Abstract
We aimed to screen, via bioinformatics analyses, potential gene markers related to BC metastasis and prognosis. A gene expression dataset (GSE102818) and relevant clinical information were downloaded from the GEO database and TCGA, respectively. Then, differentially expressed genes (DEGs) were identified between primary and metastatic BC samples, followed by survival analyses. Subsequently, functional enrichment and protein–protein interaction (PPI) analyses were performed. Finally, transcriptional regulation analysis was performed to extract the pivotal genes. A total of 146 DEGs were screened. Of these, 56 downregulated DEGs were closely associated with the survival outcomes of BC. Furthermore, functional enrichment analysis showed that these survival-related genes, including CCR7, CD27, and IL2RB, played notable roles in pathways such as cytokine-cytokine receptor interaction and immune responses. Furthermore, PPI network and enriched sub-module network analyses revealed that CCR7, CD27, and IL2RB served as hub genes with a high degree of association. Accordingly, they were key players in BC-associated transcriptional regulatory networks. Notably, these genes exhibited strong relationships with miR-1908 and miR-663. CCR7, CD27, IL2RB, miR-1908, and miR-663 may participate in the molecular mechanisms underlying BC metastasis, probably by influencing cytokine-related pathways and immune responses; thus, they are potential, novel therapeutic targets for BC management.
Introduction
Breast cancer (BC), one of the most common malignant diseases, is a leading cause of mortality among women globally, especially, in developing nations (Bonilla et al. Citation2017). Investigators report that the incidence of BC will continuously increase and reach a value of approximately 85 in 100,000 women by 2021 (Akram et al. Citation2017). Notably, a large number of BC patients suffer from metastasis and recurrence (Siegel et al. Citation2017). Fortunately, advanced diagnostic techniques, sophisticated surgical operations, and improved pharmacological treatments, have considerably enhanced the survival rate of BC patients over the past few years (Peart Citation2015; Castaneda and Strasser Citation2017). Recently, multiple studies have focused on clarifying the molecular mechanisms underlying the etiology of diseases using a wide range of analytical approaches, including conventional experimental techniques, association analysis, microarrays, and high-throughput sequencing (Selvaraj et al. Citation2021) (Nangraj et al. Citation2020) (Huang et al. Citation2020). There have also been many reports on the molecular mechanism of BC (Marotti et al. Citation2017). For example, Segovia-Mendoza et al identified numerous components in immune cells that are involved in the breast tumor microenvironment and contribute to the development of BC (Segovia-Mendoza and Morales-Montor Citation2019). Moreover, Fatima et al identified a 32-bp deletion in chemokine CC receptor 5 that is closely correlated with the risk of BC and claimed that this signal may be responsible for the activation and invasion of immune cells at the site of tumorigenesis (Fatima et al. Citation2019). Qi et al suggested that some vital genes (CD27 and CR2) and pathways, such as immune responses, are possibly involved in the pathogenesis of triple-negative BC, according to microarray analysis data (Qi et al. Citation2019). Yang et al also identified several potential genetic markers (CCNB2, BUB1, and NDC80) associated with the occurrence and progression of basal-like BC using bioinformatics analyses based on two microarrays (Chen et al. Citation2019).
Additionally, the invasion and metastasis of BC has been reported to appear in a very short time-frame after the occurrence of the primary tumor (Weigelt et al. Citation2005). Using cDNA microarray analysis, Li et al previously found that AF1Q may be a potential genetic marker for predicting BC metastasis. Zheng et al identified several differentially expressed genes (DEGs) between normal and invasive ductal carcinoma breast samples and found that multiple genes, such as CAPG, TP53INP1, and SPI1 are possibly associated with BC metastasis (Zheng et al. Citation2017). Although existing evidence has explored the potential molecular markers for the development and metastasis of BC, there is a need for a deeper understanding of the mechanisms underlying, and progression of, BC.
