduced to 0. In Design D, when all five interventions are present, only the effect of the behavioral intervention Condoms is reduced to 0 in the negative interaction scenario.
Detailed interaction scenarios
Following our discussion on the interaction scenarios in the main text, we provide a more detailed formulation of these scenarios below:
Formulation of the Interaction scenarios in two factor designs (Designs B and C). Let us now consider a Biomedical intervention (Bi) with underlying Hazard Ratio (HR)
and a Behavioral intervention (Be) with underlying HR
We note the Hazard Ratio for the combination of these two interventions in different interaction scenarios below:
No interaction: HR of the combination therapy,
Positive interaction: HR of the combination therapy,
Negative interaction: HR of the combination therapy,
Formulation of the Interaction scenarios in Design D. Let the specific Hazard Ratios for the two Biomedical interventions, TasP and PrEP be respectively
and
Similarly let the specific Hazard Ratios for the three Behavioral interventions, LtC, Counseling and Condoms be respectively
and
We now note the Hazard Ratio for the combination of these five interventions in different interaction scenarios below:
No interaction: HR of the combination therapy,
Positive interaction: HR of the combination therapy,
Negative interaction: HR of the combination therapy,
Detailed HR settings
(1) Setting I (Robust Benefit): In the first setting we consider, each intervention has a meaningful underlying efficacy. Hazard ratio (HR) for PrEP is set at 0.56 (following the results of the iPrEx study [Grant RM et al (2010)]) and that for TasP is set at 0.3 (following the results of HPTN 052), while the hazard ratios of the three behavioral interventions, namely LtC, Counseling and Condoms Use are respectively set at 0.9, 0.8 and 0.7 respectively. So, while the biomedical interventions are highly effective, the behavioral interventions are only marginally effective, LtC being the least effective among them. Condoms Use, on the other hand is the most effective, and is associated with a 30% risk reduction in HIV acquisition. Under Design A, each intervention is evaluated separately in a randomized clinical trial. Under Design B, a pair of a biomedical and a behavioral intervention are evaluated in a two-factor randomized factorial trial, while in Design C, one biomedical only arm and one behavioral only arm is evaluated against the placebo-control arm. In both these Designs, which simultaneously consider two interventions for their evaluation and subsequent implementation, the efficacy of a combination therapy that implements both of them depends on the underlying interaction scenario. In the no interaction scenario, the efficacy of the combination is just the product of their individual efficacies. For example, the hazard ratio of a combined regimen of PrEP and Condoms Use is given as 0.56 × 0.7 = 0.392 in this scenario. In the positive interaction scenario, the efficacy of such a combination therapy is given by the product of the efficacy of the biomedical intervention with and that of the behavioral squared. Hence, the hazard ratio of a combined regimen of PrEP and Condoms Use in this scenario will be equal to 0.56 × 0.72=0.2744. On the other hand in the negative interaction scenario, the efficacy of such a combination therapy is given just by the efficacy of the biomedical intervention alone, such that the hazard ratio of a combined regimen of PrEP and Condoms Use in this scenario is just equal to the HR of PrEP alone, 0.56. Under Design D, one arm receives all five interventions, while the other arm receives none. Just like Designs B and C, the efficacy of the combination of all five therapies in Design D will depend on the underlying interaction scenario. Again, in the no interaction scenario, the efficacy of the combination therapy is the product of their individual efficacies, that is, HR = 0.56 × 0.3 × 0.9 × 0.8 × 0.7 ≈ 0.0847. In the positive interaction scenario, the efficacy of such a combination therapy is given by the product of the squared efficacy of the Condoms Use with the efficacy of each of the two biomedical and two remaining behavioral interventions, that is, HR = 0.56 × 0.3 ×0.9 × 0.8 × 0.72 ≈ 0.0593. In the negative interaction scenario, the efficacy of such a combination therapy is given by the product of the efficacy of each of the biomedical and behavioral interventions, except for Condoms Use, that is, HR = 0.56 × 0.3 × 0.9 × 0.8 ≈ 0.1210.
(2) Setting II (Several ineffective): In the second setting we consider here, several of the behavioral interventions are ineffective, and PrEP also has only a marginal effect on the risk of HIV acquisition. Hazard ratio for PrEP is set at 0.7 here, while that for TasP is 0.3. Two of the behavioral interventions, LtC and Counseling, have hazard ratios equal to 1, while that of Condoms Use is still 0.7. As mentioned before, the efficacy of a combination therapy of two interventions (one biomedical and one behavioral), pertinent to Designs B and C, will depend on the interaction scenario. In the no interaction scenario, the underlying hazard ratio of a combined regimen of PrEP and Condoms Use is given as 0.7 × 0.7 = 0.49. In the positive interaction scenario, the hazard ratio of such a combined regimen will be equal to 0.7 ×0.72 = 0.343. On the other hand, in the negative interaction scenario, it will be just equal to 0.7. In Design D, in the no interaction scenario, the hazard ratio of the combination therapy is given as, HR = 0.7 × 0.3 ×1 × 1 × 0.7 = 0.147. In the positive interaction scenario, the hazard ratio is equal to 0.7 × 0.3 ×1 × 1 × 0.72 = 0.1029, while in the negative interaction scenario, it is given as, HR = 0.7 × 0.3 × 1 × 1 = 0.21.
