Abstract
Autophagy, a major lysosomal degradation process, has been implicated in the pathogenesis of colorectal cancer (CRC). However, it still remains unclear what role all the autophagy-related genes (ARGs) play in CRC prognosis. A univariate Cox analysis was conducted to select ARGs related with overall survival (OS) for CRC cohort of the TCGA database. A multivariate Cox analysis was used to develop an ARGs expression signature, whose risk score stratified CRC patients into two groups. The OS time between the above two groups was estimated using the Kaplan–Meier curve and log-rank test. In order to evaluate the predictive performance of this expression signature and other clinicopathological parameters for CRC prognosis, the area under the curve (AUC) was calculated. Nine out of 20 OS-related ARGs were finally incorporated into this ARGs expression signature, whose risk score stratified CRC patients into either high-risk or low-risk group. The OS time of patients in low-risk group was higher than that in high-risk group and the difference was statistically significant (P < 0.001). The AUC for this risk score was higher than other clinicopathological parameters, including TNM stage. This study presents an ARGs expression signature, which outperforms other clinicopathological parameters in predicting CRC prognosis.
Introduction
Autophagy is a major catabolic mechanism by which denatured, damaged or aging organelle and protein are delivered to lysosome for self-degradation in eukaryotic cells (Bagherniya et al. Citation2018; Packer Citation2020). By eliminating these abnormal macromolecular components, autophagy contributes to the maintenance of intracellular homeostasis (Fang et al. Citation2020). Autophagy abnormality participates in the development of many diseases, including immune dysfunction, neurodegenerative disease, chronic disease and tumor (Ait-Aissa et al. Citation2020; Li et al. Citation2020; Zhao and Zhang Citation2019). However, it still remains controversial what role autophagy plays in tumor (Levy et al. Citation2017; Xu et al. Citation2020). In premalignant stage, stimulation of autophagy could prevent cancer development. However, increased autophagic flux enables tumor growth in advanced cancer. Thus, it is valuable to further explore the role of autophagy in tumor, which could help provide the important lessons for cancer therapies by deliberately manipulating autophagy.
Colorectal cancer (CRC) is currently one of the most frequent malignant tumors (Abu et al. Citation2020; Mauri et al. Citation2019; Zhou et al. Citation2020). With the increasing incidence of CRC in the young, there will be an estimated 2.5 million newly diagnosed CRC cases in 2035. Although the current treatment options, including surgery, chemotherapy and molecular targeting agents have been used to extend survival for CRC patients, poor prognosis still remains a serious problem (Al Bandar and Kim Citation2017; Dekker et al. Citation2019). Although the TNM classification was frequently used to predict CRC prognosis (Amin et al. Citation2017), it could not sufficiently assess individual patient outcome. Moreover, patients within the same TNM stage could have a significant difference in clinical prognostication (Huang et al. Citation2019). Therefore, it is essential to identify novel potential biomarkers and gene expression signature for CRC prognosis.
Recently, accumulating evidence has reported that autophagy is implicated in CRC development and progression, which could provide a new insight for CRC therapeutic strategies by selectively targeting autophagy (Mokarram et al. Citation2017). For example, Wei et al. found that FAT4 could suppress epithelial–mesenchymal transition (EMT) of CRC by stimulating autophagy in part through the P13K-AKT signaling pathway (Wei et al. Citation2019). Yu et al. revealed that gut microbiota could promote CRC resistance to chemotherapy by activating the autophagy pathway (Yu et al. Citation2017). However, previous researches only highlighted the role of one or a few autophagy-related genes (ARGs) in CRC. Thus, the purpose of this research focuses on the development of a novel autophagy-related expression signature for CRC prognosis, based on a comprehensive analysis of the ARGs.
Materials and methods
Data acquisition
Two hundred and thirty-two human genes involved in autophagy were collected from the Human Autophagy Database (HADb, http://www.autophagy.lu/clustering/index.html). Both expression profiles of ARGs in RNA-sequencing (RNA-seq) and clinical data of CRC cohort were obtained and extracted from TCGA website (https://portal.gdc.cancer.gov/).