In this study, the dataset GSE102818 from the Gene Expression Omnibus (GEO) was downloaded, including the gene expression data from primary tumors and metastatic lesions of BC cases. Next, the DEGs between primary and metastatic patients were identified. Meanwhile, the relevant clinical data from The Cancer Genome Atlas (TCGA) were also obtained to screen for survival-associated genes. Moreover, functional annotation, protein interaction, and transcriptional regulatory analyses of these survival-related genes were also performed. This work will contribute towards the screening of promising novel therapeutic targets for controlling the development and metastasis of BC and facilitate the development of new strategies to improve the prognosis of BC patients.
Materials and methods
Data acquisition
Gene expression profile data (from the GSE102818 dataset) were retrieved and downloaded from the National Center for Biotechnology Information GEO (Barrett et al. Citation2007) repository (http://www.ncbi.nlm.nih.gov/geo/). The GSE102818 dataset contains 31 primary BC samples and 17 corresponding metastatic BC samples. These 48 samples corresponded to 36 patients (10 ER-negative and 26 ER-positive), some of which came from paired primary and metastatic tissues of 10 patients (for one patient, tissues from two different metastases were obtained, and for another, two different biopsies from one primary tumor was performed). All samples were subjected to analysis using the GPL23249 Nanostring nCounter Gene Expression Assay (Pan Cancer Immune Panel). In addition, the RNA sequencing data of BC specimens and the relevant clinical data were obtained from the TCGA database, which included 1,212 BC samples. After sample examination, survival data from 1,100 BC samples were successfully obtained.
Data pre-processing and identification of dEgs
The raw microarray data was pre-processed using the scale method in R software. The affy package in R was used to normalize the data, which included format conversion, supplementing missing values, analyzing the background correction, and log2 transformation. The Limma package and t-test in R were used to extract the DEGs the between primary and metastatic BC samples. Moreover, all p values were computed and subsequently adjusted (adj.p.value) using the Benjamini & Hochberg method. Of note, the DEGs in this research were defined based on the following thresholds: adj.p.value < 0.05 and | log fold change (FC) | > 1.
Survival analysis of DEGs
TCGA has effectively enhanced the prevention and diagnosis of a wide variety of cancers, owing to its abundance of genetic and clinical data. Here, the clinical information of about 1,100 BC patients, including overall survival (OS) and OS status, was collected, and the screened DEGs were further matched with relevant clinicopathologic data. Briefly, the expression values of these DEGs were calculated, and accordingly, the specimens were divided into the high-expression and low-expression groups. Subsequently, a Log-rank test was implemented (p value < 0.05) to identify the DEGs related to the survival of BC patients. Finally, the survival curve was obtained using the Kaplan–Meier method.
Gene ontology (GO) and pathway enrichment analyses
The Database for Annotation, Visualization and Integrated Discovery (DAVID) (Huang et al. Citation2009), a free web-accessible bioinformatics resource, provided integrated functional annotation tool suites. Therefore, for candidate DEGs associated with BC survival, GO (Ashburner et al. Citation2000) functional annotation and Kyoto Encyclopedia of Genes and Genomes (KEGG) (Kanehisa and Goto Citation2000) pathway enrichment analyses were performed using DAVID. A p value < 0.05 and count > 2 were determined as the cutoffs for the functional analyses.
