Abstract
We aimed to identify co-stimulatory molecule clusters and explore their associations with immune infiltration and tumor mutation burden (TMB) in non-small cell lung cancer (NSCLC), using a systematic analysis based on RNA-seq and mutation data in TCGA database. Twelve immune-related prognostic co-stimulatory molecules were screened. Based on the expression of these molecules, all samples were divided into four clusters by Consensus clustering analysis. Clusters 2 and 4 were associated with worse survival and favorable prognosis, respectively. The proportion of patients with different age, Pathologic T and Pathologic stage among these four clusters showed significant differences. Cluster 4 was associated with a higher proportion of patients with pathological N0 and T1–2, as well as early tumors (stages I–II). Additionally, these clusters significantly differed in terms of immune infiltration of memory B cells, regulatory T cells, M2 macrophages, and activated memory CD4+ T cells, as well as the expression of six checkpoint genes. Worse survival was associated with a high rather than a low TMB; among the clusters, clusters 4 and 2 had the lowest and highest TMB, respectively. In conclusion, four co-stimulatory molecule clusters identified in NSCLC significantly differed in terms of survival, tumor stage, immune response, and TMB.
Highlights
Four co-stimulatory molecule clusters were identified that correlated differently with survival.
Feature genes of four clusters were enriched in different functions.
Four clusters significantly differed in terms of tumor stage, immune infiltration, and checkpoint gene expression.
Worse survival was associated with high, compared with low TMB.
Survival was better for cluster 4 than other clusters, and TMB was low.
Introduction
Global cancer statistics indicates that lung cancer is the most commonly diagnosed cancer, accounting for ∼11.6% of all cancers, and a major cause of ∼18.4% of all cancer-related deaths (Bray et al. Citation2018). Non-small cell lung cancer (NSCLC) is the most prevalent histological type of lung cancer, comprising (80–85%) of all lung cancers (Suster and Mino-Kenudson Citation2020). Lung squamous-cell carcinoma (LUSC) and lung adenocarcinoma (LUAD) the two most prevalent subtypes of NSCLC (Zhang et al. Citation2020b). Early diagnosis of NSCLC is difficult, and ∼66% of patients already have regional or distant metastasis at the time of diagnosis, which leads to a poor 5-year survival rate (Herbst et al. Citation2018). Tyrosine kinase inhibitors have improved therapy for patients with NSCLC carrying mutations on genes such as EGFR, NTRK, and ALK. The introduction of immune checkpoint inhibitors (ICIs) have also significantly altered the landscape of NSCLC therapy (Duma et al. Citation2019).
Immunotherapy has become a new direction of tumor therapy, as it can improve anti-tumor effects by stimulating the immune system (Anichini et al. Citation2020; Lei et al. Citation2020). Anti-tumor immunity in humans is mainly cellular immunity mediated by T cells, and specific activation to develop the immune response requires double signal stimulation, especially the second signal provided by co-stimulatory molecules (Azuma Citation2019; Schorer et al. Citation2019). Co-stimulatory molecules are a group of heterogeneous cell surface molecules that amplify or counteract T-cell receptor signals by interacting with antigen/MHC, thereby affecting T-cell differentiation and function (Magee et al. Citation2012; Nagai and Azuma Citation2019).
Programmed cell death-1 (PD-1) and its ligand PD-L1 belong to the CD28/B7 family. They function as co-stimulatory molecules, and are implicated in mediating anti-tumor immunity (Fife and Bluestone Citation2008). Immune checkpoint inhibitors targeting PD-1/ PD-L1 have revolutionized NSCLC therapy (Xia et al. Citation2019; Eguren-Santamaria et al. Citation2020). Pembrolizumab (a humanized monoclonal anti-PD1 antibody) has been approved as a first-line therapy for patients with PD-L1-positive metastatic NSCLC (Reck et al. Citation2016). A prognostic model comprising five co-stimulatory molecules can predict overall survival and immunotherapy response in LUAD (Zhang et al. Citation2020a). These studies emphasize the importance of co-stimulatory molecules in the development and immunotherapy of NSCLC. Therefore, the present study investigated associations between co-stimulatory molecules and prognosis as well as immune status, and identified molecular clusters based on immune-related prognostic co-stimulatory molecules. Survival, functions, immune microenvironment, and tumor mutation burden (TMB) were the compared among the different clusters.