Enrichment analysis of differentially expressed ARGs
Differentially expressed ARGs between CRC and non-tumor samples were determined using limma package in R software. The adjust P-value < 0.05 and |log2 fold change (FC)| > 1 were chosen as the threshold criteria. Then, the gene ontology (GO) and Kyoto Encyclopedia of Genes and Genomes (KEGG) enrichment analysis of these differentially expressed genes were performed to find the biological significance and essential pathways by clusterprofiler R package. To provide visual information, the enriched results for GO and KEGG analysis were presented as the bubble plot and circle map, respectively.
Development of an autophagy-related gene signature
Log2 (count+1) transformation was used to normalize ARGs expression profiles of TCGA-CRC cohort. Univariate Cox analysis was first conducted to select the ARGs remarkedly related with overall survival (OS) of TCGA-CRC cohort. Subsequently, multivariate Cox regression analysis was performed using the above OS-related ARGs. Some genes, which might not serve as an independent predictor in CRC prognosis, were removed. Finally, an autophagy-related gene signature incorporating several prognostic genes was developed based on ARGs expression values multiplied by Cox regression coefficients. CRC patients were then split into two groups with a cutoff point of the median risk score. The OS time between the above two groups was estimated using the Kaplan–Meier curve and log-rank test. To explore whether the risk score could independently predict OS of TCGA-CRC cohort, we performed the multivariate Cox analysis by survival R package. Moreover, receiver operating characteristic (ROC) curve with multiple clinicopathological variables were plotted and area under the curve (AUC) was measured to evaluate the predictive performance by survivalROC R package. Finally, this ARGs expression signature was transformed into a visual nomogram by rms R package.
Statistical analysis
R software was used to perform all the statistics. Statistical difference was found with P-value no more than 0.05. Univariate and multivariate Cox analysis were performed to screen the OS-related ARGs and develop the ARGs expression signature, respectively.
Results
Identification of differentially expressed ARGs
TCGA-CRC cohort consisted 568 tumor and 44 non-tumor samples, whose RNA-seq and clinical data were downloaded. A total of 541 CRC patients with available gene expression value and OS time were finally included in this research. Expression values of 232 ARGs were extracted. Thirty-seven differentially expressed ARGs were obtained with the threshold criteria of FDR < 0.05 and |log2 FC| > 1 (Figure (A)). To visualize the expression profiles of these genes between CRC and non-tumor samples, a box plot was displayed, in which 19 up-regulated genes (FAS, CAPN10, SESN2, PINK1, GABARAP, TP53INP2, ERO1A, CAPN2, TNFSF10, VEGFA, BID, BIRC5, HSP90AB1, MYC, CDKN1A, CD46, EIF4EBP1, ATIC, BCL2L1) and 18 down-regulated genes (GRID2, NRG3, MAP1LC3C, TMEM74, NRG2, IFNG, CCR2, NRG1, PRKN, TP73, ATG9B, CDKN2A, SPHK1, HSPB8, NKX2−3, FKBP1B, ITPR1, BCL2) were identified (Figure (B)).
Figure 1. Gene enrichment analysis of differentially ARGs. (A) Heat map shows differentially expressed ARGs between CRC and non-tumor samples. (B) Box plot identifies 19 up-regulated genes and 18 down-regulated genes. (C) GO analysis shows the biological process, cellular component, and molecular function of differentially expressed ARGs. (D) KEGG analysis shows the signaling pathway involved in differentially expressed ARGs.
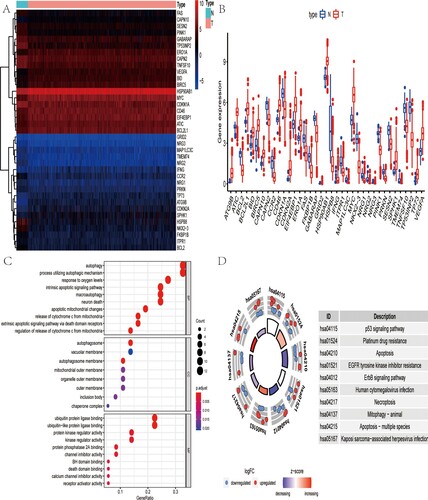
Enrichment analysis of differentially expressed ARGs
To further understand the biological significance of 37 differentially expressed ARGs, we performed GO and KEGG functional enrichment analysis. As was shown in Figure (C), the top GO enrichment terms for molecular function were: ubiquitin protein or ubiquitin-like protein ligase binding, kinase or protein kinase regulator activity; for cellular components were: autophagosome, vacuolar membrane, and autophagosome membrane; and for biological process were: autophagy, process utilizing autophagic mechanism and response to oxygen levels. Besides, the KEGG results revealed that these genes were mainly enriched in p53 signaling pathway, platinum drug resistance, apoptosis and the like (Figure (D)).