Protein–protein interaction (PPI) network construction and sub-module analysis
The Search Tool for the Retrieval of Interacting Genes/Proteins (STRING) (Szklarczyk et al. Citation2015) database is publicly available and has a powerful PPI-predictive ability. To further explore the interactions among the identified DEGs correlated with BC survival, we performed PPI analysis using a PPI score of 0.4, based on the STRING database. Next, PPI networks were constructed and visualized using Cytoscape (Shannon et al. Citation2003) software (v 3.6.1, http://www.cytoscape.org/). Furthermore, the MCODE (Bandettini et al. Citation2012) plugin in the CytoNCA software (v 1.4.2, http://apps.cytoscape.org/apps/MCODE) was utilized to analyze the topological characteristics of significantly enriched modules using the following criterion: PPI score parameter > 10.
miRNA-TF-target regulatory analysis
To gain a deeper understanding of the potential functions of the candidate DEGs, the ENCODE chip-seq dataset in the Enrichr (Chen et al. Citation2013) (http://amp.pharm.mssm.edu/Ehrichr/) database was used to predict the transcription factors (TFs) regulated by DEGs in the PPI module with the cutoff of p value < 0.05. Meanwhile, the miRTarBase dataset in the Enrichr database was used to predict miRNA-DEG pairs with a p value < 0.05 (used as the screening threshold). Finally, miRNA-TF-target regulatory networks were constructed using Cytoscape by integrating the TF-DEG interactive pairs with the miRNA-DEG interactions.
Results
Identification of dEgs and survival analysis
In total, 146 DEGs, including three significantly upregulated genes and 143 markedly downregulated genes, were extracted using the methods outlined above (Figure ). Subsequently, these DEGs were subjected to survival analysis by matching them with the 1,100 BC samples from TCGA. Our results showed that 145 DEGs were further extracted via integration with relevant clinical information (Table S1). Moreover, a total of 56 downregulated DEGs were found to be correlated with BC survival characteristics, while three upregulated DEGs were not associated with BC prognosis, as shown in the Table S2.
Functional enrichment analysis of DEGs
The GO enrichment analysis indicated that downregulated DEGs, such as CD27, were markedly enriched in 75 GO terms correlated with biological processes such as immune response, 19 GO terms involved with molecular functions including transmembrane signaling receptor activity, and 14 GO terms related to cellular components that mainly focused on the plasma membrane (Figure ). Additionally, the KEGG pathway analysis demonstrated that these downregulated DEGs, including CD27, C–C-chemokine receptor (CCR7), and interleukin 2 receptor- beta (IL2RB), played essential roles in cytokine-cytokine receptor interactions and the T cell receptor signaling pathway (Figure ).
PPI network analysis
PPI network analysis was performed using the STRING database to explore the potential PPI relationships of DEGs with BC survival. A total of 56 nodes and 518 protein pairs were obtained (Figure A). The top 15 gene nodes are shown in Table ; these were considered hub genes with a high degree of association. Subsequently, we extracted a significantly enriched sub-module using MCODE with a score ≥ 10; this sub-module included 22 nodes and 206 interactive pairs (Figure B).
Figure 3. The protein-protein interaction (PPI) network and sub-module network of survival-related genes. (A) PPI network; (B) significantly enriched sub-module network. The green circular nodes represent the downregulated genes.
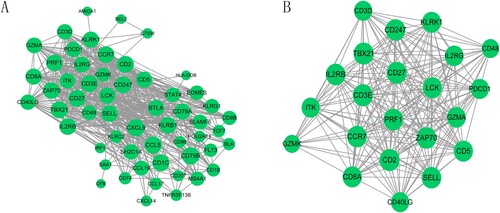
Table 1. Top 15 hub genes in protein-protein interaction and sub-module network.
miRNA- TF-target regulatory network analysis
According to the prediction analysis based on the Enrichr database, 31 miRNA-target pairs and four TF-target pairs were predicted and subsequently used to construct the TF-miRNA-target regulatory network. Moreover, the hub genes, including CCR7, CD27, and IL2RB, showed close interactions with miR-663 and miR-1908 (Table ). Our findings also suggested that 35 miRNA-TF-target regulative pairs were achieved, containing 14 significant DEGs, six miRNAs (miR-3677, miR-663, miR-1908, miR-1296, miR-3193, and miR-492), and one TF (BCL11A_GM12878_hg19) (Figure ).
Figure 4. Transcriptional regulatory network. The green circles represent the downregulated genes; the purple triangles represent the miRNAs; and the yellow hexagons represent the transcription factors.
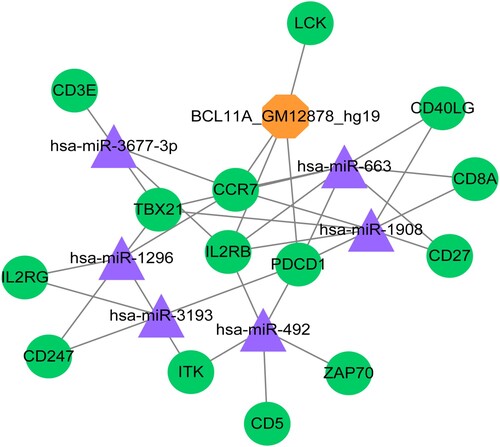
Table 2. The interaction pairs among miRNAs, transcription factors and genes.
Discussion
BC has been an international health concern due to its relatively high metastasis and recurrence rate. Despite considerable advancements in the early detection and clinical management of this condition, it remains a feared and refractory ailment; BC is the leading cause of tumor-related deaths among women. Amjad et al. discovered that a 53-mRNA signature may be a viable gene set for identifying TNM stages, as well as formulating prospective treatments; this signature may show a high performance in predicting and controlling recurrence-free survival rates at stages I, II, III, and IV, as well as in groups of patients at all stages (Amjad et al. Citation2020). Liu et al. found that TF-related signatures can independently predict the prognosis of BC patients without considering clinical variables (Liu et al. Citation2021). However, the molecular mechanism underlying BC metastasis has not been fully understood; this hinders the improvement of therapeutic interventions for BC. Therefore, in our study, comprehensive bioinformatics analyses were performed using data from TCGA and GEO datasets to screen promising new therapeutic targets to manage the development and metastasis of BC.
In this study, we identified 146 DEGs, including three upregulated DEGs and 143 downregulated DEGs, between primary BC and metastatic BC samples. Moreover, 56 DEGs were closely correlated with BC survival outcomes. Functional enrichment analysis showed that several genes, including CCR7, CD27, and IL2RB, were markedly enriched in pathways such as cytokine-cytokine receptor interaction and immune responses. Moreover, the PPI network and sub-module analyses demonstrated that downregulated genes (CCR7, CD27, and IL2RB) acted as hub genes with higher node degrees. Of note, based on the regulatory analysis, miR-1908 and miR-663 strongly interacted with these three genes.
CCR7 has been generally recognized to be responsible for tumor proliferation, migration, and invasion (Xu et al. Citation2017). Recently, a dearth of evidence has also confirmed that CCR7 is implicated with the occurrence, metastasis, and invasion of BC (Mishan et al. Citation2015; Sonbul et al. Citation2017). For example, Strien et al examined the CCR7 expression levels in different BC cells and observed that CCR7 is expressed in the overwhelming majority of primary BC tumors but is more closely related to aggressive tumors (Strien et al. Citation2017). Vehedi et al also highlighted that CCR7 shows a correlation with the stage and grade of BC (Vahedi et al. Citation2018). More interestingly, emerging research has demonstrated that CCR7 is predominately associated with BC prognosis and survival outcomes (Cassier et al. Citation2011). Herein, we found that this gene was downregulated and validated that it was a survival-related gene in BC patients. Moreover, functional analysis revealed that CCR7 primarily participated in cytokine-cytokine receptor interaction pathways. In addition, according to our network analysis, CCR7 had strong relationships with miR-663 and miR-1908. Rawlings-Goss et al previously showed that miR-1908 is a crucial regulator involved in the onset and development of tumor metastasis, indirectly implying that the CCR7/miR-1908 axis possibly participates in the metastasis of BC (Rawlings-Goss et al. Citation2014). Similarly, multiple investigators have argued that miR-663 plays significant roles in different cancers, such as colorectal cancer and prostate carcinoma (Tsikitis et al. Citation2016; Wang et al. Citation2017). Taken together, we inferred that CCR7 can be considered a promising mediator of BC metastasis and prognosis.
CD27, a member of the tumor necrosis factor family, can stimulate cell apoptosis and modulate immune lymphocyte function. Via an association analysis, Xu et al showed that the aberrant expression of CD27 could disrupt immune responses and influence BC risk (Xu et al. Citation2012). Our findings demonstrated that CD27, as a notable BC survival-associated gene, also played a prominent node in the PPI and sub-module networks, showing a high degree. Furthermore, functional enrichment analyses revealed that CD27 was significantly enriched in cytokine-cytokine receptor interaction pathways and immune responses. Accumulating evidence has implied that cytokines are mainly produced by immune cells and are engaged in intra- and intercellular communication (Gravestein and Borst Citation1998). Numerous researchers have pointed out that cytokines and their corresponding receptors participate in the metastasis of various cancers, including BC (Méndez-García et al. Citation2019); this suggests that CD27 may be involved in BC metastasis. Notably, a growing body of research has emphasized that investigating the crosstalk between the molecular footmarks of BC and the immune system serves as a promising therapeutic approach for BC. In addition, in the present study, strong interactions between CD27 and miR-1980/miR-663 were observed. Therefore, we speculated that CD27 is closely correlated with the metastasis and prognosis of BC.
IL2RB is considered a potential marker for the susceptibility to various cancers, such as lung cancer and BC (Marra et al. Citation2014; Ho et al. Citation2018). A previous study indicated that IL2RB is broadly expressed in the majority of BC cells (Ridings et al. Citation1995). Later, numerous investigators demonstrated that a higher expression of IL2RB is related to the progression of BC tumors, and more importantly, this alteration is possibly involved in the malignancy of BC tumors (García-Tuñón et al. Citation2004). Furthermore, Hong et al previously stated that the variants in IL2RB are associated with BC risk and this variant was mainly responsible for cytokine-correlated pathways (Hong et al. Citation2018). Consistently, our results suggested that IL2RB was significantly enriched in the cytokine-cytokine receptor interaction pathway. Additionally, we performed a survival analysis and found that this gene was predominately linked with the survival outcomes of BC, indicating that IL2RB may serve as a vital prognostic gene with regard to BC treatment. More intriguingly, we also observed that IL2RB closely interacted with two pivotal regulators (miR-1908 and miR-663) that were reported to be implicated with tumor progression. Thus, our results provided a novel avenue to improve the understanding of the potential molecular mechanisms underlying the role of IL2RB in BC development.
Although several prominent gene targets were screened using bioinformatics methods, our investigation had a few limitations. Firstly, according to the screening criteria, we only screened the GSE102818 data set. Therefore, more rigorous studies are required to verify our findings; these studies should primarily involve exhaustive bioinformatics analyses with a larger sample size and strong experimental evidence. Secondly, we found strong interactions between several key genes and specific signaling pathway; this may represent a crucial therapeutic strategy for BC. However, the mechanisms underlying these interactions and their roles remain to be elucidated. In addition, comprehensive clinical data should also be integrated further to evaluate and validate the prognosis of BC patients.
Conclusion
In summary, we identified vital genetic markers, such as CCR7, CD27, and IL2RB, which were correlated with the mechanisms underlying the metastasis of BC. Notably, we found that these genes were possibly associated with the survival rate of BC patients. Meanwhile, numerous pathways, including cytokine-related pathways, were also observed to play essential roles in the initiation and progression of BC. Additionally, miR-1908 and miR-663 were found to closely interact with these three genes. Thus, our findings may provide novel insights to formulate effective therapeutic interventions for the prevention and treatment of BC. However, further experimental confirmations and integrated bioinformatics analyses are required to validate the findings of the present study.
Data availability
The data that support the findings of this study are publicly available in the GEO database (https://www.ncbi.nlm.nih.gov/geo/) under the accession number GSE102818.
Disclosure statement
No potential conflict of interest was reported by the author(s).
References
- Akram M, Iqbal M, Daniyal M, Khan AU. 2017. Awareness and current knowledge of breast cancer. Biol Res. 50:33.
- Amjad E, Asnaashari S, Sokouti B, Dastmalchi S. 2020. Systems biology comprehensive analysis on breast cancer for identification of key gene modules and genes associated with TNM-based clinical stages. Sci Rep. 10:10816.
- Ashburner M, Ball CA, Blake JA, Botstein D, Butler H, Cherry JM, Davis AP, Dolinski K, Dwight SS, Eppig JT, Harris MA. 2000. Gene ontology: tool for the unification of biology. The Gene Ontology Consortium. Nat Genet. 25:25–29.
- Bandettini WP, Kellman P, Mancini C, Booker OJ, Vasu S, Leung SW, Wilson JR, Shanbhag SM, Chen MY, Arai AE. 2012. MultiContrast Delayed Enhancement (MCODE) improves detection of subendocardial myocardial infarction by late gadolinium enhancement cardiovascular magnetic resonance: a clinical validation study. J Cardiovasc Magn Reson. 14(1):1–10.
- Barrett T, Troup DB, Wilhite SE, Ledoux P, Rudnev D, Evangelista C, Kim IF, Soboleva A, Tomashevsky M, Edgar R. 2007. NCBI GEO: mining tens of millions of expression profiles–database and tools update. Nucleic Acids Res. 35(suppl_1):D760–D765.
- Bonilla JM, Tabanera MT, Mendoza LR. 2017. Breast cancer in the 21st century: from early detection to new therapies. Radiologia. 59:368–379.
- Cassier PA, Treilleux I, Bachelot T, Ray-Coquard I, Bendriss-Vermare N, Menetrier-Caux C, Tredan O, Goddard-Leon S, Pin JJ, Mignotte H, et al. 2011. Prognostic value of the expression of C-Chemokine Receptor 6 and 7 and their ligands in non-metastatic breast cancer. BMC cancer. 11:213.
- Castaneda SA, Strasser J. 2017. Updates in the Treatment of Breast Cancer with Radiotherapy. Surg Oncol Clin N Am. 26:371–382.
- Chen B, Xia Z, Deng YN, Yang Y, Zhang P, Zhu H, Xu N, Liang S. 2019. Emerging microRNA biomarkers for colorectal cancer diagnosis and prognosis. Open Biol. 9:180212.
- Chen EY, Tan CM, Kou Y, Duan Q, Wang Z, Meirelles GV, Clark NR, Ma’ayan A. 2013. Enrichr: interactive and collaborative HTML5 gene list enrichment analysis tool. BMC Bioinformatics. 14(1):1–14.
- Fatima F, Saleem S, Hameed A, Haider G, Zaidi SAA, Kanwal M, Zehra S, Azhar A. 2019. Association analysis and allelic distribution of deletion in CC chemokine receptor 5 gene (CCR5Δ32) among breast cancer patients of Pakistan. Mol Biol Rep. 46(2).
- García-Tuñón I, Ricote M, Ruiz A, Fraile B, Paniagua R, Royuela M. 2004. Interleukin-2 and its receptor complex (alpha, beta and gamma chains) in in situ and infiltrative human breast cancer: an immunohistochemical comparative study. Breast Cancer Res. 6(1):R1–R7.
- Gravestein LA, Borst J. 1998. Tumor necrosis factor receptor family members in the immune system. Semin Immunol. 10:423–434.