Methods
Data acquisition and preprocessing
The RNA-seq data of 585 LUAD and 550 LUSC subtypes were downloaded from TCGA database, and generated on an Illumina HiSeq 2000 RNA Sequencing platform. This study included 502 LUAD and 494 LUSC samples with matched clinical phenotypes among the subtypes. Batch effects of expression data within LUAD and LUSC samples were removed using the SVA package (version 3.38.0) in R 3.6.1. Figure S1 shows the workflow of the data analysis.
Screening immune-related prognostic co-stimulatory molecules
Table S1 lists 60 co-stimulatory molecules retrieved from a previous study (Zhang et al. Citation2020a). Prognostic correlations for these co-stimulatory molecules were evaluated using univariate Cox regression analysis with P < 0.05 indicating significance. Table S2 lists immune-related genes that we retrieved from the Immport database. Genes that overlapped with immune-related genes and prognostic co-stimulatory molecules were selected as immune-related prognostic co-stimulatory molecules.
Identification of molecular clusters
Based on the expression of immune-related prognostic co-stimulatory molecules, we classified all tumor samples into clusters using Consensus Cluster Plus (version 1.54.0). Differences in survival among molecular clusters were evaluated using Kaplan-Meier curves and survival package (version 2.41–3). We also compared clinical phenotype differences among the molecular clusters.
Feature genes of molecular clusters
Differentially expressed genes (DEGs) in one cluster vs. the other clusters were screened utilizing the limma package (version 3.34.7), and DEGs with FDR <0.05 and |log2FC| >0.5 were considered as the feature genes of this cluster. The involvement of DEGs in Gene Ontology (GO) biological processes and Kyoto Encyclopedia of Genes and Genomes (KEGG) enriched pathways was determined using the Database for Annotation, Visualization and Integrated Discovery (DAVID, version 6.8). P values <0.05 were considered significantly enriched. Interactions among proteins encoded by these DEGs were analyzed utilizing the Search Tool for the Retrieval of Interacting Genes (STRING, version 11.0), protein–protein interactions (PPIs) with high confidence scores (PPI > 0.9) were retrieved, then a PPI network was visualized using Cytoscape software (version 3.6.1). Functional enrichment analysis was also carried out for genes in the PPI network.
Immune microenvironment and expression of immune checkpoints
The infiltrative abundance of 22 immune cell types in tumor samples was determined using the deconvolution CIBERSORT algorithm, and differences within molecular clusters were compared utilizing the AOV function in R 3.6.1. The 22 immune cell types contains: B cells (memory, naive and plasma B cell), macrophage (M0, M1 and M2 macrophage), T cells (Tregs, CD8+, gamma delta, helper follicular, naive CD4+, activated and resting memory CD4+ T cells), activated and resting NK cells, activated and resting myeloid dendritic cells, activated and resting mast cells, neutrophil, eosinophil and monocyte. Expression of the immune checkpoints, including PD1 (PDCD1), PD-L1 (CD274), CTLA-4 (CTLA4), CD278 (ICOS), Tim3 (HAVCR2), LAG3, CD73, CD47, BTLA, TIGIT, myd1 (SIRPA), OX40 (TNFRSF4), 4-1BB (TNFRSF9), and B7-H4 (VTCN1), was compared among different molecular clusters.
TMB
Information about mutated genes were downloaded from TCGA database, and the mutation frequency of each gene in tumor samples was analyzed. Differences in gene mutation frequency within various molecular clusters were compared using t-tests. The TMBs of all samples were calculated using the tmb function provided in maftools package (version 2.6.05) and were compared among the molecular clusters.
Results
Screening immune-related prognostic co-stimulatory molecules
Batch effects of expression data within LUAD and LUSC samples were eliminated and merged into one dataset for the following analysis (Figure A,B). Expression data for 60 co-stimulatory molecules were retrieved from the merged dataset, followed by univariate Cox regression analysis to evaluate their prognostic value. Among them, 14 co-stimulatory molecules were associated with patient survival (Table S3). Furthermore, 12 genes that overlapped 14 prognostic co-stimulatory molecules and immune-related genes were considered as immune-related prognostic co-stimulatory molecules (Table S3).
Figure 1. Identification of co-stimulatory molecules clusters. Samples of LUAD and LUSC before (A) and after (B) eliminating batch effect. C, clustering analysis resulted in four clusters based on expression of 12 immune-related prognostic co-stimulatory molecules. D, K–M, Differences in survival of patients in four clusters.