Identification of prognostic ARGs
The association between ARGs expression value of 541 TCGA-CRC patients and OS was investigated, resulting in 20 OS-related ARGs. As was shown in Figure (A), most of these 20 genes were identified as risk factors. Considering the potential clinical significance, we further investigated the genetic mutations of these ARGs via cBioportal database. The results indicated that among 592 CRC patients, genetic mutation was found in 483 patients and the mutation rate was as high as 82%. The top five genes with genetic mutation were WIPI2, ATIC, RAB7A, NRG1 and MAPK9, with mutation rate of 35, 16, 15, 14 and 12%, respectively (Figure (B)).
Figure 2. Genetic alteration of OS-related ARGs. (A) Forest plot shows 20 OS-related ARGs of TCGA-CRC cohort by univariate Cox regression analysis. (B) Top 5 ARGs with genetic mutation were WIPI2, ATIC, RAB7A, NRG1 and MAPK9, with mutation rate of 35, 16, 15, 14 and 12%, respectively.
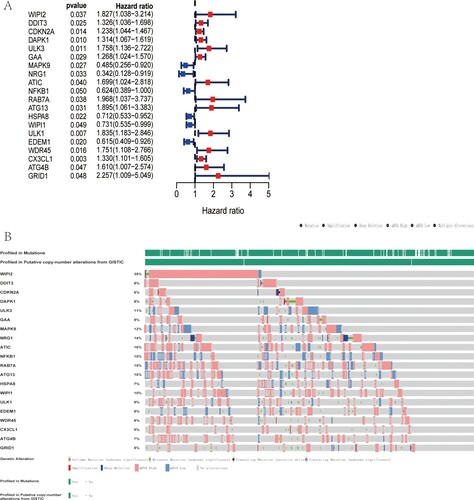
Based on the above prognostic ARGs in univariate analysis, multivariate analysis was conducted to develop the ARGs expression signature, whose risk score was [DAPK1 expression * (0.435964094)] + [ULK3 expression * (0.395252108)] + [ATIC expression * (0.630860629)] + [NFKB1 expression * (−0.584152048)] + [RAB7A expression * (0.982036429)] + [WIPI1 expression * (−0.261064362)] + [EDEM1 expression * (−0.626851909)] + [CX3CL1 expression * (0.175440139)] + [GRID1 expression * (1.48676544)]. By calculating the risk value of each patient, we divided CRC patients into two groups based on the median risk score. As was shown in Figure (A), the patients in low-risk group had longer OS time than that in high-risk group. Besides, the heatmap of prognostic ARGs expression profiles in multivariate analysis, distribution of risk score of CRC patients and relationship between survival time and risk score were presented in Figure (B,C). The AUC for risk score was 0.716, which was higher than tumor stage (AUCstage = 0.709), TNM stage (AUCT = 0.673, AUCN = 0.656, AUCM = 0.647), age (AUCage = 0.646) and gender (AUCgender = 0.433) (Figure (D)). Then, the value of the risk score in prognosis of CRC was evaluated. Univariate analysis revealed that the risk score was remarkedly related with OS (Figure (A)). Multivariate analysis showed that the risk score could independently predict CRC prognosis (Figure (B)).
Figure 3. Characteristics of this autophagy-related gene signature. (A) Kaplan–Meier analysis reveals that patients in low-risk group have longer OS time than that in high-risk group. (B) Heatmap of the expression profile of prognosis-related ARGs. (C) Distribution of risk score of CRC patients and relationship between survival time and risk score. (D) The AUC for risk score is higher than other clinicopathological parameters, including TNM stage.
