Abstract
Kidney renal clear cell carcinoma (KIRC) is the predominant pathological subtype of kidney cancer and is categorized as immunotherapy responsive. Hence, immunotherapy has become a worthwhile therapy for KIRC. Furthermore, tumor mutation burden (TMB) has been regarded as the most prevalent biomarker to predict immunotherapy response. Accordingly, we spent the effort to characterize the status and the predictive potential for immunotherapy response of gene mutations in KIRC. In this study, we identified common somatic mutations in KIRC patients from The Cancer Genome Atlas (TCGA), International Cancer Genome Consortium (ICGC), and UTokyo cohorts in cBioportal database. BRCA1-related protein 1 (BAP1) was identified as the only common gene mutation related to TMB and overall survival. We finally explored whether mutation of BAP1 was related to immune response and immune infiltration. In brief, we identified and demonstrated that BAP1 mutations commonly occurred in KIRC patients, associated with lower TMB, and indicating a poorer prognosis. Furthermore, BAP1 mutation reversed its function as a tumor suppressor via influencing Mast cells’ quantity. These findings cast light on the predictive value of BAP1 to evaluate immunotherapeutic sensitivity and presented a potential target for KIRC treatment.
Introduction
Kidney cancer is the most common urogenital tumor accounting for approximately 90% of all diagnosed renal parenchymal malignancies and comprises approximately 3% of all adult malignant diseases claiming 14,830 lives with 73,750 new confirmed cases in the USA in 2020 (Motzer et al. Citation2017; Siegel et al. Citation2019). In addition, approximately 30% of renal cell cancer (RCC) patients have metastatic disease at the time of initial diagnosis and up to 40% of the patients will relapse after complete resection of the primary tumor (Rajandram et al. Citation2012), which means a considerable medical burden (Vugrin Citation1987).
Kidney renal clear cell carcinoma (KIRC) is the predominant pathological subtype of kidney cancer accounting for approximately 85% of renal cancer (Barata and Rini Citation2017), which is considered also one of the most aggressive (Vugrin Citation1987), associated with high mortality in its metastatic form (Motzer et al. Citation2008). Although surgical interventions remain the main treatment approach hormone and cytotoxic therapies (Correa and Brown Citation2019), tyrosine kinase inhibitors (TKIs) sunitinib and pazopanib targeting vascular endothelial growth factor (VEGF) pathway, and inhibitors of the mechanistic target of rapamycin (mTOR) also play a significant role in current clinical therapy (Kowalewski et al. Citation2020). More recently, immune checkpoint inhibitors (ICIs) that block PD-1/PD-L1 or CTLA-4T-cell suppressor inhibitory have shown effectiveness in this disease, especially with the combination of VEGF-directed therapy (Motzer et al. Citation2019).
Immunotherapy has unfolded a new page for anti-cancer therapy, and KIRC has long been categorized as immunotherapy responsive (Vugrin Citation1987). However, the therapeutic methods applied in KIRC are not very effective, and the efficacy of targeted drugs varies in the patients of KIRC (Méjean et al. Citation2018). Therefore, getting a comprehensive understanding of the molecular determinants of immunotherapeutic responsiveness becomes a key challenge in KIRC. A series of next-generation sequencing studies (NGS) led to a better understanding of the genomic landscape of tumorigenesis (Salk et al. Citation2018), and recent studies have proved the potential of tumor mutational burden (TMB) to predict immunotherapy response and prognosis (Ricciuti et al. Citation2019; Pinato et al. Citation2020). Hence, we conducted this research to explore the gene mutation characteristics in KIRC and to establish the association between the mutation and the clinical prognosis. Furthermore, we hoped to unearth the correlation between the mutation and molecular features and potential therapeutic target for KIRC.
In this study, first we identified somatic mutations in KIRC patients from The Cancer Genome Atlas (TCGA), International Cancer Genome Consortium (ICGC), and UTokyo cohort from the cBioportal database. Second, we found nine common mutation genes in these three data sets and investigated the relationship between these gene mutations and TMB and prognosis. Finally, we explored whether these gene mutations were related to immune response. We identified and demonstrated BAP1 mutation as a new biomarker for TMB and a potential therapeutic target for KIRC patients.
Materials and methods
Data acquisition
Somatic gene mutations for American KIRC samples (n = 336), Chinese KIRC samples (n = 400) and Japanese KIRC samples (n = 106) were, respectively, acquired from TCGA-portal (http://portal.gdc.cancer.gov/projects) (up to December 10, 2020), ICGC-portal (http://dcc.icgc.org/ releases/current/Projects) (up to December 10, 2020) and cBioPortal (https://www.cbioportal.org/study/summary?id = ccrcc_utokyo_2013). Clinical data for 336 KIRC samples were downloaded from TCGA. For clinical data, only patients with complete information (n = 336) were included, and those lack of detailed information concerning survival time, status, age, gender, grade or TNM classification were excluded.
TMB in KIRC
TMB was defined as a value equal to the number of somatic, coding, indels mutations and base substitution per megabase of genome examined. Among them, all the base substitutions and idels in the coding region of targeted genes were counted. Notably, silent mutations failing to contribute to an amino acid change were not included. To analyze the TMB of each sample, the total number of mutations counted was divided by the size of exome (38 megabase (Mb) was distinguished as the estimate of the exome size) (Chalmers et al. Citation2017).
Bioinformatic analysis
MAF files containing somatic variants for American KIRC samples were detected using VarScan and visualized with maftools package (Mayakonda et al. Citation2018). TSV files containing somatic variants for Chinese KIRC samples were annotated according to hg19 reference genome and visualized with GenVisR package. The transcriptome profile was retrieved from TCGA-GDC. CIBERSORT (Newman et al. Citation2015), a deconvolution algorithm which could evaluate the proportions of 22 tumor-infiltrating lymphocyte subtypes in a bulk tumor sample according to the transcriptome profiles was used to estimate the relative abundance of immune cell infiltration in patients with different BAP1 mutation status. Only the results with p < 0.05 were included for further inter-group differential infiltration analysis.
Statistical analysis
All the statistical analysis and plotting were carried out by R software (version 4.0.2), and the data cleaning was processed by the Strawberry PERL programming language (Version 5.30.2.1). Kaplan-Meier methods survival curves and log-rank test survival analysis were used to investigate whether the mutation could influence the prognosis. Both univariate and multivariate Cox regression were performed to explore the independent prognostic value of clinical characteristics including age, gender, grade, stage, TMB and BAP1 mutation status. Mann–Whitney U test was applied to explore the correlation between mutation status and TMB. Besides, a two-tailed p-value <0.05 for all comparisons was considered statistically significant.
Results
Illustration of somatic mutations in KIRC
We defined 30 of the most frequently mutated genes in American KIRC samples from the TCGA cohort, and the five most frequently mutated genes were VHL, PBRM1, TTN, SETD2 and BAP1 (Figure (A)). We also analyzed the interaction of these genes finding that SETD2 and PBRM1, TTN and ANK3, SPEN and PTEN, MUC16 and BAP1, LRP2 and PBRM1, VHL and PBRM1, CSMD3 and MTOR mutations tended to co-occur (Figure (B)). Then, we made a summary of these mutated genes, showing that missense mutation and SNP were the most common variant types with 41 as the median of the number of variants per sample. (Figure (C)) We defined 30 frequently mutated genes in Chinese KIRC samples from the ICGC cohort, and the five most frequently mutated genes were VHL, PBRM1, SETD2, BAP1, and DKM5C (Figure (A)). And, the data from the UTokyo cohort indicated that the five most frequently mutated genes were VHL, PBRM1, SETD2, BAP1 and MTOR.
Figure 1. Illustration of somatic mutations in KIRC from TCGA cohort. (A) Waterfall plot illustrates the frequently mutated genes in KIRC from TCGA cohort. The top panel shows mutation frequency. The bottom panel presents mutation types. (B) Interaction plot of the mutated genes from TCGA cohort (C) A summary of the mutated genes in KIRC from TCGA cohort.
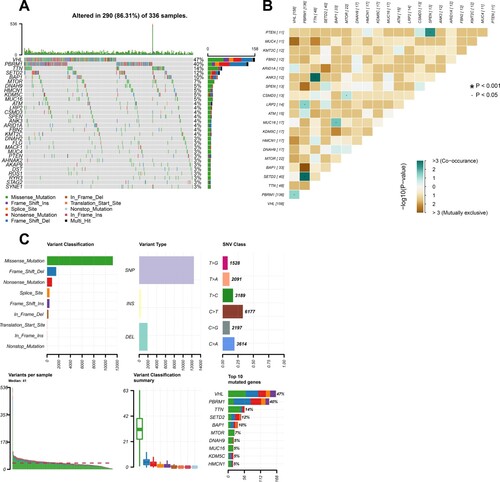
Figure 2. Illustration of somatic mutations in KIRC from ICGC cohort and Venn diagram of frequently mutated genes covered by all the three cohorts. (A) Waterfall plot illustrates the frequently mutated genes in KIRC from ICGC cohort. (B) Venn diagram of the 30 most frequently mutated genes covered by TCGA, ICGC and UTokyo cohorts.
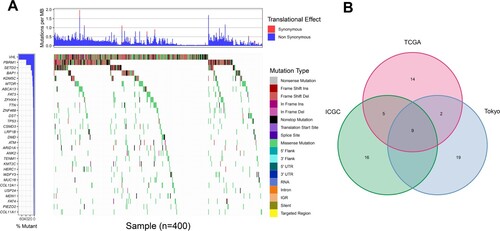
We observed some common frequent genetic mutations. Thus, we a took an intersection of 30 most frequently mutated genes among TCGA, ICGC and UTokyo data sets of KIRC and determined nine common frequently mutated genes, including VHL, PBRM1, SETD2, BAP1, MTOR, TTN, CSMD3, KMT2C and MUC16 (Figure (B)).
BAP1 mutation associated with both TMB and survival prognosis
Among these commonly mutated genes, patients with a mutation in SETD2, MTOR, TTN, CSMD3, KMT2C and MUC16 had significantly higher TMB, while patients with a mutation in VHL, PBRM1 and BAP1 had significantly lower TMB (Figure (A)). Furthermore, we performed a Kaplan-Meier analysis and log-rank test to verify whether these gene mutations associated with TMB could also influence the prognosis of patients with KIRC. Interestingly, only BAP1 mutation (p = 0.002) was associated with a significantly poorer prognosis (Figure (B)). The former results indicated that BAP1 mutation presented a high tendency to co-occur with MUC16 in Figure (B). Accordingly, we additionally investigated the interaction effect of BAP1 and MUC16 on the prognosis of the patients. Patients who carried both BAP1 and MUC16 mutations manifested to harbor a dreadful prognosis (Figure (C)). However, BAP1 mutation did not remain statistically significant after considering age, gender, grade, stage and TMB status in the multivariate Cox regression model (Figure ), indicating that BAP1 mutation was related with other clinicopathologic factors.
Figure 3. Investigation of gene mutation associated with both TMB and overall survival. (A) Several common mutated genes’ mutation is associated with TMB. (B) Kaplan-Meier survival curves of patients with gene mutations. (n = 336). The log-rank p-value is marked in each graph. (C) Influence of the interaction between BAP1 mutation and MUC16 mutation on the prognosis in KIRC patients.
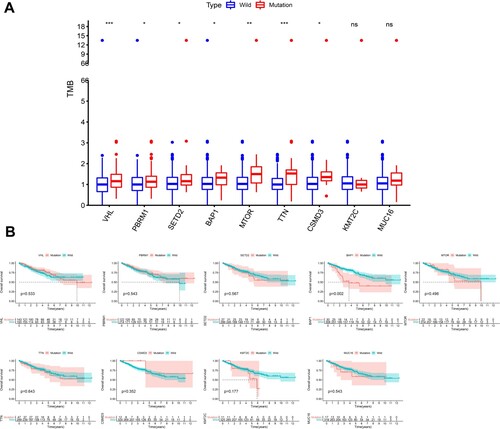
Figure 4. Univariate and multivariate cox regression analysis. Univariate and multivariate overall survival analysis considering age, gender, grade, stage and TMB status in the Cox regression model. The left green one was univariate cox regression and the right red one represented the results of multivariate cox regression.
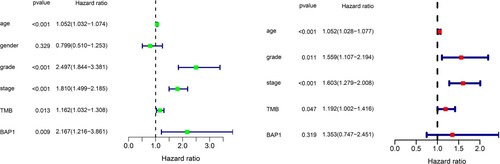
Tumor-infiltrating immune cells associated with BAP1 mutation in KIRC
We analyzed the association between BAP1 mutation and tumor-infiltrating immune cells in the KIRC tumor microenvironment by the CIBERSORT algorithm. As indicated in Figure (A), the composition of 22 immune cell types in each sample varied significantly. Meanwhile, we found that T cells regulatory (T regs) and Mast cells resting were more enriched in the wild-type group (Figure (B)). Furthermore, the result from the correlation matrix uncovered that T regs had a positive correlation with T cells CD8, and Mast cells resting had a negative correlation with T cells follicular helper (Figure (C)).
Figure 5. Association between BAP1 mutation and tumor-infiltrating immune cells. (A) Stacked bar chart illustrates distribution of 22 immune cells in each sample. (B) Violin plot shows the differentially infiltrated immune cells between BAP1-mutant groups and BAP1-wild group. (C) Correlation matrix of immune cell proportions. The red color represents positive correlation and the blue color represents negative correlation.
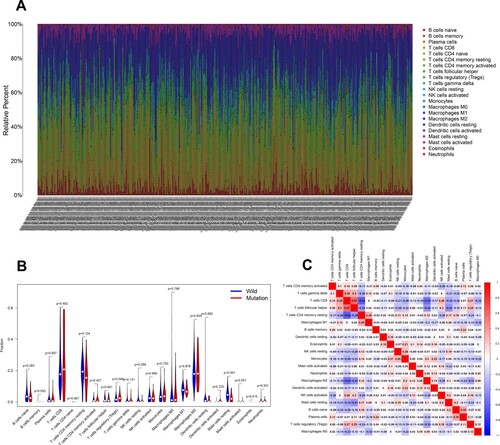
Discussion
We, respectively, analyzed the somatic mutation landscape of 336 American KIRC samples from the TCGA data set, 400 Chinese KIRC samples from the ICGC data set, and 106 Japanese KIRC samples from the UTokyo data set. Then, we discovered that BAP1 was frequently mutated in these three data sets, and its mutation was associated with lower TMB and harmful clinical prognosis. Tumor-infiltrating immune cells results indicated that BAP1 mutated samples were less infiltrated in Tregs and mast cell resting.
BRCA1-related protein 1 (BAP1), encoded by the BAP1 gene, was first discovered as a noble ubiquitin carboxyl-terminal hydrolase in 1998, which is responsible for removing ubiquitin from protein substrate (Jensen et al. Citation1998). Clinical reports have demonstrated that BAP1 is commonly lost or inactivated in a variety of cancers (Chau et al. Citation2019). In our study, the mutation of BAP1 in KIRC was observed as unfavorable for the prognosis of the patients. In former publications, germline mutations of BAP1 were reported to confer a novel tumor predisposition syndrome, characterized by a high incidence of malignancies consisting of melanoma, mesothelioma, cutaneous melanoma and other cancers (Chau et al. Citation2019). BAP1 plays a significant role as a tumor suppressor in numerous cellular processes, including DNA damage response, cell cycle regulation, cell growth and immune response (Zhao et al. Citation2017; Shrestha et al. Citation2019). Therefore, reduced levels of BAP1, as observed in carriers of heterozygous BAP1 mutations, may increase genetic damage that occurs spontaneously during cell division or when exposed to environmental carcinogens (Carbone et al. Citation2020). A recent study also reported that BAP-1 mutations were associated with aggressive clinical behavior, frequent papillary architecture, eosinophilic cytoplasm and eosinophilic cytoplasmic globules, which corresponded to our outcomes (Gallan et al. Citation2021).
In addition, we discovered that BAP1 and MUC16 tightly interacted. MUC16 mutation showed no significant effect on the survival of KIRC patients. Nevertheless, the co-occurrence of BAP1 and MUC16 mutations had a significant dreadful influence on the prognosis. Increased levels of MUC16 were reported to be correlated with poor prognosis and advanced tumor stage (Moore et al. Citation2021). However, further investigations of the crosstalk between BAP1 and MUC16 were warranted.
TMB is defined as an indicator of genomic alterations based on extensive sequencing data and has been illustrated to be positively related to better prognosis and immune response. DNA repair deficiency drives genomic instability, dysregulated tumor microenvironment and increased secretion of cytokines, which may explain the association between the BAP1 mutation with low TMB (Tan et al. Citation2020). As a response, tumor cells evade this immune surveillance by increased expression of immune checkpoint receptors, suggesting BAP1 as a predictor for immunotherapeutic response (Shrestha et al. Citation2019).
A previous study demonstrated that ASXL1–BAP1 expression promoted the expansion of myeloid cells (Balasubramani et al. Citation2015), responding to the reduction of mast cells in BAP1 mutation patients. Mast cells (MCs) are essential for numerous immune and physiologic functions (Merluzzi et al. Citation2015). MCs have proven to be major contributors to release several inflammatory mediators, such as TNF-a, TGF-b or IL-10 (Landskron et al. Citation2014). Although MCs’ role in tumor generation and progression remains controversial (Varricchi et al. Citation2019), they have been believed to participate in antitumor immunity including inducing tumor cell apoptosis or eosinophil recruitment by mast cell proteases and IL-5 (Dudeck et al. Citation2019) and assisting IL-9–secreting T cells to mount B16F10 (Purwar et al. Citation2012). Therefore, we found that BAP1 mutation elicited the variation of infiltrated immune cells contributing to antitumor immunity in KIRC.
Additionally, Federico et al. confirmed a significant visceral adipose tissue accumulation in KIRC patients, and the increase was associated with genetic mutations in KIRC (Greco et al. Citation2020; Greco and Mallio Citation2021). The possible interaction between visceral adipose tissue with TMB and BAP1 mutations could also influence the prognosis in KIRC patients. The role of visceral adipose tissue could be further investigated in prospect.
The main limitation in this study lies in the lack of corresponding survival data of ICGC and UTokyo cohorts containing Chinese and Japanese KIRC patients, for which we cannot confirm whether BAP1 mutation is related to the prognosis or the immune response of KIRC patients in Asian race. Assuming that we could get similar results from ICGC and UTokyo data sets, the effect may be somewhat heterogeneous between different ethnic groups. As a result, the association of BAP1 mutation and prognosis, including the analysis in infiltrated immune cells need further validation in more KIRC patients from other races.
Conclusion
In summary, we identified that BAP1 mutations commonly occurred in KIRC patients and demonstrated that BAP1 mutations were associated with lower TMB and a poorer prognosis. Furthermore, BAP1 mutation reversed its function as a tumor suppressor via influencing MCs’ quantity. These findings cast light on BAP1 whose mutation could be regarded as a biomarker to predict immunotherapeutic response.
Geolocation information
Department of Urology, Tongji Hospital, Tongji Medical College, Huazhong University of Science and Technology, Wuhan, People’s Republic of China.
Acknowledgments
We thank all the R programming package developers.
Disclosure statement
No potential conflict of interest was reported by the author(s).
Data availability
The data that support the findings of this study are available in TCGA-portal at http://portal.gdc.cancer.gov/projects (Weinstein et al. Citation2013), ICGC-portal at http://dcc.icgc.org/releases/current/Projects (Zhang et al. Citation2011) and cBioPortal at https://www.cbioportal.org/study/summary?id = ccrcc_utokyo_2013 (Cerami et al. (Citation2012).
References
- Balasubramani A, Larjo A, Bassein JA, Chang X, Hastie RB, Togher SM, et al. 2015. Cancer-associated ASXL1 mutations may act as gain-of-function mutations of the ASXL1-BAP1 complex. Nat Commun. 6:7307. https://pubmed.ncbi.nlm.nih.gov/26095772/.
- Barata PC, Rini BI. 2017. Treatment of renal cell carcinoma: current status and future directions. CA Cancer J Clin 67(6):507–524. https://pubmed.ncbi.nlm.nih.gov/28961310/.
- Carbone M, Harbour JW, Brugarolas J, Bononi A, Pagano I, Dey A, et al. 2020. Biological mechanisms and clinical significance of BAP1 mutations in human Cancer. Cancer Discov. 10:1103–1120. https://pubmed.ncbi.nlm.nih.gov/32690542/.
- Cerami E, Gao J, Dogrusoz U, Gross BE, Sumer SO, Aksoy BA, et al. 2012 May. The cBio cancer genomics portal: an open platform for exploring multidimensional cancer genomics data. Cancer Discov. 2(5):401–404.
- Chalmers ZR, Connelly CF, Fabrizio D, Gay L, Ali SM, Ennis R, et al. 2017. Analysis of 100,000 human cancer genomes reveals the landscape of tumor mutational burden. Genome Med. 9(1):34. https://pubmed.ncbi.nlm.nih.gov/28420421/.
- Chau C, van Doorn R, van Poppelen NM, van der Stoep N, Mensenkamp AR, Sijmons RH, et al. 2019. Families with BAP1-tumor predisposition syndrome in the Netherlands: path to identification and a proposal for genetic screening guidelines. Cancers. 11(8):1114. https://pubmed.ncbi.nlm.nih.gov/31382694/.
- Correa JK, Brown TA. 2019. Expression of generalized anxiety disorder across the lifespan. J Psychopathol Behav Assess. 41(1):53–59. https://pubmed.ncbi.nlm.nih.gov/31938010/.
- Dudeck A, Köberle M, Goldmann O, Meyer N, Dudeck J, Lemmens S, et al. 2019. Mast cells as protectors of health. J Allergy Clin Immunol. 144(4):S4–18. https://pubmed.ncbi.nlm.nih.gov/30468774/.
- Gallan AJ, Parilla M, Segal J, Ritterhouse L, Antic T. 2021. BAP1-mutated clear cell renal cell carcinoma. Am J Clin Pathol. 155(5):718–728.
- Greco F, Mallio CA. 2021. Relationship between visceral adipose tissue and genetic mutations (VHL and KDM5C) in clear cell renal cell carcinoma. Radiol Med. 126(5):645–651. doi:10.1007/s11547-020-01310-y.
- Greco F, Quarta LG, Grasso RF, Beomonte Zobel B, Mallio CA. 2020. Increased visceral adipose tissue in clear cell renal cell carcinoma with and without peritumoral collateral vessels. Br J Radiol. 93(1112):20200334. doi:10.1259/bjr.20200334.
- Jensen DE, Proctor M, Marquis ST, Gardner HP, Ha SI, Chodosh LA, et al. 1998. BAP1: a novel ubiquitin hydrolase which binds to the BRCA1 RING finger and enhances BRCA1-mediated cell growth suppression. Oncogene. 16(9):1097–1112. https://pubmed.ncbi.nlm.nih.gov/9528852/.
- Kowalewski A, Zdrenka M, Grzanka D, Szylberg Ł. 2020. Targeting the deterministic evolutionary trajectories of clear cell renal cell carcinoma. Cancers MDPI AG. 12:1–13. https://pubmed.ncbi.nlm.nih.gov/33182233/.
- Landskron G, De La Fuente M, Thuwajit P, Thuwajit C, Hermoso MA. 2014. Chronic inflammation and cytokines in the tumor microenvironment. J. Immunol. Res. 2014:149185. https://pubmed.ncbi.nlm.nih.gov/24901008/.
- Mayakonda A, Lin DC, Assenov Y, Plass C, Koeffler HP. 2018. Maftools: efficient and comprehensive analysis of somatic variants in cancer. Genome Res. 28(11):1747–1756. https://pubmed.ncbi.nlm.nih.gov/30341162/.
- Méjean A, Ravaud A, Thezenas S, Colas S, Beauval J-B, Bensalah K, et al. 2018. Sunitinib alone or after nephrectomy in metastatic renal-cell carcinoma. N Engl J Med. 379(5):417–427. https://pubmed.ncbi.nlm.nih.gov/29860937/.
- Merluzzi S, Betto E, Ceccaroni AA, Magris R, Giunta M, Mion F. 2015. Mast cells, basophils and B cell connection network. Mol. Immunol. 63:94–103. https://pubmed.ncbi.nlm.nih.gov/24671125/.
- Moore AL, Batavia AA, Kuipers J, Singer J, Burcklen E, Schraml P, et al. 2021. Spatial distribution of private gene mutations in clear cell renal cell carcinoma. Cancers. 13(9):2163. https://pubmed.ncbi.nlm.nih.gov/33946379.
- Motzer RJ, Bukowski RM, Figlin RA, Hutson TE, Michaelson MD, Kim ST, et al. 2008. Prognostic nomogram for sunitinib in patients with metastatic renal cell carcinoma. Cancer. 113(7):1552–1558. https://pubmed.ncbi.nlm.nih.gov/18720362/.
- Motzer RJ, Jonasch E, Agarwal N, Bhayani S, Bro WP, Chang SS, et al. 2017. Kidney cancer, version 2.2017: clinical practice guidelines in oncology. J Natl Compr Cancer Netw. 15:804–834. https://pubmed.ncbi.nlm.nih.gov/28596261/.