- Ho KH, Chang CJ, Huang TW, Shih CM, Liu AJ, Chen PH, Cheng KT, Chen KC. 2018. Gene landscape and correlation between B-cell infiltration and programmed death ligand 1 expression in lung adenocarcinoma patients from The Cancer Genome Atlas data set. PLoS One. 13:e0208459.
- Hong C-C, Sucheston-Campbell LE, Liu S, Hu Q, Yao S, Lunetta KL, Haddad SA, Ruiz-Narváez EA, Bensen JT, Cheng T-YD, et al. 2018. Genetic Variants in Immune-Related Pathways and Breast Cancer Risk in African American Women in the AMBER Consortium. Cancer Epidemiol Biomark Prev. 27:321–330.
- Huang DW, Sherman BT, Lempicki RA. 2009. Systematic and integrative analysis of large gene lists using DAVID bioinformatics resources. Nat Protoc. 4:44–57.
- Huang S, Wei YK, Kaliamurthi S, Cao Y, Nangraj AS, Sui X, Chu D, Wang H, Wei DQ, Peslherbe GH, et al. 2020. Circulating miR-1246 Targeting UBE2C, TNNI3, TRAIP, UCHL1 Genes and Key Pathways as a Potential Biomarker for Lung Adenocarcinoma: Integrated Biological Network Analysis. J Pers Med. 10:162-162.
- Kanehisa M, Goto S. 2000. KEGG: kyoto encyclopedia of genes and genomes. Nucleic Acids Res. 28:27–30.
- Liu J, Liu Z, Zhou Y, Zeng M, Pan S, Liu H, Liu Q, Zhu H. 2021. Identification of a Novel Transcription Factor Prognostic Index for Breast Cancer. Front Oncol. 11:666505.
- Marotti JD, de Abreu FB, Wells WA, Tsongalis GJ. 2017. Triple-Negative Breast Cancer: Next-Generation Sequencing for Target Identification. Am J Pathol. 187:2133–2138.
- Marra P, Mathew S, Grigoriadis A, Wu Y, Kyle-Cezar F, Watkins J, Rashid M, De Rinaldis E, Hessey S, Gazinska P, et al. 2014. IL15RA drives antagonistic mechanisms of cancer development and immune control in lymphocyte-enriched triple-negative breast cancers. Cancer Res. 74:4908–4921.
- Méndez-García LA, Nava-Castro KE, Ochoa-Mercado TDL, Palacios-Arreola MI, Ruiz-Manzano RA, Segovia-Mendoza M, Solleiro-Villavicencio H, Cázarez-Martínez C, Morales-Montor J. 2019. Breast Cancer Metastasis: Are Cytokines Important Players During Its Development and Progression? J Interferon Cytokine Res. 39:39–55.
- Mishan MA, Heirani-Tabasi A, Mokhberian N, Hassanzade M, Moghaddam HK, Bahrami AR, Ahmadiankia N. 2015. Analysis of Chemokine Receptor Gene Expression in Esophageal Cancer Cells Compared with Breast Cancer with Insights into Metastasis. Iran J Public Health. 44:1353–1358.
- Nangraj AS, Selvaraj G, Kaliamurthi S, Kaushik AC, Cho WC, Wei DQ. 2020. Integrated PPI- and WGCNA-Retrieval of Hub Gene Signatures Shared Between Barrett's Esophagus and Esophageal Adenocarcinoma. Front Pharmacol. 11:881.
- Peart O. 2015. Breast intervention and breast cancer treatment options. Radiol Technol. 86:535M–558M.
- Qi F, Qin WX, Zang YS. 2019. Molecular mechanism of triple-negative breast cancer-associated BRCA1 and the identification of signaling pathways. Oncol Lett. 17:2905–2914.
- Rawlings-Goss RA, Campbell MC, Tishkoff SA. 2014. Global population-specific variation in miRNA associated with cancer risk and clinical biomarkers. BMC Med Genomics. 7. doi:10.1186/1755-8794-1187-1153.