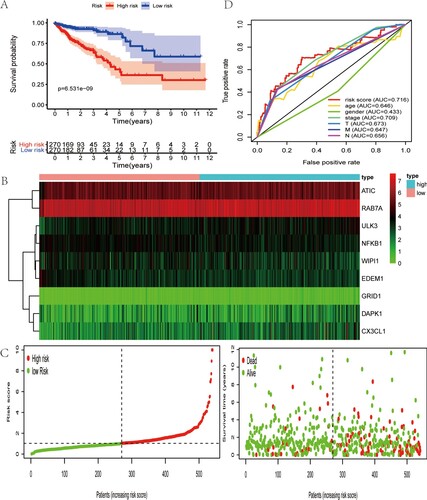
Clinical utility of prognostic signature and construction of the nomogram
Finally, the association between risk score and various clinicopathological parameters was analyzed. Results revealed that the risk score was positively related with TNM stage (PT = 0.025, PN < 0.001, PM = 0.009) and tumor stage (P < 0.001) (Figure ). To help clinicians predict prognosis of CRC patients more conveniently, the ARGs expression signature was finally presented as a visual nomogram (Figure ).
Discussion
CRC is a major lethal malignant tumor that urgently requires effective prognostic biomarkers (Shi et al. Citation2018). Accumulating evidence has identified the role of autophagy in the pathogenesis of CRC, which opens a new perspective for its diagnosis and prognosis (Wei et al. Citation2019; Yu et al. Citation2017). However, most of the previous studies only focused on the role of a single gene from the perspective of autophagy. A comprehensive analysis of relationship between all the ARGs expression profiles and CRC prognosis was not performed.
With the advances in sequencing technology, many databases, including TCGA and GEO, were used to screen significant autophagy-related gene signatures (An et al. Citation2018; Mo et al. Citation2019; Wang et al. Citation2019). To search molecular biomarkers for CRC prognosis, the ARGs expression profiles of TCGA-CRC cohort were deeply mined in our research. First, by comparing expression value between CRC and non-tumor samples, 37 differentially expressed ARGs were identified, of which GO and KEGG analysis were performed. Interestingly, the enrichment results revealed that ubiquitin protein ligase binding and p53 signaling pathway were the most significant molecular function and KEGG pathway. On the basis of these results, we hypothesized that these differentially ARGs may function as ubiquitin protein ligase, which could bind to p53 tumor suppressor gene, resulting in its ubiquitylation and subsequent degradation (Khoo et al. Citation2014). Dysregulation of p53 was involved in the pathogenesis of CRC (Li et al. Citation2015). This may provide a new insight into targeting p53-binding ARGs for CRC therapy.
Twenty ARGs were found associated with OS of TCGA-CRC cohort in univariate survival analysis. Then, nine key prognosis-related ARGs identified in multivariate analysis were used to develop an autophagy-related gene signature, which could serve as an independent predictor in CRC prognosis. Besides, this gene signature was useful for stratifying outcome of CRC patients. Accumulating evidence revealed that the existing TNM stage could not accurately predict the prognosis between stage II c and III a in CRC, partly due to overestimating the weight of N stage and underestimating the role of T stage (Li et al. Citation2014; Citation2016). Our research revealed that this ARGs expression signature outperformed other clinicopathological parameters including TNM stage, in predicting survival of CRC patients, which could provide a new strategy for CRC prognosis.
Most of these nine genes have been found related with the prognosis of CRC or other malignant tumors. DAPK1 was reported to be a metastasis suppressor, which was associated with its role of inhibiting integrin-mediated cell adhesion and migration. Decreased DAPK1 protein was found related with the metastasis and worse prognosis of multiple malignant tumors including CRC (Steinmann et al. Citation2019). ULK3, as a serine and threonine kinase, was involved in the phosphorylation of GL1 proteins to promote their transcriptional activity, resulting in positive regulation of Hedgehog pathway (Maloverjan et al. Citation2010). Abnormal activation of Hedgehog pathway was strongly implicated in the pathogenesis of various cancers, including CRC (Chowdhury et al. Citation2013). ATIC, as a key enzyme involved in the de novo purine synthesis, was strongly related with the tumor proliferation. Li et al reported aberrant ATIC upregulation would result in proliferation, migration and colony formation of hepatocellular cells by affecting the AMPK-mTOR-S6 K1 pathway, a pivotal axis protecting against various pathological alterations (Li et al. Citation2017; Citation2020). NFKB1 worked as a transcription factor, which was strongly implicated in the relationship between chronic inflammation and CRC (Patel et al. Citation2018). Cooks et al. demonstrated NF-κB activation induced p53 suppressor gene mutation, which would aggravate inflammatory response and participate in inflammation-related CRC carcinogenesis (Cooks et al. Citation2013). RAB7A, as a member of RAS oncogene family, encoded Rab-7a protein, which participated in the multiple biological processes, including apoptosis, cell cycle arrest and EMT. Xie et al. revealed that Rab7a overexpression would promote the ability of proliferation, growth and migration in breast cancer (Xie et al. Citation2019). CX3CL1 was a multifunctional chemokine, which could promote cancer survival and recruit anti-tumor leukocytes. In CRC, Conroy et al. reported higher CX3CL1 was positively related with higher tumor infiltrations of lymphocytes, resulting in inhibition of metastasis and better prognosis (Conroy and Lysaght Citation2020). Su et al. reported that no relationship was found between the polymorphism of GRID1 and CRC in the Chinese Han population (Su et al. Citation2015). However, in our research, GRID1 overexpression was found significantly related with advanced TNM stage and tumor stage. Besides, GRID1 could serve as an independent prognostic factor. Thus, future researches were required to explore the role of GRID1 in CRC.
Several shortcomings should be acknowledged in our study. The most obvious is that some inherent bias may be introduced by the retrospective nature. Second, the potential mechanisms between nine prognosis-related genes and CRC are not elucidated. Future studies are required to explore the underlying mechanisms. Finally, this autophagy-related gene signature is not validated in other CRC cohorts. Further researches are needed to ensure the robustness of our ARGs expression signature.
Conclusion
In summary, based on TCGA-CRC cohort, we develop an expression signature based on nine ARGs, which is an independent prognostic indicator for CRC prognosis. Additionally, this ARGs signature could stratify CRC patient outcome and outperform other clinicopathological parameters including TNM stage. These ARGs signature may help accelerate personalized treatment. However, future prospective researches are required to assess its clinical utility.
Acknowledgements
All authors thank the family for participating in the study. No sources of funding were required for this work.
Authors’ contributions
L.X. performed the statistical procedure and drafted the paper, Y.Q. helped edit the paper, and Q.Z. provided language help.
Disclosure of interest
The authors report no conflict of interest.
Data Availability Statement
Data sharing is not applicable to this article as no new data were created or analyzed in this study.
References
- Abu N, Othman N, Ab Razak NS, Bakarurraini N, Nasir SN, Soh JEC, Mazlan L, Azman ZAM, Jamal R. 2020. Extracellular vesicles derived from colorectal cancer affects CD8 T cells: An analysis based on body Mass index. Front Cell Dev Biol. 8:564648. doi:10.3389/fcell.2020.564648.
- Ait-Aissa K, Nguyen QM, Gabani M, Kassan A, Kumar S, Choi SK, Gonzalez AA, Khataei T, Sahyoun AM, Chen C, Kassan M. 2020. MicroRNAs and obesity-induced endothelial dysfunction: key paradigms in molecular therapy. Cardiovasc Diabetol. 19(1):136. doi:10.1186/s12933-020-01107-3.
- Al Bandar MH, Kim NK. 2017. Current status and future perspectives on treatment of liver metastasis in colorectal cancer (Review). Oncol Rep. 37(5):2553–2564. doi:10.3892/or.2017.5531.
- Amin MB, Greene FL, Edge SB, Compton CC, Gershenwald JE, Brookland RK, Meyer L, Gress DM, Byrd DR, Winchester DP. 2017. The Eighth edition AJCC cancer staging manual: Continuing to build a bridge from a population-based to a more “personalized” approach to cancer staging. CA Cancer J Clin. 67(2):93–99. doi:10.3322/caac.21388.
- An Y, Bi F, You Y, Liu X, Yang Q. 2018. Development of a novel autophagy-related prognostic signature for serous ovarian cancer. J Cancer. 9(21):4058–4071. doi:10.7150/jca.25587.
- Bagherniya M, Butler AE, Barreto GE, Sahebkar A. 2018. The effect of fasting or calorie restriction on autophagy induction: A review of the literature. Ageing Res Rev. 47:183–197. doi:10.1016/j.arr.2018.08.004.