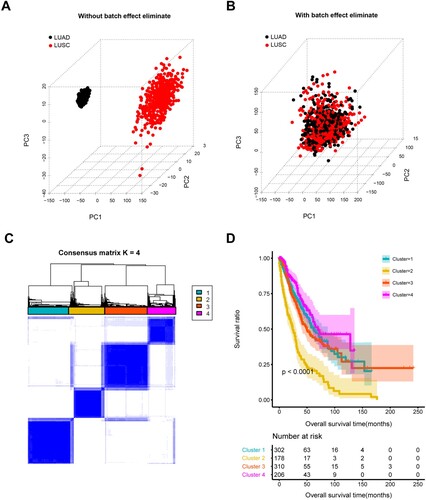
Based on the expression of these 12 immune-related prognostic co-stimulatory molecules, all 996 NSCLC samples were clustered into four molecular clusters (Figure C), and survival analysis revealed significant differences among them. Cluster 2 was associated with worse survival compared with the other clusters, and cluster 4 was associated with a favorable prognosis (Figure D). The clinical phenotypes of these four clusters were further compared (Table and Figure S2). The proportions of patients of different ages with pathological T and tumor stages significantly differed among the four clusters. Cluster 4 was associated with a higher proportion of patients with pathological N0 and T1–2, as well as early tumors (stages I–II), which might have resulted in differences in patient survival associated with these four clusters.
Table 1. Clinical characteristics within these four subtypes.
Feature genes of four molecular clusters
Genes that were differentially expressed in one cluster vs. the other three were analyzed using limma, and genes with FDR <0.05 and |log2FC|>0.5 were considered as feature genes of this cluster. We screened 188, 240, 402 and 235 feature genes for clusters 1–4, respectively (Table S4). Functional enrichment analysis revealed that the feature genes of cluster 1 were mainly enriched in drug metabolism-cytochrome P450, potassium ion transport and transmembrane transport, and epithelial cell differentiation. The feature genes of cluster 2 were mainly implicated in cytokine–cytokine receptor interaction, chemokine-mediated signaling pathway, and positive regulation of leukocyte chemotaxis. The feature genes of clusters 3 and 4 were mainly enriched in immune-related functions, such as B/T-cell receptor signaling, immune response, adaptive immune response, and B-cell differentiation (Figure ).
Network of interactions among proteins encoded by feature genes
Interactions among proteins encoded by these feature genes were analyzed using the STRING database (version 11.0), and we retrieved 1,310 interactions with PPI scores >0.9. Figure A shows the PPI network, of which GNG8 (degree = 64), GNG13 (degree = 63), SAA1 (degree = 53), CASR (degree = 52), AGTR2 (degree = 47), PENK (degree = 45), PPBP (degree = 45), PF4 (degree = 40), and CXCL8 (degree = 40) were hub genes with higher degrees in the network. We further investigated the functions of the genes in the PPI network. We found that these genes were significantly enriched in 39 biological processes, and the top 20 terms included chemokine-mediated signaling pathway, immune response, inflammatory response, and chemotaxis. Eighteen enriched KEGG pathways included cytokine–cytokine receptor interaction, chemokine signaling pathway, and neuroactive ligand–receptor interaction (Figure B,C).
Figure 3. Protein–protein interaction (PPI) network and functional enrichment. A, PPI network of feature genes in four clusters. Node size, degree of each node in network. Bar charts show 20 genes in PPI network that are significantly enriched in biological processes (B) and KEGG pathways (C). Lengths of bars, gene count in each function terms. Color change from red to blue, P values from low to high.
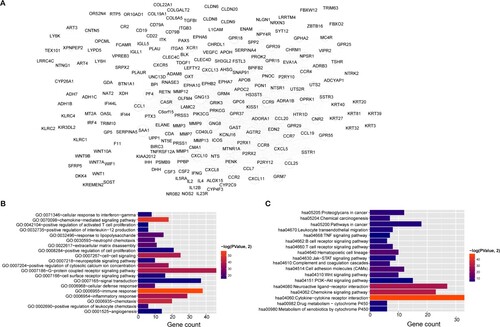
Differences in immune infiltration and checkpoint genes among four clusters
The infiltrative abundance of 22 immune cells in tumor samples was evaluated using the deconvolution CIBERSORT algorithm, and differences in this abundance were compared among the four molecular clusters. Memory B cells, regulatory T cells (Tregs), M2 macrophages, and activated memory CD4+ T cells significantly differed among the four clusters (Table S5). Compared with other clusters, cluster 4 had a relative higher abundance of tumor-infiltrating Tregs and memory B cells, and a low abundance of tumor-infiltrating M2 macrophages (Figure ).
Figure 4. Tumor-infiltrating immune cells differ among clusters. Boxplots show significant differences in tumor-infiltrating memory B cells (A), M2 macrophages (B), regulatory T cells (C), and activated memory CD4+ T cells (D) among four clusters.
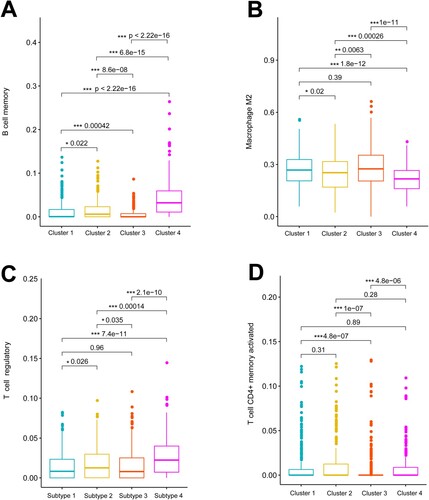
We compared the expression of 13 immune checkpoint genes among the four clusters. The checkpoint genes, CTLA4, BTLA, LAG3, PDCD1, TIGIT, and TNFRSF4, significantly differed among the four clusters. The expression of these checkpoint genes was the highest in cluster 4 and the lowest in cluster 3 (Figure ).
Differences in TMB and genes with high-frequency mutations among four clusters
Figure A shows the top 10 genes with the highest mutation frequency in tumor samples. The mutation frequency of TP53, TTN, CSMD3, MUC16 and RYR2 was high in NSCLC samples. Figure B shows that among the top 20 genes with significantly different mutation frequency within the four clusters, the mutation frequency was the highest in TP53. The mutation frequency of TP53, SYNE1, PKHD1L1 was higher in clusters 1 and 3 than in clusters 2 and 4.
Figure 6. Tumor mutation burden (TMB) and genes with high-frequency mutations among four clusters. A, Top 20 genes ranked by mutation frequency. B, Top 20 genes with significant differences in mutation frequency among four clusters, C, K–M Survival curves of patients with high and low TMB. D, significant differences in TMB among four clusters.
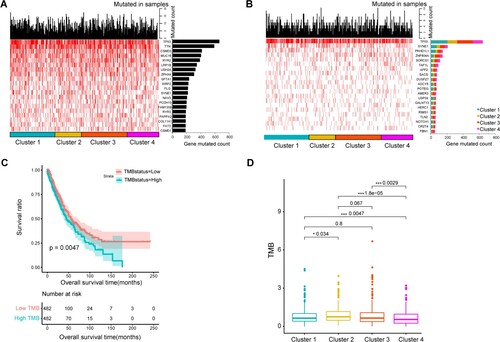
We found that survival was worse when the TMB was high (Figure C). The differences in TMB among these four clusters were further compared. The results showed that clusters 4 and 2 had the lowest highest TMB, respectively, compared with the other clusters (Figure D). This was consistent with the finding that cluster 2 was associated with worse survival than other clusters, and that cluster 4 was associated with a favorable prognosis.
Discussion
Co-stimulatory molecules are a group of heterogeneous cell surface molecules that amplify or counteract T-cell receptor signals, which play crucial roles in mediating T-cell function and anti-tumor immune responses (Capece et al. Citation2012). Immune checkpoint inhibitors targeting these molecules have been regarded as promising adjunctive cancer immunotherapy (Yang et al. Citation2020; Ye L et al. Citation2020). Recent studies have demonstrated the important roles of co-stimulatory molecules in predicting prognosis risk and immunotherapy response in various tumors, such as LUAD (Zhang et al. Citation2020a), clear cell renal cell carcinoma (Hua et al. Citation2021), prostate cancer (Ge et al. Citation2021), head and neck squamous cell carcinoma (Aye et al. Citation2021). Therefore, co-stimulatory molecules were scientifically investigated in NSCLC in this study.