(3) Setting III (Intermediate 1): The third setting we consider here, referred to as the first of two intermediate settings, is very similar to Setting 1, except that the efficacy of PrEP is attenuated somewhat from Setting 1. Hazard ratio for PrEP is set at 0.78 here, while that for TasP continues to be 0.3. Similarly, the behavioral interventions, LtC, Counseling, and Condoms Use have hazard ratios equal to 0.9, 0.8 and 0.7 respectively. Again, the efficacy of a combination therapy of two interventions, one biomedical and the other behavioral, will depend on the interaction scenario. In the no interaction scenario, the hazard ratio of a combined regimen of PrEP and Condoms Use will be equal to 0.78 × 0.7 = 0.546. In the positive interaction scenario, the hazard ratio of the combined regimen of PrEP and Condoms Use will be equal to 0.78 × 0.72=0.3822. On the other hand, in the negative interaction scenario, its hazard ratio will be equal to 0.78. In Design D, in the no interaction scenario, the hazard ratio of the combination therapy is given as, HR = 0.78 × 0.3 × 0.9 × 0.8 × 0.7 ≈ 0.1180. In the positive interaction scenario, it is equal to 0.78 × 0.3 × 0.9 × 0.8 × 0.72 ≈ 0.0826, while in the negative interaction scenario, it is given as, HR = 0.7 × 0.3 × 0.9 × 0.8 ≈ 0.1685.
(4) Setting IV (Intermediate 2): In the last setting we consider here, also the second of two intermediate settings we have here, is somewhat similar to both Setting 1 and 2. The efficacies of the biomedical interventions remain the same as Setting 1, that is, PrEP and TasP have respective hazard ratios of 0.56 and 0.3. Similarly the hazard ratio of Condoms Use is still 0.7. In fact, the hazard ratios of TasP and Condoms Use stay the same across these four settings. However, like Setting 2, LtC and Counseling have no contribution to reduction in the risk of HIV, and both have hazard ratio equal to 1. For a combination therapy of one biomedical and one behavioral intervention, when there is no interaction, the hazard ratio of a combined regimen of PrEP and Condoms Use will be equal to 0.56 × 0.7 = 0.392. In the positive interaction scenario, the hazard ratio of the combined regimen of PrEP and Condoms Use will be equal to 0.56 × 0.72 = 0.2744. In the negative interaction scenario, it will be equal to 0.56. In Design D, in the no interaction scenario, the hazard ratio of the combination therapy is given as, HR = 0.56 × 0.3 × 1 ×1 × 0.7 = 0.1176. In the positive interaction scenario, the hazard ratio is equal to 0.56 × 0.3 × 1 ×1 × 0.72 ≈ 0.0823, while in the negative interaction scenario, it is given as, HR = 0.56 × 0.3 × 1 × 1 = 0.168.
PHI score
To assess and compare the population impact of a specific design in assessing one or more of these interventions in real-world settings, we calculate its Public Health Impact (PHI) score as a weighted sum of the underlying efficacies associated with each of the specific interventions or their combinations. In this section, let’s consider a Biomedical intervention (Bi) with underlying Hazard Ratio (HR) and a Behavioral intervention (Be) with underlying HR
and define the PHI score for each of the designs under different interaction scenarios.
(1) Design A (Single factor). Let be the probability that Bi is selected for real-world implementation from a trail employing design A. Then the PHI score for the trial involving the Biomedical intervention Bi is given as
Let
be the same for the behavioral intervention Be. Then the PHI score for the trial involving the Behavioral intervention Be is given as
(2) Design B (Factorial). Let be the probability that only Bi is selected for real-world implementation from a trial employing design B in interaction scenario
where ‘noint’ denotes no interaction, ‘pos’ denotes positive interaction, and ‘neg’ denotes negative interaction. Similarly let
be the probability that only Be is selected and let
be the probability that both Bi and Be are selected in scenario
Then the PHI scores for a design B trial involving both interventions under different interaction scenarios are given as:
No interaction:
Positive interaction:
Negative interaction:
(3) Design C (Multi-arm). Let be the probability that only Bi is selected,
be the probability that only Be is selected and let
be the probability that both Bi and Be are selected for real-world implementation from a trial employing Design C. Then the PHI scores for a design C trial involving both interventions in different interaction scenarios are given as:
No interaction:
Positive interaction:
Negative interaction:
(4) Design D (All-in-one). In a trial employing Design D, the combination of all five interventions will be given to one arm of the trial, while the other arm will only receive the control. Let the specific Hazard Ratios for the two Biomedical interventions, TasP and PrEP be respectively and
Similarly let the specific Hazard Ratios for the three Behavioral interventions, LtC, Counseling and Condoms be respectively
and
Now if we let
be the probability that the combination of all 5 interventions is selected for implementation. Then the PHI score for a design D trial involving all interventions in different interaction scenarios are given as:
No interaction:
Positive interaction:
Negative interaction: