Abstract
Jin Ning Fang (JNF), a classic Chinese medicine formula, is widely used to treat lung cancer. However, its molecular mechanism remains unclear. Thus, we aimed to explore the pharmacological mechanisms of JNF against lung cancer. JNF targets and lung cancer-related genes were derived from public databases. Key compounds, potential therapeutic targets, and pathways were determined via bioinformatics analyses. Further, molecular docking, in vitro experiments, and real-time PCR were performed to verify the findings. A total of 27 active compounds and 25 genes were selected. Pharmacological network revealed that quercetin, calcium carbonate, and beta-sitosterol might be candidate agents; ESR1, AR, AKT1, FDPS, PIM1, VCAM1, SLC29A1, NQO1, and ANPEP could be potential drug targets. These genes were involved in apoptosis pathway. Docking analysis showed that beta-sitosterol combined well with AKT1, AR, and ESR1. In vitro experiments confirmed that JNF could inhibit proliferation and promote apoptosis of A549 cells. JNF treatment inhibited the level of FDPS, PIM1, VCAM1, SLC29A1, NQO1, and ESR1, while increased the level of AR and ANPEP. This study illuminated that JNF exerts anti-lung cancer effects through regulating multiple targets and pathways, and the identified genes may be used as potential therapeutic targets for lung cancer.
Introduction
Lung cancer is one of the leading causes of cancer-related deaths in China, and its incidence and mortality rates have been significantly increased in the past decades (Cao and Chen Citation2019; Guo et al. Citation2019). In recent years, traditional Chinese medicine (TCM) has been widely used as the main or adjuvant medicine in the treatment of lung cancer with minimal side effects (Zhang et al. Citation2018; Su et al. Citation2020). However, owing to the complex chemical composition and diversity of TCM prescriptions, the elucidation of their potential active compounds and anti-cancer mechanisms has been challenging.
Jin Ning Fang (JNF), a traditional TCM prescription, is composed of five herbs, namely, Selaginella doederleinii Hieron. (Shishangbai), Salvia chinensis Benth. (Shijianchuan), Bufo bufo gargarizans Cantor (Chanpi), Ostrea gigas Thunberg (Muli), and Bombyx batryticatus (Jiangcan) (Wu et al. Citation2015). This prescription has the function of clearing away heat and detoxifying, resolving phlegm, and alleviating blood coagulation (Yu et al. Citation2020). Evidences have revealed the potential therapeutic effects of JNF against lung cancer in clinical. For example, our previous study has reported that JNF can inhibit the proliferation of the human lung cancer cell line (95-D) within 24–48 h; in addition, we also reveal that JNF can also promote cell apoptosis via regulating the expression level of Caspase 3 and Caspase 8 (Wu et al. Citation2015). Moreover, JNF combined with postoperative adjuvant chemotherapy may inhibit metastasis recurrence in patients with stage III non-small cell lung cancer (NSCLC) after surgery (Shou et al. Citation2014). Besides, it exerts a relatively stable and controlled effect on the main serum tumor markers and thus can significantly improve the quality of life of patients with NSCLC (Shou et al. Citation2014). However, despite the known therapeutic effects of JNF, its molecular and pharmacological mechanism of the anti-lung cancer effect remains unclear.
Several methods can be used to investigate the mechanisms of the anti-tumor effects of TCM, including network pharmacology. The concept of network pharmacology was first proposed by Hopkins in 2007 (Hopkins Citation2007). Subsequently, the herb network in the TCM research framework was updated as ‘Phenotype network,’ and ‘Network target’ was proposed as a new concept for clarifying the herb formulas of TCM (Yoo et al. Citation2018). Notably, network pharmacology provides support for rational drug design and a better understanding of the mechanisms of multiple drug actions.
In this study, we utilized network pharmacology to explore the potential pharmacological mechanism of JNF against lung cancer. First, the active compounds of JNF were screened from the Traditional Chinese Medicine Systems Pharmacology Database and Analysis Platform (TCMSP) and the Traditional Chinese Medicine Information Database (TCMID). Next, the targets of the active compounds were predicted and then integrated with lung cancer-related genes to obtain potential therapeutic targets. Subsequently, based on the functional pathway analysis and protein–protein interaction (PPI) analysis, a pharmacological network was constructed. Further, in vitro experiments were conducted to observe the inhibitory effect of JNF on the lung cancer cells (A549). Meanwhile, real-time PCR (qPCR) was performed to investigate the expression level of the identified genes. Our study might help clarify the potential mechanism of the anti-lung cancer effect of JNF and provide a theoretical basis for the development of novel drugs in lung cancer treatment.
Material and methods
Active ingredients of JNF
The chemical compounds present in JNF were screened from TCMSP (https://tcmsp-e.com/tcmsp.php) (Ru et al. Citation2014). For the herbs not recorded in TCMSP, we searched the TCMID database (http://www.megabionet.org/tcmid/) (Xue et al. Citation2013) for complete information. Furthermore, the active compounds of JNF were queried using absorption, distribution, metabolism, and excretion (ADME) analysis (Yamashita and Hashida Citation2004), wherein the active compounds of JNF were selected using the following criteria: oral bioavailability ≥ 30% and drug-likeness ≥ 0.18.