- Motzer RJ, Penkov K, Haanen J, Rini B, Albiges L, Campbell MT, et al. 2019. Avelumab plus axitinib versus sunitinib for advanced renal-cell carcinoma. N Engl J Med. 380(12):1103–1115. https://pubmed.ncbi.nlm.nih.gov/30779531/.
- Newman AM, Liu CL, Green MR, Gentles AJ, Feng W, Xu Y, et al. 2015. Robust enumeration of cell subsets from tissue expression profiles. Nat Methods. 12(5):453–457. https://pubmed.ncbi.nlm.nih.gov/25822800/.
- Pinato DJ, Urus H, Newsom-Davis T, Du Parcq P, Belessiotis K, Mapara L, et al. 2020. Applicability of routine targeted next-generation sequencing to estimate tumor mutational burden (TMB) in patients treated with immune checkpoint inhibitor therapy. J Immunother. 43(2):53–56. https://pubmed.ncbi.nlm.nih.gov/31567705/.
- Purwar R, Schlapbach C, Xiao S, Kang HS, Elyaman W, Jiang X, et al. 2012. Robust tumor immunity to melanoma mediated by interleukin-9-producing T cells. Nat Med. 18(8):1248–1253. https://pubmed.ncbi.nlm.nih.gov/22772464/.
- Rajandram R, Bennett NC, Morais C, Johnson DW, Gobe GC. 2012. Renal cell carcinoma: resistance to therapy, role of apoptosis, and the prognostic and therapeutic target potential of TRAF proteins. Med Hypotheses. 78(2):330–336. https://pubmed.ncbi.nlm.nih.gov/22153576/.
- Ricciuti B, Kravets S, Dahlberg SE, Umeton R, Albayrak A, Subegdjo SJ, et al. 2019. Use of targeted next generation sequencing to characterize tumor mutational burden and efficacy of immune checkpoint inhibition in small cell lung cancer. J Immunother Cancer. 7(1):87. https://pubmed.ncbi.nlm.nih.gov/30922388/.
- Salk JJ, Schmitt MW, Loeb LA. 2018. Enhancing the accuracy of next-generation sequencing for detecting rare and subclonal mutations. Nat Rev Genet. 19:269–285. https://pubmed.ncbi.nlm.nih.gov/29576615/.
- Shrestha R, Nabavi N, Lin YY, Mo F, Anderson S, Volik S, et al. 2019. BAP1 haploinsufficiency predicts a distinct immunogenic class of malignant peritoneal mesothelioma. Genome Med. 11(1):8. https://pubmed.ncbi.nlm.nih.gov/30777124/.
- Siegel RL, Miller KD, Jemal A. 2019. Cancer statistics, 2019. CA Cancer J Clin. 69(1):7–34. https://pubmed.ncbi.nlm.nih.gov/30620402/.
- Tan G, Xuan Z, Li Z, Huang S, Chen G, Wu Y, et al. 2020. The critical role of BAP1 mutation in the prognosis and treatment selection of kidney renal clear cell carcinoma. Transl Androl Urol. 9(4):1725–1734. http://tau.amegroups.com/article/view/49617/html.
- Varricchi G, De Paulis A, Marone G, Galli SJ. 2019. Future needs in mast cell biology. Int J Mol Sci. 20:4397. https://pubmed.ncbi.nlm.nih.gov/31500217/.
- Vugrin D. 1987. Systemic therapy of metastatic renal cell carcinoma. Vol. 7, seminars in nephrology. N Engl J Med. 7(2):152–162. https://pubmed.ncbi.nlm.nih.gov/28121507/.
- Weinstein JN, Collisson EA, Mills GB, Shaw KRM, Ozenberger BA, Ellrott K, et al. 2013. The cancer genome atlas pan-cancer analysis project. Nat Genet. 45(10):1113–1120.
- Zhang J, Baran J, Cros A, Guberman JM, Haider S, Hsu J, et al. 2011. International cancer genome consortium data portal – a one-stop shop for cancer genomics data. Database. 2011:bar026. doi:10.1093/database/bar026
- Zhao W, Steinfeld JB, Liang F, Chen X, Maranon DG, Jian Ma C, et al. 2017. BRCA1-BARD1 promotes RAD51-mediated homologous DNA pairing. Nature. 550(7676):360–365. https://pubmed.ncbi.nlm.nih.gov/28976962/.