- Ridings J, Macardle PJ, Byard RW, Skinner J, Zola H. 1995. Cytokine receptor expression by solid tumours. Ther Immunol. 2:67–76.
- Segovia-Mendoza M, Morales-Montor J. 2019. Immune Tumor Microenvironment in Breast Cancer and the Participation of Estrogens and Its Receptors Into Cancer Physiopathology. Front Immunol. 10. doi:10.3389/fimmu.2019.00348.
- Selvaraj G, Kaliamurthi S, Peslherbe GH, Wei DQ. 2021. Identifying potential drug targets and candidate drugs for COVID-19: biological networks and structural modeling approaches. F1000Res. 10:127.
- Shannon P, Markiel A, Ozier O, Baliga NS, Wang JT, Ramage D, Amin N, Schwikowski B, Ideker T. 2003. Cytoscape: a software environment for integrated models of biomolecular interaction networks. Genome Res. 13:2498–2504.
- Siegel RL, Miller KD, Fedewa SA, Ahnen DJ, Meester RG, Barzi A, Jemal A. 2017. Cancer Statistics, 2017. CA Cancer J Clin. 67:7–30.
- Sonbul SN, Gorringe KL, Aleskandarany MA, Mukherjee A, Green AR, Ellis IO, Rakha EA. 2017. Chemokine (C-C motif) receptor 7 (CCR7) associates with the tumour immune microenvironment but not progression in invasive breast carcinoma. J Pathol Clin Res. 3:105–114.
- Strien L, Joensuu K, Heikkilä P, Leidenius MH. 2017. Different Expression Patterns of CXCR4, CCR7, Maspin and FOXP3 in Luminal Breast Cancers and Their Sentinel Node Metastases. Anticancer Res. 37:175–182.
- Szklarczyk D, Franceschini A, Wyder S, Forslund K, Heller D, Huerta-Cepas J, Simonovic M, Roth A, Santos A, Tsafou KP, Kuhn M. 2015. STRING v10: protein-protein interaction networks, integrated over the tree of life. Nucleic Acids Res. 43:D447–D452.
- Tsikitis VL, Potter A, Mori M, Buckmeier JA, Preece CR, Harrington CA, Bartley AN, Bhattacharyya AK, Hamilton SR, Lance MP, Thompson PA. 2016. MicroRNA Signatures of Colonic Polyps on Screening and Histology. Cancer Prev Res (Phila). 9:942–949.
- Vahedi L, Ghasemi M, Yazdani J, Ranjbar S, Nouri B, Alizadeh A, Afshar P. 2018. Investigation of CCR7 Marker Expression Using Immunohistochemical Method and Its Association with Clinicopathologic Properties in Patients with Breast Cancer. Int J Hematol Oncol Stem Cell Res. 12:103–110.
- Wang S, Liu J, Li C, Yang X. 2017. Research of the effect of miR-663 on the proliferation of prostate cancer cells, and the correlations of miR-663 with pathological grade and clinical stage. J BUON. 22:1011–1016.
- Weigelt B, Peterse JL, Van't Veer LJ. 2005. Breast cancer metastasis: markers and models. Nat Rev Cancer. 5:591–602.
- Xu B, Zhou M, Qiu W, Ye J, Feng Q. 2017. CCR7 mediates human breast cancer cell invasion, migration by inducing epithelial-mesenchymal transition and suppressing apoptosis through AKT pathway. Cancer Med. 6:1062–1071.
- Xu F, Li D, Zhang Q, Fu Z, Yuan W, Pang D, Li D. 2012. Association of CD27 and CD70 gene polymorphisms with risk of sporadic breast cancer in Chinese women in Heilongjiang Province. Breast Cancer Res Treat. 133:1105–1113.
- Zheng T, Wang A, Hu D, Wang Y. 2017. Molecular mechanisms of breast cancer metastasis by gene expression profile analysis. Mol Med Rep. 16:4671–4677.