Identification of targets of compounds
The targets of active compounds were predicted by using the Bioinformatics Analysis Tool for Molecular Mechanism of Traditional Chinese Medicine (BATMAN-TCM) database (http://bionet.ncpsb.org/batman-tcm/) (Liu et al. Citation2016). BATMAN-TCM is an online platform integrating database sources including Drug Bank (Wishart et al. Citation2006), Kyoto Encyclopedia of Genes and Genomes (KEGG) (Kanehisa and Goto Citation2000), and Therapeutic Target Database (TTD) (Chen et al. Citation2002). It provides drug-gene interactions based on the similarity-based target prediction method, which has practical value for systematically revealing the mechanism of TCMs. Specially, target with score ≥‘Score cutoff’ is considered as potential target of investigated compound. In this study, the name of each herbal medicine in JNF was inputted into BATMAN-TCM website, and then ‘Score cutoff’ and ‘adjusted P value cutoff’ were set as ‘20’ and ‘0.05’ to identify the potential target of JNF. These targets were selected for further analyses.
Identification of lung cancer-related genes and potential therapeutic targets
Lung cancer-related genes were collected from two databases, including the Comparative Toxicogenomics Database (CTD, 2018 update, http://ctdbase.org/) (Davis et al. Citation2019) and DisGeNET database (Piñero et al. Citation2015).
CTD is aimed to promote the understanding of information on environmental risks affecting human health and it provides information on the interrelation between chemical phenotype, genes, gene interactions, and disease (Davis et al. Citation2019). CTD can retrieve target genes associated with specific compounds and ranked them according to the interaction (Barabasi and Albert Citation1999; Li and Liang Citation2009). Thus, we used ‘lung cancer’ as keyword to predict the target genes of lung cancer. Target with an inference score ≥20 was selected as a candidate gene.
In addition, DisGeNET database (https://www.disgenet.org/) is a comprehensive platform that integrates human disease-related genes and mutation information (Piñero et al. Citation2015). We searched this database with the keyword ‘lung cancer’ to obtain disease-related genes.
Further, lung cancer-related genes obtained from CTD and DisGeNET databases were integrated with predicted targets of compounds, and the overlapping genes were selected as anti-lung cancer targets of JNF, which were used for further analysis.
Functional enrichment analysis of potential therapeutic targets
To elucidate the biological function of the therapeutic targets, functional enrichment analysis was performed. The overlapping genes were used as the input set. Gene ontology (GO) function analysis was conducted using the two plugins of Cytoscape: ClueGO and CluePedia (Ashburner et al. Citation2000; Bindea et al. Citation2009). In brief, the relevant biological process (GO-BP) was selected and global options GO levels 3–8 were used to construct a functional network, that was visualized by Cytoscape (Shannon et al. Citation2003). The parameters for selection were as follows: P.adjust ≤ 0.01 and Kappa score threshold = 0.4 (Wang et al. Citation2019). Meanwhile, the KEGG pathway (Kanehisa and Goto Citation2000) was analyzed using clusterProfiler, with P ≤ 0.05 as significant.
PPI analysis
The STRING database (version 10.0, http://www.string-db.org/) (Szklarczyk et al. Citation2015) provides predicted and experimental information of protein interactions. Here, the therapeutic genes were entered into the STRING database to construct a PPI network and explore the interactions between the genes. A high confident interaction score of > 0.9 was selected as the threshold for PPI analysis. Next, the PPI network was visualized by Cytoscape.
Construction of pharmacological network
To better understand the molecular mechanism of JNF against lung cancer, a pharmacological network based on compounds, targets, and pathways was constructed using Cytoscape. In brief, the network was obtained by integrating the herb-compound-target pairs and target-related pathways.
Validation of compound-target via molecular docking
The three-dimensional (3D) structures of the candidate genes were downloaded from the RCSB Protein Data Bank database (http://www.rcsb.org/). This analysis removed the water molecule and separated the proteins and smaller molecules. Meanwhile, the molecular structures of the key compounds were downloaded from the DrugBank database (https://go.drugbank.com/) (Wishart et al. Citation2006). Subsequently, the identified key compound (beta-sitosterol) was used as a ligand and its corresponding target genes (AKT1, AR, and ESR1) were used as receptors. Based on the information of the BPD or the sites of the original ligands of the proteins, the binding sites that contacted the receptor and the ligand were searched. Next, the molecular docking was performed using Autodock (version 4.2.6) (Trott and Olson Citation2010).
Preparation of the JNF
JNF is composed of five dried medicinal herbs, including Selaginella doederleinii Hieron. (Selaginellaceae), 30 g; Salvia chinensis Benth. (Labiatae), 20 g; Bufo deadline gargarizans Cantor (Bufonidae), 5 g; Bombyx batryticatus (Bombycidae), 5 g; and Ostrea gigas Thunberg (Ostreidae), 10 g. JNF solution was prepared according to the previous study (Wu et al. Citation2015). In brief, the mixed herbs were soaked in 500 mL distilled water for 1 h and the mixture was then decocted for 20 min at 100°C. The liquid was collected as the first filtrate. Next, an equivalent amount of drinking water was added to the rest of the mixed herbs, and above extraction step was repeated, and then the liquid obtained from this process was considered as the second filtrate. Finally, the two filtrates were combined and concentrated to form the JNF decoction (1.5 g/mL of crude drug) and stored at 4°C.
Acquisition of JNF-containing serum
Four adult male Sprague–Dawley (SD) rats (240–260 g) were purchased from Shanghai Keting Biological Technology Co., Ltd. and allowed to acclimate for 1 week under standard conditions. The rats were randomly divided into two groups (n = 2): control and JNF groups. The drug-containing serum was obtained according to previous literature (Gao et al. Citation2020), with some modification. In brief, the rats in the JNF group were subjected to intragastric administration of the JNF decoction (14 g/kg/d; equal to clinical dosage), and the rats in the control group were administered an equal volume of saline. After 7 d of administration, the rats were anesthetized with sodium pentobarbital (30 mg/kg) and blood was collected from their abdominal aorta. The collected blood was centrifuged at 3000 rpm/min for 15 min, and the supernatant was obtained. Next, the supernatant was inactivated at 56°C for 30 min, and then filtered via a 0.22 µm membrane. Finally, the serum was dispensed into 10 mL bottles and preserved at −80°C for further use. All animal experiments were performed in accordance with the NIH Guide for the Care and Use of Laboratory Animals, and the procedures were approved by the Institutional Animal Care and Use Committee (IACUC) of the Longhua Hospital affiliated to Shanghai TCM University (IACUC21-0649).
Cell culture
The human lung cancer cell line A549 was obtained from the National Collection of Authenticated Cell Cultures (Shanghai, China). A549 cells were cultured in RPMI-1640 medium (GibcoTM, Invitrogen Corporation, France) containing 10% fetal bovine serum (FBS), 100 µg/mL penicillin, and 100 mg/mL streptomycin. The cells were maintained in humidified condition with 5% CO2 at 37°C (Wang et al. Citation2017). Subsequently, the cells in the logarithmic growth phase were trypsinized and centrifuged at 300 g for 3 min. After discarding the supernatant, 3 mL of fresh medium was added to resuspend the cells. Next, the cells were cultured in 96-well plates with 4000 cells per well at 37°C and 5% CO2 for 1 d. The culture medium was then changed and the cells were divided into two groups: control group (basic medium with 10% normal rat serum) and JNF group (basic medium with 10% rat JNF-containing serum).
CCK-8 assay
CCK-8 assay was performed to assess cell viability according to the method described in the previous study (Zhang et al. Citation2020). After incubation for 48 h, 100 µL of fresh serum-free medium containing 10% CCK-8 solution (5 mg/mL) was added to each well. After culturing in the dark for 1 h, the absorbance was detected at 450 nm using a microplate reader (Infinite M100 PRO) and cell viability was calculated.
Apoptosis assay
The ratio of apoptotic cells was examined using an Annexin V-PE/7-AAD apoptosis kit (#88-8102-74; Ebioscience, AUT) according to the manufacturer’s instructions. Apoptosis assay was conducted based on the previous described (Fan et al. Citation2020). In brief, the treated cells were washed with 1 mL of cold PBS, and then re-suspended in 100 µL 1× binding buffer. Next, 5 µL Annexin V-PE and 5 µL 7-AAD (50 ug/mL) were added to the cell suspension. After mixing, the cells were incubated in the dark at 25°C for 15 min. Finally, the cell samples were detected by FACSCalibur flow cytometry (BD Biosciences) and analyzed using Flowjo7.6.1 software.
qPCR assay
In order to determine the mRNA expression level of several key genes obtained in the pharmacological network, qPCR analysis was conducted as previously described (Nolan et al. Citation2006). In brief, the treated cells were washed with PBS, then 1 mL of Trizol was added to extract the total RNA. Reverse transcription was performed using the ReverTra Ace® qPCR RT Master Mix kit (#FSQ-201; TOYOBO co., ltd.) and qPCR was conducted using the Power SYBR Green PCR Master Mix kit (#A25742; Thermo Fisher Scientific). The relative expression level of genes was normalized to that of GADPH. The primer sequences for the genes examined in this experiment are listed in Table S1.
Statistical analysis
All experiments were repeated three times and data are presented as the mean ± standard deviation (SD). Statistical analysis was performed using the Graphpad prism 5 (Graphpad Software, San Diego, CA). Statistical comparisons were conducted using the unpaired t test or the two-tailed test. Data with P < 0.05 were considered to be statistically significant.
Results
Screening of active compounds
The number of chemical compounds identified for each ingredient of JNF was as follows: Selaginella doederleinii Hieron., 30; Salvia chinensis Benth., 16; Bufo GraphPad gargarizans Cantor, 24; Ostrea gigas Thunberg, 10; and Bombyx batryticatus, 4. After screening using the ADME criteria, a total of 27 active compounds were identified from the five ingredients of JNF. Information regarding the chemical composition of each ingredient is presented in Table . In addition, 203 targets of seven active compounds from two ingredients (Chinese sage and oyster) were searched in the BATMAN database (Table S2).
Table 1. Important chemical compounds in Jin Ning Fang decoction.
Screening and cross-validation of target proteins
A total of 3681 lung cancer-related genes were obtained from the CTD and DisGeNET databases (Table S3). After integrating these genes with targets of the active compounds, 25 overlapping genes were obtained for further analysis (Figure ).
Functional enrichment pathway analysis of cross-targets
GO functional analysis revealed that these overlapping genes were significantly enriched in 45 GO functional terms (Figure A), and these terms were divided into seven categories based on the Kappa coefficient, such as negative regulation of vitamin metabolic processes and regulation of lipid biosynthetic processes (Figure B). Moreover, the GO functional network showed that 19 targets were significantly enriched in 45 GO-BP terms (Figure A). The most enriched GO-BP terms were endogenous apoptosis signaling pathways of DNA damage, cell responses to ketones, cell responses stimulated by corticosteroids, and lymphocyte homeostasis.
Figure 2. Results of Gene Ontology (GO) functional analysis. A: Histogram. The vertical axis is the GO term, and the horizontal axis is the percentage of genes enriched in each related GO functional term. GO functional grouping is distinguished by the color of the bars. The number on the right of the bar represents the number of genes enriched in each related GO functional term. * P < 0.05, ** P < 0.01. B: Pie chart.

Figure 3. Functional enrichment analysis of 25 targets. A: GO-BP functional network. Larger dots indicate smaller P values. Higher kappa coefficients indicate a stronger term association. B: The Kyoto Encyclopedia of Genes and Genomes (KEGG) pathway analysis for 25 targets. The vertical axis is the path name, and the horizontal axis is the number of enriched genes.
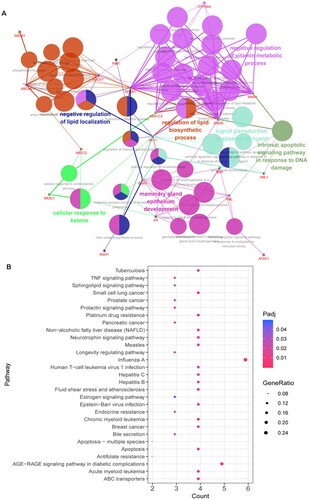
Pathway enrichment analysis of cross-targets was performed using clusterProfiler, and a total of 158 pathways were obtained. Pathways with P.adjust < 0.05 are presented in Figure (B). The results showed that these genes were mainly involved in ABC transport, platinum drug resistance, and apoptosis pathways.
PPI network analysis
Based on the 25 candidate genes, a PPI network was constructed by importing the gene ID of these targets to the STRING database. As shown in Figure , a total of 23 nodes and 61 edges were obtained. The top 10 proteins with the highest degree of interactions are shown in Table , including AKT1, AR, VCAM1, and ESR1, and these proteins were recognized as hub proteins.
Figure 4. Protein-protein interaction (PPI) network of target proteins. The size of the dots represents the degree of association with other proteins. The larger the dot, the stronger the degree of association.
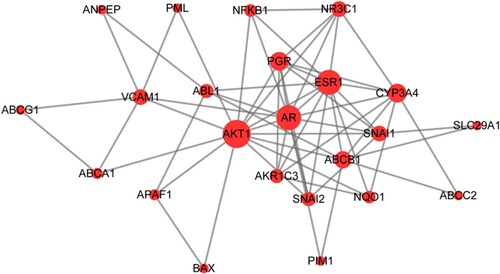
Table 2. Top ten genes in protein-protein interaction (PPI) network.
Pharmacological network analysis
Furthermore, a pharmacological network was constructed based on the ingredients, active components, target proteins, and pathways, and the results are shown in Figure . This network was composed of 59 nodes and 134 pairs. Among these nodes, there were two ingredients (Chinese sage and oyster), three chemical composition nodes (quercetin, calcium carbonate, and beta-sitosterol), 25 target proteins (including AKT1, AR, VCAM1, FDPS, and ESR1), as well as 29 pathways (including ABC transport, platinum drug resistance, and apoptosis).
Results of molecular docking
The analysis in the pharmacological network revealed genes (AKT1, AR, and ESR1) and active compounds (beta-sitosterol) as key nodes. Thus, molecular docking analysis was performed using Autodock 4.2.6 to visualize the interaction between beta-sitosterol and its potential targets. A total of 10 docking models were obtained for the three genes and beta-sitosterol. Binding energy was calculated in the molecular docking analysis; a lower score indicates a stronger binding affinity. Thus, we sorted the docking scores and selected the best docking model for the three genes (the complete data are listed in Table S4). The optimal molecular docking models are shown in Figure . In brief, beta-sitosterol was predicted to dock into the binding pocket of AKT1 via hydrogen bonds with TYP-272 and ASN-64 (Figure A and B). The hydrogen bond was predicted between beta-sitosterol and AR (Figure C and D). Meanwhile, beta-sitosterol was predicted to dock in the binding pocket of ESR1 via hydrogen bond with PRO-535 (Figure E and F). Beta-sitosterol showed good docking with the corresponding proteins, and the key amino acids around it mainly played a role in the formation of hydrogen bonds. Hydrogen bonds minimize the energy of small compounds; thus, small compounds are the most stable. The binding energies of AKT1, AR, and ESR1 were −10.36 kcal/mol, −8.16 kcal/mol, and −8.97 kcal/mol, respectively. The docking energy scores of these compounds were relatively low, suggesting that they could stably bind to the receptor proteins and exert their effects.
Figure 6. Molecular models of the binding of beta-sitosterol to its predicted protein targets. A: Global map of AKT1-beta-sitosterol molecular docking. Off-white represents the receptor, while the pink ball indicates the small ligand beta-sitosterol. B: Binding interaction between AKT1 and beta-sitosterol. C: Global map of the AR-beta-sitosterol model. D: Binding interaction between AR and beta-sitosterol. E: Global map of the ESR1-beta-sitosterol model. F: Binding interaction between ESR1 and beta-sitosterol.
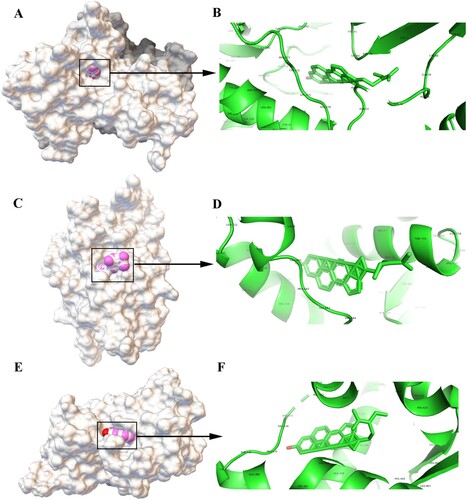
Effect of JNF on the proliferation and apoptosis of the lung cancer cell line
Further, A549 cells were treated with JNF for 48 h. As shown in Figure (A), cell viability in JNF was significantly lower than in the control group (100.00 ± 1.47 vs. 82.59 ± 2.81, P < 0.01), suggesting that JNF observably inhibited the proliferation of lung cancer cells. In addition, JNF increased the apoptosis rate in A549 cells compared to that in the control group (0.74 ± 0.03 vs. 2.20 ± 0.14, P < 0.01), indicating that JNF treatment may induce apoptosis in lung cancer cells (Figure B and C).
Figure 7. Effect of JNF on the proliferation and apoptosis of lung cancer cells. A: CCK-8 assay was performed to observe cell viability. B: Flow cytometry was used to detect the apoptosis of A549 cells. C: Quantification of living cells and apoptosis cells. Data are presented as mean ± SD. ** P < 0.01.
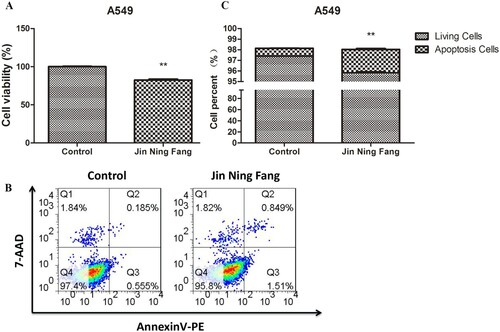
Effect of JNF on mRNA expression levels of several genes in lung cancer cells
In order to observe the effect of JNF on gene expression levels in lung cancer cells, several identified genes, including FDPS, PIM1, VCAM1, SLC29A1, NQO1, ANPEP, ESR1, and AR, were detected by qPCR. JNF could significantly decrease the expression level of FDPS, PIM1, VCAM1, SLC29A1, NQO1, and ESR1 (all P < 0.05), while JNF markedly increased the mRNA levels of AR and ANPEP (all P < 0.05, Figure ).
Discussion
JNF is a classical TCM preparation for the clinical treatment of lung cancer. In our study, we applied a network pharmacology method to explore the molecular mechanism of JNF against lung cancer via constructing a ‘herb-compound-target-pathway’ network. A total 27 active compounds and 25 putative genes were obtained from public databases. PPI analysis revealed that genes such as AKT1, AR, and ESR1 might play roles in JNF against lung cancer. The pharmacological network revealed that quercetin, calcium carbonate, and beta-sitosterol could be the key compounds in JNF. Molecular docking analysis indicated that beta-sitosterol docked well with AKT1, AR, and ESR1. Moreover, we also observed that JNF could inhibit proliferation and promote apoptosis of lung cancer cells. Importantly, the expression level of several genes, including FDPS, PIM1, VCAM1, SLC29A1, NQO1, ANPEP, ESR1, and AR, was significantly altered after JNF treatment.
We also determined that quercetin, calcium carbonate, and beta-sitosterol could be considered the key compounds in the anti-lung cancer effect exerted by JNF. Quercetin is a flavanol and is closely associated with proteins involved in apoptosis, the cell cycle, detoxification, antioxidant replication, and angiogenesis (Kashyap et al. Citation2016). Chang et al. (Citation2017) showed that quercetin, by inhibiting Akt activation, suppresses the metastatic ability of lung cancer. Beta-sitosterol is a medicine derived from a traditional plant. It can regulate various pathways related to cell signaling, such as the cell cycle, cell apoptosis, and cell proliferation (Ulbricht Citation2016). This compound also has anti-cancer properties against multiple cancers, including breast and lung cancers (Bin Sayeed and Ameen Citation2015). Moreover, calcium carbonate is used as a drug carrier targeting cancer cells (Maleki Dizaj et al. Citation2019). Taken together, these studies provide evidence for the potential role of these compounds in the treatment of lung cancer.
In the both PPI and pharmacological networks, several hub genes were identified, such as AKT1, AR, ESR1, FDPS, and VCAM1. AKT1 is belonged to the AKT family, which can regulate biological function, including cell survival, metabolism, and proliferation, to influence the growth of cancer cells (Hers et al. Citation2011). A previous study indicated that overexpression of AKT1 promoted the local tumor growth of breast cancer (Riggio et al. Citation2017). Notably, AKT1 is involved in the treatment of lung cancer via regulating some signaling pathways, especially the PI3K-AKT signaling (Gao et al. Citation2018) and AKT1 signaling (Wu et al. Citation2019). AR is mainly associated with the regulation of male sex steroids, and its expression has been detected within normal lung cells and some lung cancer cells (Mikkonen et al. Citation2010). AR and their variants promote tumorigenesis and metastasis in some hormone-related tumors, such as breast cancer (Anestis et al. Citation2020), hepatocellular carcinoma (Kanda et al. Citation2014), and prostate cancer (Pisano et al. Citation2021). However, the relationship between AR and lung cancer has not been explored, and further study still need to be conducted. Proteins encoded by ESR1 are involved in DNA binding, transcription activation and hormone binding. Overexpression of ESR1 is related to the prognosis of patients with lung cancer (Suga et al. Citation2008). Meanwhile, ESR1 is a predictive biomarker that is of therapeutic importance in lung cancer (Atmaca et al. Citation2014). In this study, we found that JNF treatment decreased the mRNA level of ESR1 in the A549 cell, indicating JNF may play role in lung cancer treatment via regulating the expression of ESR1. FDPS (also called FPPS) has been recently reported to serve a role in cancer progression. Lin et al. (Citation2018) revealed that the expression level of FPPS was significantly correlated with the TNM staging and metastasis of NSCLC, and it mediated NSCLC cell invasion. Moreover, VCAM1 encodes a cell surface sialoglycoprotein expressed by the cytokine-activated endothelium, which is a member of the Ig superfamily. A previous study indicated that VCAM1 was overexpressed in the lung cancer-associated fibroblasts; in addition, VCAM1 could promote the growth and invasion of lung cancer cells via activating the AKT and MAPK signaling (Zhou et al. Citation2020). In this study, we observed that the level of VCAM1 was significantly decreased in the JNF-treated lung cancer cell, which further suggested that VCAM1 might be a therapeutic target for lung cancer. Taken together, these results highlighted the direct and indirect relationship between the identified key genes and lung cancer treatment, indicating that these genes might be potential therapeutic targets for JNF against lung cancer.
Molecular docking is the most widely used method for calculating protein-compound interactions, and we performed this assay using Autodock to explore probable binding modes. Our results indicated that beta-sitosterol had a high binding affinity for AKT1, AR, and ESR1. These findings also indirectly showed that the results of molecular docking were consistent with those of network pharmacology analysis, which further verified the reliability of the targets predicted via network pharmacology.
This is the first study to examine the potential pharmacological mechanisms of JNF in treating lung cancer. We not only screened the potential pharmacological targets of JNF using bioinformatics analyses, but also validated the results at the cellular level. We observed that JNF treatment could inhibited the proliferation of lung cancer cells as well as induce apoptosis in lung cancer cells. However, our study has some limitations. First, there are many subtypes of lung cancer and the scope of this study do not include detailed analyses of the different types. Second, the identified targets of JNF are involved in some signaling pathways, such as prolactin and neurotrophin signaling pathways, yet the specific regulatory mechanism has not been studied in detail. Besides, our findings have not been validated in vivo, and the identified key compounds have not been detected by using the high-performance liquid chromatography (HPLC). Therefore, the focus of our follow-up research is to resolve these limitations.
Conclusion
In conclusion, this study explored that JNF exerted its anti-cancer effects in lung cancer via regulating multiple compounds, targets, and pathways. Five hub genes, including AKT1, AR, ESR1, FDPS, and VCAM1, may be used as potential targets of JNF on lung cancer. We also demonstrated that JNF may inhibit the proliferation and promote the apoptosis of lung cancer cells. These findings provide a potential pharmacological mechanism of JNF against lung cancer. However, the obtained results should be verified by further clinical trials.
Ethics approval
All animal experiments were performed in accordance with the NIH Guide for the Care and Use of Laboratory Animals, and the procedures were approved by the Institutional Animal Care and Use Committee (IACUC) of Longhua Hospital Affiliated to Shanghai TCM University (IACUC21-0649).
Informed consent
Not applicable.
Authors’ contributions
Conception and design of the research: Chunxiao Wu, Qi Bao.
Acquisition of data: Qiquan Yu.
Analysis and interpretation of data: Weizhen Shou, Wentao Guo.
Statistical analysis : Kun Zhang, Yang Li.
Drafting the manuscript: Chunxiao Wu.
Revision of the manuscript for important intellectual content: Qi Bao.
Supplemental Material
Download Zip (1.2 MB)Data availability statement
The data analyzed in network pharmacology approach are derived from the following resources available in the public domain: Traditional Chinese Medicine Systems Pharmacology Database (TCMSP, https://tcmsp-e.com/tcmsp.php); Traditional Chinese Medicine Integrated Database (TCMID, http://www.megabionet.org/tcmid/); Bioinformatics Analysis Tool for Molecular Mechanism of Traditional Chinese Medicine (BATMAN-TCM, http://bionet.ncpsb.org/batman-tcm/); Comparative Toxicogenomics Database (CTD, 2018 update, http://ctdbase.org/); and DisGeNET database (https://www.disgenet.org/). The data that support the findings of CCK-8, apoptosis, and qPCR assays are available in 4TU. ResearchData at https://www.doi.org/10.4121/18092750.
Disclosure statement
No potential conflict of interest was reported by the author(s).
References
- Anestis A, Zoi I, Papavassiliou AG, Karamouzis MV. 2020. Androgen receptor in breast cancer-clinical and preclinical research insights. Molecules. 25:358.
- Ashburner M, Ball CA, Blake JA, Botstein D, Butler H, Cherry JM, Davis AP, Dolinski K, Dwight SS, Eppig JT, et al. 2000. Gene ontology: tool for the unification of biology. The gene ontology consortium. Nat Genet. 25:25–29.
- Atmaca A, Al-Batran S-E, Wirtz RM, Werner D, Zirlik S, Wiest G, Eschbach C, Claas S, Hartmann A, Ficker JH, et al. 2014. The validation of estrogen receptor 1 mRNA expression as a predictor of outcome in patients with metastatic non-small cell lung cancer. Int J Cancer. 134:2314–2321.