Among the 60 co-stimulatory molecules expressed in NSCLC, 14 co-stimulatory molecules were found to be associated with survival of patients, of which 12 co-stimulatory molecules were immune-related, including TNFRSF12A, TNFRSF13C, TNFRSF1A, TNFRSF19, TNFRSF10D, TNFRSF11A, TNFRSF17, TNFSF18, TNFRSF13B, TNFSF9, CD70 (also named TNFRSF5), and CD40LG (also named TNFSF5). These 12 co-stimulatory molecules belong to the TNF/TNFR superfamily. Co-stimulatory signals initiated by interaction within TNF/TNFR contribute to the clonal expansion, differentiation, and survival of antigen-primed CD4+ and CD8+ T cells, and play important roles in sustaining T-cell responses after initial T-cell activation (Watts Citation2005; So and Ishii Citation2019). Interactions with the TNF/TNFR superfamily can affect T-cell responses in various ways, such as affecting inflammation and innate immunity, activation of antigen-presenting cells, lymphoid organization, and providing direct signals to T cells (Watts Citation2005). For example, CD70 acts as a co-stimulator to regulate the survival of tumor cells and Tregs and contributes to the expansion and differentiation of T cells by interacting with its ligand, CD27 (Jacobs et al. Citation2015). Elevated CD70 expression correlates with immune evasion in tumor cells; CD70–CD27 interactions can increase the malignant phenotypes of malignant mesothelioma of the pleura and decrease the anti-tumor immune response of patients (Inaguma et al. Citation2020). Cisplatin when combined with anti-CD70 significantly decreases tumor cell survival in NSCLC (Jacobs et al. Citation2017).
Four clusters were identified based on the expression of these 12 immune-related prognostic co-stimulatory molecules. Cluster 2 was associated with worse survival than other clusters, and cluster 4 was associated with a favorable prognosis. We investigated possible reasons for these differences in patient survival by analyzing associations between clusters and clinical phenotypes, the functions of feature genes, immune infiltration, and the TMB. Comparisons of clinical phenotypes revealed a higher proportion of patients with pathologic N0 and pathologic T1–2 tumors and early tumors (stage I–II) in cluster 4, which might have affected the survival of patients in this cluster. The feature genes of cluster 4 were mainly enriched in immune-related functions, such as B/T-cell receptor signaling pathway, immune response, adaptive immune response, and B-cell differentiation. Consistently, immune infiltration analysis associated cluster 4 with a relatively higher abundance of tumor-infiltrating Tregs and memory B cells and a lower abundance of tumor-infiltrating M2 macrophages than the other clusters. Regulatory T cells are defined as immunosuppressive cells that prevent overt immune responses and autoimmunity, whereas aberrant infiltration of Tregs into tumors contributes to establishing an immunosuppressive microenvironment by inhibiting immune surveillance and thus promoting tumor progression (Sasidharan Nair and Elkord Citation2018; Pacella et al. Citation2020). Tumor-associated macrophages can be separated into different subsets with different functions, namely, anti-tumor M1 and pro-tumor M2 phenotypes. The different polarization states between M1/M2 macrophage phenotypes on tumors directly affect antitumor immune responses. A decrease in the ratio of M1/M2 phenotypes correlates with tumor progression (Wu et al. Citation2020). Tumors with macrophage polarization might convert tumor-associated macrophages from the pro-tumor M2 phenotype towards the anti-tumor M1 phenotype, thus generating more beneficial immunological responses (Cao et al. Citation2019; Reichel et al. Citation2019). Although the TMB seemed to correlate positively with the survival of patients treated with ICI, TMB cutoffs significantly varies among different tumor types (Altz et al. Citation2019). An elevated TMB of circulating NSCLC tumor DNA is associated with poor patient survival (Chae et al. Citation2019). Our results were consistent with this insofar as we also associated a high TMB with worse survival than a low TMB. Clusters 4 and 2 had the lowest and highest TMBs among the four clusters, respectively. This result was also consistent with the finding that cluster 2 was associated with worse survival than the other clusters, and that the prognosis of cluster 4 was favorable.
In conclusion, we screened 12 immune-related prognostic co-stimulatory molecules, and identified four molecule clusters. Cluster 2 was associated with worse survival than other clusters, and cluster 4 was associated with a favorable prognosis. The four clusters significantly differed in terms of tumor stage, immune response and TMB. Our findings should help to provide potential biomarkers of the immune response to treatment for NSCLC, and to develop personalized treatment strategies by stratifying patients with NSCLC.
Authors’ contributions
Study concept and design, QB and CW; data acquisition, QY, WS, KZ, YL, and WG; data analysis and interpretation, QY, WS, KZ, YL, and WG; statistical analysis, QY, WS, KZ, YL, and WG; drafting the manuscript, CW; revision of manuscript for important intellectual content, QB. All authors read and approved the final version of the manuscript.
Supplemental Material
Download Zip (2.5 MB)Disclosure statement
No potential conflict of interest was reported by the author(s).
Data availability statement
The data that support the findings of this study are available in The Cancer Genome Atlas (TCGA) database at https://gdc-portal.nci.nih.gov/, including the standardized log (FPKM+1, 2) expression data, clinical phenotype and mutation data of lung adenocarcinoma (TCGA-LUAD) and lung squamous carcinoma (TCGA-LUSC).
References
- Altz LSZ, Hi S, Baselga J, Razavi P, Ca K, Yaeger R, Nh S, Gy K, Rp D, Ladanyi M, et al. 2019. Tumor mutational load predicts survival after immunotherapy across multiple cancer types. Nat Genet. 51:202–206.
- Anichini A, Perotti VE, Sgambelluri F, Mortarini R. 2020. Immune escape mechanisms in non small cell lung cancer. Cancers (Basel). 12:3605.
- Aye L, Song X, Yang J, Hu L, Sun X, Zhou J, Liu Q, Yu H, Wang D. 2021. Identification of a costimulatory molecule gene signature to predict survival and immunotherapy response in head and neck squamous cell carcinoma. Front Cell Dev Biol. 9:695533.
- Azuma M. 2019. Co-signal molecules in T-cell activation: historical overview and perspective. Adv Exp Med Biol. 1189:3–23.
- Bray F, Ferlay J, Soerjomataram I, Siegel RL, Torre LA, Jemal A. 2018. Global cancer statistics 2018: GLOBOCAN estimates of incidence and mortality worldwide for 36 cancers in 185 countries. CA Cancer J Clin. 68:394–424.
- Cao M, Yan H, Han X, Weng L, Wei Q, Sun X, Lu W, Wei Q, Ye J, Cai X, et al. 2019. Ginseng-derived nanoparticles alter macrophage polarization to inhibit melanoma growth. J Immunother Cancer. 7:326.
- Capece D, Verzella D, Fischietti M, Zazzeroni F, Alesse E. 2012. Targeting costimulatory molecules to improve antitumor immunity. J Biomed Biotechnol. 2012:926321.
- Chae YK, Davis AA, Agte S, Pan A, Simon NI, Iams WT, Cruz MR, Tamragouri K, Rhee K, Mohindra N, et al. 2019. Clinical implications of circulating tumor DNA tumor mutational burden (ctDNA TMB) in non-small cell lung cancer. Oncologist. 24:820–828.
- Duma N, Santana-Davila R, Molina JR. 2019. Non-Small cell lung cancer: epidemiology, screening, diagnosis, and treatment. Mayo Clin Proc. 94:1623–1640.
- Eguren-Santamaria I, Sanmamed MF, Goldberg SB, Kluger HM, Idoate MA, Lu BY, Corral J, Schalper KA, Herbst RS, Gil-Bazo I. 2020. PD-1/PD-L1 blockers in NSCLC brain metastases: challenging paradigms and clinical practice. Clin Cancer Res. 26:4186–4197.
- Fife BT, Bluestone JA. 2008. Control of peripheral T-cell tolerance and autoimmunity via the CTLA-4 and PD-1 pathways. Immunol Rev. 224:166–182.
- Ge S, Hua X, Chen J, Xiao H, Zhang L, Zhou J, Liang C, Tai S. 2021. Identification of a costimulatory molecule-related signature for predicting prognostic risk in prostate cancer. Front Genet. 12:666300.
- Herbst RS, Morgensztern D, Boshoff C. 2018. The biology and management of non-small cell lung cancer. Nature. 553:446–454.
- Hua X, Ge S, Zhang J, Xiao H, Tai S, Yang C, Zhang L, Liang C. 2021. A costimulatory molecule-related signature in regard to evaluation of prognosis and immune features for clear cell renal cell carcinoma. Cell Death Discov. 7:252.