- Chowdhury S, Pradhan RN, Sarkar RR. 2013. Structural and logical analysis of a comprehensive hedgehog signaling pathway to identify alternative drug targets for glioma, colon and pancreatic cancer. PLoS One. 8(7):e69132. doi:10.1371/journal.pone.0069132.
- Conroy MJ, Lysaght J. 2020. CX3CL1 signaling in the tumor microenvironment. Adv Exp Med Biol. 1231:1–12. doi:10.1007/978-3-030-36667-4_1.
- Cooks T, Pateras IS, Tarcic O, Solomon H, Schetter AJ, Wilder S, Lozano G, Pikarsky E, Forshew T, Rosenfeld N, et al. 2013. Mutant p53 prolongs NF-κB activation and promotes chronic inflammation and inflammation-associated colorectal cancer. Cancer Cell. 23(5):634–646. doi:10.1016/j.ccr.2013.03.022.
- Dekker E, Tanis PJ, Vleugels JLA, Kasi PM, Wallace MB. 2019. Colorectal cancer. Lancet. 394(10207):1467–1480. doi:10.1016/s0140-6736(19)32319-0.
- Fang Y, Ji L, Zhu C, Xiao Y, Zhang J, Lu J, Yin J, Wei L. 2020. Liraglutide alleviates hepatic steatosis by activating the TFEB-regulated autophagy-lysosomal pathway. Front Cell Dev Biol. 8:602574. doi:10.3389/fcell.2020.602574.
- Huang Z, Liu J, Luo L, Sheng P, Wang B, Zhang J, Peng SS. 2019. Genome-wide identification of a novel autophagy-related signature for colorectal cancer. Dose Response. 17(4):1559325819894179. doi:10.1177/1559325819894179.
- Khoo KH, Verma CS, Lane DP. 2014. Drugging the p53 pathway: Understanding the route to clinical efficacy. Nat Rev Drug Discov. 13(3):217–236. doi:10.1038/nrd4236.
- Levy JMM, Towers CG, Thorburn A. 2017. Targeting autophagy in cancer. Nat Rev Cancer. 17(9):528–542. doi:10.1038/nrc.2017.53.
- Li J, Guo BC, Sun LR, Wang JW, Fu XH, Zhang SZ, Poston G, Ding KF. 2014. TNM staging of colorectal cancer should be reconsidered by T stage weighting. World J Gastroenterol. 20(17):5104–5112. doi:10.3748/wjg.v20.i17.5104.
- Li M, Jin C, Xu M, Zhou L, Li D, Yin Y. 2017. Bifunctional enzyme ATIC promotes propagation of hepatocellular carcinoma by regulating AMPK-mTOR-S6 K1 signaling. Cell Commun Signal. 15(1):52. doi:10.1186/s12964-017-0208-8.
- Li T, Mu N, Yin Y, Yu L, Ma H. 2020. Targeting AMP-activated protein kinase in aging-related cardiovascular diseases. Aging Dis. 11(4):967–977. doi:10.14336/ad.2019.0901.
- Li J, Yi CH, Hu YT, Li JS, Yuan Y, Zhang SZ, Zheng S, Ding KF. 2016. TNM staging of colorectal cancer should be reconsidered according to weighting of the T stage: Verification based on a 25-year follow-up. Medicine (Baltimore. 95(6):e2711. doi:10.1097/md.0000000000002711.
- Li XL, Zhou J, Chen ZR, Chng WJ. 2015. P53 mutations in colorectal cancer - molecular pathogenesis and pharmacological reactivation. World J Gastroenterol. 21(1):84–93. doi:10.3748/wjg.v21.i1.84.
- Maloverjan A, Piirsoo M, Michelson P, Kogerman P, Osterlund T. 2010. Identification of a novel serine/threonine kinase ULK3 as a positive regulator of Hedgehog pathway. Exp Cell Res. 316(4):627–637. doi:10.1016/j.yexcr.2009.10.018.
- Mauri G, Sartore-Bianchi A, Russo AG, Marsoni S, Bardelli A, Siena S. 2019. Early-onset colorectal cancer in young individuals. Mol Oncol. 13(2):109–131. doi:10.1002/1878-0261.12417.