- Barabasi AL, Albert R. 1999. Emergence of scaling in random networks. Science. 286:509–512.
- Bindea G, Mlecnik B, Hackl H, Charoentong P, Tosolini M, Kirilovsky A, Fridman W-H, Pagès F, Trajanoski Z, Galon J. 2009. ClueGO: a Cytoscape plug-in to decipher functionally grouped gene ontology and pathway annotation networks. Bioinformatics. 25:1091–1093.
- Bin Sayeed MS, Ameen SS. 2015. Beta-Sitosterol: a promising but orphan nutraceutical to fight against cancer. Nutr Cancer. 67:1214–1220.
- Cao M, Chen W. 2019. Epidemiology of lung cancer in China. Thorac Cancer. 10:3–7.
- Chang J-H, Lai S-L, Chen W-S, Hung W-Y, Chow J-M, Hsiao M, Lee W-J, Chien M-H. 2017. Quercetin suppresses the metastatic ability of lung cancer through inhibiting snail-dependent Akt activation and snail-independent ADAM9 expression pathways. Biochim Biophys Acta Mol Cell Res. 1864:1746–1758.
- Chen X, Ji ZL, Chen YZ. 2002. TTD: therapeutic target database. Nucleic Acids Res. 30:412–415.
- Davis AP, Grondin CJ, Johnson RJ, Sciaky D, McMorran R, Wiegers J, Wiegers TC, Mattingly CJ. 2019. The comparative toxicogenomics database: update 2019. Nucleic Acids Res. Jan. 8(47):D948–D954. Epub 2018/09/25.
- Fan J, Ren D, Wang J, Liu X, Zhang H, Wu M, Yang G. 2020. Bruceine D induces lung cancer cell apoptosis and autophagy via the ROS/MAPK signaling pathway in vitro and in vivo. Cell Death Dis. 11:126.
- Gao Y, Yang J, Cai Y, Fu S, Zhang N, Fu X, Li L. 2018. IFN-γ-mediated inhibition of lung cancer correlates with PD-L1 expression and is regulated by PI3K-AKT signaling. Int J Cancer. 143:931–943.
- Gao Z, Deng G, Li Y, Huang H, Sun X, Shi H, Yao X, Gao L, Ju Y, Luo M. 2020. Actinidia chinensis planch prevents proliferation and migration of gastric cancer associated with apoptosis, ferroptosis activation and mesenchymal phenotype suppression. Biomed Pharmacother. 126:110092.
- Guo H, Chang Z, Wu J, Li W. 2019. Air pollution and lung cancer incidence in China: who are faced with a greater effect? Environ Int. 132:105077.
- Hers I, Vincent EE, Tavaré JM. 2011. Akt signalling in health and disease. Cell Signal. 23:1515–1527.
- Hopkins AL. 2007. Network pharmacology. Nat Biotechnol. Oct. 25:1110–1111. Epub 2007/10/09.
- Kanda T, Jiang X, Yokosuka O. 2014. Androgen receptor signaling in hepatocellular carcinoma and pancreatic cancers. World J Gastroenterol. 20:9229–9236.
- Kanehisa M, Goto S. 2000. KEGG: kyoto encyclopedia of genes and genomes. Nucleic Acids Res. 28:27–30.
- Kashyap D, Mittal S, Sak K, Singhal P, Tuli HS. 2016. Molecular mechanisms of action of quercetin in cancer: recent advances. Tumour Biol. 37:12927–12939.
- Li H, Liang S. 2009. Local network topology in human protein interaction data predicts functional association. PLoS One. 4:e6410.
- Lin L, Li M, Lin L, Xu X, Jiang G, Wu L. 2018. FPPS mediates TGF-β1-induced non-small cell lung cancer cell invasion and the EMT process via the RhoA/Rock1 pathway. Biochem Biophys Res Commun. 496:536–541.
- Liu Z, Guo F, Wang Y, Li C, Zhang X, Li H, Diao L, Gu J, Wang W, Li D, et al. 2016. BATMAN-TCM: a bioinformatics analysis tool for molecular mechanism of traditional Chinese medicine. Sci Rep. 6:21146.
- Maleki Dizaj S, Sharifi S, Ahmadian E, Eftekhari A, Adibkia K, Lotfipour F. 2019. An update on calcium carbonate nanoparticles as cancer drug/gene delivery system. Expert Opin Drug Deliv. 16:331–345.
- Mikkonen L, Pihlajamaa P, Sahu B, Zhang F-P, Jänne OA. 2010. Androgen receptor and androgen-dependent gene expression in lung. Mol Cell Endocrinol. 317:14–24.
- Nolan T, Hands RE, Bustin SA. 2006. Quantification of mRNA using real-time RT-PCR. Nat Protoc. 1:1559–1582. Epub 2007/04/05.
- Piñero J, Queralt-Rosinach N, Bravo À, Deu-Pons J, Bauer-Mehren A, Baron M, Sanz F, Furlong LI. 2015. DisGeNET: a discovery platform for the dynamical exploration of human diseases and their genes. Database (Oxford). 2015:bav028.
- Pisano C, Tucci M, Di Stefano RF, Turco F, Scagliotti GV, Di Maio M, Buttigliero C. 2021. Interactions between androgen receptor signaling and other molecular pathways in prostate cancer progression: current and future clinical implications. Crit Rev Oncol Hematol. 157:103185.
- Riggio M, Perrone MC, Polo ML, Rodriguez MJ, May M, Abba M, Lanari C, Novaro V. 2017. AKT1 and AKT2 isoforms play distinct roles during breast cancer progression through the regulation of specific downstream proteins. Sci Rep. 7:44244.
- Ru J, Li P, Wang J, Zhou W, Li B, Huang C, Guo Z, Tao W, Yang Y, Xu X, et al. 2014. TCMSP: a database of systems pharmacology for drug discovery from herbal medicines. J Cheminform. 6:13. Epub 2014/04/17.
- Shannon P, Markiel A, Ozier O, Baliga NS, Wang JT, Ramage D, Amin N, Schwikowski B, Ideker T. 2003. Cytoscape: a software environment for integrated models of biomolecular interaction networks. Genome Res. 13:2498–2504.
- Shou W, Yu Q, Zhu Z, Zhang X, Niu H, Bao Q, Wu X, Li Y. 2014. Clinical effect of “Jinning formula” on postoperative relapse and metastasis in stage III non-small cell lung cancer patients. Shanghai Journal of Tranditional Chinese Medicine. 48:30–33.
- Su X-L, Wang J-W, Che H, Wang C-F, Jiang H, Lei X, Zhao W, Kuang H-X, Wang Q-H. 2020. Clinical application and mechanism of traditional Chinese medicine in treatment of lung cancer. Chin Med J (Engl). 133:2987–2997.
- Suga Y, Miyajima K, Oikawa T, Maeda J, Usuda J, Kajiwara N, Ohira T, Uchida O, Tsuboi M, Hirano T, et al. 2008. Quantitative p16 and ESR1 methylation in the peripheral blood of patients with non-small cell lung cancer. Oncol Rep. 20:1137–1142.
- Szklarczyk D, Franceschini A, Wyder S, Forslund K, Heller D, Huerta-Cepas J, Simonovic M, Roth A, Santos A, Tsafou KP, et al. 2015. STRING v10: protein-protein interaction networks, integrated over the tree of life. Nucleic Acids Res. 43:D447–D452.
- Trott O, Olson AJ. 2010. Autodock vina: improving the speed and accuracy of docking with a new scoring function, efficient optimization, and multithreading. J Comput Chem. 31:455–461.
- Ulbricht CE. 2016. An evidence-based Systematic Review of beta-sitosterol, sitosterol (22,23- dihydrostigmasterol, 24-ethylcholesterol) by the natural standard research collaboration. J Diet Suppl. 13:35–92. Epub 2015/08/14.
- Wang Y, Wang H, Pan T, Li L, Li J, Yang H. 2017. STIM1 silencing inhibits the migration and invasion of A549 cells. Mol Med Rep. 16:3283–3289.
- Wang Y, Yu W, Shi C, Jiao W, Li J, Ge J, Hong Y, Shi G. 2019. Network pharmacology of Yougui Pill combined with Buzhong Yiqi decoction for the treatment of sexual dysfunction. Evid Based Complement Alternat Med. 2019:1243743.
- Wishart DS, Knox C, Guo AC, Shrivastava S, Hassanali M, Stothard P, Chang Z, Woolsey J. 2006. Drugbank: a comprehensive resource for in silico drug discovery and exploration. Nucleic Acids Res. 34:D668–D672.
- Wu H, Liu HY, Liu WJ, Shi YL, Bao D. 2019. miR-377-5p inhibits lung cancer cell proliferation, invasion, and cell cycle progression by targeting AKT1 signaling. J Cell Biochem. 120:8120–8128.
- Wu X, Yu J, Han X. 2015. Study on the suppressive effect of metabolites of Jin Ning Fang on the proliferation of lung cancer cells. Guiding J of Tranditional Chinese Med Pharm. 21:42–45.
- Xue R, Fang Z, Zhang M, Yi Z, Wen C, Shi T. 2013. TCMID: traditional Chinese medicine integrative database for herb molecular mechanism analysis. Nucleic Acids Res. 41:D1089–D1095.
- Yamashita F, Hashida M. 2004. In silico approaches for predicting ADME properties of drugs. Drug Metabolism and Pharmacokinetics. Oct. 19:327–338. Epub 2004/11/19.
- Yoo S, Nam H, Lee D. 2018. Phenotype-oriented network analysis for discovering pharmacological effects of natural compounds. Sci Rep. Aug. 3(8):11667.
- Yu Q, Zhang H, Yang Y, Shou W, Zhang K, Li Y. 2020. Protective effect of Jinning Fang on immunosuppressive mice induced by cyclophosphamide. Chinese Traditional Patent Medicine. 42:2463–2466.
- Zhang X-W, Liu W, Jiang H-L, Mao B. 2018. Chinese herbal medicine for advanced Non-small-cell lung cancer: a systematic review and meta-analysis. Am J Chin Med. 46:923–952.
- Zhang Z-Y, Gao X-H, Ma M-Y, Zhao C-L, Zhang Y-L, Guo S-S. 2020. CircRNA_101237 promotes NSCLC progression via the miRNA-490-3p/MAPK1 axis. Sci Rep. 10:9024.
- Zhou Z, Zhou Q, Wu X, Xu S, Hu X, Tao X, Li B, Peng J, Li D, Shen L, et al. 2020. VCAM-1 secreted from cancer-associated fibroblasts enhances the growth and invasion of lung cancer cells through AKT and MAPK signaling. Cancer Lett. 473:62–73.