- Inaguma S, Lasota J, Czapiewski P, Langfort R, Rys J, Szpor J, Waloszczyk P, Okoń K, Biernat W, Schrump DS, et al. 2020. CD70 expression correlates with a worse prognosis in malignant pleural mesothelioma patients via immune evasion and enhanced invasiveness. J Pathol. 250:205–216.
- Jacobs J, Deschoolmeester V, Rolfo C, Zwaenepoel K, Van Den Bossche J, Deben C, Silence K, De Haard H, Hermans C, Rottey S, et al. 2017. Preclinical data on the combination of cisplatin and anti-CD70 therapy in non-small cell lung cancer as an excellent match in the era of combination therapy. Oncotarget. 8:74058–74067.
- Jacobs J, Deschoolmeester V, Zwaenepoel K, Rolfo C, Silence K, Rottey S, Lardon F, Smits E, Pauwels P. 2015. CD70: an emerging target in cancer immunotherapy. Pharmacol Ther. 155:1–10.
- Lei X, Lei Y, Li JK, Du WX, Li RG, Yang J, Li J, Li F, Tan HB. 2020. Immune cells within the tumor microenvironment: biological functions and roles in cancer immunotherapy. Cancer Lett. 470:126–133.
- Magee CN, Boenisch O, Najafian N. 2012. The role of costimulatory molecules in directing the functional differentiation of alloreactive T helper cells. Am J Transplant. 12:2588–2600.
- Nagai S, Azuma M. 2019. The CD28-B7 family of co-signaling molecules. Adv Exp Med Biol. 1189:25–51.
- Pacella I, Grimaldi A, Piconese S. 2020. Assessment of lipid load in tumor-infiltrating Tregs by flow cytometry. Methods Enzymol. 632:283–294.
- Reck M, Rodríguez-Abreu D, Robinson AG, Hui R, Csőszi T, Fülöp A, Gottfried M, Peled N, Tafreshi A, Cuffe S, et al. 2016. Pembrolizumab versus chemotherapy for PD-L1-positive non-small-cell lung cancer. N Engl J Med. 375:1823–1833.
- Reichel D, Tripathi M, Perez JM. 2019. Biological effects of nanoparticles on macrophage polarization in the tumor microenvironment. Nanotheranostics. 3:66–88.
- Sasidharan Nair V, Elkord E. 2018. Immune checkpoint inhibitors in cancer therapy: a focus on T-regulatory cells. Immunol Cell Biol. 96:21–33.
- Schorer M, Kuchroo VK, Joller N. 2019. Role of co-stimulatory molecules in T helper cell differentiation. Adv Exp Med Biol. 1189:153–177.
- So T, Ishii N. 2019. The TNF-TNFR family of co-signal molecules. Adv Exp Med Biol. 1189:53–84.
- Suster DI, Mino-Kenudson M. 2020. Molecular pathology of primary non-small cell lung cancer. Arch Med Res. 51:784–798.
- Watts TH. 2005. TNF/TNFR family members in costimulation of T cell responses. Annu Rev Immunol. 23:23–68.
- Wu K, Lin K, Li X, Yuan X, Xu P, Ni P, Xu D. 2020. Redefining tumor-associated macrophage subpopulations and functions in the tumor microenvironment. Front Immunol. 11:1731.
- Xia L, Liu Y, Wang Y. 2019. PD-1/PD-L1 blockade therapy in advanced non-small-cell lung cancer: current status and future directions. Oncologist. 24:S31–s41.
- Yang S, Wei W, Zhao Q. 2020. B7-H3, a checkpoint molecule, as a target for cancer immunotherapy. Int J Biol Sci. 16:1767–1773.
- Ye L JK, Wang L, Li W, Chen B, Liu Y, Wang H, Zhao S, He Y, Zhou C. 2020. CD137, an attractive candidate for the immunotherapy of lung cancer. Cancer Sci. 111:1461–1467.
- Zhang C, Zhang Z, Sun N, Zhang Z, Zhang G, Wang F, Luo Y, Che Y, He J. 2020a. Identification of a costimulatory molecule-based signature for predicting prognosis risk and immunotherapy response in patients with lung adenocarcinoma. Oncoimmunology. 9:1824641.
- Zhang L, Chen J, Cheng T, Yang H, Li H, Pan C. 2020b. Identification of the key genes and characterizations of tumor immune microenvironment in lung adenocarcinoma (LUAD) and lung squamous cell carcinoma (LUSC). J Cancer. 11:4965–4979.