- Mo S, Dai W, Xiang W, Li Y, Feng Y, Zhang L, Li Q, Cai G. 2019. Prognostic and predictive value of an autophagy-related signature for early relapse in stages I-III colon cancer. Carcinogenesis. 40(7):861–870. doi:10.1093/carcin/bgz031.
- Mokarram P, Albokashy M, Zarghooni M, Moosavi MA, Sepehri Z, Chen QM, Hudecki A, Sargazi A, Alizadeh J, Moghadam AR, et al. 2017. New frontiers in the treatment of colorectal cancer: Autophagy and the unfolded protein response as promising targets. Autophagy. 13(5):781–819. doi:10.1080/15548627.2017.1290751.
- Packer M. 2020. Autophagy-dependent and -independent modulation of oxidative and organellar stress in the diabetic heart by glucose-lowering drugs. Cardiovasc Diabetol. 19(1):62. doi:10.1186/s12933-020-01041-4.
- Patel M, Horgan PG, McMillan DC, Edwards J. 2018. NF-κB pathways in the development and progression of colorectal cancer. Transl Res. 197:43–56. doi:10.1016/j.trsl.2018.02.002.
- Shi F, Li T, Liu Z, Qu K, Shi C, Li Y, Qin Q, Cheng L, Jin X, Yu T, et al. 2018. FOXO1: Another avenue for treating digestive malignancy? Semin Cancer Biol. 50:124–131. doi:10.1016/j.semcancer.2017.09.009.
- Steinmann S, Kunze P, Hampel C, Eckstein M, Bertram Bramsen J, Muenzner JK, Carlé B, Ndreshkjana B, Kemenes S, Gasparini P, et al. 2019. DAPK1 loss triggers tumor invasion in colorectal tumor cells. Cell Death Dis. 10(12):895. doi:10.1038/s41419-019-2122-z.
- Su Q, Wang Y, Zhao J, Ma C, Wu T, Jin T, Xu J. 2015. Polymorphisms of PRLHR and HSPA12A and risk of gastric and colorectal cancer in the Chinese Han population. BMC Gastroenterol. 15:107. doi:10.1186/s12876-015-0336-9.
- Wang SS, Chen G, Li SH, Pang JS, Cai KT, Yan HB, Huang ZG, He RQ. 2019. Identification and validation of an individualized autophagy-clinical prognostic index in bladder cancer patients. Onco Targets Ther. 12:3695–3712. doi:10.2147/ott.S197676.
- Wei R, Xiao Y, Song Y, Yuan H, Luo J, Xu W. 2019. FAT4 regulates the EMT and autophagy in colorectal cancer cells in part via the PI3K-AKT signaling axis. J Exp Clin Cancer Res. 38(1):112. doi:10.1186/s13046-019-1043-0.
- Xie J, Yan Y, Liu F, Kang H, Xu F, Xiao W, Wang H, Wang Y. 2019. Knockdown of Rab7a suppresses the proliferation, migration, and xenograft tumor growth of breast cancer cells. Biosci Rep. 39:2. doi:10.1042/bsr20180480.
- Xu JL, Yuan L, Tang YC, Xu ZY, Xu HD, Cheng XD, Qin JJ. 2020. The role of autophagy in gastric cancer chemoresistance: Friend or foe? Front Cell Dev Biol. 8:621428. doi:10.3389/fcell.2020.621428.
- Yu T, Guo F, Yu Y, Sun T, Ma D, Han J, Qian Y, Kryczek I, Sun D, Nagarsheth N, et al. 2017. Fusobacterium nucleatum promotes chemoresistance to colorectal cancer by modulating autophagy. Cell. 170(3):548–563. e516. doi:10.1016/j.cell.2017.07.008.
- Zhao YG, Zhang H. 2019. Core autophagy genes and human diseases. Curr Opin Cell Biol. 61:117–125. doi:10.1016/j.ceb.2019.08.003.
- Zhou X, Lin N, Zhang M, Wang X, An Y, Su Q, Du P, Li B, Chen H. 2020. Circulating soluble receptor for advanced glycation end products and other factors in type 2 diabetes patients with colorectal cancer. BMC Endocr Disord. 20(1):170. doi:10.1186/s12902-020-00647-9.